1. Real-time Satellite Image Analysis
AI-driven computer vision algorithms can process satellite imagery rapidly to detect early signs of storms, hurricanes, and other weather anomalies, improving lead times for warnings.
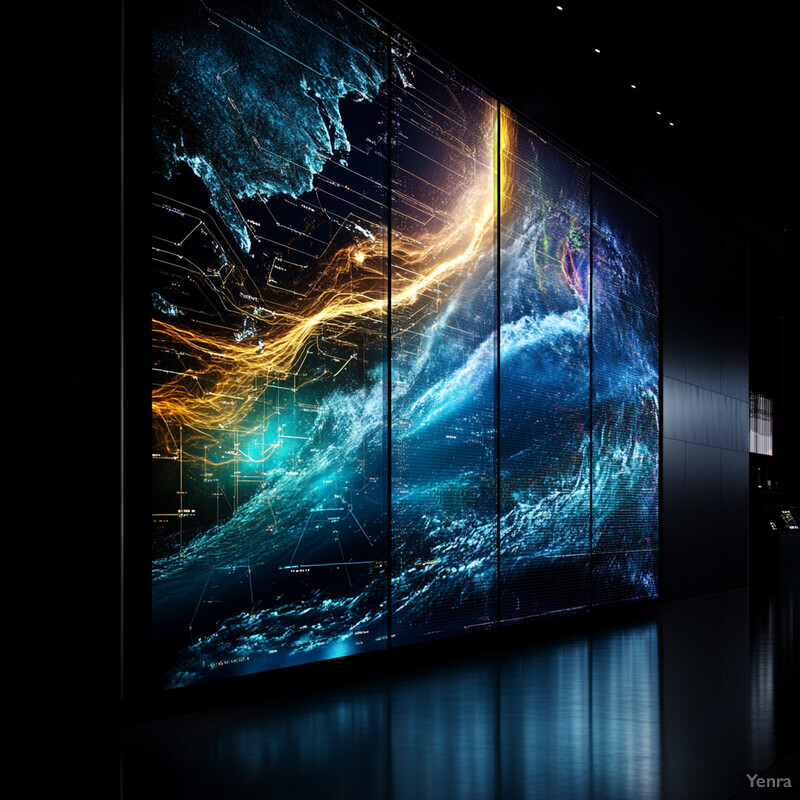
AI-driven image recognition systems can process incoming data from satellites almost instantaneously, identifying subtle shifts in cloud patterns, changes in water vapor content, and variations in ground temperature. By leveraging machine learning algorithms trained on extensive historical datasets, these systems can spot evolving storms, forming cyclones, and early indicators of weather anomalies long before human analysts could. Additionally, AI can continuously monitor multiple sources, blending them into a cohesive picture of atmospheric conditions. This rapid, large-scale analysis allows meteorologists and disaster management agencies to issue preemptive warnings, improving the timeliness and accuracy of responses to imminent natural events.
2. Enhanced Weather Forecasting Models
Machine learning models integrate vast datasets—including atmospheric, oceanic, and terrestrial information—to generate more accurate and granular weather predictions, which help anticipate extreme weather events.
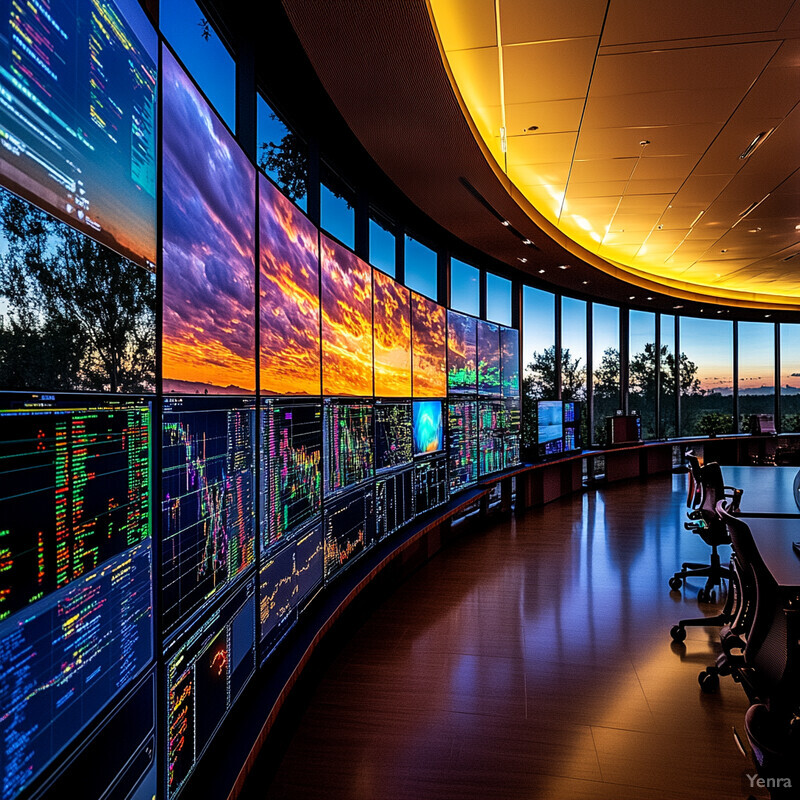
Traditional weather forecasting models rely heavily on complex physics-based calculations, which, while accurate, can be computationally intensive and slower to update. AI-enhanced models integrate machine learning techniques that sift through vast amounts of meteorological and climatological data more efficiently. By identifying patterns hidden in historical weather data, these models can improve short-term forecasts—such as predicting localized heavy rainfall or sudden temperature shifts—and produce more precise long-term outlooks. As a result, communities benefit from actionable intelligence that guides resource allocation, infrastructure planning, and public safety measures well before conditions reach critical thresholds.
3. Early Earthquake Detection
Advanced AI techniques can identify subtle seismic patterns from large sensor networks, enabling the rapid detection of initial quake waves and issuing early warnings before the most damaging waves arrive.
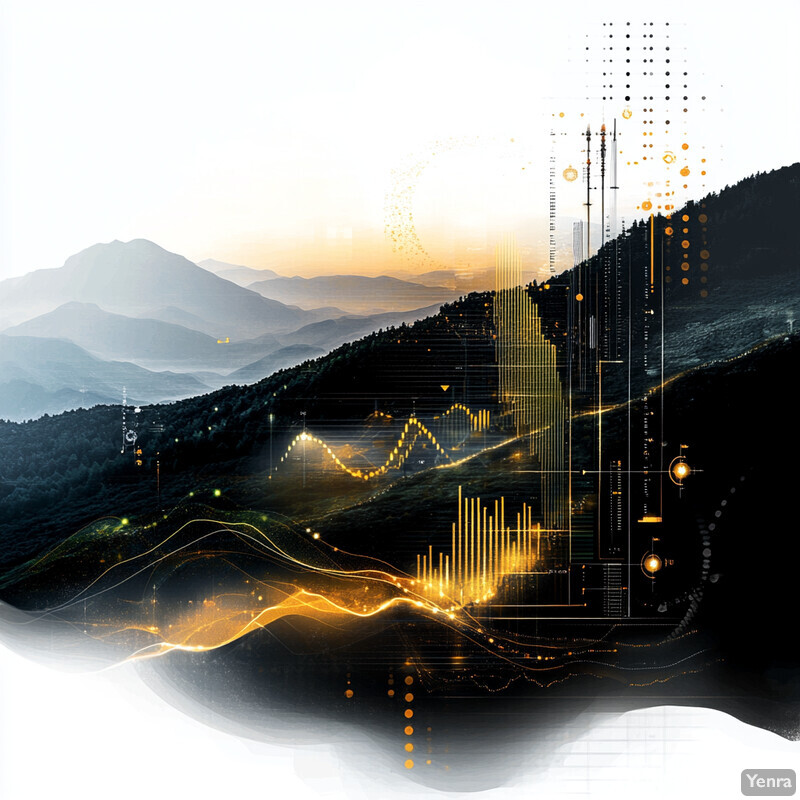
Seismic sensor networks generate massive amounts of raw data, and identifying the earliest signatures of seismic activity is crucial for issuing timely warnings. AI algorithms trained to recognize precursory seismic waves can detect minute vibrations, foreshocks, or the initial release of stress along fault lines. Once flagged, these early indicators can trigger automated alerts, giving populations precious seconds—or even minutes—to prepare for the main shock. Early detection also aids critical infrastructure controls, such as halting trains, securing industrial processes, and alerting hospitals to brace for potential surges in demand, ultimately reducing casualties and damage.
4. Improved Flood Prediction
AI models can combine precipitation forecasts, river flow data, topographical maps, and soil moisture readings to predict when and where flooding might occur, giving communities time to evacuate.
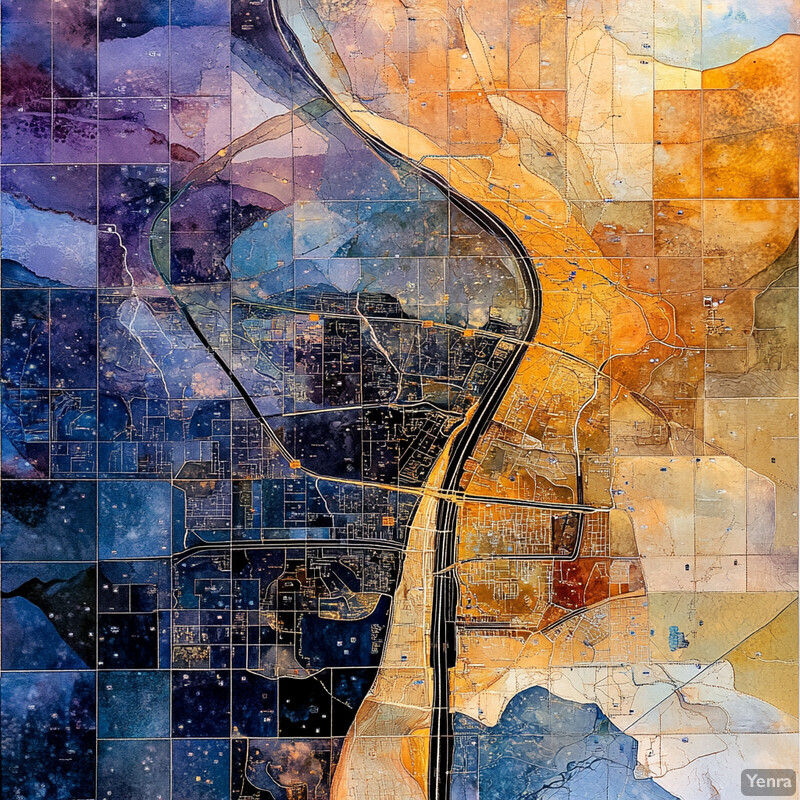
Predicting floods is inherently challenging due to the complex interplay of precipitation, terrain, soil saturation, and river hydrodynamics. AI-powered models take into account radar-based rainfall measurements, digital elevation maps, soil moisture sensors, and historical flooding patterns. By blending these data streams, machine learning algorithms can forecast when and where rivers will crest or stormwater runoff will overwhelm drainage systems. These insights allow authorities to issue evacuation orders, reinforce flood defenses, and strategically deploy emergency response teams. Enhanced flood predictions minimize economic losses, safeguard agricultural lands, and ultimately save lives.
5. Storm Surge and Tsunami Forecasting
Neural networks can be trained on historical oceanic and seismic data to predict the scale and timing of storm surges or tsunamis, allowing for quicker coastal evacuations.
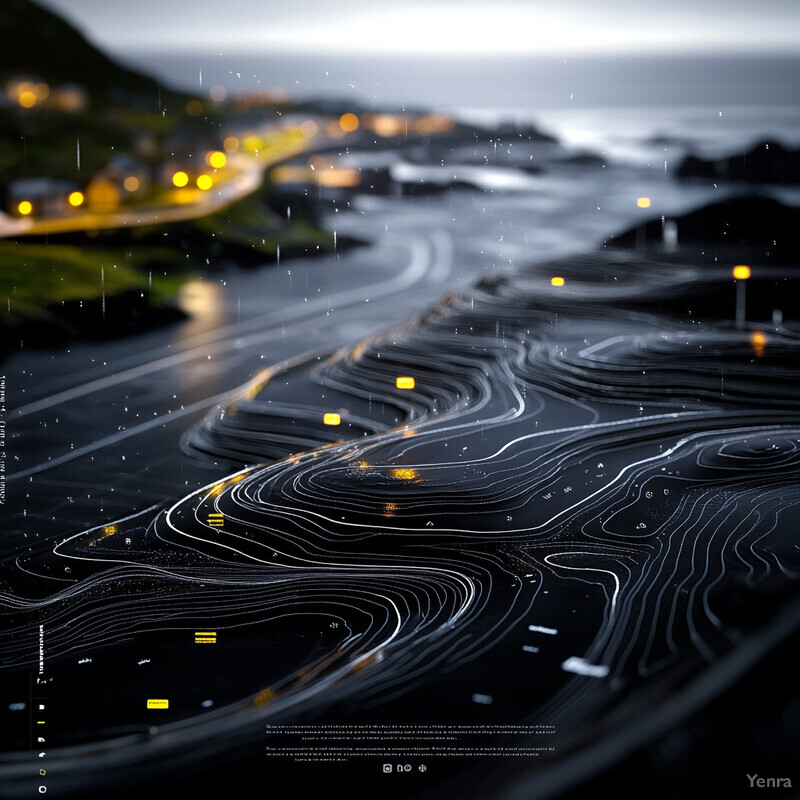
Coastal communities face severe risks from storm surges and tsunamis, where minutes of warning can be the difference between life and death. AI-based forecasting tools analyze oceanographic data, wave dynamics, wind speed, and seismic readings to predict how water levels might rise following cyclones, hurricanes, or undersea earthquakes. These systems learn from historical events, refining their predictive accuracy with each new data point. By delivering timely alerts, emergency managers can close roads, ready evacuation shelters, and implement protective measures such as deploying temporary flood barriers. Ultimately, AI-driven surge and tsunami forecasting offers communities a vital head start against rapidly advancing coastal threats.
6. Volcanic Activity Monitoring
AI can analyze tremor patterns, gas emissions data, and thermal imagery to better predict volcanic eruptions, providing local authorities with early warnings and evacuation guidance.
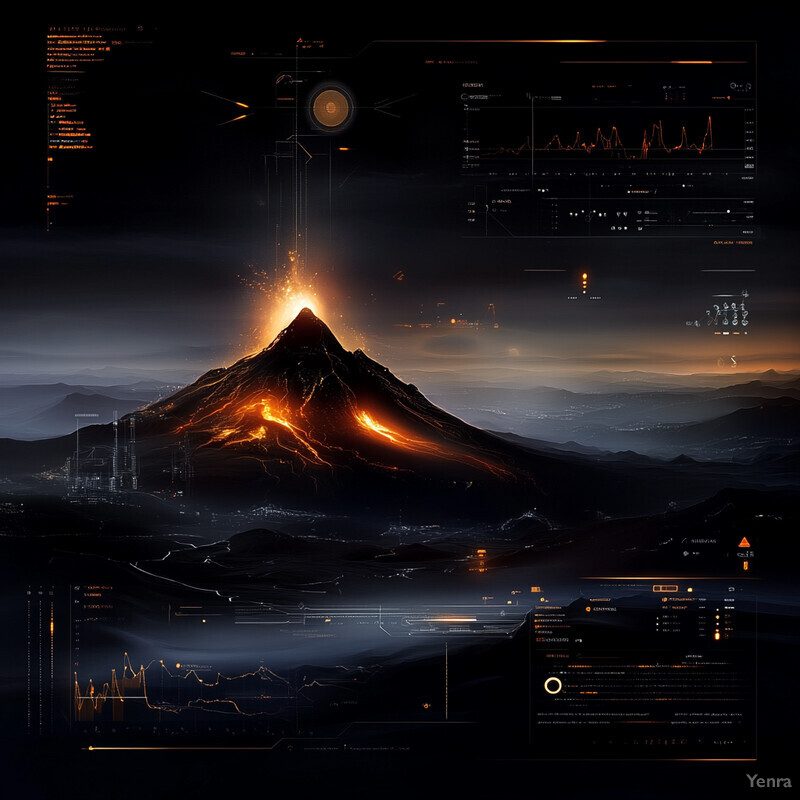
Volcanic eruptions often provide subtle clues before they occur, ranging from increased seismic activity beneath a volcano’s edifice to shifts in gas composition and heat emissions. AI can aggregate and interpret these signals in real time, distinguishing between routine geological fluctuations and genuine warning signs of an impending eruption. By examining patterns from previous eruptions, AI-driven models become adept at forecasting both the timing and potential magnitude of future events. Early warnings give residents and authorities the opportunity to arrange evacuations, secure critical infrastructure, and minimize the far-reaching impacts of ash falls, pyroclastic flows, and lahars.
7. Wildfire Spread Modeling
Machine learning algorithms can forecast wildfire trajectories by integrating weather forecasts, vegetation density, and topographic information. This helps firefighters and emergency planners allocate resources efficiently.
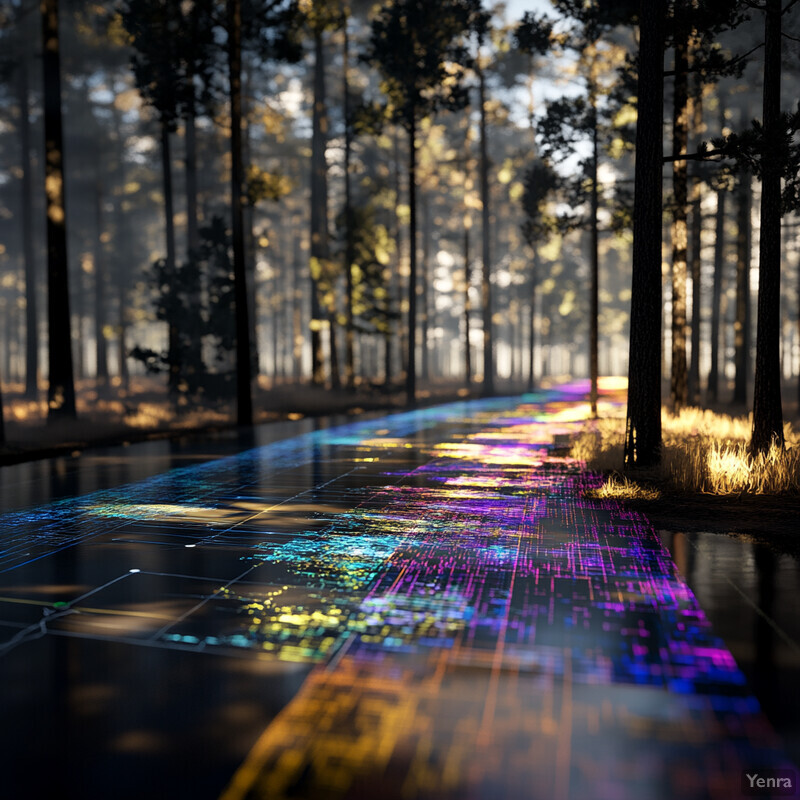
Wildfires can ignite and spread rapidly under conditions influenced by wind direction, humidity, vegetation health, and terrain features. AI-based modeling tools integrate weather forecasts, satellite imagery, and sensor data from remote camera networks to predict how fires will move and grow. Machine learning algorithms simulate various scenarios to estimate the rate of spread, hotspots of intensity, and potential trajectories. With this information, firefighters can position crews and equipment where they are most needed, land managers can preemptively clear vegetation in vulnerable areas, and policymakers can issue timely evacuation orders. As a result, the damage from wildfires can be reduced, saving both lives and natural habitats.
8. Landslide Susceptibility Analysis
AI-driven risk maps incorporate rainfall patterns, soil stability metrics, slope conditions, and land usage data to identify areas prone to landslides well before they occur.
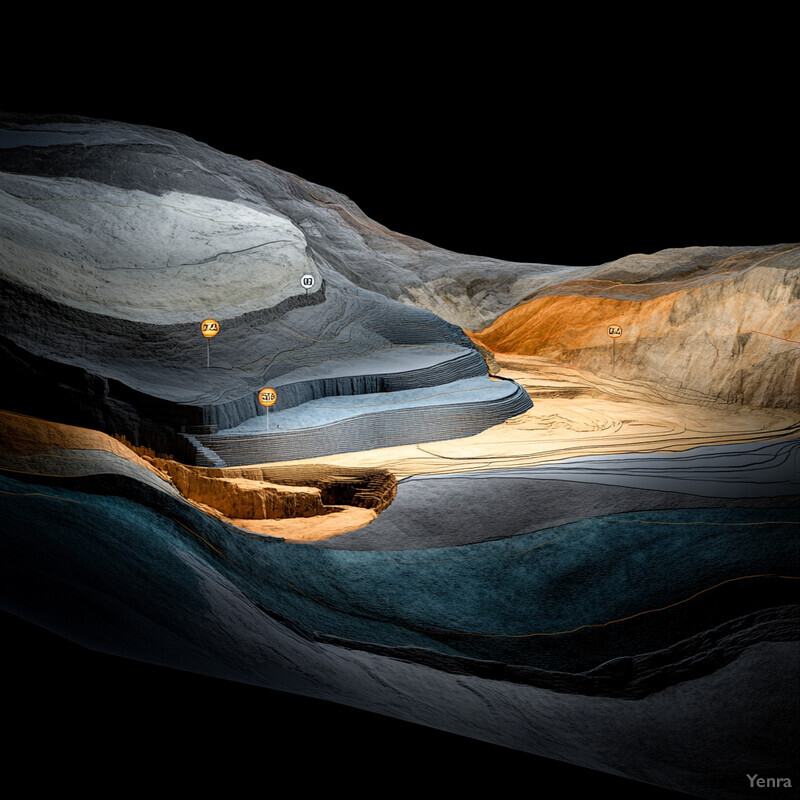
Landslides result from a complex interplay of topography, geology, rainfall, and land use. AI-driven models assimilate data on slope gradients, soil composition, vegetation cover, and recent weather patterns to create accurate, area-specific risk maps. By learning from past events, these models identify patterns that precede slope failures and pinpoint the regions most susceptible to future landslides. Such insights enable authorities to prioritize stabilization projects, direct the installation of early warning sensors, and guide infrastructure development away from high-risk zones. In turn, communities receive timely alerts to evacuate or reinforce vulnerable structures before the earth moves.
9. Integration of Multimodal Data Sources
AI systems can simultaneously process data from sensors, satellites, social media feeds, radar networks, and historical records to create a holistic early warning platform.
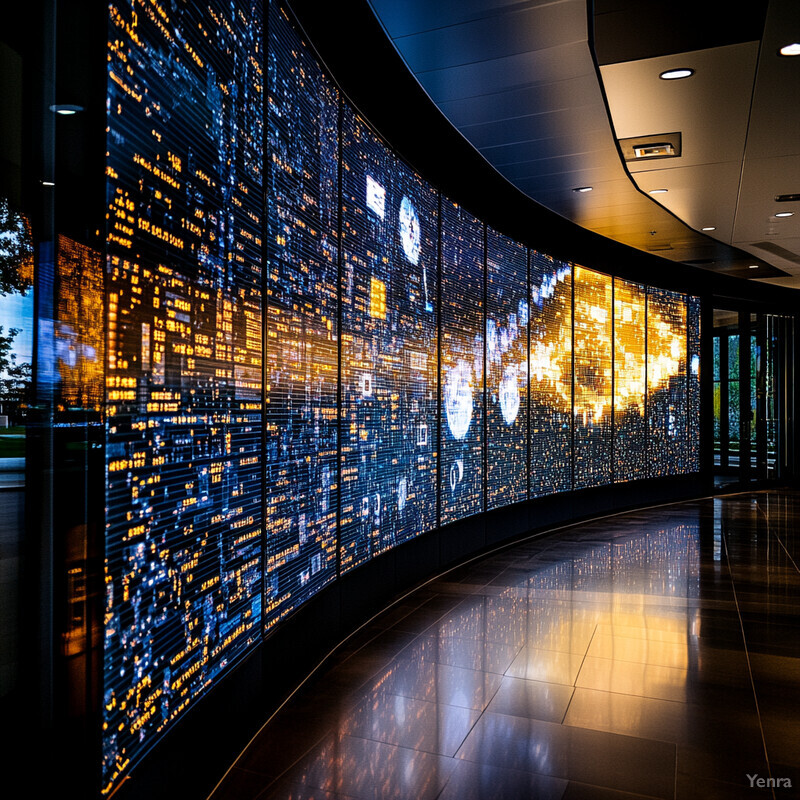
In the realm of disaster prediction, no single data source is sufficient. By integrating satellite imagery, ground-based sensor readings, radar scans, weather station outputs, and even crowdsourced reports, AI systems achieve a holistic understanding of evolving conditions. Advanced fusion techniques draw connections between disparate inputs, refining accuracy and reducing false alarms. With a comprehensive environmental view, these AI platforms can provide more nuanced forecasts and richer situational awareness. This integrated approach ensures that early warning systems not only detect the signs of an impending disaster but also understand its potential scale and impacts.
10. Adaptive Thresholding for Alerts
Intelligent systems adjust alert thresholds dynamically based on evolving conditions—such as changing wind direction or updated precipitation forecasts—ensuring warnings remain relevant and timely.
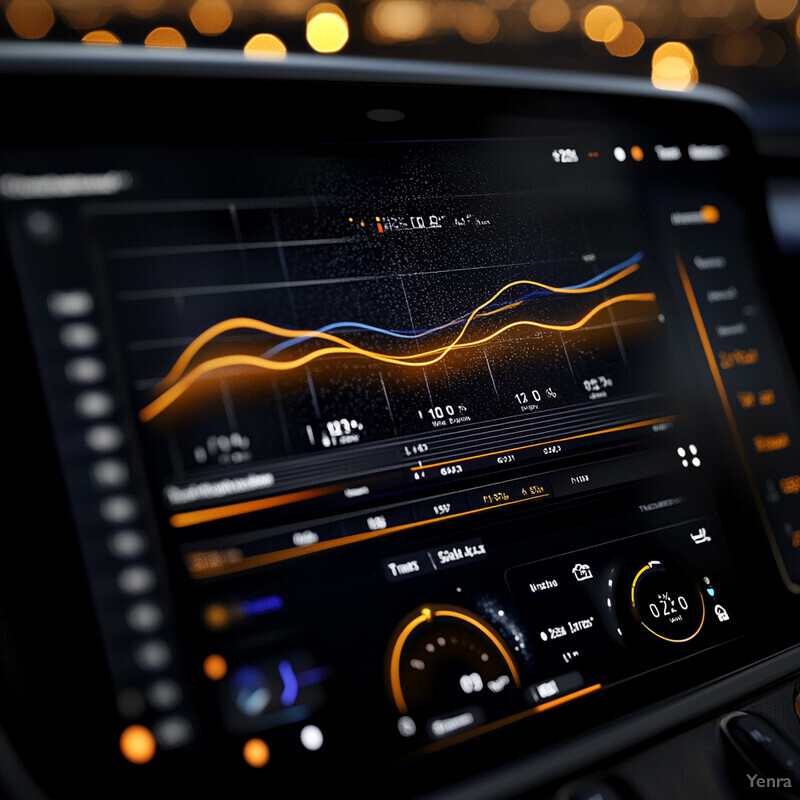
Static thresholds for issuing warnings often fail to capture the nuances of evolving environmental conditions. AI introduces adaptive thresholding, meaning that the parameters triggering an alert are automatically adjusted based on real-time data. If wind speeds unexpectedly shift, humidity spikes, or rainfall intensifies, the system recalibrates to ensure warnings are neither premature nor delayed. This approach reduces false positives—preventing unnecessary panic—and decreases false negatives, ensuring that communities receive timely alerts. Ultimately, adaptive thresholding leads to a more responsive early warning framework that instills trust and encourages prompt action.
11. Predictive Maintenance of Sensors
Machine learning models can detect early signs of sensor malfunction or data anomalies, ensuring that critical seismic or meteorological instrumentation is always functioning optimally.
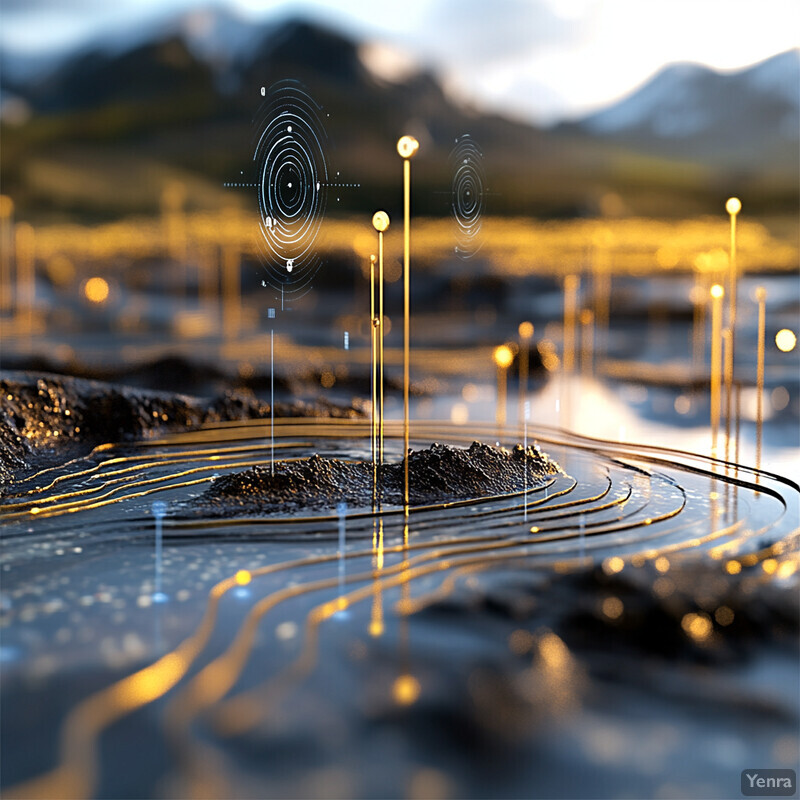
Early warning systems depend on a network of reliable sensors—from seismic stations to weather buoys. AI algorithms monitor the health and performance of these devices by analyzing data quality, detecting anomalies that might indicate sensor drift, damage, or power issues. When a fault is predicted, maintenance crews can intervene before the sensor fails, ensuring that critical infrastructure remains operational. By keeping data streams uninterrupted, these systems maintain a high level of situational awareness, enabling more accurate and timely hazard detection. Reliable sensor networks are the backbone of effective early warning strategies.
12. Automated Risk Assessment
AI can evaluate the vulnerability of infrastructure, population density, and community resilience factors to identify which areas need prioritized warnings and support measures.
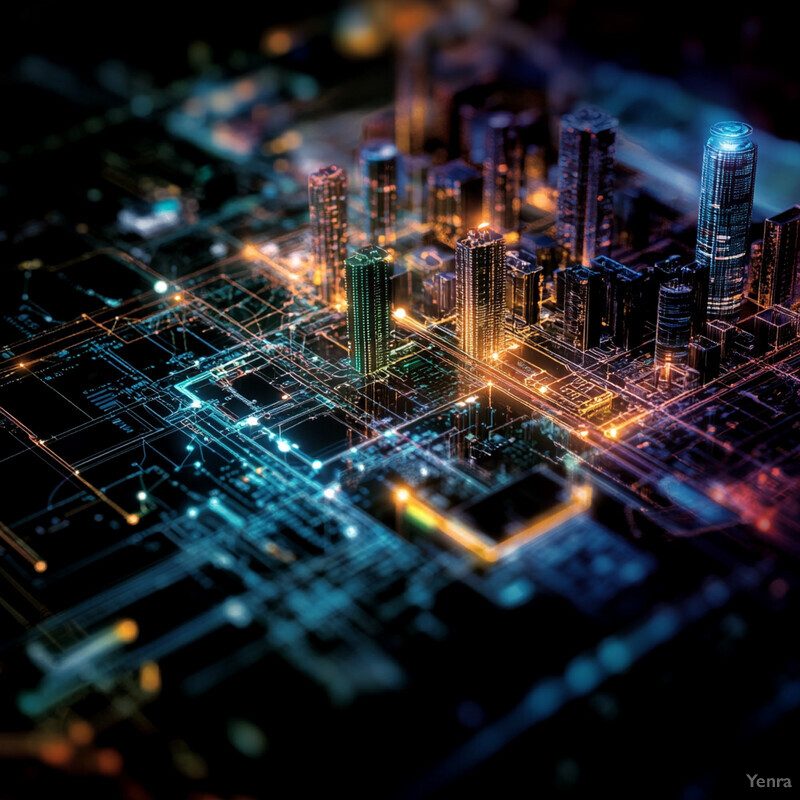
AI can combine hazard forecasts with socioeconomic and infrastructural datasets to perform comprehensive risk assessments. By factoring in population density, building codes, economic assets, and vulnerability indices, machine learning models produce an integrated picture of where the greatest threats lie. This allows policymakers and emergency managers to focus efforts where they will have the most impact, such as reinforcing dams in flood-prone areas or providing evacuation resources to low-mobility populations. Automated risk assessments guide investment in mitigation measures, disaster preparedness training, and long-term resilience planning, ensuring that communities are better prepared before a warning even needs to be issued.
13. Long-term Climate Trend Analysis
By analyzing large historical datasets, AI can identify long-term trends and shifts in weather patterns—anticipating increased frequency or intensity of certain hazards—thus improving early warning frameworks.
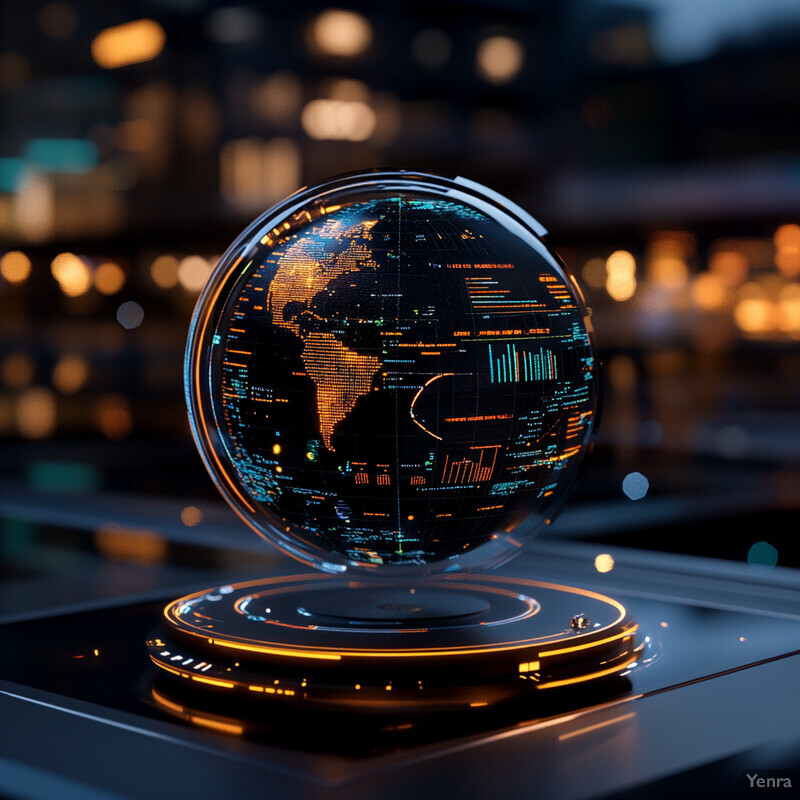
Some natural hazards, like droughts and coastal erosion, are tied to shifting climate patterns that evolve over decades. AI excels at finding subtle trends in large, complex datasets, including historical weather records, satellite observations, and greenhouse gas measurements. These analyses help experts project how climate change may alter the frequency, intensity, or geographical distribution of disasters. By recognizing these long-term shifts early, communities can adapt their infrastructure, agricultural practices, and disaster preparedness programs. In essence, AI provides foresight into future risks, enabling strategies that reduce vulnerability over generations.
14. Optimized Evacuation Routing
Intelligent algorithms can model traffic flow, road conditions, and available shelters in real-time, helping emergency managers design effective evacuation routes when warnings are issued.
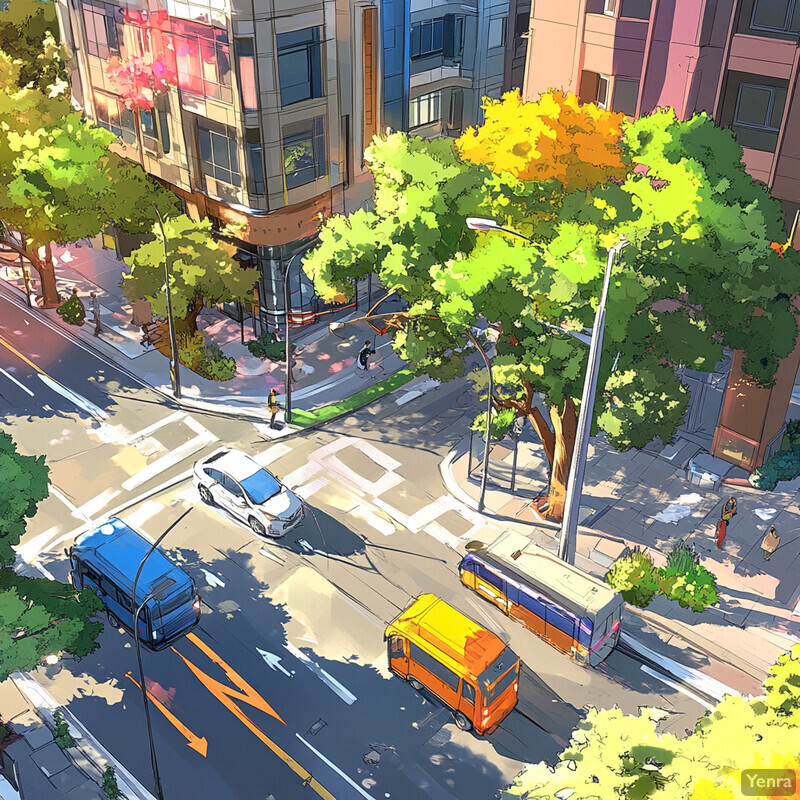
When the window between hazard detection and impact is narrow, well-planned evacuations can mean the difference between safety and catastrophe. AI models can simulate traffic flow in real time, factoring in road capacity, driver behavior, and vehicle distribution. They can also incorporate changing weather conditions, blocked roads, and available shelter space. Using these parameters, machine learning algorithms suggest optimal evacuation routes, reducing congestion and speeding up population movement away from danger zones. The result is more orderly evacuations, fewer bottlenecks, and improved chances that everyone reaches safety promptly.
15. High-resolution Forecasting
Advances in AI-driven modeling can downscale global climate models to local resolutions, improving the precision of predictions and delivering earlier and more accurate region-specific warnings.
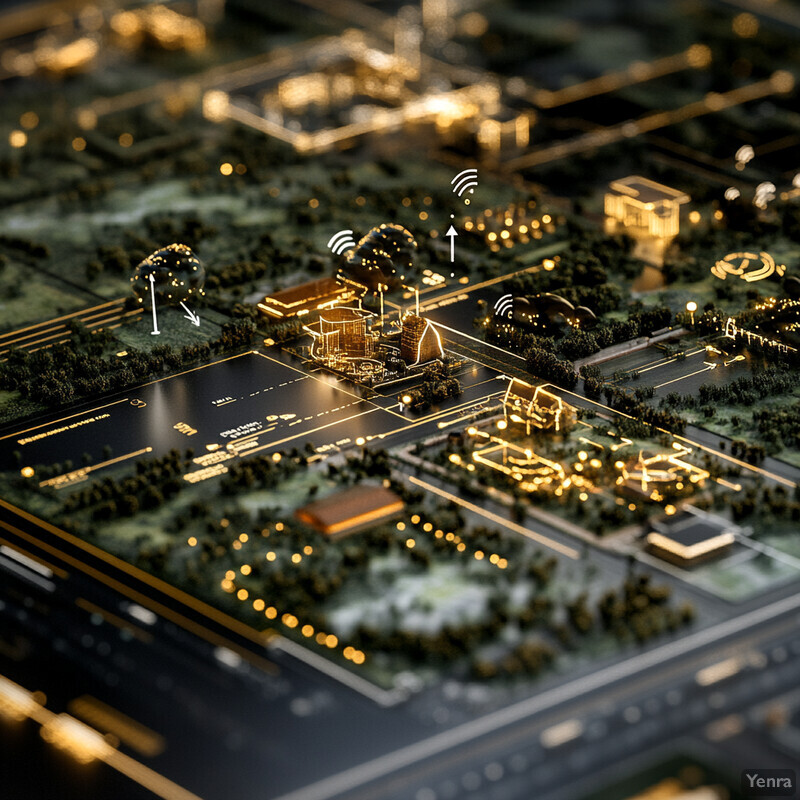
Global climate and weather models produce valuable insights but often lack the fine spatial resolution needed for localized decision-making. AI-driven downscaling techniques take coarse global data and refine it to yield detailed forecasts at the scale of a single valley, neighborhood, or watershed. This granular view of conditions allows emergency planners to predict which parts of a city might flood first or which rural communities will be hit hardest by a heatwave. High-resolution forecasting transforms general warnings into pinpoint advisories, increasing their relevance and usefulness for on-the-ground responders and community leaders.
16. Probabilistic Hazard Forecasting
AI enables the estimation of event likelihood and intensity with confidence intervals, aiding decision-makers in understanding the certainty and severity of impending disasters.
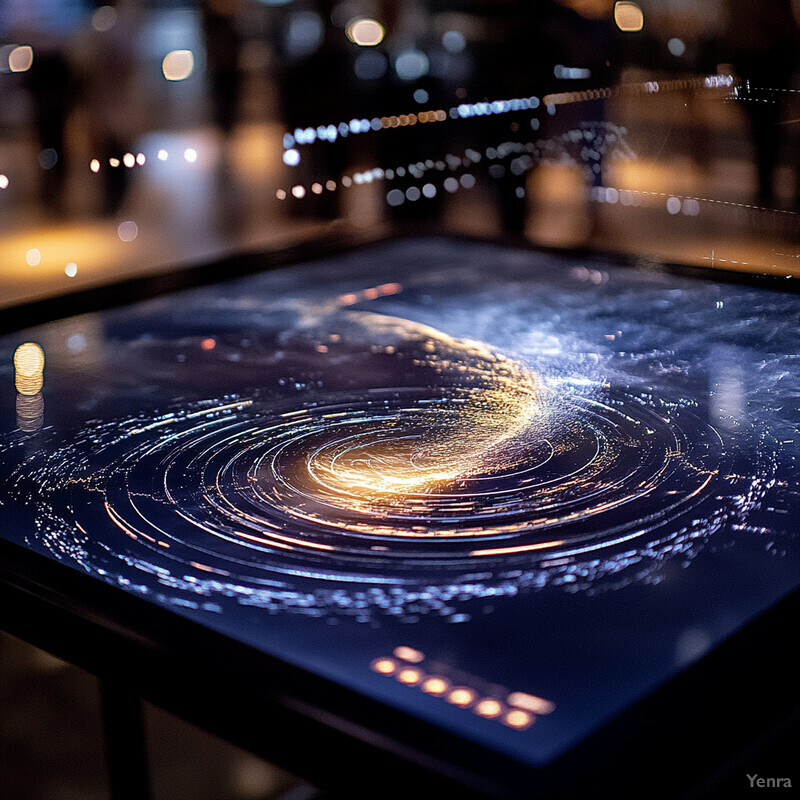
Instead of issuing binary, all-or-nothing predictions, AI can produce probabilistic forecasts that show the likelihood of different outcomes. For example, there may be a 70% chance that a hurricane will intensify beyond a certain category or a 30% chance that a landslide will occur on a particular hillside. These probability-based warnings equip decision-makers with a clearer understanding of uncertainty, helping them balance the costs of preventive action against the risks. Knowing the probabilities enables more nuanced disaster preparedness strategies, encouraging proactive but measured responses.
17. Social Media and Public Data Insights
Machine learning can detect early warning signals hidden in social media posts, local news, and community reports—helping authorities identify emerging threats faster than traditional monitoring methods.
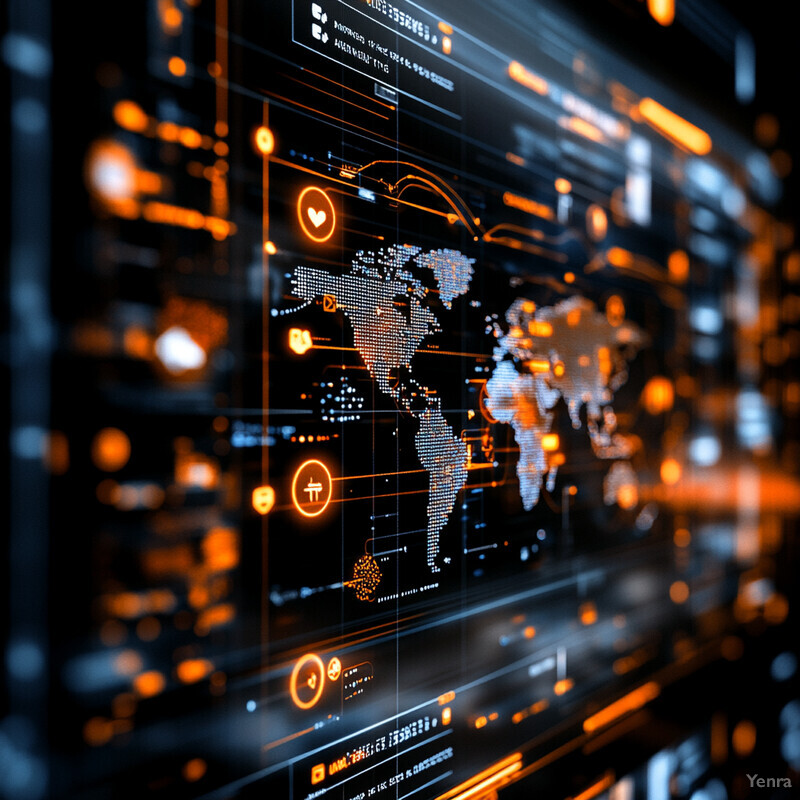
In the early stages of a developing disaster, information from citizens on social media and local community forums can provide the first signals of trouble. AI natural language processing tools can sift through millions of posts, detecting keywords and sentiment changes that point to emerging hazards. For example, a sudden surge in mentions of unusual smoke in a region could indicate a new wildfire. By incorporating these unconventional data sources into official warning systems, emergency managers gain ground-level intelligence, improving both the speed and accuracy of their alerts.
18. Automated Decision Support Systems
AI can fuse predictive analytics with decision-making rules, automatically recommending actions such as issuing evacuation alerts or mobilizing emergency response teams.
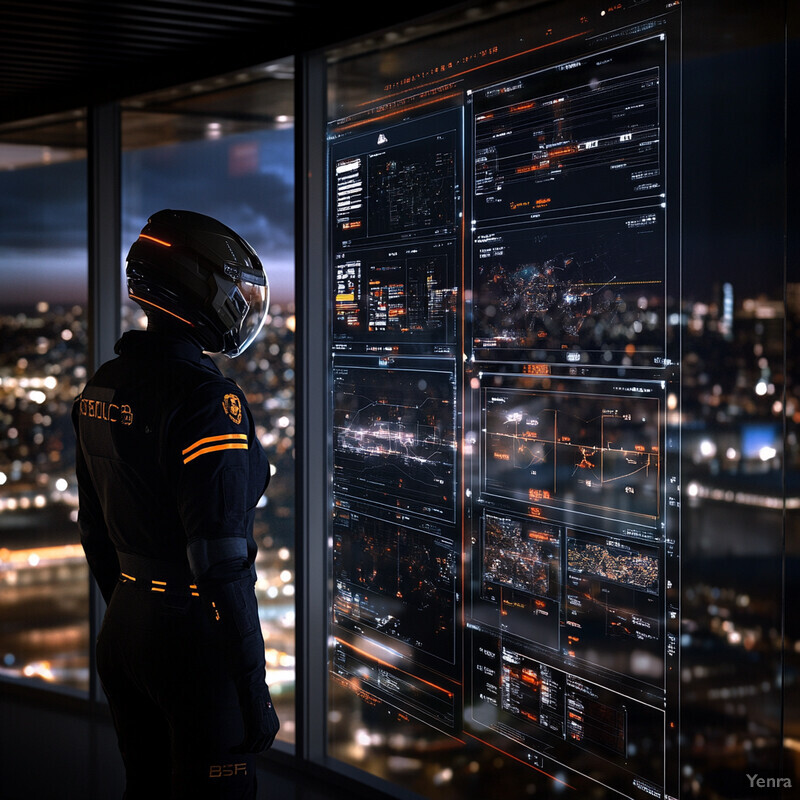
Early warning is only as good as the actions it prompts. AI-driven decision support systems combine predictive analytics with predefined emergency protocols to recommend concrete steps. For instance, if sensors indicate imminent flooding, the system might automatically suggest closing specific roads, sending text alerts to residents, and deploying rescue boats. By streamlining the decision-making process and removing guesswork, these AI tools ensure that emergency responses are both timely and effective. This reduces the cognitive load on human operators and allows them to focus on coordination and communication.
19. Scenario Simulations and Training
AI tools can generate realistic disaster scenarios for training emergency personnel and policymakers, improving the quality and speed of responses once early warnings are activated.
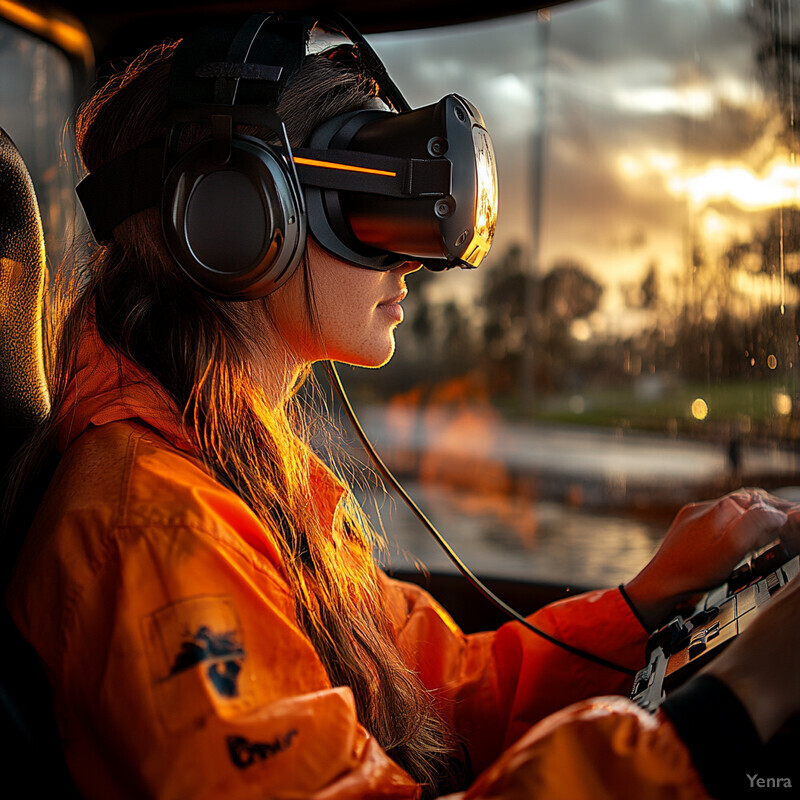
AI can generate realistic disaster scenarios—such as hypothetical earthquakes, severe floods, or volcanic eruptions—based on historical records, current conditions, and potential future changes in climate or land use. Emergency personnel, urban planners, and policymakers can use these simulations as training exercises, refining their contingency plans, communication strategies, and resource management. This preparation ensures that when a real warning is issued, responders have rehearsed similar situations and can act with confidence and speed. These simulations contribute to a culture of readiness, reducing the impact of actual disasters.
20. Continuous Model Improvement
Advanced machine learning approaches (like reinforcement learning) update predictive models continuously with new data, ensuring that early warning systems become more accurate and reliable over time.
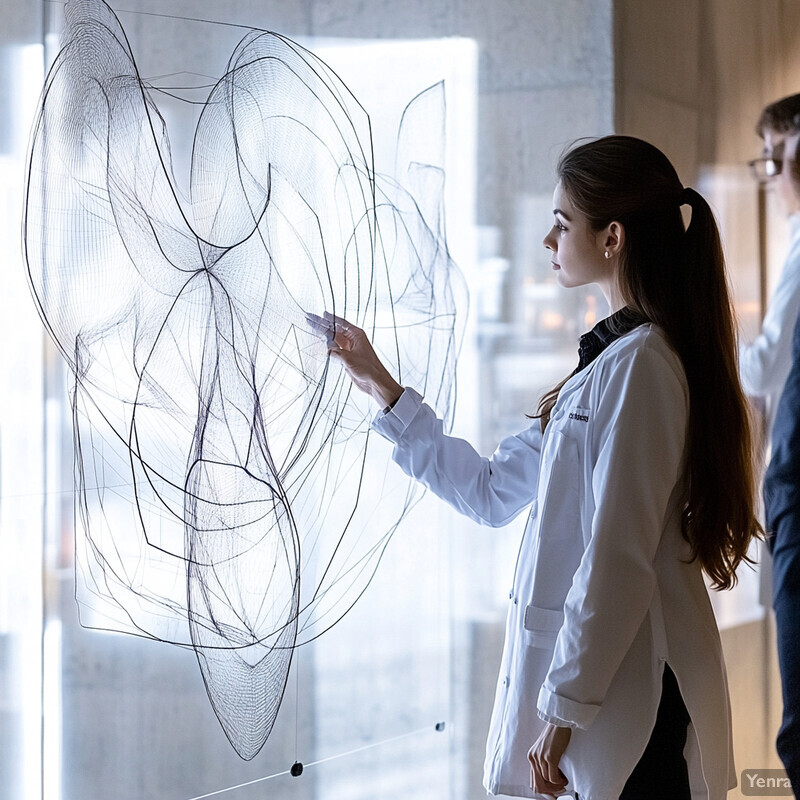
Disaster forecasting models cannot remain static; they must evolve as new data becomes available. AI-driven approaches, including reinforcement learning and continuous retraining, ensure that predictive models improve over time. As more events occur—whether false alarms, near-misses, or direct hits—the system refines its algorithms, reduces biases, and enhances accuracy. This iterative learning process means that early warning systems grow more reliable and trustworthy, strengthening their value to communities and emergency response agencies. Over time, continuous improvement instills greater resilience in societies facing an uncertain and changing climate.