1. Multimodal Data Integration
AI systems can fuse large volumes of heterogeneous data—from electronic health records (EHRs) to imaging, genomics, labs, and lifestyle metrics—thereby creating richer patient profiles that improve the accuracy of outcome predictions.
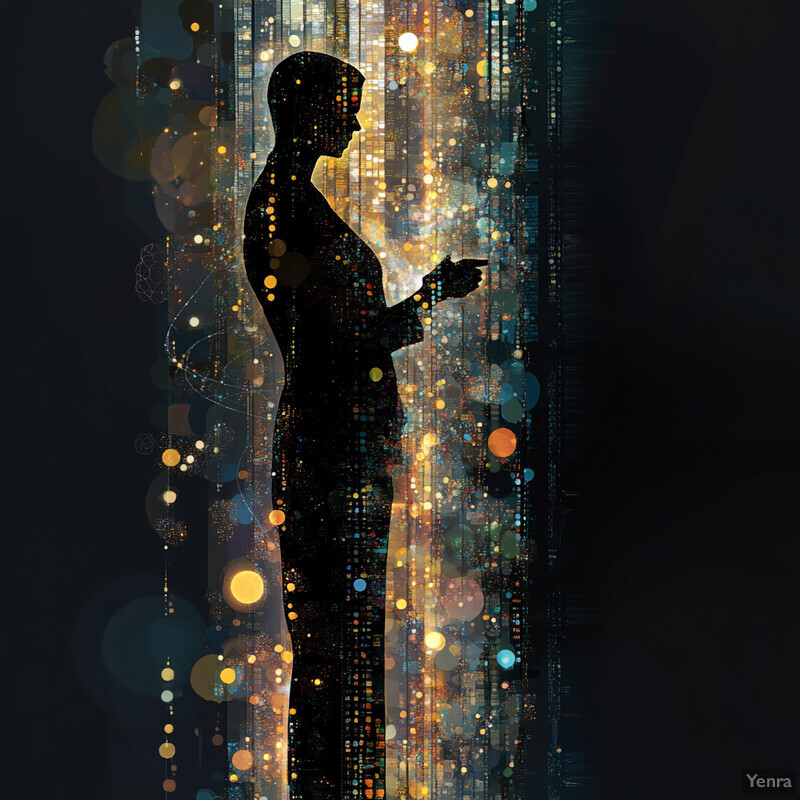
AI-driven models excel at integrating data from multiple sources—such as electronic health records (EHRs), medical imaging, laboratory tests, genomic profiles, and even wearable device outputs—into a single, cohesive patient representation. By doing so, these models can capture complex clinical scenarios that may not be evident when looking at data streams individually. For example, combining a patient’s genetic predispositions, imaging findings, and recent lab results may reveal nuanced prognostic patterns. The ability to interpret and correlate these diverse data types enables more precise predictions about disease trajectories and treatment effectiveness. Ultimately, this comprehensive view of patient health provides clinicians with more reliable guidance, helping them make informed decisions that improve long-term patient outcomes.
2. Prognostic Risk Stratification
Advanced machine learning models help clinicians stratify patients into risk categories for future events such as heart attacks, strokes, or hospital readmissions, allowing for targeted interventions.
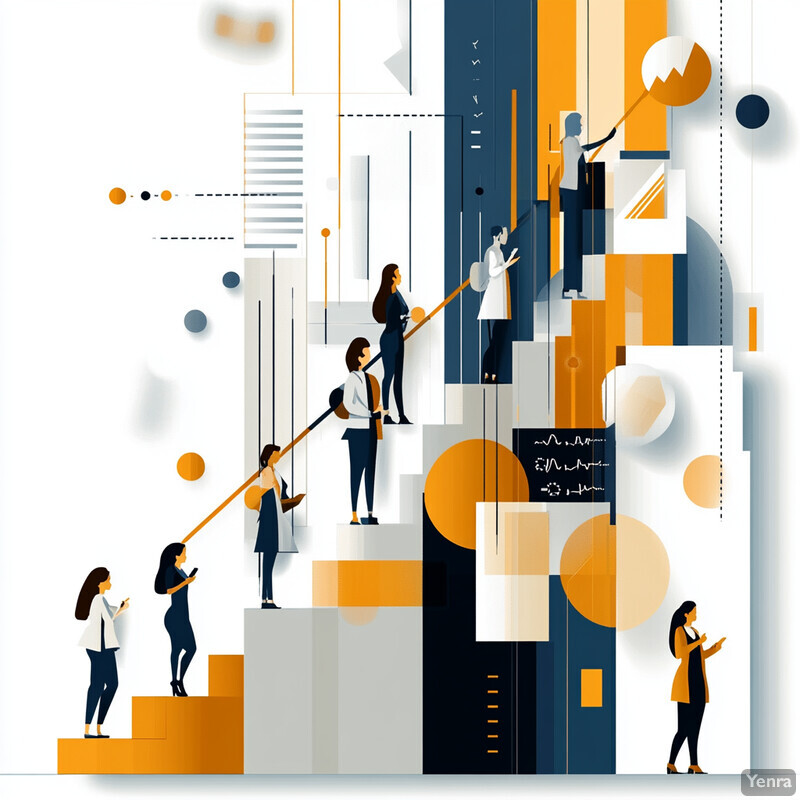
In traditional healthcare models, patients are often grouped by broad diagnoses or demographic categories. AI-driven prognostic risk stratification goes further by using machine learning algorithms to cluster patients into risk groups based on subtle clinical indicators and historical outcomes. These models weigh numerous risk factors, such as past hospitalizations, medication adherence, and specific biomarkers, to create individualized risk scores. With these risk profiles, clinicians can prioritize high-risk patients for more intensive monitoring, preventive treatments, or early interventions. Consequently, patients receive care that is proportionate to their predicted risk, which leads to more efficient resource utilization and better overall patient safety.
3. Disease Progression Modeling
Time-series analysis and deep learning models track subtle clinical signs over time, predicting how diseases like cancer, diabetes, or neurodegenerative disorders will advance, and when interventions may be most effective.
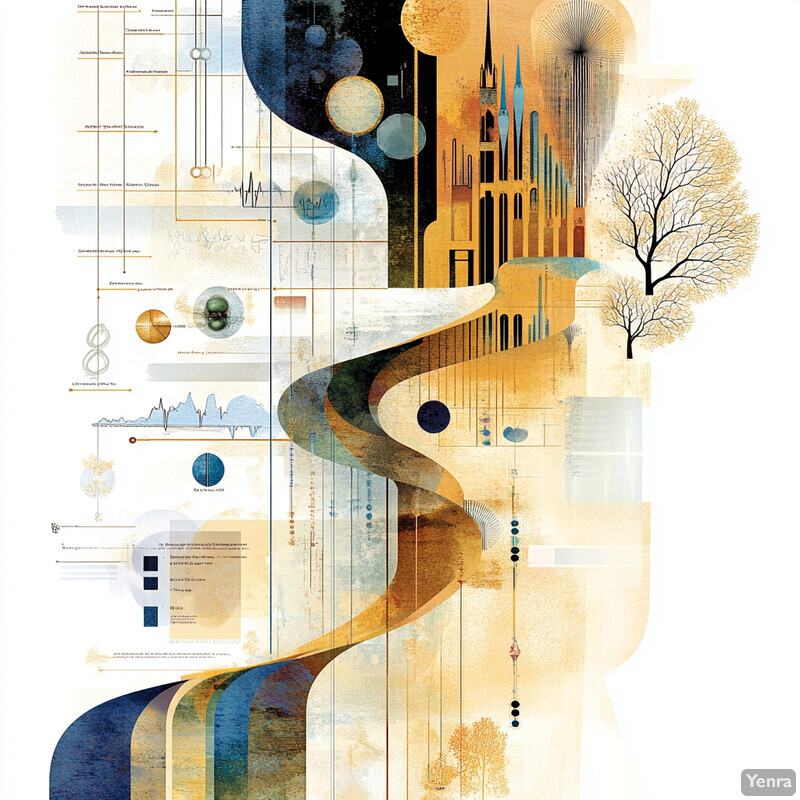
Machine learning models, particularly those using temporal or time-series data, help track a patient’s clinical journey over days, months, or even years. They can identify patterns indicative of disease progression that may be too subtle or complex for human clinicians to detect. For instance, in chronic conditions like diabetes or heart failure, AI can map out progression trajectories, signaling when a patient’s condition might worsen or when complications are likely to arise. These insights allow healthcare providers to intervene earlier with lifestyle changes, medication adjustments, or more aggressive therapies at the precise moments they will be most beneficial. Such anticipatory care can slow disease advancement and improve patients’ quality of life.
4. Personalized Treatment Response Prediction
Predictive models incorporate individual patient characteristics—genetic markers, comorbidities, or past treatment responses—to forecast how well a patient might respond to specific therapies.
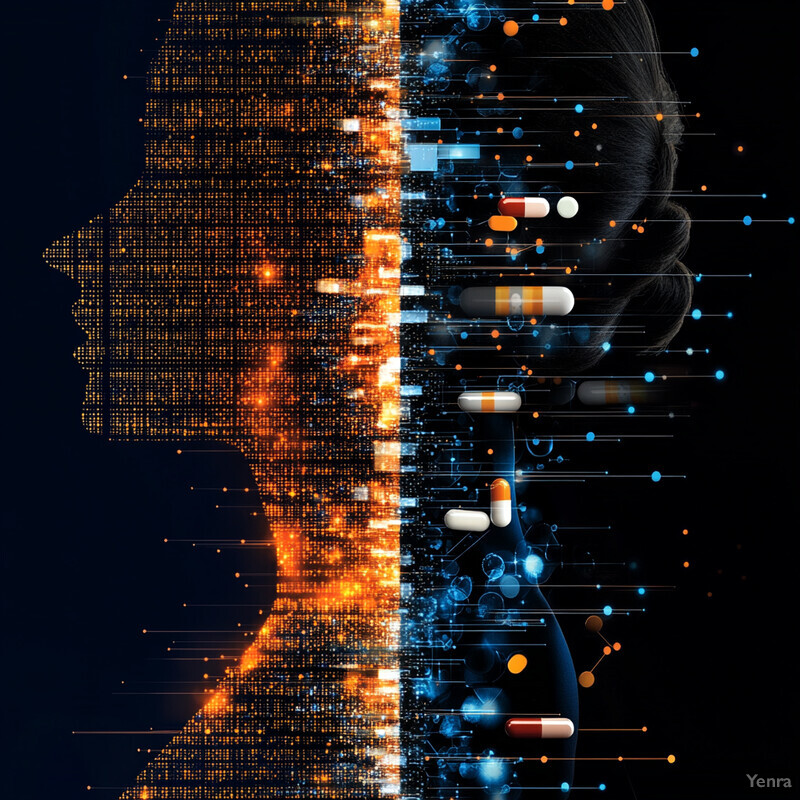
Not all patients respond to the same treatment in the same way. AI models leverage individual data—such as genetic variants, previous drug responses, lifestyle factors, and comorbidities—to predict how a particular patient might react to a specific medication or therapeutic procedure. By estimating likely treatment outcomes before commencing therapy, clinicians can reduce the trial-and-error period often associated with complex conditions. This personalized insight allows for more tailored care plans, selecting the treatments that promise the best efficacy and the fewest side effects. In doing so, patient comfort and satisfaction improve, along with clinical success rates.
5. Early Detection of Clinical Deterioration
By continuously monitoring vital signs and lab values, AI models can anticipate patient deterioration or sepsis onset hours before conventional clinical signs emerge, improving survival rates.
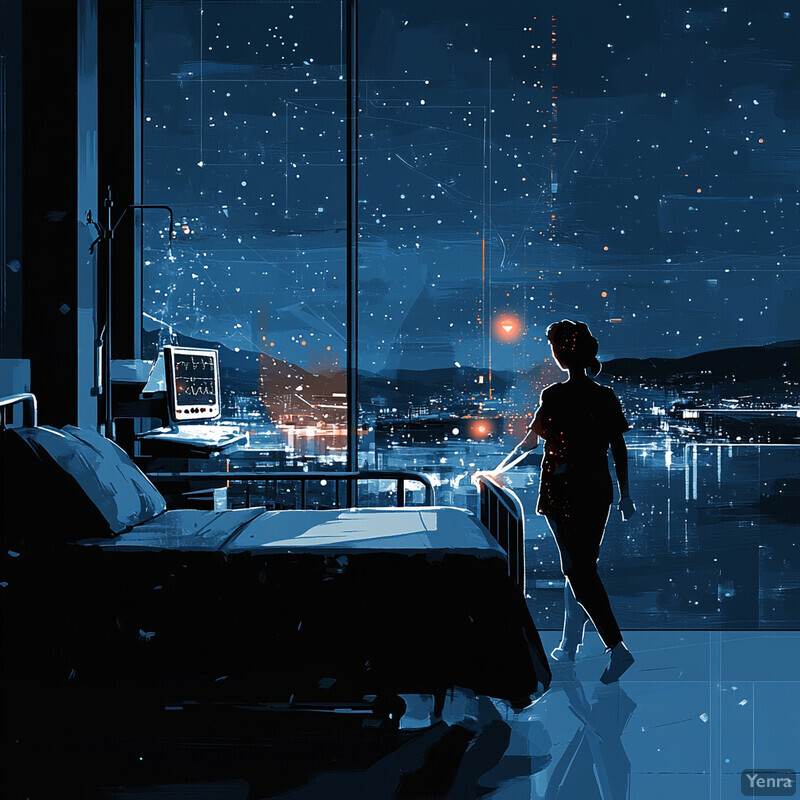
Constant streams of vital signs, lab results, and nurse assessments can overwhelm clinicians, making it difficult to spot early indicators of deterioration. AI algorithms excel at identifying patterns that suggest impending crises—such as sepsis, acute respiratory distress, or cardiac instability—often hours before traditional methods. By alerting healthcare professionals to subtle warning signs, these systems enable timely interventions that can stabilize patients, prevent ICU admissions, and significantly reduce mortality rates. This proactive approach to patient care turns reactive “firefighting” into methodical prevention, ultimately improving clinical outcomes and saving lives.
6. Imaging-Based Predictive Analytics
Deep learning applied to medical imaging (e.g., MRI, CT, X-ray) can spot subtle patterns indicative of disease progression, long-term complications, or treatment outcomes.
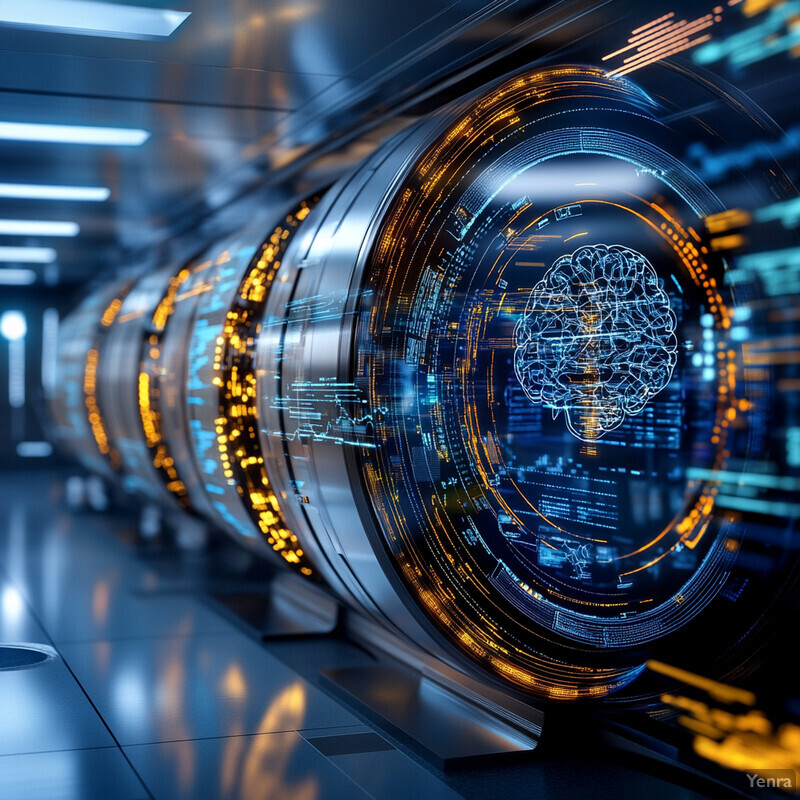
Medical imaging modalities—like X-ray, CT, and MRI—capture a wealth of information about a patient’s internal structures. AI-driven image analysis tools can detect anomalies too subtle for even highly trained radiologists. Beyond detection, these tools can also predict patient outcomes based on imaging-derived features. For instance, certain patterns in tumor shape, density, or growth rate might indicate how likely it is to spread or respond to treatment. By quantifying these patterns, the algorithm can forecast patient prognoses and guide oncologists toward more effective treatment plans. This imaging-based predictive capability enhances diagnostic precision and paves the way for truly personalized radiological assessments.
7. Natural Language Processing (NLP) for Unstructured Data
NLP algorithms analyze physician notes, discharge summaries, and clinical narratives to extract risk factors and prognostic indicators previously hidden in text-based data.
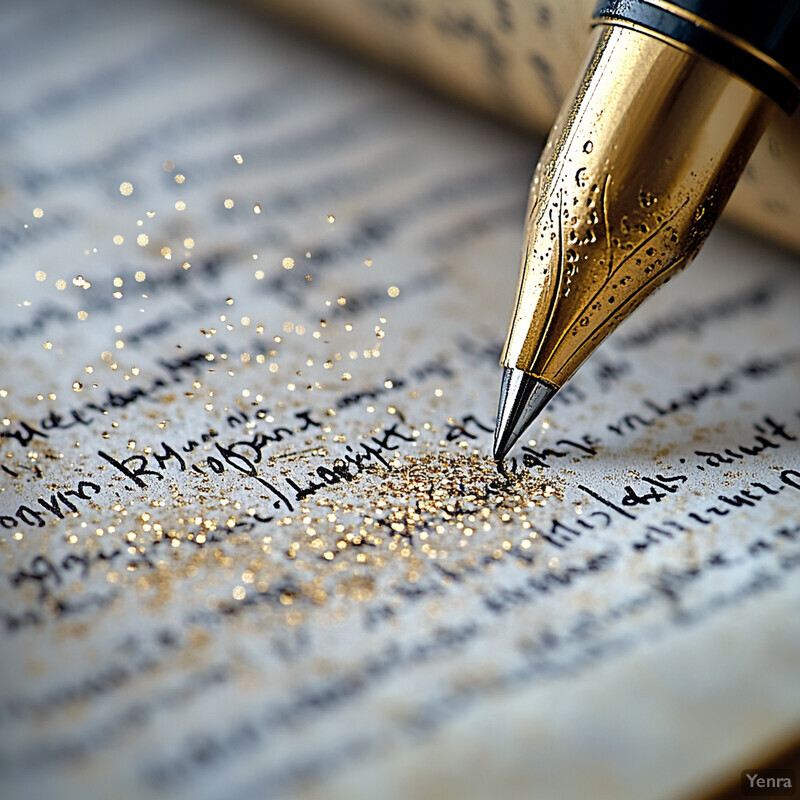
A significant portion of valuable clinical information is contained in free-text fields—physician notes, discharge summaries, and patient narratives—rather than in structured data forms. NLP algorithms convert these complex, narrative data sources into structured insights by identifying relevant medical concepts, symptoms, timelines, and risk factors. By mining and interpreting these hidden details, AI can build more complete patient profiles and derive robust prognostic indicators that might otherwise go unnoticed. The result is a richer, more contextual understanding of patient health, leading to improved accuracy in predicting outcomes and tailoring treatment plans.
8. Predicting Length of Hospital Stay
With models that consider patient severity, comorbid conditions, and procedural complexity, hospitals can more accurately predict length of stay, improving resource allocation and discharge planning.
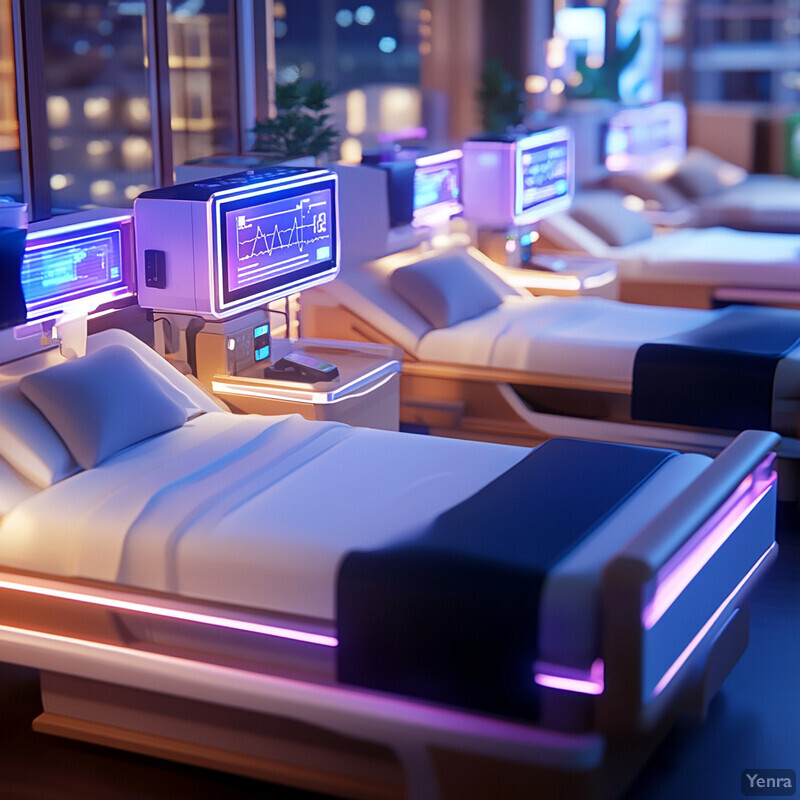
Accurate predictions of hospital length of stay (LOS) are critical for operational efficiency and resource management. AI models that incorporate patient complexity, comorbidities, severity of illness, planned procedures, and institutional factors can forecast LOS with remarkable precision. By knowing which patients are likely to stay longer, hospital administrators can optimize bed assignments, plan staffing schedules, and reduce bottlenecks in critical areas. Clinically, understanding LOS predictions can help guide discharge planning and ensure that patients receive adequate post-discharge support, reducing the risk of readmissions and improving overall care quality.
9. Anticipating Readmissions
AI-driven predictive analytics identify patients likely to be readmitted due to post-discharge complications, guiding follow-up care plans and preventive measures.
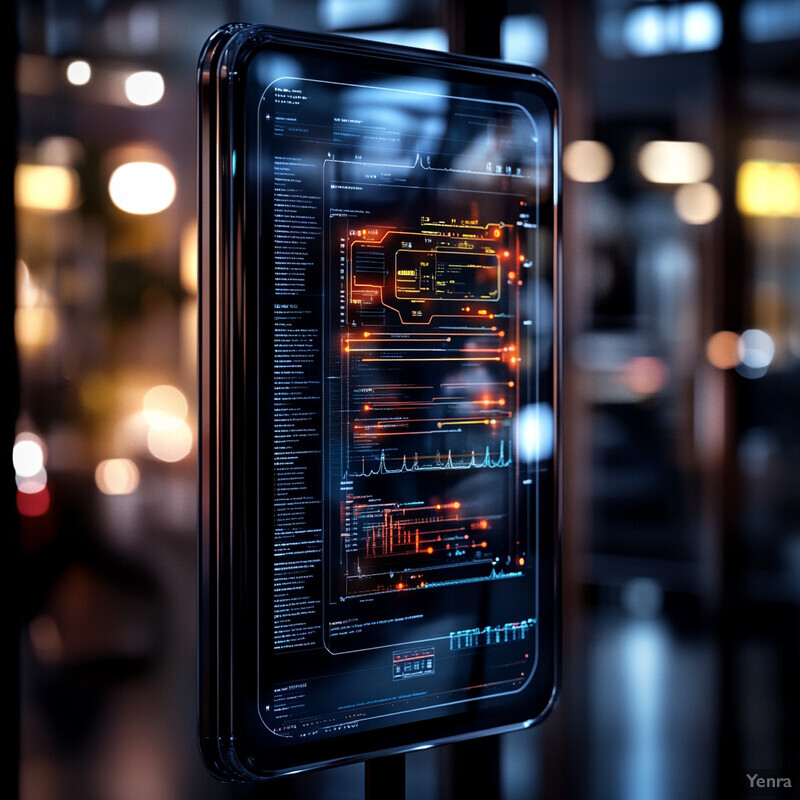
Hospital readmissions pose both clinical and financial challenges, reflecting gaps in continuity of care. AI-driven predictive models analyze a wide range of patient-specific data—medications, follow-up appointments, social determinants, and past hospitalization patterns—to identify which patients have a high risk of returning to the hospital. Armed with this foresight, care teams can implement targeted interventions, such as more comprehensive discharge instructions, medication reconciliations, or home health visits, to prevent unnecessary readmissions. This proactive approach helps maintain patient health after discharge and alleviates the burden on healthcare systems.
10. Guiding Surgical Outcomes
Machine learning models predict outcomes such as postoperative complications, mortality risk, or rehabilitation timelines, supporting better informed surgical decision-making and patient counseling.
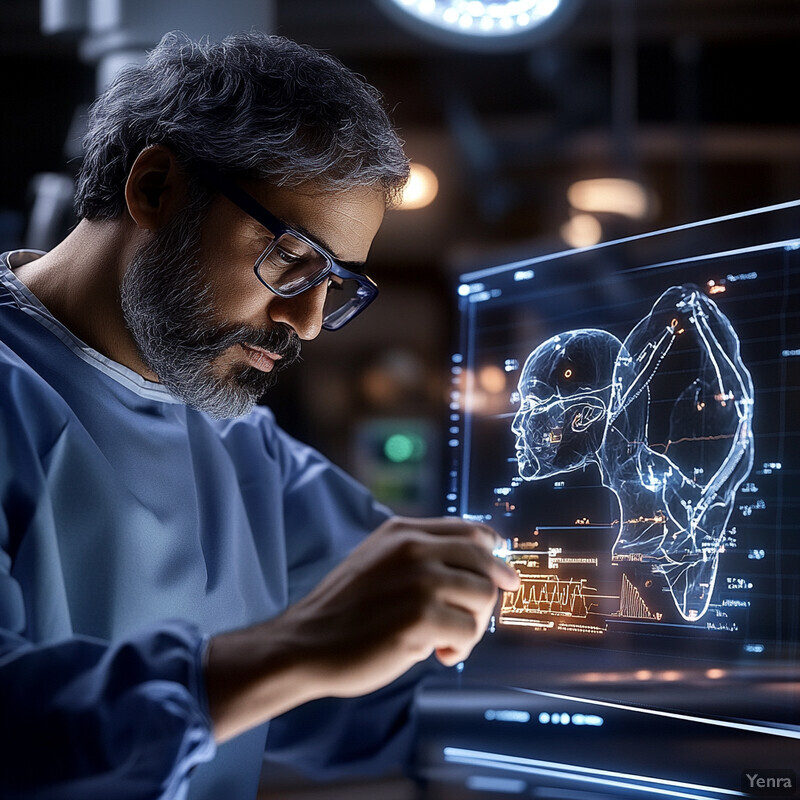
Predicting surgical outcomes involves assessing numerous factors: patient frailty, comorbidities, the complexity of the procedure, and even the surgeon’s experience. AI models integrating all these elements can project likely postoperative complications, patient recovery times, and overall prognosis. These predictions assist surgeons and anesthesiologists in surgical planning, inform patients about risks and expected recovery timelines, and enable more tailored perioperative care. Ultimately, improved accuracy in surgical outcome prediction leads to better patient counseling, more efficient resource use in the operating room, and reduced complication rates.
11. Chemotherapy and Radiotherapy Response Prediction
By analyzing biomarkers, tumor genetics, and imaging data, AI can predict which patients are more likely to benefit from certain oncology treatments and at what doses.
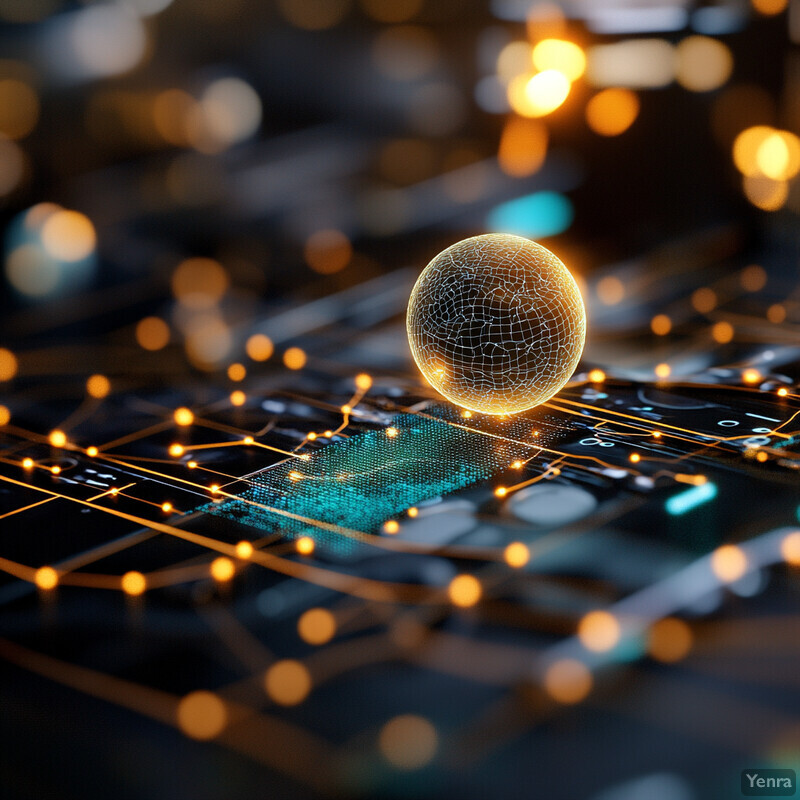
Oncologists face challenges in choosing the right treatment regimen for cancer patients, as responses can vary widely. AI models trained on large datasets of treatment outcomes, genomic profiles, and tumor characteristics can help predict which patients will benefit most from a certain chemotherapy combination or radiation therapy dose. Identifying likely responders enables clinicians to avoid over-treatment, minimize toxicity, and deliver the most effective therapy upfront. This reduces trial-and-error in cancer care, spares patients from unnecessary side effects, and improves the overall success rates of oncological treatments.
12. Pharmacogenomic Insights
Integrating genetic information with clinical and environmental data helps predict which medications will be most effective and least likely to produce adverse effects in individual patients.
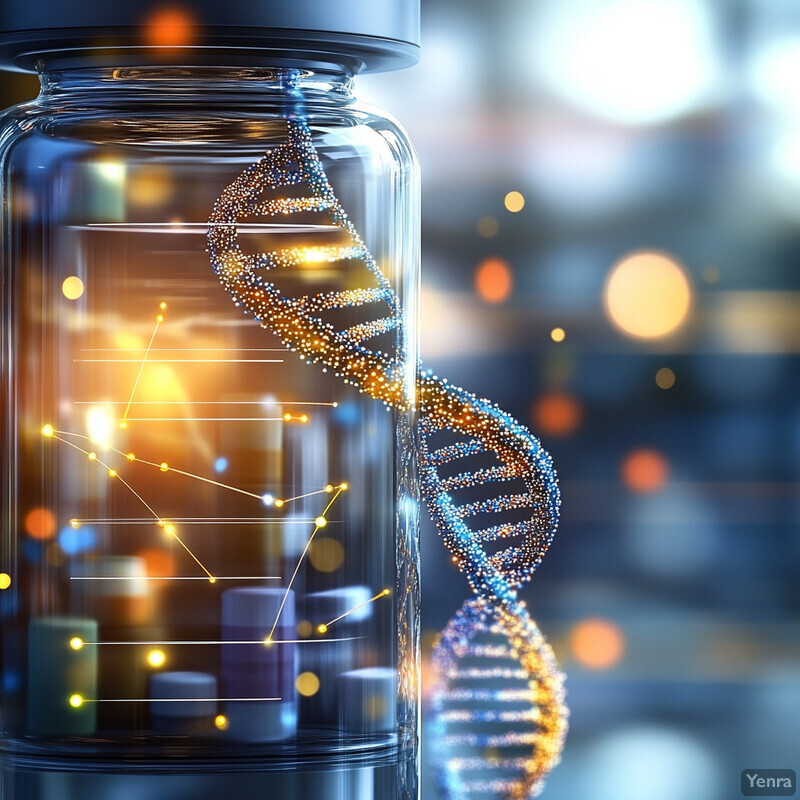
The integration of genetic data into predictive models allows AI to suggest which medications will be best suited to an individual patient’s genetic makeup. By understanding how genetic variants affect drug metabolism and efficacy, clinicians can anticipate which treatments will work optimally and which might cause adverse reactions. This approach streamlines medication selection, shortens time-to-effectiveness, and lowers the risk of severe drug-related complications. As a result, personalized pharmacotherapy guided by AI-based pharmacogenomic insights enhances medication safety and ensures patients receive the most beneficial therapies.
13. Chronic Disease Management
AI-based predictive tools can forecast flare-ups, progression, or complications in chronic conditions (e.g., heart failure, COPD) enabling proactive care and reduced hospitalizations.
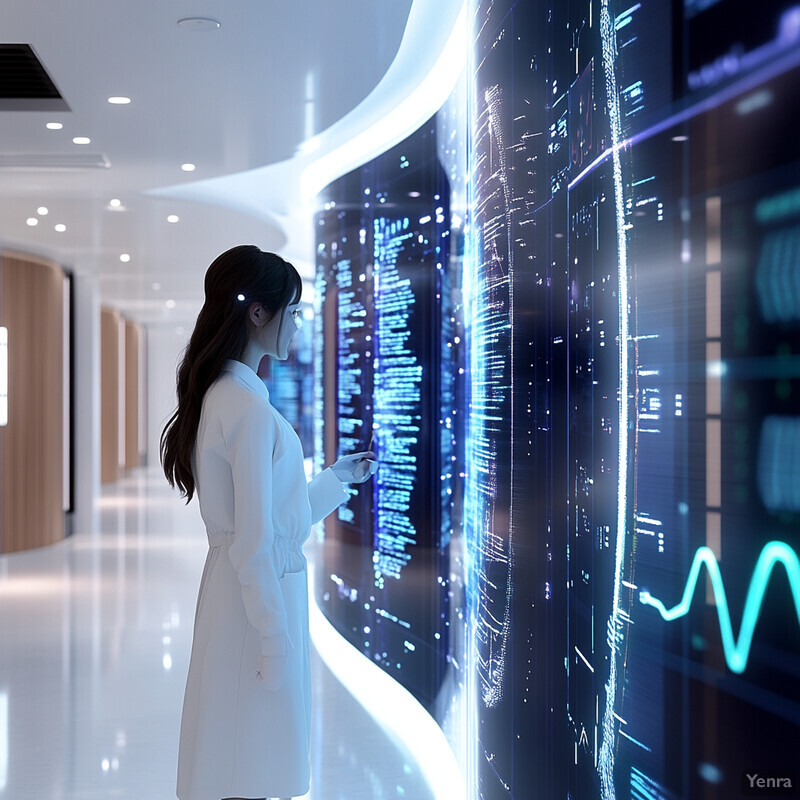
Chronic conditions—such as diabetes, heart failure, and chronic obstructive pulmonary disease—require long-term oversight to prevent complications. AI models incorporating continuous patient data (e.g., remote monitoring devices, lab values, and health app inputs) can predict when a patient’s condition is likely to worsen. With this foresight, clinicians can adjust medications, recommend lifestyle changes, or arrange specialist visits before a serious event occurs. This proactive management approach helps maintain patient stability, lowers hospitalization rates, and improves patient independence and quality of life.
14. Patient-Reported Outcome Integration
Models incorporating wearable sensor data, home monitoring devices, and patient-reported outcomes can predict deterioration or improvement in real-world settings, beyond the clinical encounter.
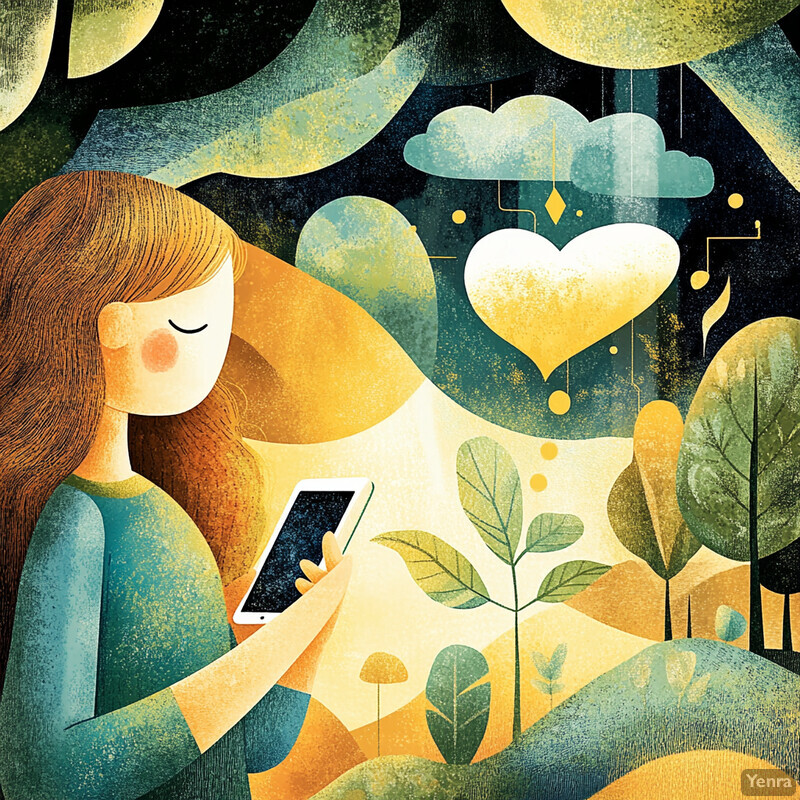
Patient-reported outcomes (PROs) offer a valuable perspective on a patient’s health status outside the clinical setting, capturing pain levels, mood, mobility, and more. AI models that analyze this data can correlate patient experiences with clinical indicators and predict deterioration or improvement trends. By factoring in these lived experiences, healthcare providers gain a more holistic understanding of patient health, beyond what objective metrics can tell them. As a result, treatment plans become more patient-centric, addressing symptoms that matter most to patients and boosting treatment adherence and satisfaction.
15. Enhanced Survival Analysis
Advanced statistical and deep learning models refine survival curves, giving clinicians and patients a clearer timeline and probability of disease recurrence, remission, or mortality.
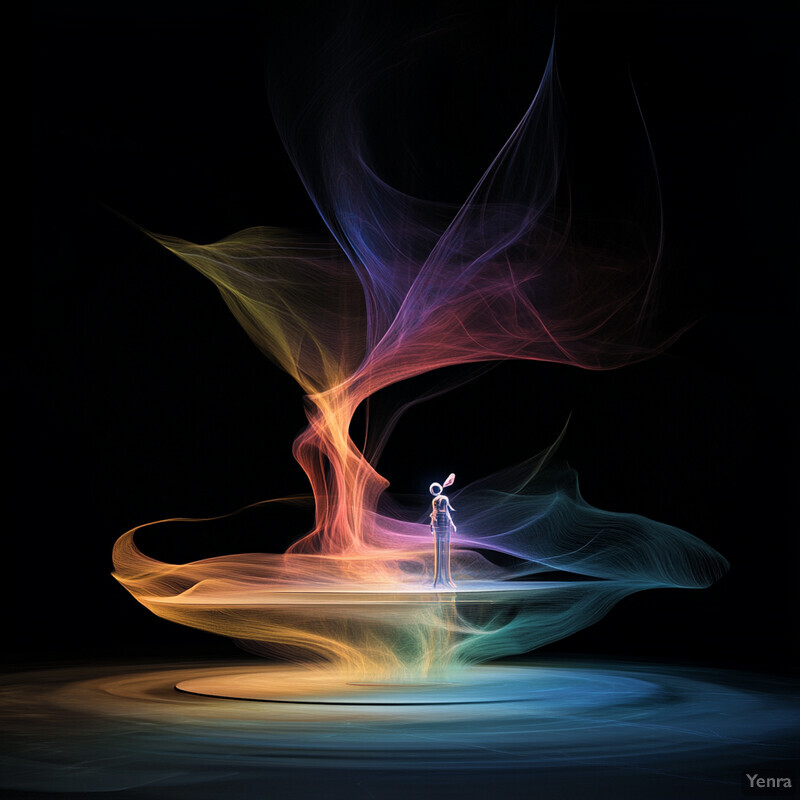
Traditional survival analysis methods can be limited in handling complex, high-dimensional data. AI-powered survival models leverage machine learning techniques to incorporate a wide range of patient attributes—demographics, treatment history, biomarkers, and imaging features—to produce more accurate survival curves. These predictions help clinicians understand a patient’s probable disease course and long-term prognosis. Improved survival analysis informs better clinical decision-making, helps guide end-of-life care discussions, and ensures that patients and families have realistic expectations about outcomes and timelines.
16. Improved Diagnostic Accuracy for Rare Diseases
AI can detect and predict outcomes in patients with rare conditions by identifying complex, uncommon patterns in large datasets, ultimately guiding more precise interventions.
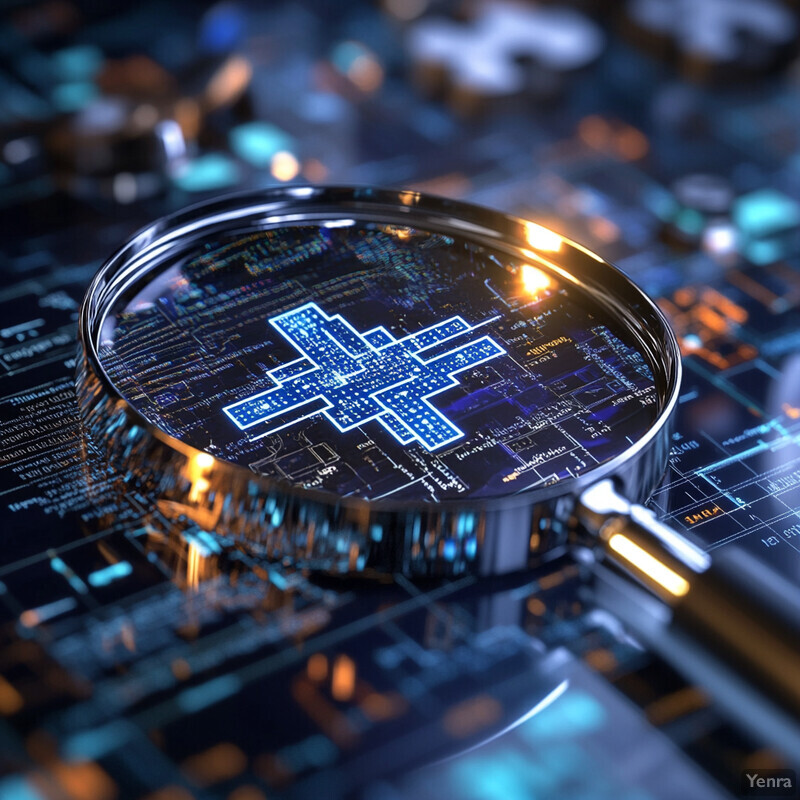
Rare diseases often lack well-established diagnostic criteria, making early detection and outcome prediction challenging. AI models trained on large, diverse datasets can spot subtle clinical patterns indicative of these uncommon conditions. By detecting the disease early and modeling its likely course, clinicians can intervene sooner and potentially improve prognoses. This use of AI democratizes expertise, bringing insights from global patient populations to local care settings. Ultimately, improved diagnostics and outcomes predictions for rare diseases ensure that patients receive timely, appropriate treatment that might otherwise be missed.
17. Real-Time Clinical Decision Support
With continuous data streaming from patient monitors and EHRs, AI engines can produce real-time predictive alerts, preventing complications like sudden cardiac arrest or respiratory failure.
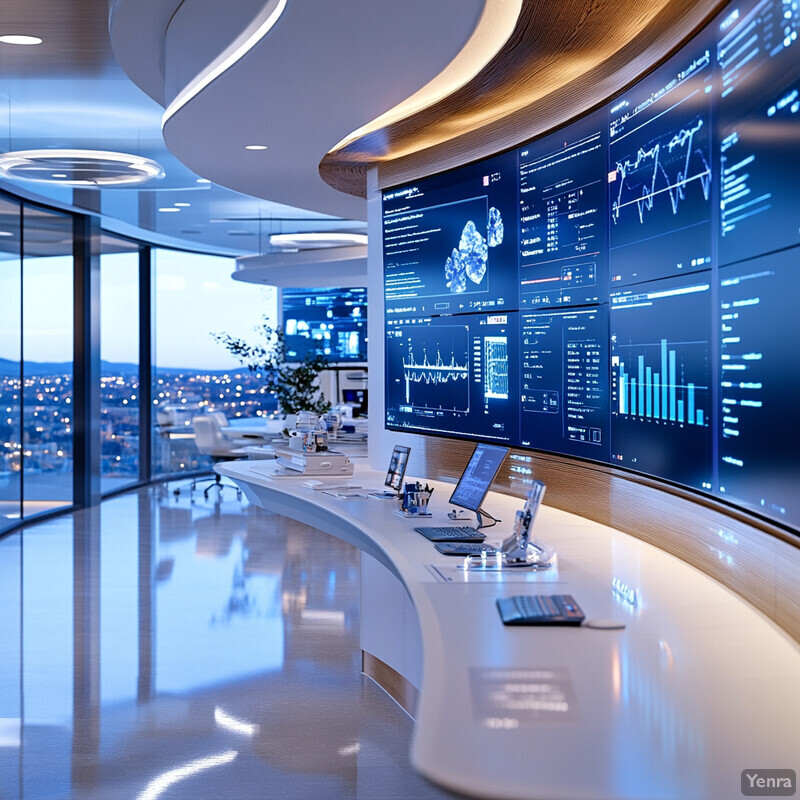
Modern healthcare settings produce continuous data streams—monitoring vitals, lab values, and patient activity in near real-time. AI-driven decision support systems can ingest and process this data as it arrives, signaling when a patient’s condition is taking a concerning turn. Whether it’s warding off sudden cardiac arrests or alerting staff to declining respiratory function, these predictive alerts enable clinicians to respond before an emergency fully unfolds. Such dynamic, just-in-time guidance makes care more agile, prevents critical complications, and enhances patient safety across the continuum of care.
18. Social Determinants of Health Integration
Including socioeconomic, environmental, and behavioral data in predictive models provides a more holistic view of patient risk, leading to more equitable and effective care plans.
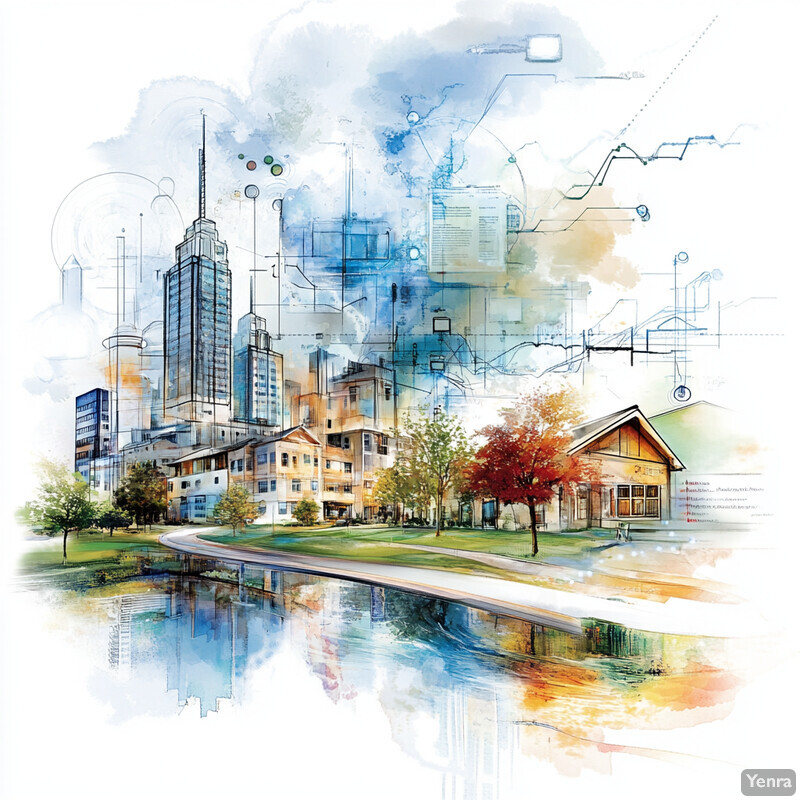
A patient’s environment, income level, education, and access to healthy food all influence health outcomes. AI models that incorporate these social determinants of health (SDOH) data can predict how socioeconomic factors contribute to a patient’s risk profile. Recognizing that non-clinical variables often drive health disparities allows clinicians and care coordinators to offer tailored support—such as assistance with transportation, nutrition counseling, or community services. Integrating SDOH into outcome predictions advances health equity, helping patients achieve better health regardless of their social circumstances.
19. Adaptive Predictive Models
Models that self-update with new data ensure that predictions remain accurate as treatment protocols evolve, patient populations shift, or emerging diseases arise.
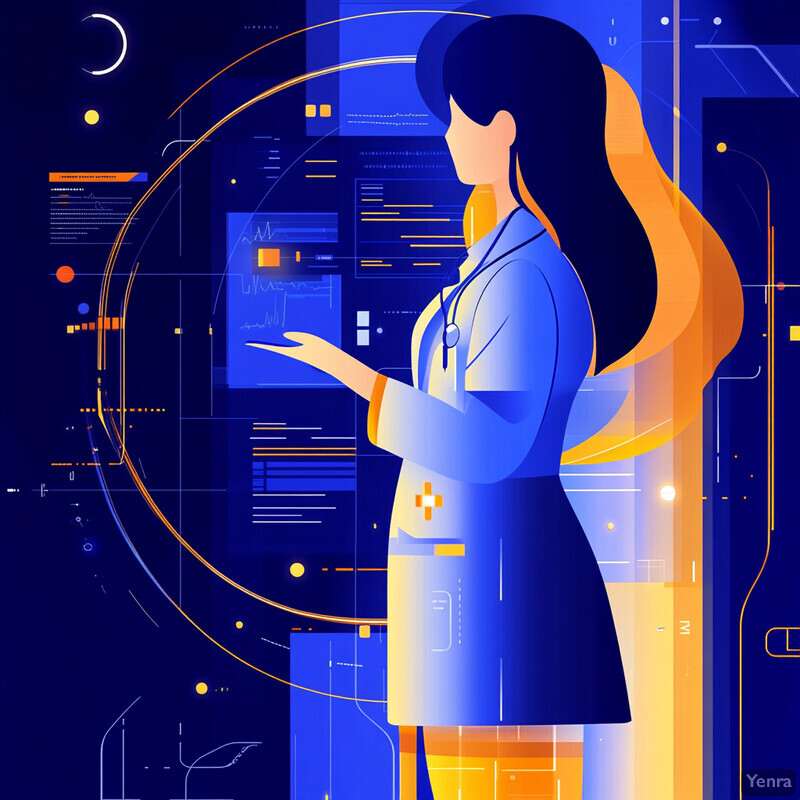
Clinical practice and patient populations are ever-evolving, so predictive models must also adapt. AI can continuously update and improve its algorithms as new data streams in—be it from new treatments, emerging pathogens, or shifting demographics. This flexibility ensures that predictions remain relevant and accurate over time, reflecting real-world changes in medicine and patient populations. By staying current, adaptive models help clinicians stay a step ahead, ensuring that their decision-making tools are always aligned with the latest evidence and trends, ultimately improving patient care quality.
20. Data-Driven Policy and Resource Allocation
Aggregated predictive analytics inform hospital administrators and policymakers, improving decision-making regarding staffing, equipment distribution, and public health interventions.
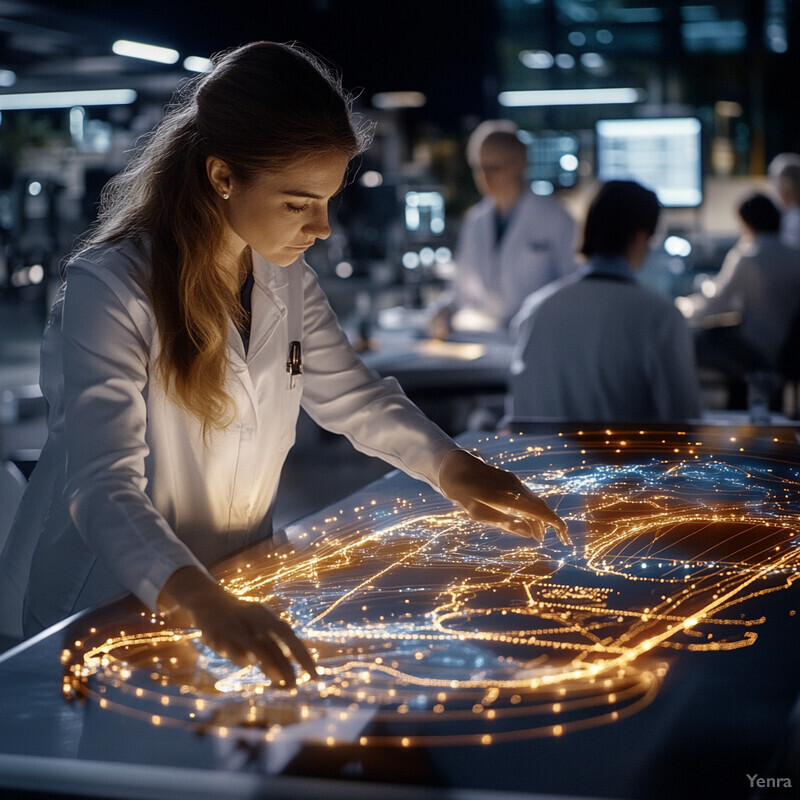
Beyond individual patient care, AI-driven predictions can influence healthcare policy and resource allocation at institutional or population-wide levels. For instance, knowing the likely future burden of chronic disease complications in a community can guide public health interventions, staffing decisions, or the acquisition of critical supplies. Predictive models inform administrators about where to invest in training, infrastructure, or preventive programs to improve patient outcomes systemically. When policies and resource distribution are informed by predictive analytics, the entire healthcare ecosystem becomes more efficient, responsive, and patient-centered.