1. Automated Geological Data Integration
AI-driven platforms can quickly integrate large, heterogeneous datasets—such as drill logs, geological maps, seismic data, and geochemical assays—enabling geologists to gain a more holistic understanding of the subsurface.
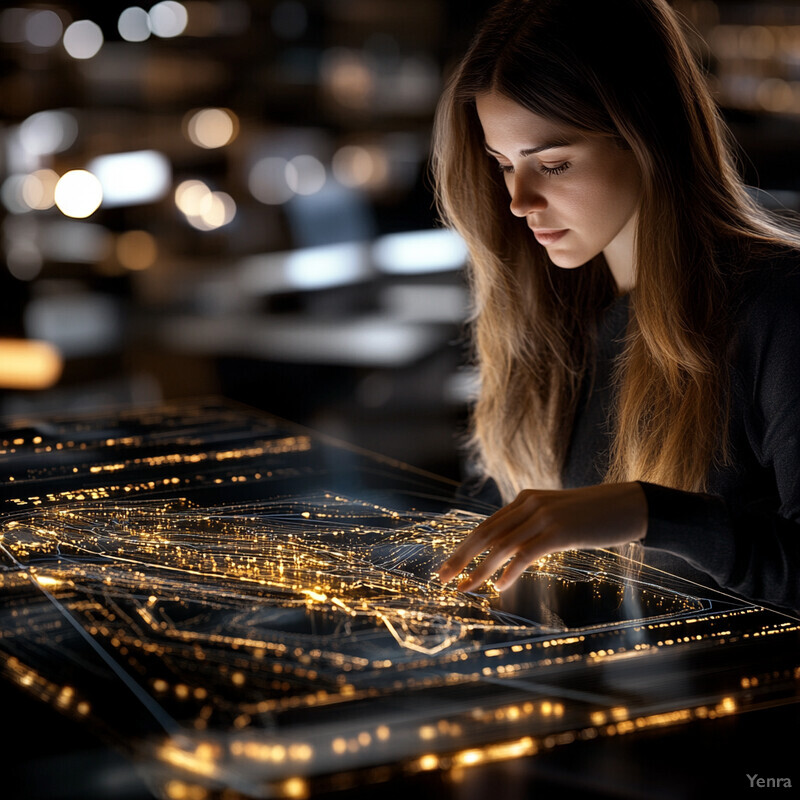
Modern exploration generates enormous quantities of diverse geological and geophysical data, including drillhole logs, satellite imagery, seismic profiles, gravity and magnetic surveys, geochemical assays, and more. Traditionally, compiling and correlating these sources to form a coherent geological interpretation demanded significant human effort and time. AI-driven platforms can handle these data streams simultaneously, rapidly cleaning, classifying, and merging multiple formats. By analyzing patterns across datasets, machine learning algorithms help geologists detect subtle relationships that might be overlooked by manual inspection, leading to a more comprehensive and accurate geological model. As a result, companies can streamline their initial exploration phases, reduce data redundancy, and achieve better-informed decision-making on where to focus fieldwork.
2. Advanced Geophysical Interpretation
Machine learning (ML) models can interpret magnetic, gravity, electromagnetic, and seismic data more efficiently and accurately, identifying subtle anomalies that may indicate mineralization.
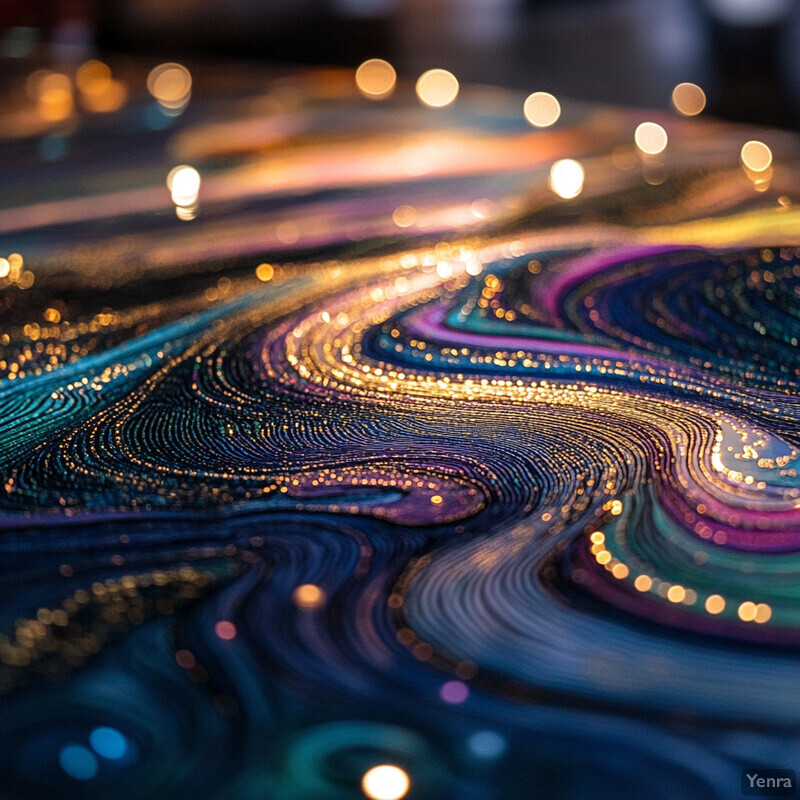
Geophysical survey data are often complex, noisy, and require expert interpretation. AI techniques, such as convolutional neural networks and ensemble learning, can identify patterns in seismic attributes, gravity and magnetic anomalies, and electrical or electromagnetic responses more efficiently than conventional manual methods. By training models on known mineralized systems, these algorithms can highlight geophysical signatures that correspond to particular ore deposit styles, lithological boundaries, or structural features. This automation not only speeds up the interpretation process but also reduces the risk of human bias, producing more robust targets for follow-up. Through continuous refinement with new data, AI tools enhance the predictive power and resolution of geophysical models, ultimately guiding exploration teams toward regions most likely to host economically significant mineralization.
3. Faster Mineral Targeting
By detecting patterns and correlations in complex data, AI algorithms can generate high-priority drilling targets more rapidly, reducing the exploration timeline and associated costs.
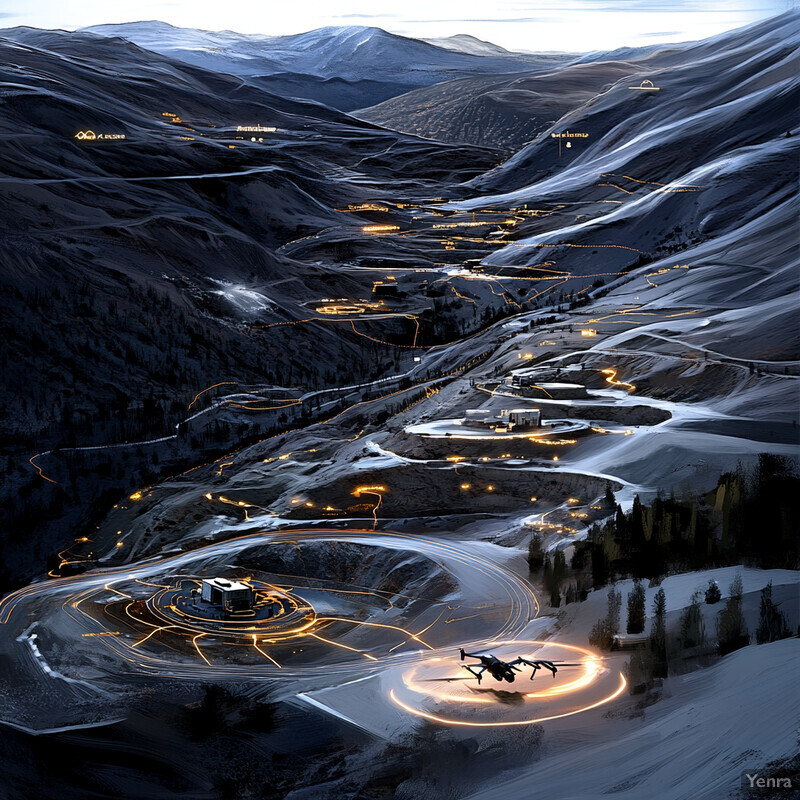
Traditional target generation processes can be slow and uncertain, often involving piecemeal data analysis and expert judgment. AI-driven mineral targeting systems combine geophysical data, geochemical patterns, structural models, and satellite imagery to rapidly identify zones with characteristics similar to known deposits. These algorithms can rank and prioritize potential targets based on probability scores, geoscientific criteria, and economic factors. By doing so, they significantly shorten the discovery timeline, enabling companies to focus their resources—both financial and human—on areas with the highest likelihood of success. This data-driven approach also encourages more systematic and transparent decision-making, reducing guesswork and potentially increasing the odds of finding viable mineral resources.
4. Predictive Resource Modeling
Deep learning models can predict mineral resources and reserves by analyzing historical patterns of known deposits and extrapolating the likelihood of mineral presence in underexplored regions.
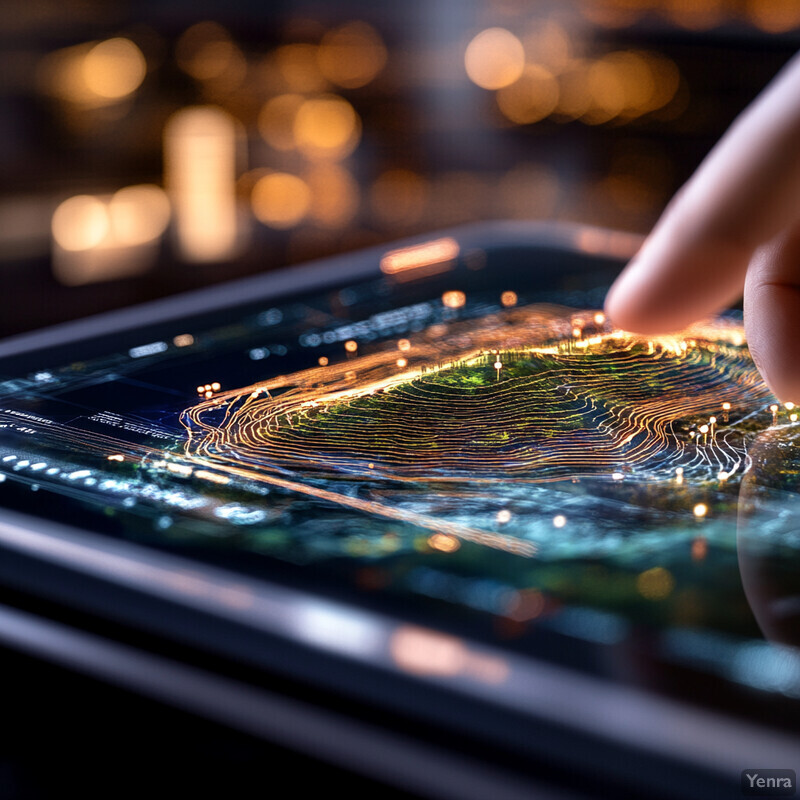
Estimating resources and reserves is a complex task involving interpolation, geological modeling, and geostatistics. Machine learning models, including random forests, gradient boosting, and deep neural networks, can mine historical data from known deposits and apply learned patterns to underexplored regions. They can integrate geological constraints, structural configurations, mineral chemistry, and more to produce predictive maps indicating where certain mineral concentrations are likely. Such modeling not only cuts down on expensive drilling campaigns by providing better initial estimates but also updates dynamically as new data become available, continuously refining the resource model. This real-time improvement in predictive accuracy can help exploration teams confidently plan the next steps in their projects.
5. Machine Vision in Core Logging
Computer vision algorithms can automatically identify mineral veins, textures, and alteration patterns in core samples, improving the speed and accuracy of geological logging.
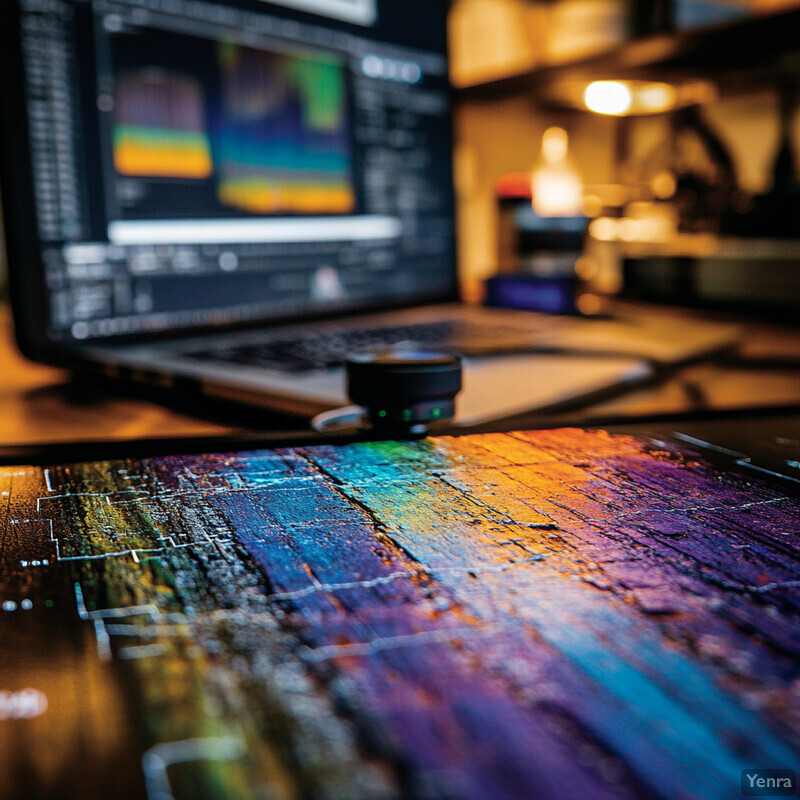
Manual core logging is time-consuming and can be subject to human error or bias, especially over long campaigns. Applying computer vision and image recognition algorithms to high-resolution photographs of drill cores allows the automatic identification and classification of minerals, rock textures, alteration patterns, and structural features. Through training on large datasets, these models can quickly and consistently annotate core samples, ensuring a higher degree of objectivity. This efficiency frees up geologists to focus on more interpretative work, fosters consistency in logging—particularly across different teams or contractors—and provides a permanent digital record for auditing. Over time, the growing database of “read” cores further refines the algorithms, improving their accuracy and utility.
6. Intelligent Drilling Guidance
AI can guide drilling campaigns by adjusting drilling paths in real-time based on new data, ensuring that exploration expenditures are focused on the most promising areas.
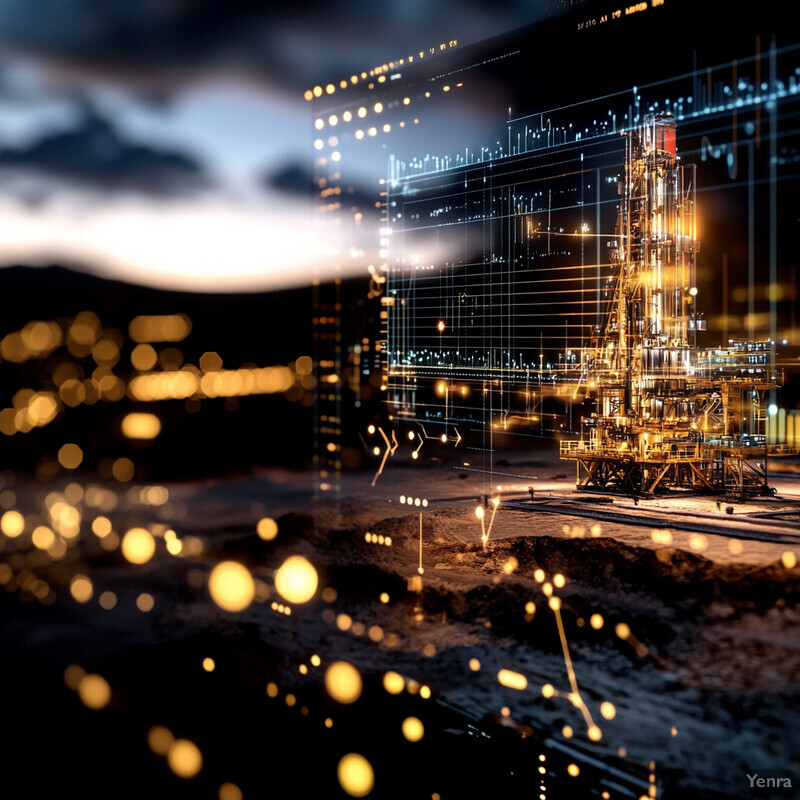
Drilling costs form a large part of exploration expenditures, and drilling in the wrong location can waste substantial resources. AI can help guide drilling in real-time, integrating data from sensors that measure parameters like rock hardness, drill penetration rate, acoustic signals, and downhole geophysical probes. By continually updating the subsurface model and analyzing where the drill bit is headed, AI systems can suggest course corrections, recommend stopping depths, or highlight when it might be prudent to move to a new target. This dynamic approach helps exploration teams reach prospective zones faster, avoid non-productive ground, and adapt their strategies immediately in response to on-the-fly data, ultimately improving the drilling success rate and cost-effectiveness.
7. Enhanced Grade Estimation
ML-based kriging and other geostatistical methods can produce more accurate resource grade estimates, reducing the uncertainty inherent in traditional interpolation approaches.
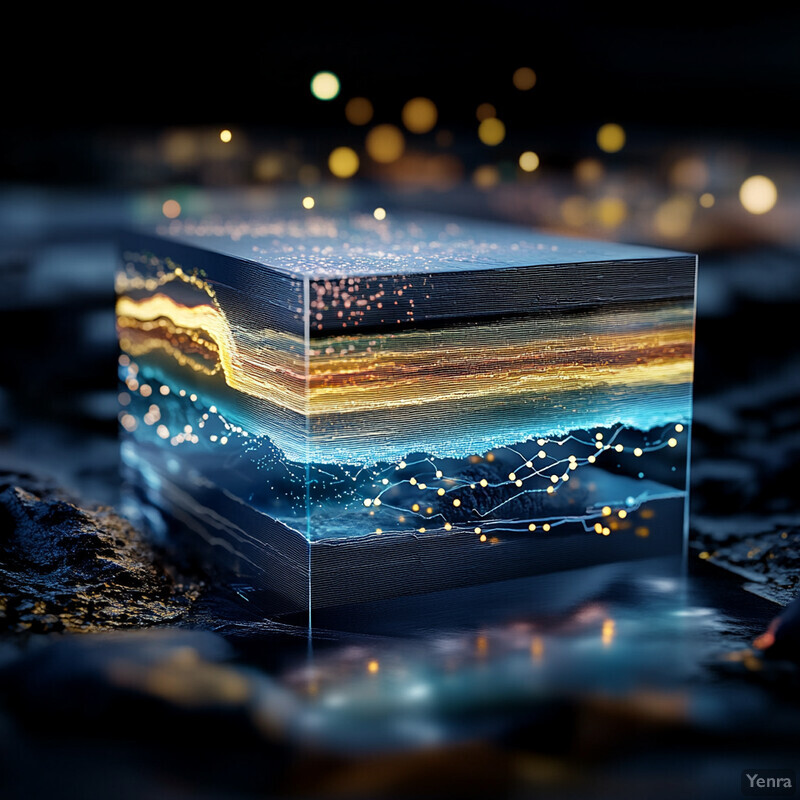
Traditional methods for estimating mineral grades, such as kriging and inverse distance weighting, rely heavily on geostatistical principles and human interpretation. While robust, these methods can sometimes oversimplify spatial relationships. Machine learning models can incorporate more complex patterns and can handle multimodal distributions, subtle geochemical gradients, and nonlinear relationships between variables. By training on actual assay results and known sample distributions, AI-driven grade estimation methods refine block models with higher precision and lower variance. The result is a more accurate understanding of resource quality, which in turn improves economic assessments, mine planning, and long-term project feasibility analyses.
8. Geochemical Anomaly Detection
AI models can sift through large geochemical datasets, detecting subtle geochemical signals or ratios that may indicate hidden mineralization zones not obvious to human experts.
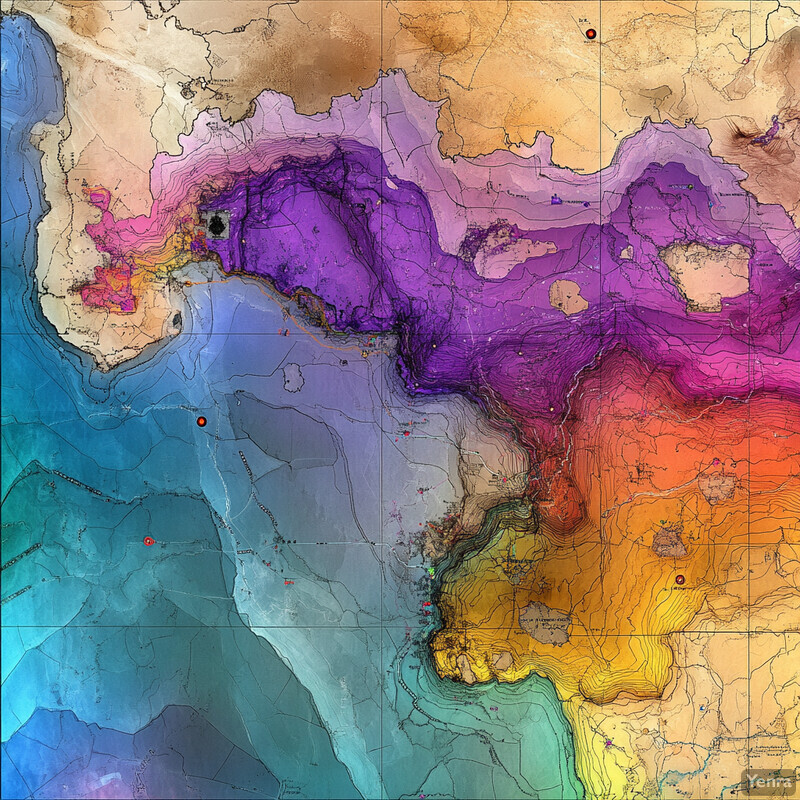
The subtle geochemical footprints of ore deposits may be concealed within large datasets of multi-element assay results, soil samples, rock chips, and stream sediments. Machine learning algorithms excel at identifying these hidden signals by filtering out noise and isolating patterns that correspond to mineralization processes. Through clustering, anomaly detection models, and dimensionality reduction techniques, AI systems highlight elemental associations that might be critical indicators of buried deposits. Once identified, these anomalies can guide more targeted field inspections, reducing reliance on broad reconnaissance surveys. Over time, geologists can fine-tune these models to reflect specific deposit types, metallogenic belts, or exploration criteria, thereby enhancing their predictive ability.
9. Seismic Attribute Extraction
Neural networks can automatically extract and classify seismic attributes, making the interpretation process faster and less reliant on time-consuming human analysis.
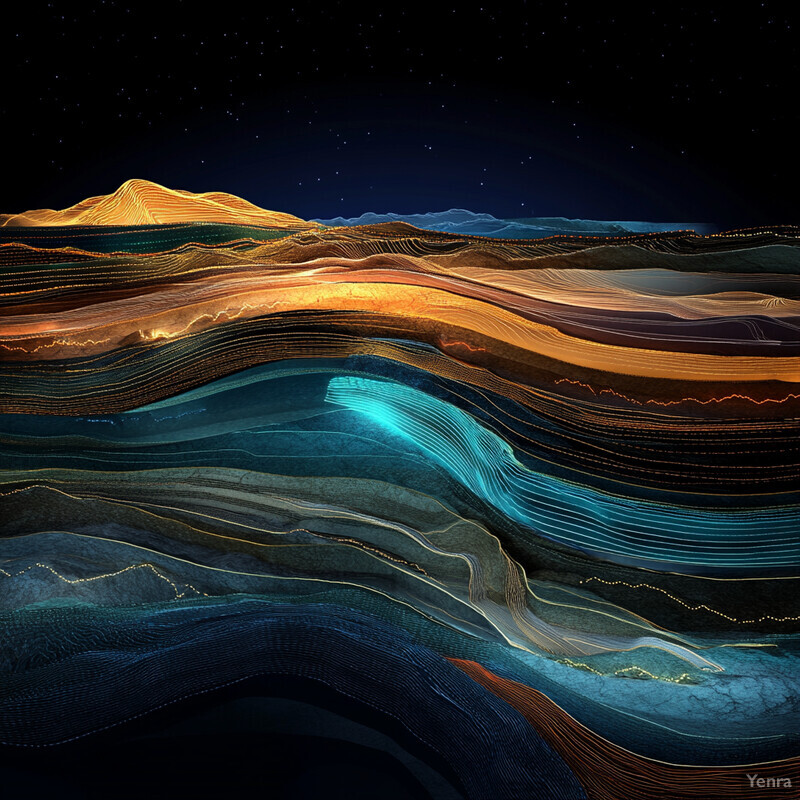
Interpreting seismic data can be time-intensive, and significant expertise is required to differentiate meaningful features from noise. AI-driven seismic interpretation tools apply pattern recognition to the abundance of attributes like amplitude, frequency, phase, and coherence. Neural networks can classify features that correspond to particular stratigraphic horizons, faults, or potential ore-hosting lithologies. By doing so more efficiently than manual interpretation, AI enables quicker decisions regarding where to drill or conduct follow-up surveys. The software can continuously learn from new well data, refining its ability to predict lithological changes and providing increasingly accurate seismic attribute mapping over time.
10. Improved Geological Domain Modeling
Automated clustering and classification algorithms can define geological domains, stratigraphic units, and structural zones more objectively, resulting in clearer 3D models.
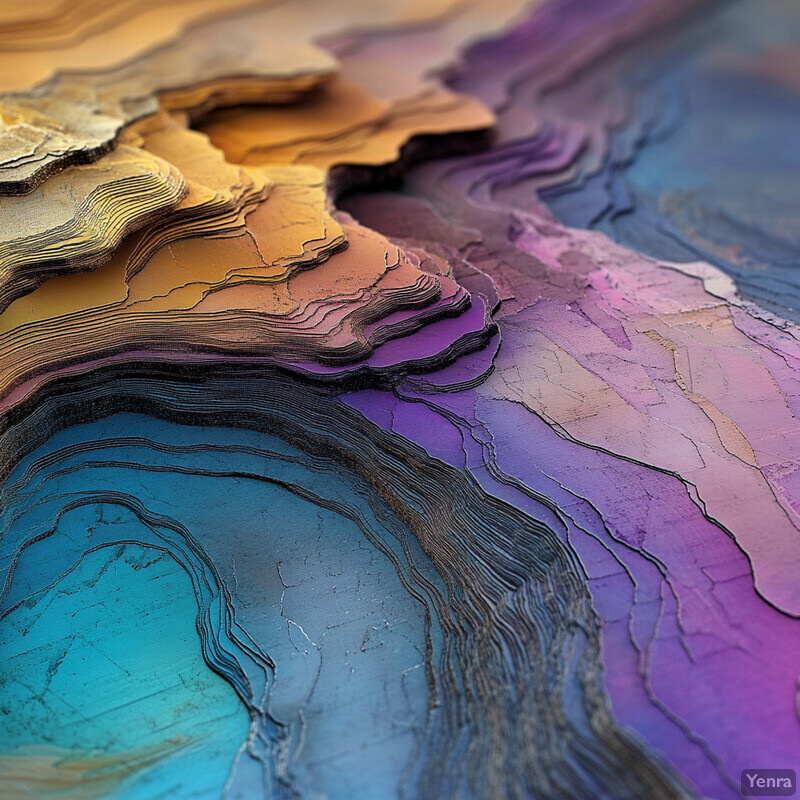
Traditional domain modeling often relies on manual interpretation, expert bias, and time-consuming 3D geological modeling. AI-driven clustering, classification, and segmentation techniques can automatically delineate geological units, structural domains, and alteration halos by identifying patterns in multiple data layers. This objective delineation helps build more accurate and consistent geological models, improving the basis for resource estimations and mine design. As the AI learns from each iteration—incorporating new drilling data, assay results, and structural measurements—the geological domains become more reliable frameworks on which subsequent resource and reserve calculations can be built.
11. Rapid Hyperspectral Image Analysis
AI can process hyperspectral data to identify mineral composition at the pixel level, accelerating the identification of prospective lithologies and alteration halos.
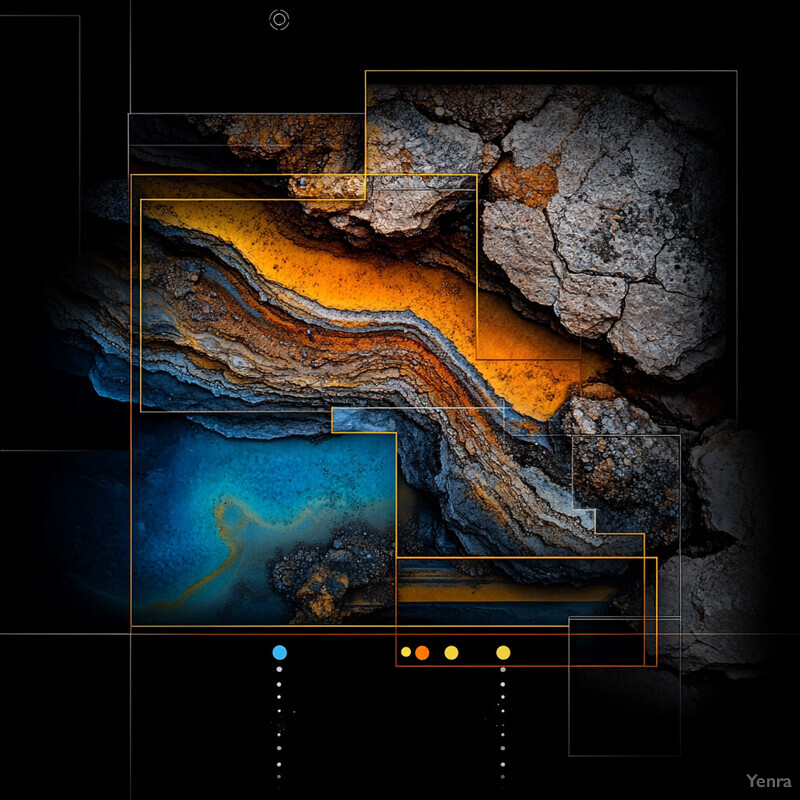
Hyperspectral imagery provides detailed information about the mineralogical composition of rocks and soils. Interpreting this data manually is a challenge due to the high dimensionality of the spectra. Machine learning and deep learning models can quickly classify minerals on a pixel-by-pixel basis, revealing subtle mineralogical changes associated with hydrothermal alteration or ore-related metamorphism. The rapid, automated analysis of hyperspectral data accelerates field mapping, target identification, and environmental baseline studies. By integrating these results into geological models, exploration teams gain a clearer picture of the mineral system’s footprint, thereby focusing field activities more efficiently.
12. Optimized Resource Estimation Workflows
By integrating AI solutions into existing software workflows (such as Datamine, Leapfrog, or Micromine), geologists can streamline resource modeling from data import to block model creation.
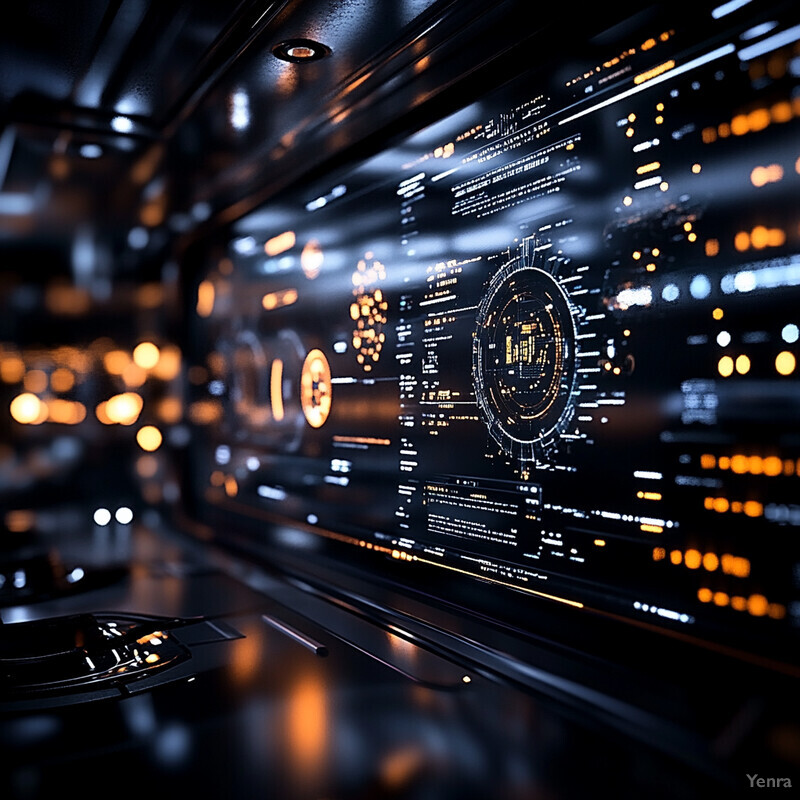
The integration of AI into commercial mining software packages streamlines the entire resource estimation process. Instead of following a linear sequence of manual steps—data cleaning, geostatistical modeling, block modeling, and validation—AI can automate and optimize these workflows. For example, it can identify the best estimation parameters, detect inconsistent data, suggest improved variogram models, and highlight areas where more sampling might reduce uncertainty. By making the process iterative and responsive, exploration teams can produce more robust resource models in less time, improving confidence in resource figures and shortening the gap between exploration, feasibility, and ultimately, production decisions.
13. Drillhole Spacing Optimization
Intelligent algorithms can suggest optimal drillhole spacing and orientation, maximizing resource definition while minimizing drilling costs.
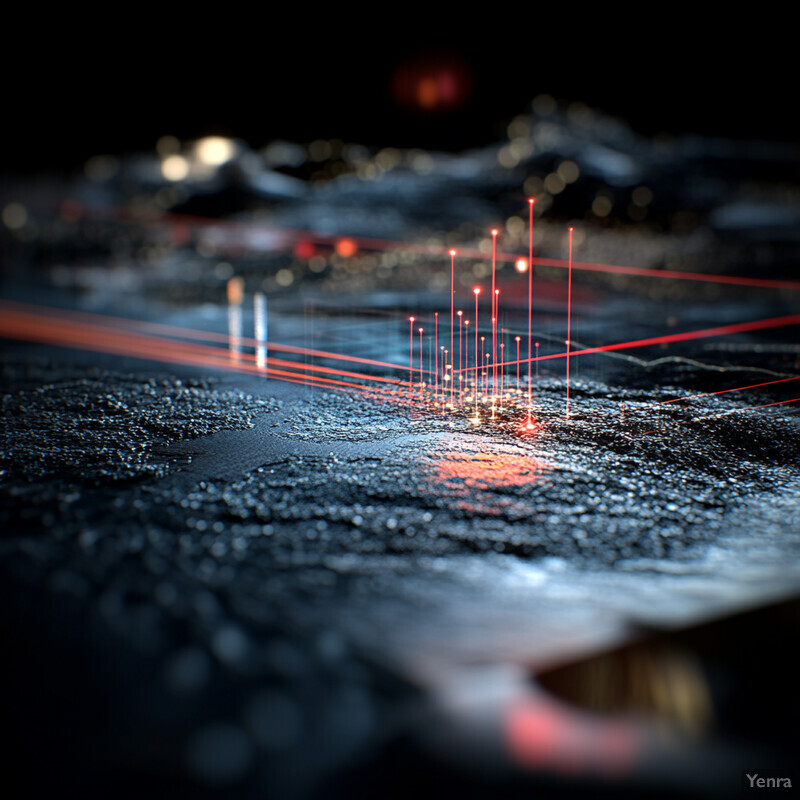
Determining drillhole density is often a balance between cost constraints and the desire for detailed information. AI models can simulate various drilling patterns and their impact on resource estimation confidence. By training on historical datasets, the algorithm can predict the incremental value of each new hole and recommend configurations that achieve desired confidence levels at the lowest cost. Through iterative optimization, companies can avoid over-drilling in some areas and under-drilling in others. This data-driven approach ensures that exploration budgets are allocated efficiently, shortening discovery timelines and generating more reliable resource estimates earlier in the project lifecycle.
14. Real-time Sensor Data Analysis
Online AI applications can continuously process sensor and logging-while-drilling data, adapting resource models instantaneously as new information becomes available.
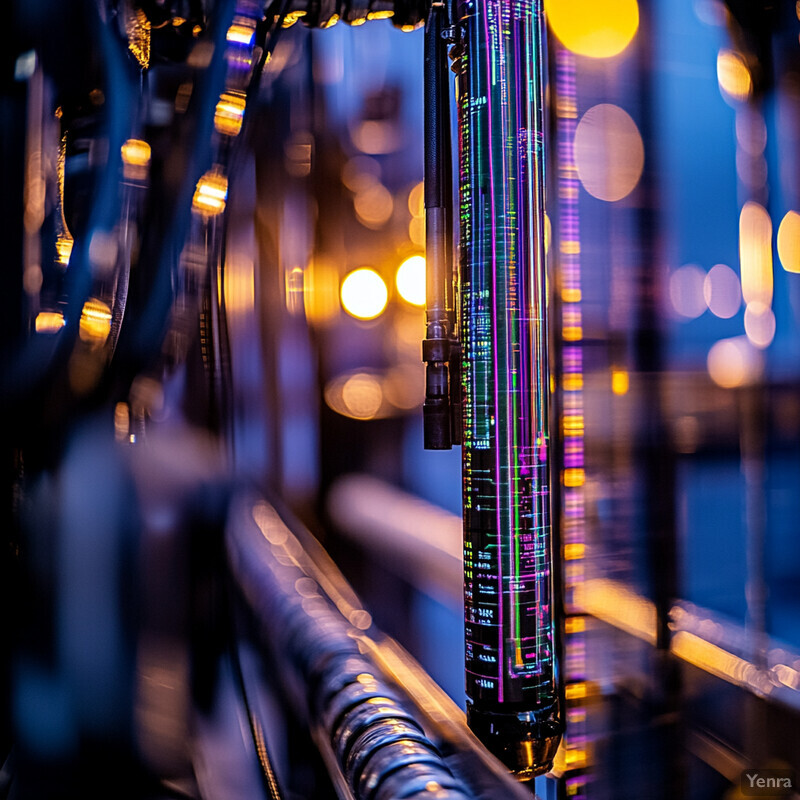
Advances in sensor technologies now allow the collection of drillhole geophysical readings, downhole imagery, and geochemical data on-the-fly. AI systems integrated into drill rigs and logging tools can interpret this data in real-time, updating geological models as drilling progresses. If a sensor indicates the presence of certain minerals or detects a structural boundary, the AI platform can recommend adjustments to the drilling plan immediately. This closed-loop process ensures that exploration activities can pivot rapidly in response to new information, increasing the chance of intersecting significant mineralization and reducing the time spent drilling barren ground.
15. Reduced Exploratory Risk
By providing a more data-driven exploration strategy, AI reduces the risks associated with “blind” drilling and guesswork, improving the probability of successful discoveries.
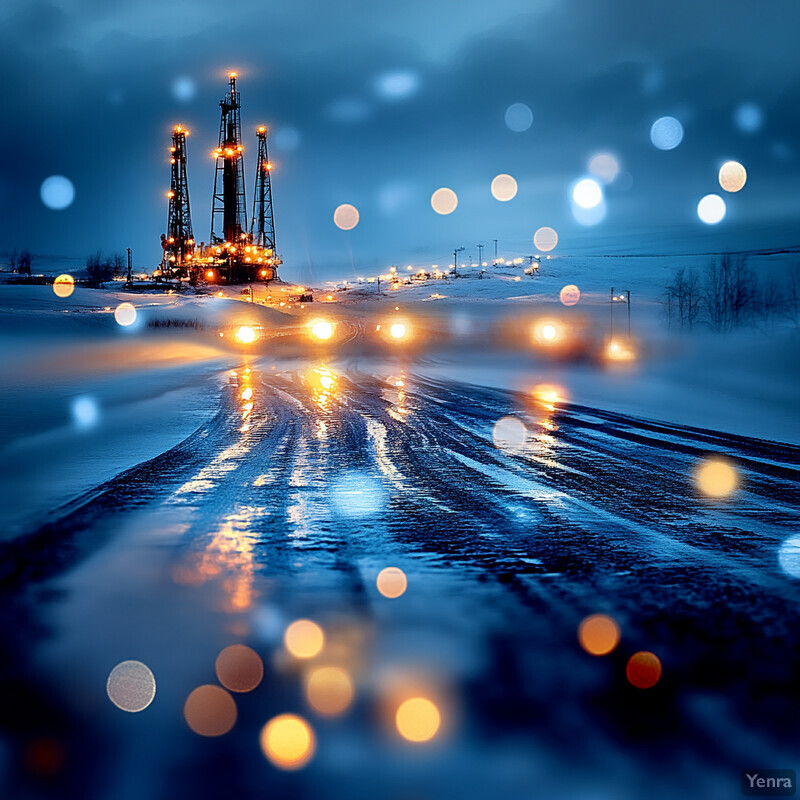
Mineral exploration inherently involves uncertainty, but AI can mitigate some of these risks by leveraging large datasets and advanced predictive modeling. By identifying patterns associated with known deposits, AI tools help focus exploration efforts on areas with the greatest potential. This reduces “blind” drilling campaigns and lowers the likelihood of sinking capital into fruitless ventures. With more data-driven target generation, geologists and investors alike gain confidence that their exploration budgets are well spent, improving the overall risk profile of early-stage mining projects. In a capital-intensive industry, such risk reduction is a significant advantage.
16. Uncertainty Quantification
AI methods can quantify and visualize uncertainty in resource estimates, helping decision-makers understand the confidence levels associated with various scenarios.
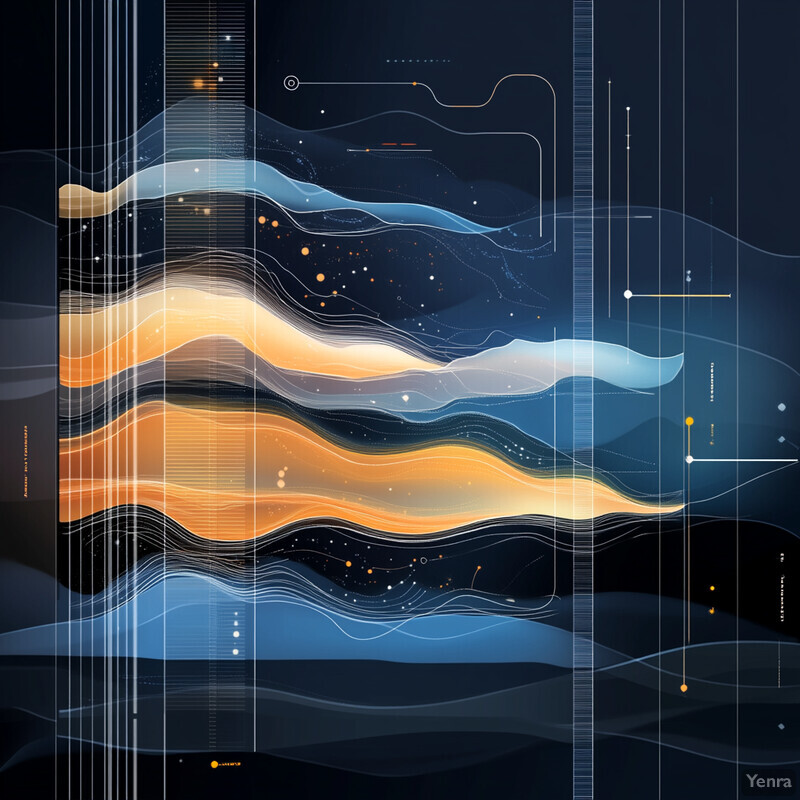
Even the best models carry a certain degree of uncertainty. AI techniques can quantify this uncertainty, providing ranges or probability distributions of resource estimates and highlighting geological parameters that contribute most to variance. This information helps decision-makers understand the confidence levels of their models and informs them where to gather additional data to reduce uncertainty. By incorporating probabilistic modeling and ensemble approaches, the AI-generated results can guide exploration strategies that target areas where a small amount of additional data could greatly improve the reliability of resource predictions. Ultimately, this leads to more informed decision-making and better risk management in exploration programs.
17. Integration of Legacy Data
Machine learning can leverage decades-old analog data—such as old maps, reports, and logs—by digitizing and interpreting them, improving exploration models without requiring fresh fieldwork.
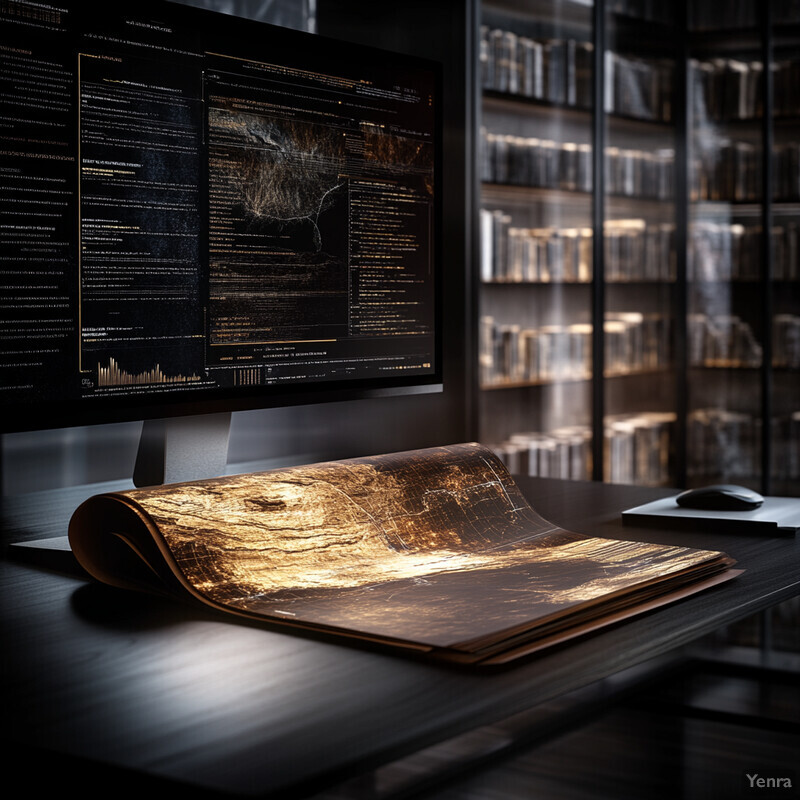
Historic data—often in the form of old paper maps, incomplete spreadsheets, and legacy reports—can be challenging to incorporate into modern 3D models. AI-driven digitization and natural language processing systems can extract meaningful information from archival documents and georeference historical maps. Machine learning can also correlate legacy geochemical or geophysical data with modern datasets, uncovering trends that might have been missed by previous generations of geologists. By breathing new life into older information, exploration teams can build on prior knowledge, saving time and money that would otherwise be spent re-acquiring the same data. The result is a richer, more complete foundation on which to target new exploration efforts.
18. Structural Geology Analysis
AI tools can automatically identify and map faults, folds, and shear zones from geophysical and geological datasets, giving clearer structural frameworks to guide exploration.
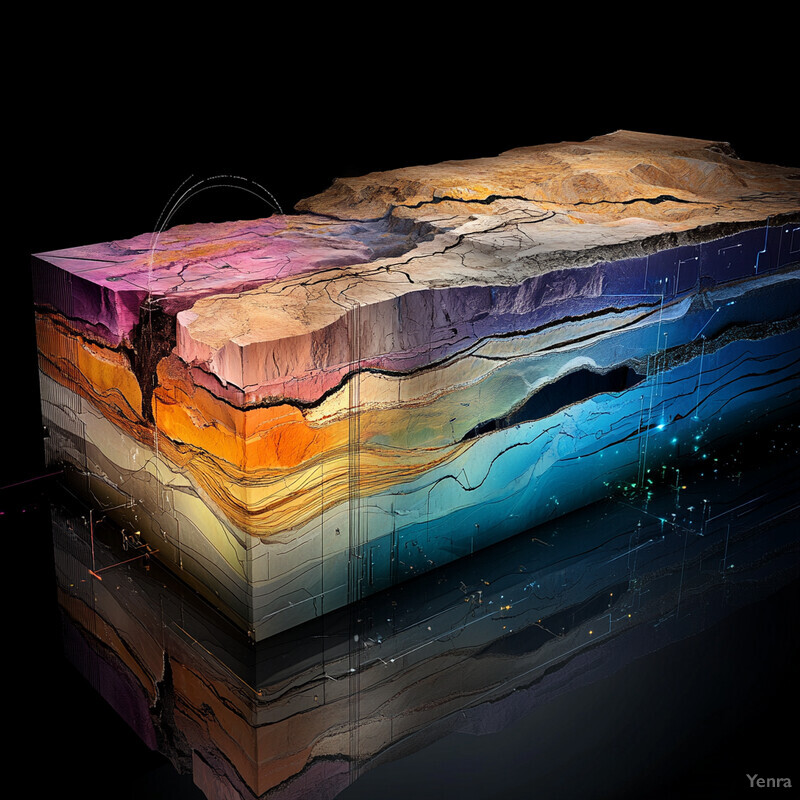
Structural controls often play a crucial role in the localization and concentration of mineral deposits. Identifying fault lines, fold axes, lineaments, and shear zones from geophysical and geological data can be complex. AI systems trained to detect these features can swiftly process large volumes of data and delineate structures with consistent criteria. Whether working from surface mapping data, airborne geophysics, or subsurface drillhole data, the AI’s output helps geologists understand how mineralizing fluids may have moved through the rock mass. This knowledge refines targeting strategies by focusing on structural traps and fluid pathways that commonly host high-grade zones, ultimately guiding drilling toward more prospective areas.
19. Automated Classification of Rock Types
Using pattern recognition, AI can classify rock types from thin-section images or drill cuttings photographs, saving time and ensuring consistency in lithological logging.
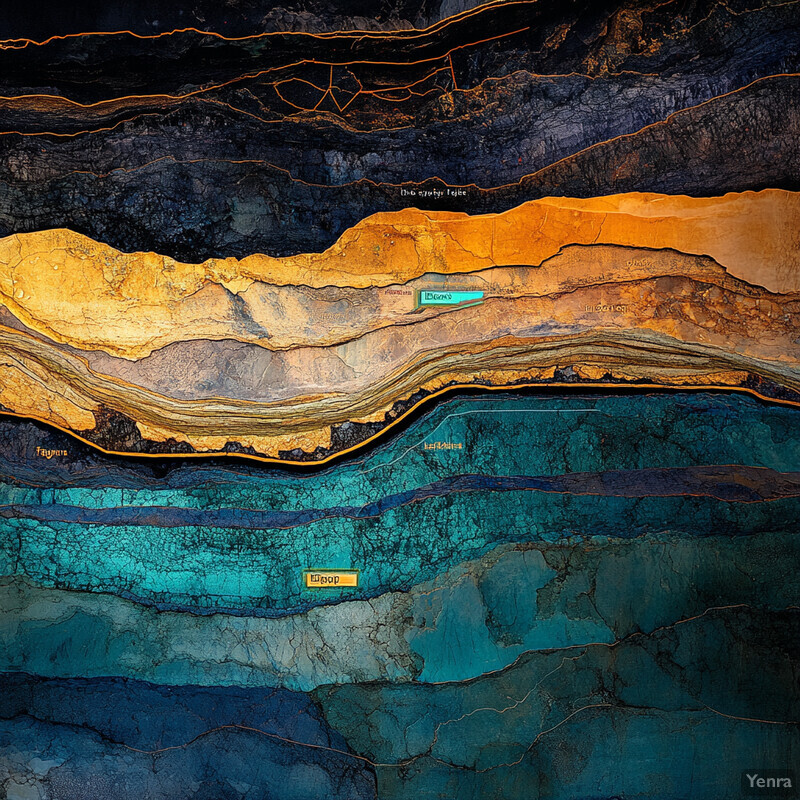
Determining rock types through thin sections, drill chips, or outcrop photographs is a fundamental step in exploration. AI-assisted image recognition can classify rocks based on mineral proportions, grain sizes, textures, and spectral signatures. This eliminates subjectivity, ensures consistent lithological logging, and allows for rapid processing of field samples. The resulting digital logs can feed directly into 3D geological models, enhancing their accuracy. As these models continue to learn from expanding image libraries and verified classifications, they become more adept at handling complex lithologies and subtle variations, making them indispensable tools in modern exploration teams’ workflow.
20. Continuous Improvement via Feedback Loops
As new data (e.g., from additional drilling, new assays, or updated geological models) is incorporated, AI systems learn and refine their predictions, continuously improving resource estimates over the life of an exploration project.
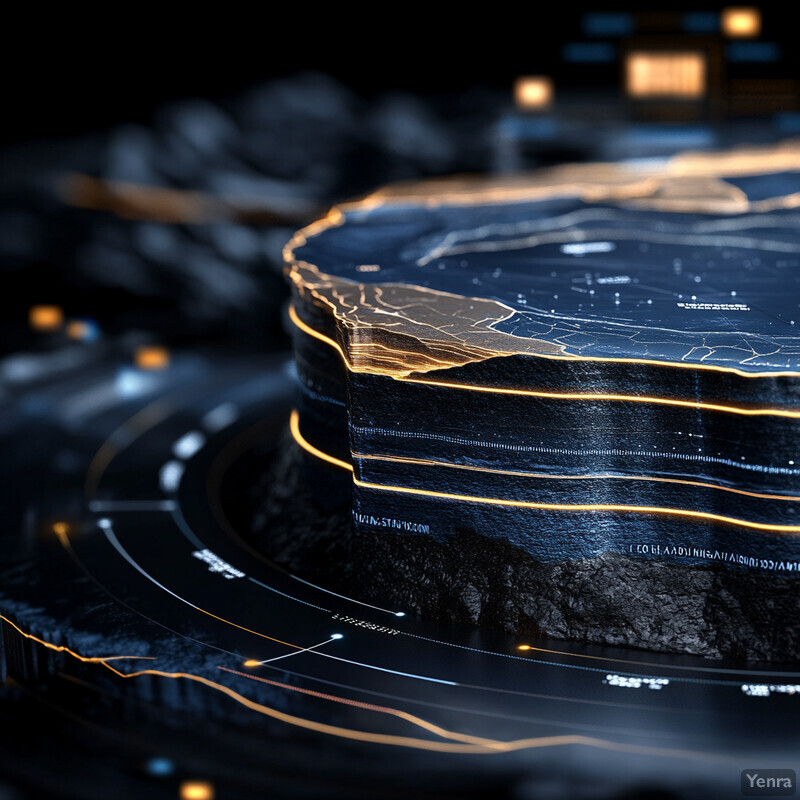
AI models are not static; they improve as they absorb new information. Exploration programs generate fresh data at every stage, from early geochemical surveys to advanced drilling campaigns. By integrating these datasets back into the modeling process, AI algorithms refine their predictions about geology, resource distribution, and deposit geometry. This iterative feedback loop ensures that resource estimates and geological interpretations evolve dynamically, aligning models more closely with reality over time. As a project progresses, this continuous learning approach results in increasing confidence, reduced uncertainty, and ultimately, a more robust path from discovery through to feasibility studies and eventual mine development.