1. Predictive Modeling of Land Suitability
Machine learning models analyze historical data on climate, soil quality, topography, and infrastructure to predict optimal land uses (e.g., which areas are best for agriculture, housing, industry, or conservation).
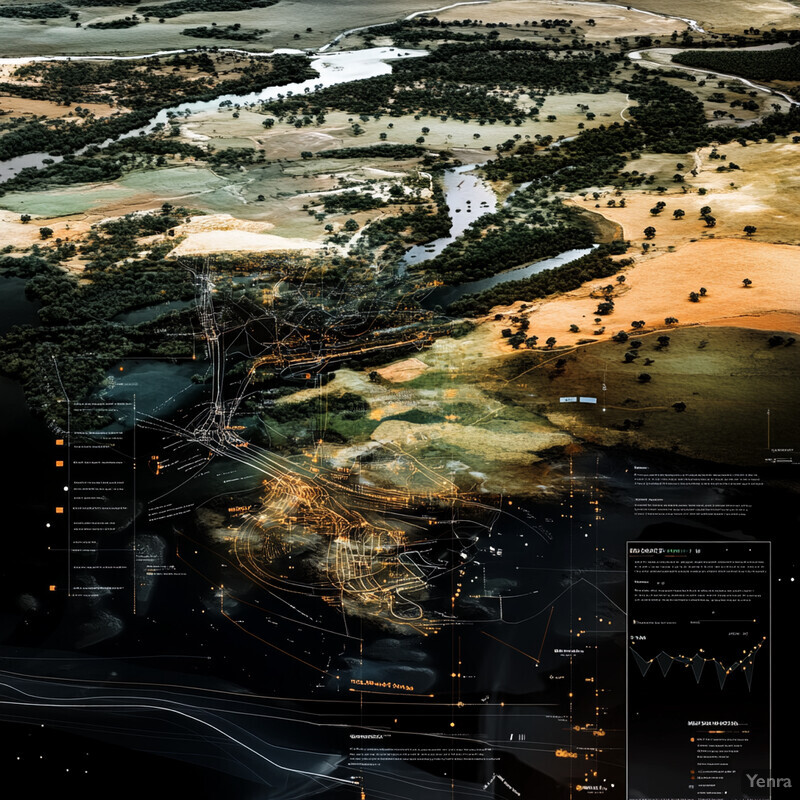
AI-driven predictive modeling tools leverage historical and real-time data to determine which areas of land are best suited for various purposes. By incorporating environmental factors such as soil composition, topography, precipitation patterns, and historical crop yields, these models identify the most productive agricultural lands, the best sites for housing developments, or the most suitable areas for industrial use. In addition, integrating socioeconomic and infrastructural data can help ensure that the recommended land use aligns with market demands, resource availability, and regulatory constraints. Ultimately, this holistic approach allows planners and policymakers to make informed, data-driven decisions that optimize land usage in the long term.
2. Automated Zoning Recommendations
Advanced AI algorithms can generate zoning plans that balance competing interests—commercial, residential, agricultural, and natural areas—while adhering to regulatory constraints.
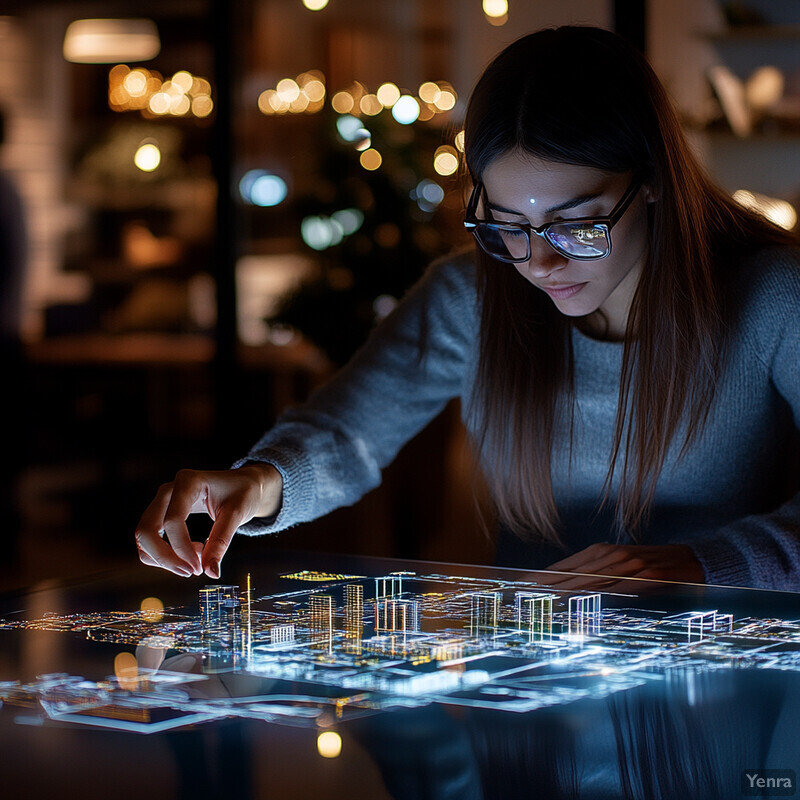
Traditionally, zoning decisions often rely on human expertise and manual data analyses, which can be time-consuming and prone to bias. AI-based zoning recommendation systems automate this process, quickly evaluating countless variables—environmental regulations, community development goals, economic considerations, and more—to propose tailored zoning maps. These systems factor in constraints like building height limits, density guidelines, or green space requirements, ensuring that the final zoning layout balances community interests, regulatory compliance, and sustainable development objectives. As a result, decision-makers can expedite the planning process and achieve outcomes that are more equitable, resilient, and aligned with future growth projections.
3. Multi-Objective Optimization
AI techniques such as genetic algorithms can simultaneously optimize for multiple factors—like economic returns, environmental impact, and social equity—allowing planners to identify land-use solutions that satisfy a range of stakeholder priorities.
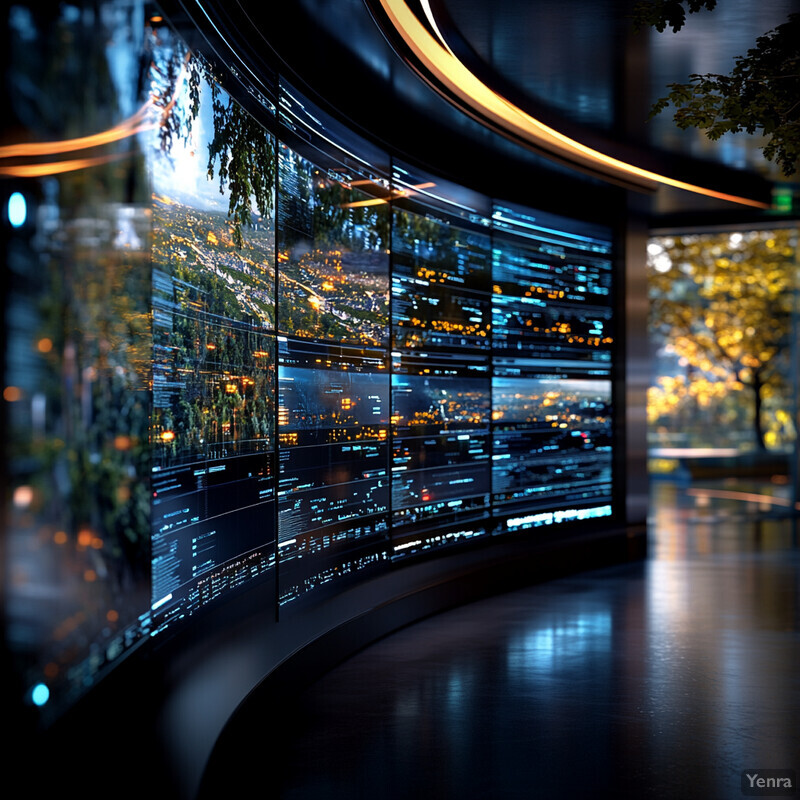
Land use optimization often involves trade-offs among competing goals—maximizing economic returns, preserving ecological integrity, and ensuring social equity. AI-driven multi-objective optimization uses sophisticated algorithms, such as genetic algorithms or multi-criteria decision analysis, to find an optimal balance among these diverse objectives. By processing numerous scenarios simultaneously, AI can highlight land use strategies that, for example, support economic development without excessively harming biodiversity or displacing vulnerable communities. The resulting “best-fit” solutions empower policymakers and stakeholders to choose among several well-calibrated options, each reflecting a carefully calculated equilibrium among the many factors influencing land utilization.
4. Remote Sensing and Image Analysis
Deep learning models process satellite and aerial imagery to detect land cover changes, classify different vegetation types, and identify illegal land uses (e.g., deforestation) faster and more accurately than traditional methods.
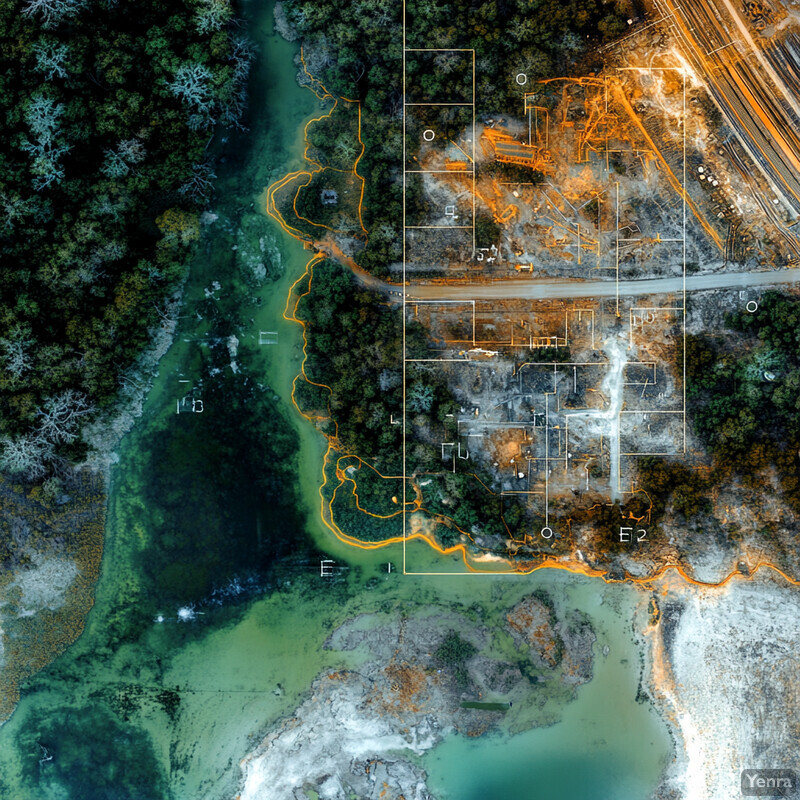
Advances in computer vision and deep learning enable AI systems to interpret remotely sensed images from satellites, drones, or aircraft. These models classify different land covers—forests, wetlands, croplands, urban areas—and detect subtle changes over time, such as illegal deforestation or urban encroachment onto farmland. By rapidly processing large volumes of imagery, AI helps planners monitor dynamic landscapes, identify emerging trends, and take corrective actions more swiftly than manual survey methods would allow. Improved land cover mapping not only supports enforcement of environmental regulations but also guides informed allocation of resources, helping maintain ecological balance and productivity.
5. Real-Time Data Integration
AI systems can ingest continuous data streams from sensors, weather stations, and market feeds, dynamically adjusting land use recommendations in response to changes in climate conditions, water availability, and commodity prices.
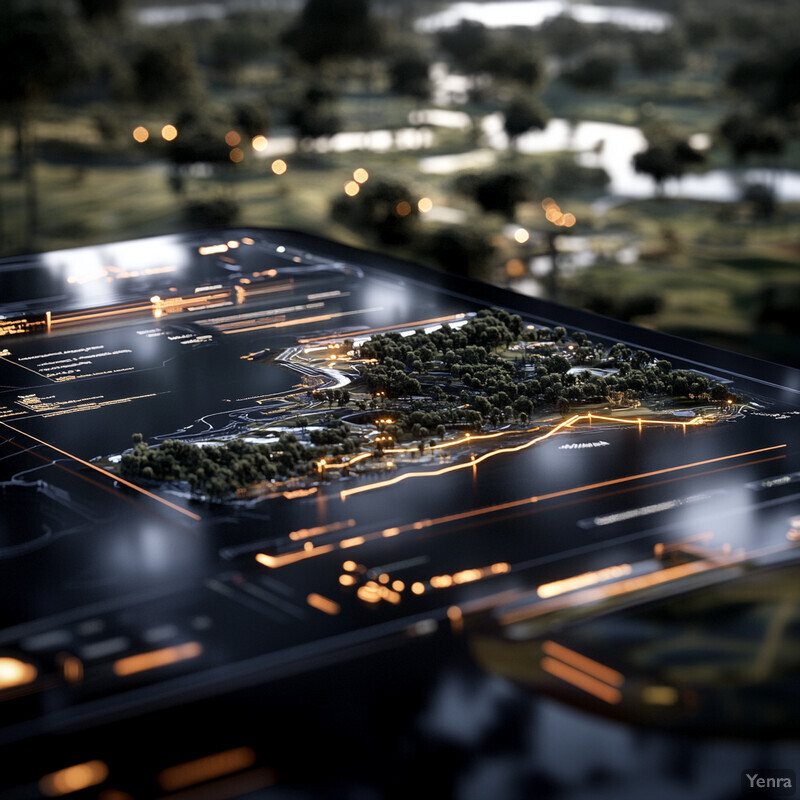
The availability of continuous data streams—from weather sensors, economic indicators, environmental monitors, and social media—means land use planning can become adaptive and responsive. AI systems aggregate and interpret these streams in real time, adjusting recommendations as conditions evolve. For instance, if drought conditions intensify, AI models might suggest shifting agricultural lands toward more drought-tolerant crops or adjusting planned developments to ensure water supply resilience. This responsiveness ensures that land use decisions remain robust against unforeseen changes, ultimately contributing to more stable and sustainable long-term land management strategies.
6. Climate Adaptation Planning
By coupling AI-driven land-use models with long-term climate forecasts, decision-makers can anticipate shifts in temperature, precipitation, and extreme weather events, allocating land more resiliently and sustainably.
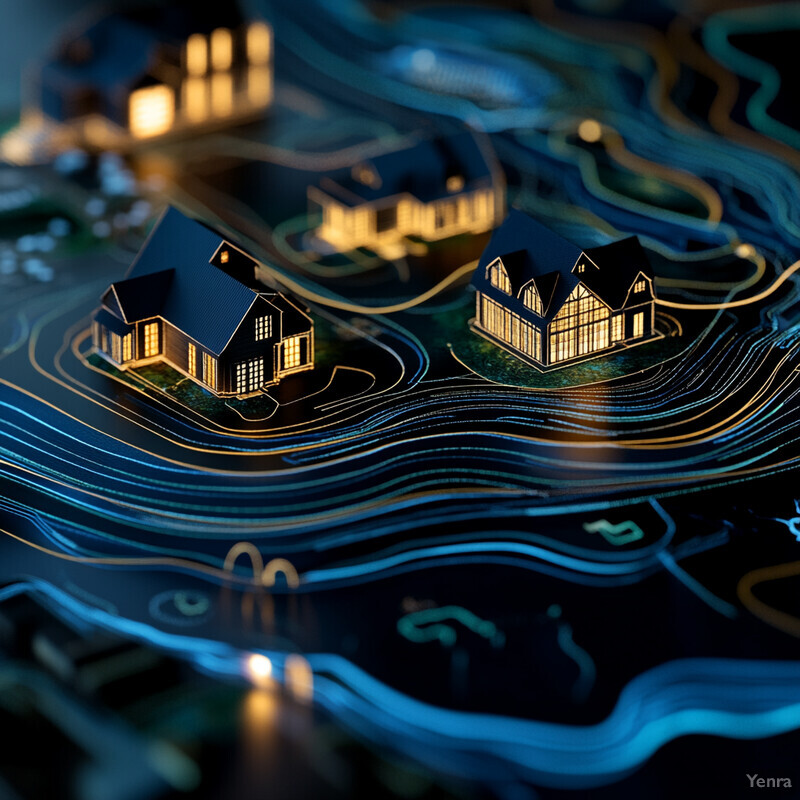
As climate change alters temperature patterns, precipitation regimes, and the frequency of extreme weather events, AI can assist in envisioning future landscapes that remain viable under these new conditions. By integrating climate models and forecasts with land use optimization algorithms, planners can identify areas at risk of flooding or drought and designate them for uses that minimize damage. For example, restoring wetlands or planting flood-tolerant vegetation in certain zones can mitigate flood risks, while concentrating drought-resistant agriculture in vulnerable regions reduces future crop failures. AI thus helps build climate resilience into land use plans, increasing the long-term security of communities and ecosystems.
7. Urban Growth Simulation
Machine learning models simulate various scenarios of urban expansion, suggesting optimal patterns of development that minimize sprawl, reduce transportation costs, and preserve green space.
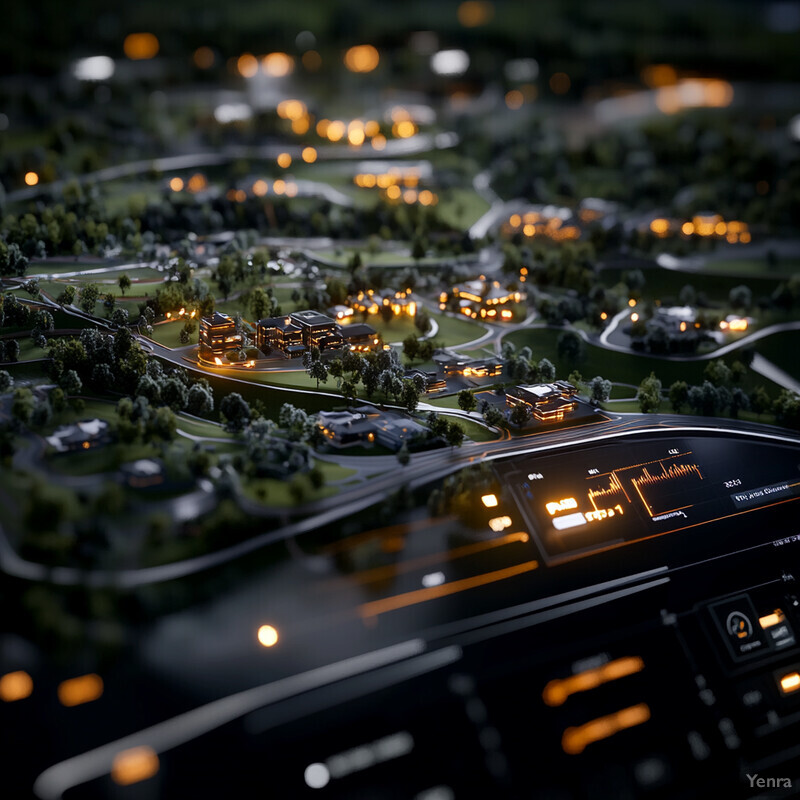
Urbanization often proceeds in an unstructured, haphazard manner, leading to congestion, inefficient infrastructure, and environmental degradation. AI-powered urban growth simulation tools model how cities might expand under various policy, economic, and infrastructural scenarios. By adjusting parameters—such as housing density, road placement, or public transportation networks—AI can reveal the future shape of a city under different decision frameworks. Planners can use these simulations to encourage more compact development, reduce commuting times, preserve green spaces, and maintain environmental quality, ensuring that urban growth proceeds in an orderly and sustainable manner.
8. Resource Allocation for Agriculture
AI can refine land allocation for different crops, using predictive yield models, soil analysis, and rainfall predictions to maximize agricultural productivity and sustainability.
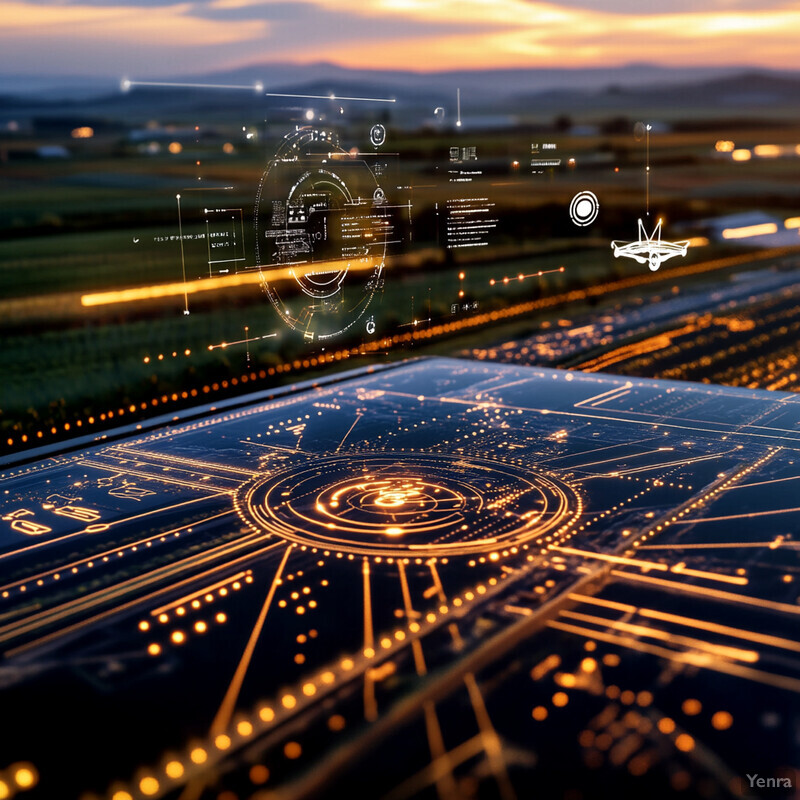
Agricultural productivity depends on matching the right crops with the right conditions. AI can analyze soil properties, climate forecasts, and market trends to identify optimal cropping patterns across large landscapes. By suggesting where to grow drought-tolerant varieties, introduce climate-smart irrigation systems, or invest in precision fertilization, these systems help maximize yields while minimizing inputs and environmental degradation. In doing so, AI-guided resource allocation supports food security, reduces waste, and helps maintain the ecological health of farmlands, ensuring that agricultural lands remain productive and sustainable for future generations.
9. Biodiversity Preservation
Reinforcement learning and evolutionary algorithms can identify configurations of protected areas that maintain wildlife corridors and preserve critical habitats while allowing for efficient use of adjacent lands.
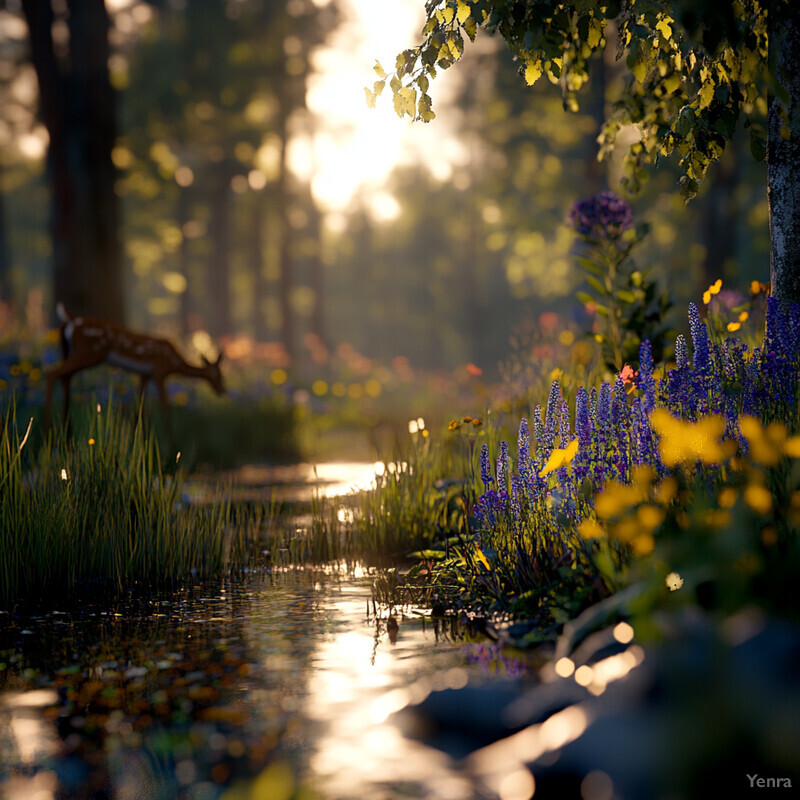
Preserving biodiversity often involves designating protected areas that maintain habitat connectivity and shelter endangered species. AI algorithms, including reinforcement learning and heuristic optimization, can help identify which parcels of land should be conserved to maintain key wildlife corridors and ecological networks. By modeling species distribution, migration patterns, and habitat requirements, these tools ensure that conservation areas are not chosen in isolation but as part of a broader ecological strategy. The result is a more holistic approach to land use that balances human activities with the long-term well-being of native species and ecosystems.
10. Disaster Risk Mitigation
AI-driven hazard mapping evaluates flood, fire, and landslide risks, suggesting where construction should be limited or infrastructural improvements made, thus optimizing land use for safety.
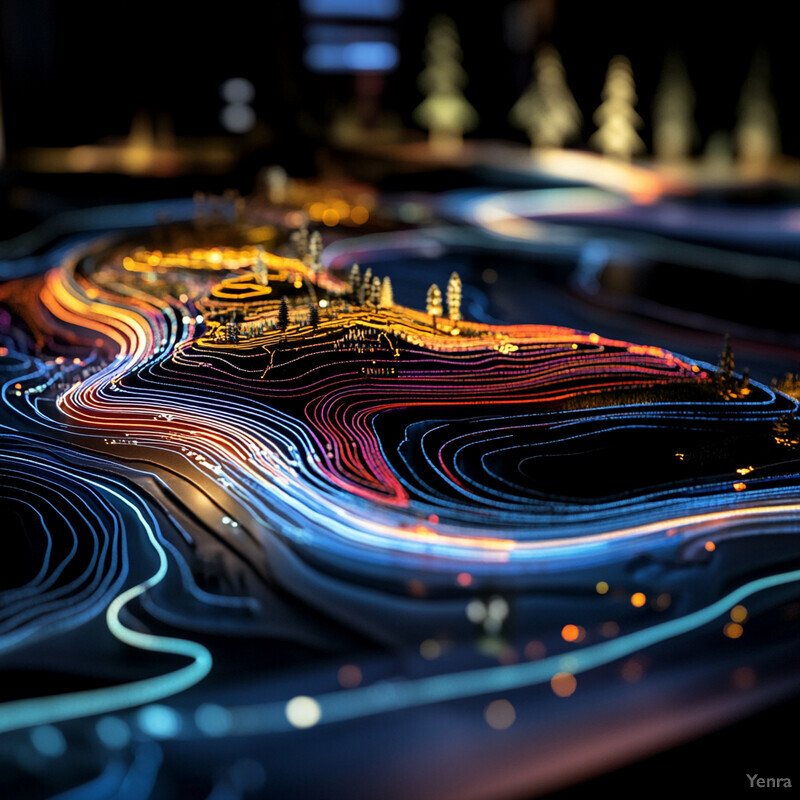
Natural disasters like floods, landslides, or wildfires pose ongoing threats to human settlements and infrastructure. AI can integrate topographic data, land cover information, weather patterns, and historical disaster records to identify areas of elevated risk. With this insight, planners can limit construction in vulnerable zones, reinforce infrastructure where necessary, or devote certain lands to buffering natural hazards (e.g., using forests as wildfire breaks or wetlands as flood retention zones). By incorporating disaster risk analysis into land use optimization, AI helps reduce future loss of life, property damage, and associated economic costs.
11. Scenario Planning and Simulation
AI tools generate 'what-if' scenarios that explore the impacts of policy changes—like shifting subsidies or imposing zoning restrictions—helping stakeholders make informed decisions before land-use policies are enacted.
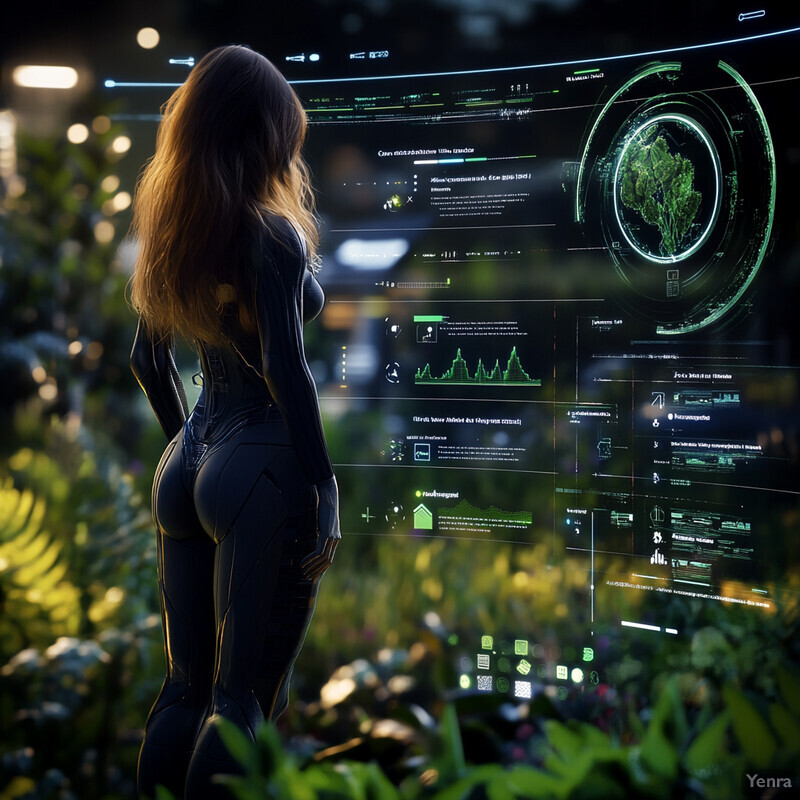
Before enacting policies, land managers and policymakers need to understand their potential consequences. AI-powered scenario analysis tools generate 'what-if' models that simulate how land use patterns evolve under different regulatory changes, economic incentives, or infrastructure projects. Users can explore outcomes—such as changes in housing affordability, ecosystem health, or agricultural output—without actually implementing the policies. This 'virtual policymaking' environment helps stakeholders weigh pros and cons, reach consensus, and choose measures that best align with strategic goals and community values.
12. Spatial Pattern Recognition
Clustering and pattern recognition techniques identify spatial patterns in land usage—such as urban heat islands or areas of frequent soil erosion—guiding targeted interventions to improve land management.
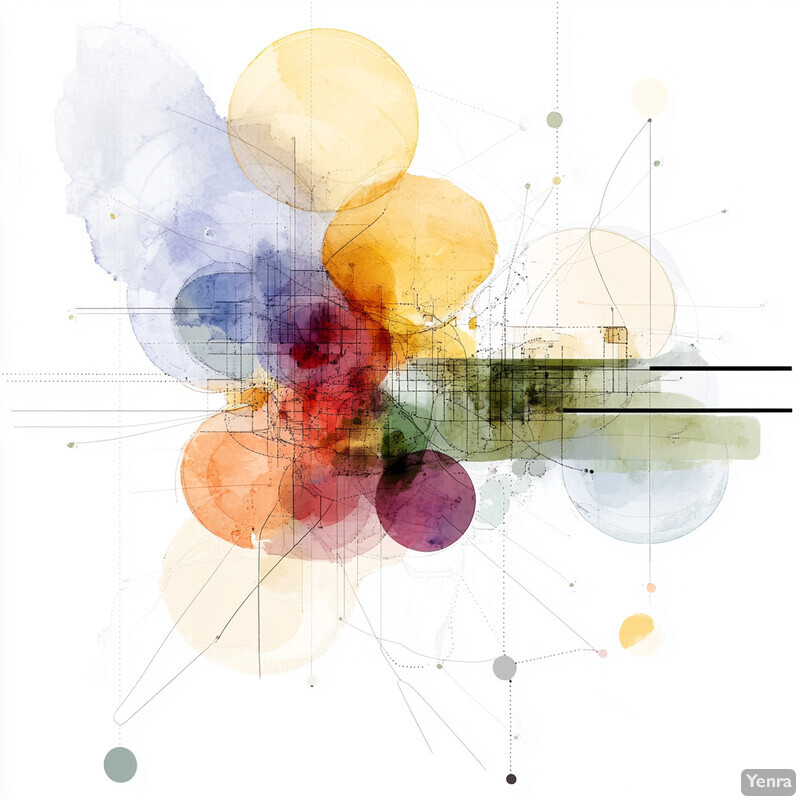
Identifying patterns in how land is used can reveal underlying problems or opportunities. AI-based clustering and pattern recognition algorithms scan maps and datasets to detect trends in settlement distribution, pollution sources, soil erosion hotspots, and more. Insights gained can guide targeted interventions—such as reforestation in areas prone to erosion or strategic public transportation routes to reduce traffic congestion. Recognizing these patterns is crucial for understanding how human activities interact with the environment, paving the way for more nuanced and effective land management strategies.
13. Infrastructure Placement Optimization
AI helps determine the best locations for roads, utilities, and public services, reducing construction costs, minimizing environmental disruption, and ensuring equitable access.
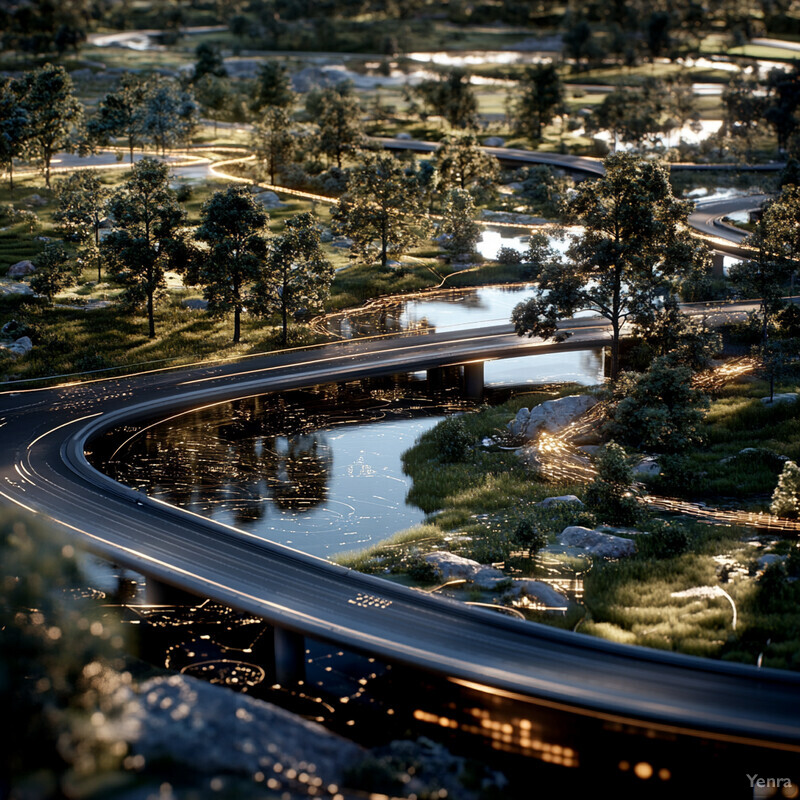
Infrastructure—from roads and pipelines to schools and hospitals—shapes land use patterns and influences accessibility, economic activity, and quality of life. AI models can consider numerous factors (population density, land cost, environmental sensitivities, projected growth) to propose optimal placement and design of infrastructure networks. The result is a layout that reduces travel times, improves public services, lowers environmental impact, and ensures equitable resource distribution. In this way, AI transforms the traditional trial-and-error approach into a more strategic, evidence-based process.
14. Carbon Footprint Analysis
Machine learning models evaluate how different land uses influence carbon sequestration and emissions, aiding in the development of carbon-neutral or carbon-negative land management strategies.
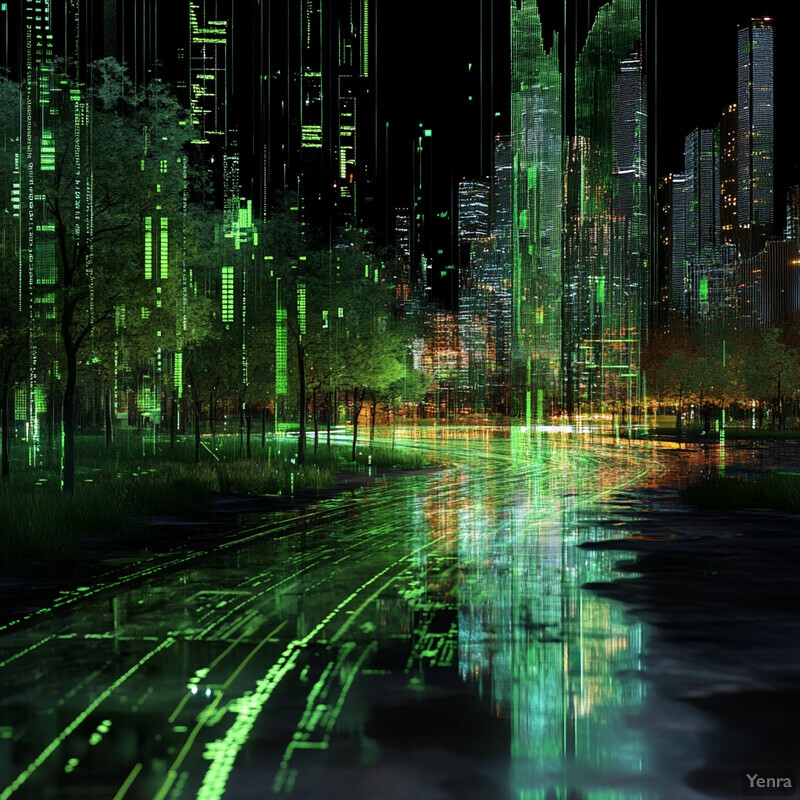
With growing interest in sustainability and climate goals, evaluating land use in terms of carbon sequestration and emissions is a priority. Machine learning models can estimate how different land use scenarios—afforestation, wetland restoration, urban greening—affect carbon storage and greenhouse gas emissions. By helping planners choose land uses that reduce net carbon footprints, AI supports climate mitigation strategies and aligns land management with national and global climate targets. This alignment ensures that land planning contributes positively to environmental stewardship and sustainability goals.
15. Cultural and Social Sensitivity Modeling
By integrating survey data, demographic information, and cultural heritage maps, AI ensures that proposed land uses respect community values, cultural sites, and social well-being.
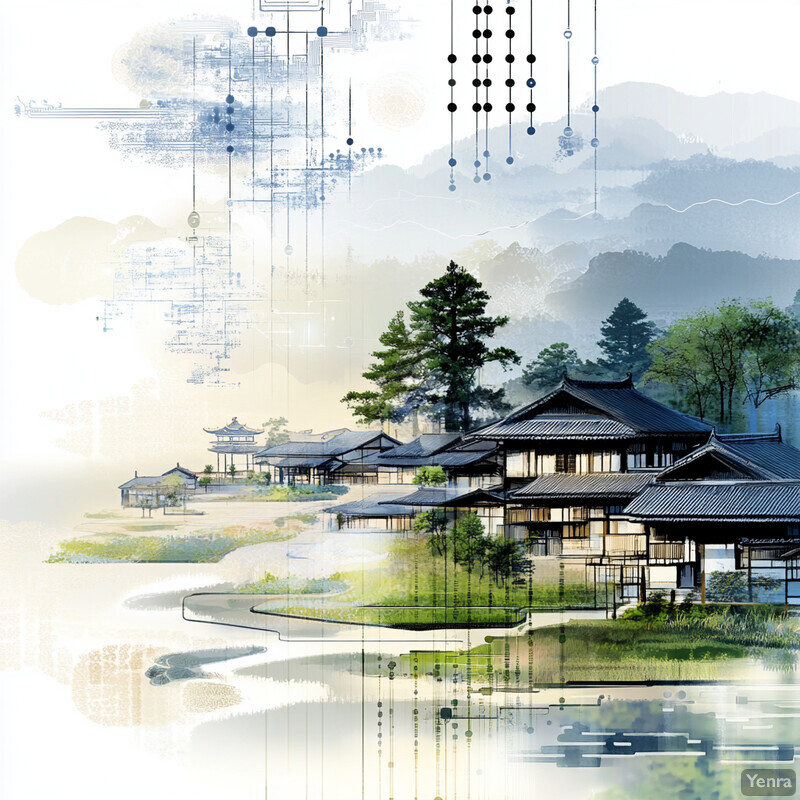
Land is not only an environmental and economic resource; it also has cultural and social significance. AI can incorporate qualitative data—such as survey responses, public meeting transcripts, and ethnographic studies—into its optimization frameworks. By doing so, it ensures that proposed land uses respect local traditions, religious sites, historical landmarks, and community values. This human-centered approach helps prevent conflicts, respects indigenous knowledge, and fosters community trust in land use planning processes, ultimately leading to more inclusive and enduring solutions.
16. Land Value Forecasting
Predictive analytics tools forecast future land values based on economic trends, infrastructure development, and demographic shifts, helping investors, planners, and policymakers allocate land more efficiently.
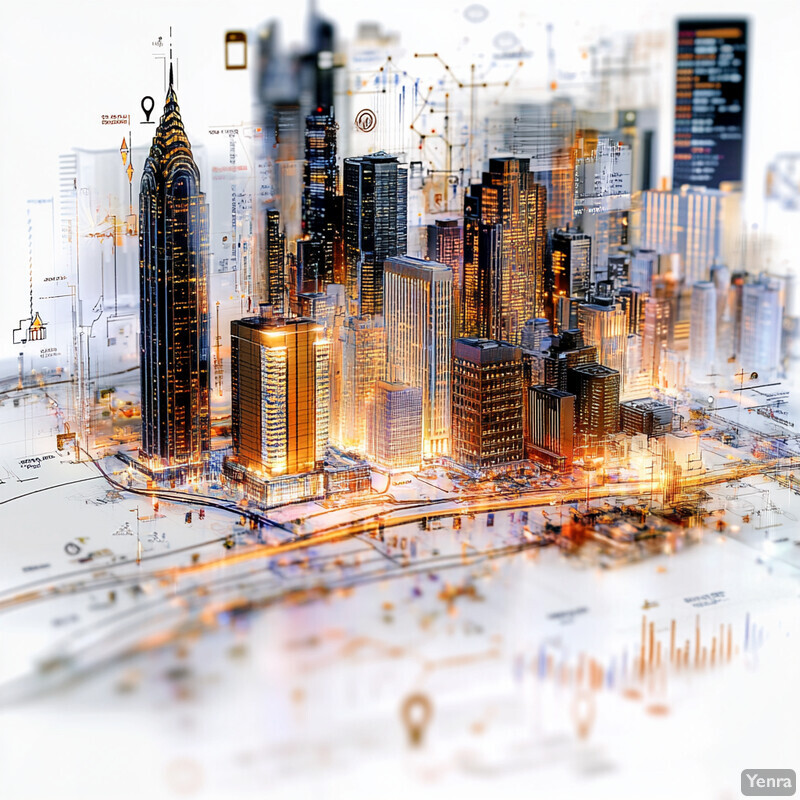
Understanding how land values evolve over time can guide investment decisions and policy interventions. AI-driven forecasting tools use historical sales data, infrastructure developments, demographic changes, and economic trends to predict future land values. Armed with this information, policymakers can strategically plan public infrastructure projects to maximize long-term returns, while private investors can identify high-potential areas for development. Such forecasts also inform property tax policies and financial planning, ensuring that land use decisions are economically sound and sustainable.
17. Precision Conservation
AI-based terrain and vegetation analysis can prioritize conservation efforts to protect the most ecologically significant areas, optimizing the investment of limited conservation resources.
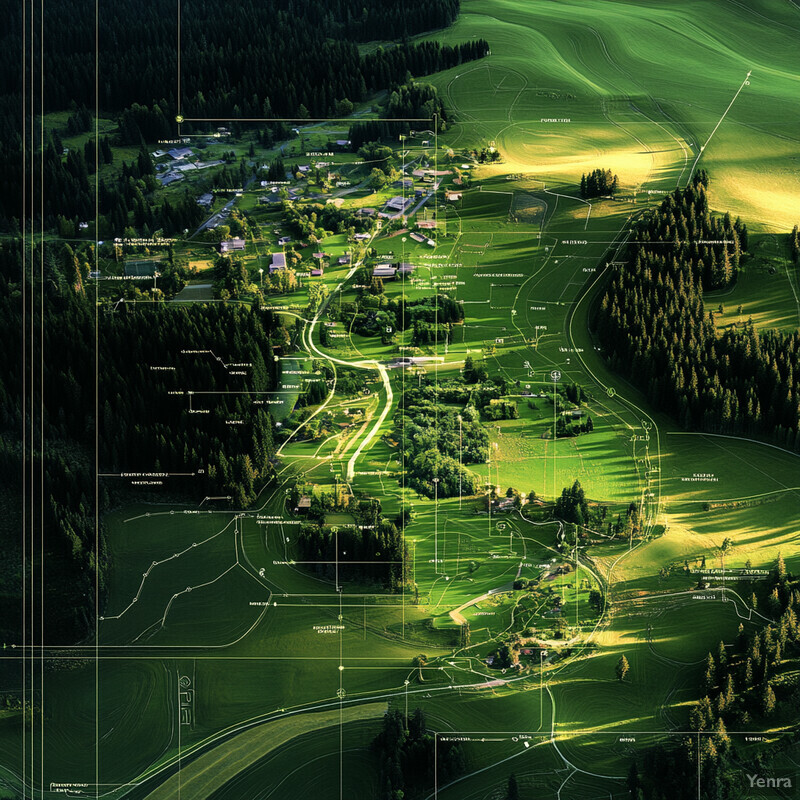
Conservation budgets are often limited, necessitating targeted and strategic allocation of resources. AI can identify which areas provide the greatest ecological benefit if conserved, such as habitats essential for threatened species or regions that maintain crucial ecosystem services like pollination or water purification. By directing conservation efforts to the most impactful areas, stakeholders can do more with fewer resources—thus maximizing biodiversity protection while leaving other lands available for sustainable development. Precision conservation ensures that every conservation dollar spent yields substantial ecological returns.
18. Sustainable Intensification of Agriculture
Machine learning can determine where intensifying agriculture (e.g., through vertical farming or precision irrigation) makes sense, thus reducing land conversion and protecting natural habitats.
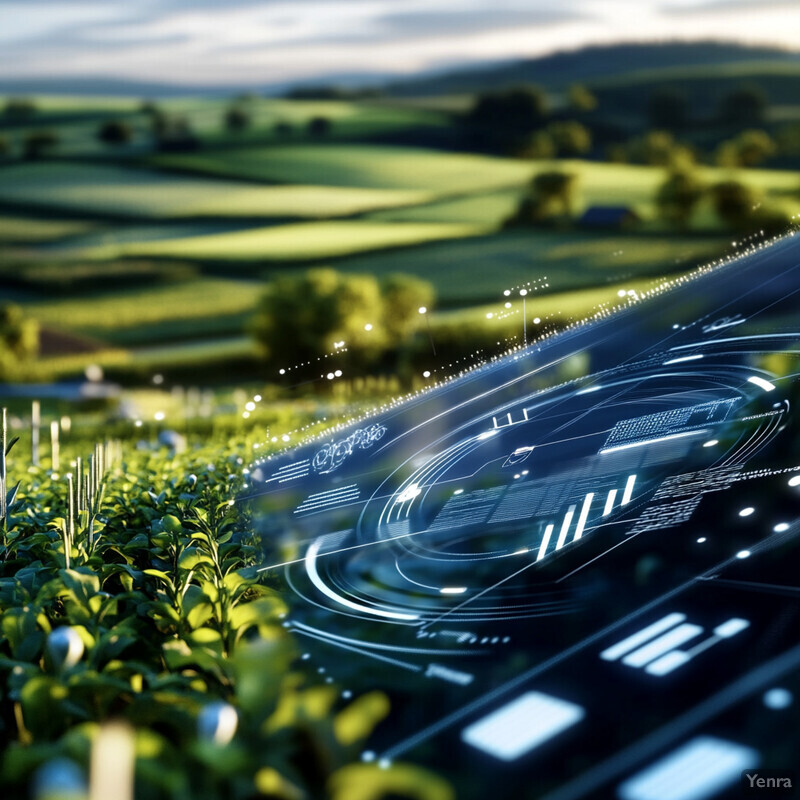
To feed a growing global population without expanding farmland, we must intensify agriculture sustainably. AI guides this process by identifying where advanced techniques—like precision irrigation, vertical farming, improved crop rotations, or agroforestry—can be most effectively implemented. By making agriculture more productive without enlarging its footprint, AI helps protect natural habitats and reduces pressure on forests and wetlands. Over time, sustainable intensification can increase resilience to climate shocks, improve food security, and stabilize rural economies.
19. Energy Infrastructure Siting
AI models facilitate the identification of optimal sites for wind, solar, and hydro installations, balancing energy yields, environmental impacts, and land-use trade-offs.
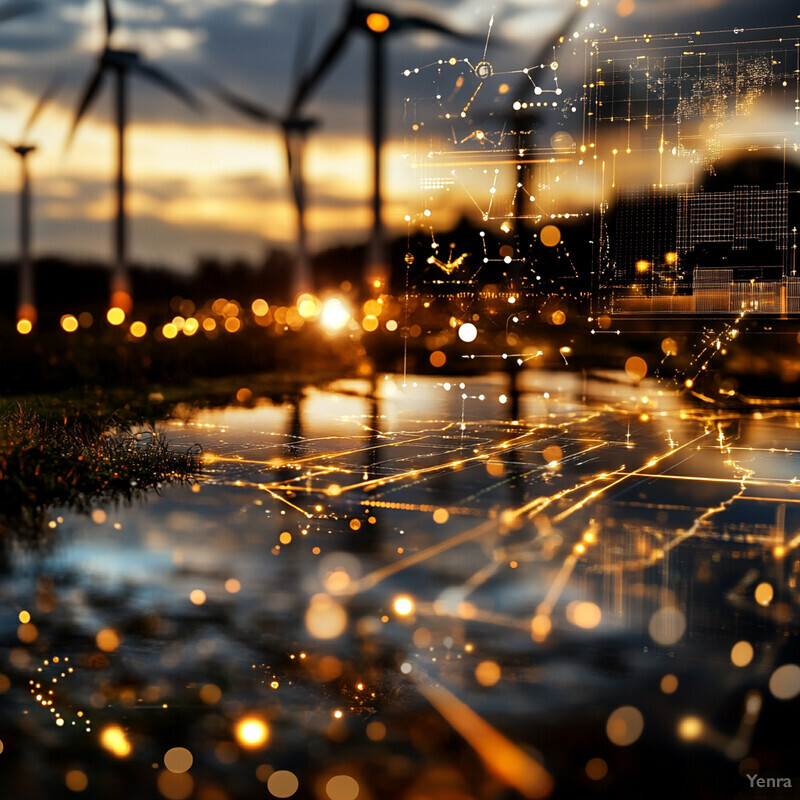
Renewable energy projects like solar farms, wind turbines, and hydroelectric facilities require optimal siting to balance energy yield, ecological impact, and local community concerns. AI can analyze factors such as wind speed distributions, solar irradiance patterns, transmission line proximity, land cost, and wildlife migration routes to pinpoint ideal locations. By ensuring that energy installations are both efficient and environmentally responsible, AI helps accelerate the transition to clean energy while minimizing land use conflicts and ecological damage.
20. Stakeholder Engagement Tools
Natural language processing and sentiment analysis tools interpret community feedback from public consultations, incorporating residents’ preferences directly into AI-driven land-use optimization models.
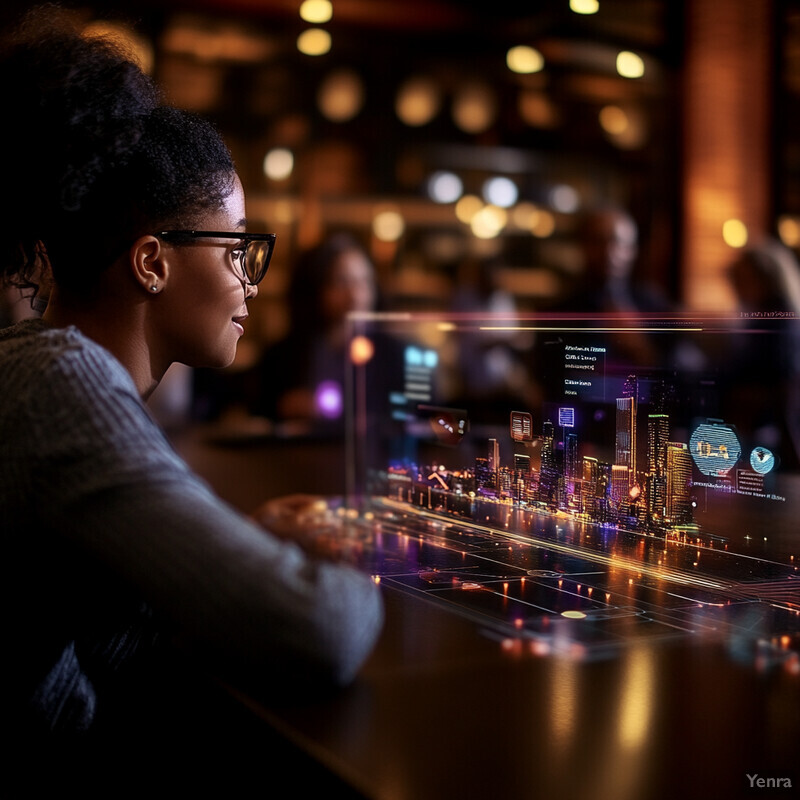
Effective land use planning must incorporate input from diverse stakeholders—local communities, environmental groups, industry representatives, and government agencies. AI-driven natural language processing and sentiment analysis tools can process public comments from open forums, social media platforms, and community surveys to extract key concerns and preferences. This feedback can then be integrated into land use optimization models, ensuring that policy outcomes reflect the values and priorities of those affected. Enhanced stakeholder engagement builds legitimacy and trust, paving the way for smoother implementation of land use plans.