1. Predictive Crime Mapping
AI-driven analytics use historical incident data to forecast potential crime hotspots, enabling police to proactively patrol high-risk areas before crimes occur.
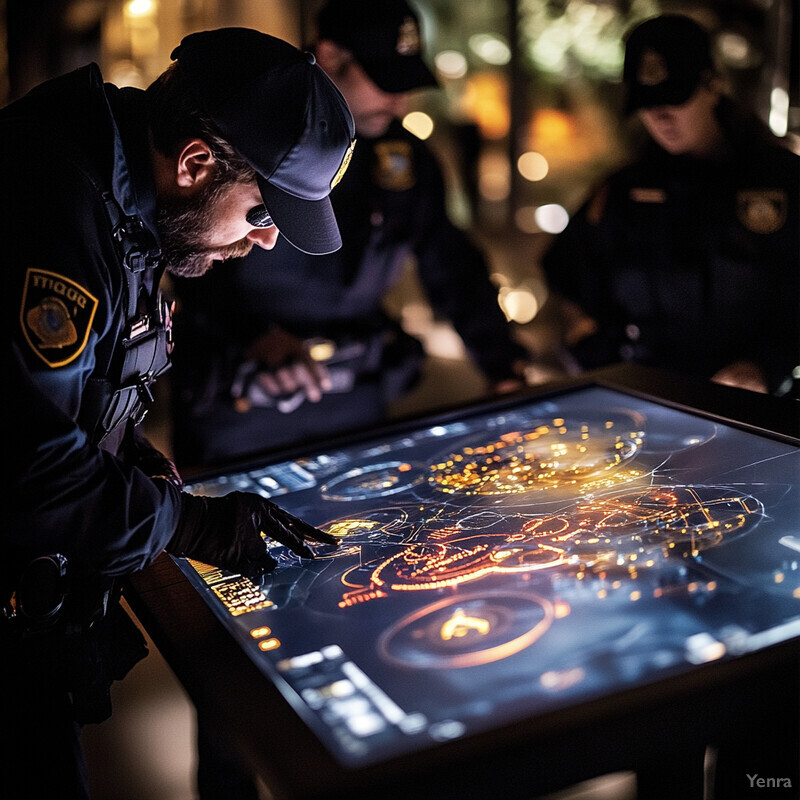
Predictive analytics employ historical crime data, demographic information, environmental factors, and temporal patterns to identify areas most likely to experience certain types of criminal activity. By analyzing these multidimensional datasets, advanced algorithms generate 'heat maps' that pinpoint evolving hotspots of crime. Police departments can then strategically allocate patrols, direct community outreach efforts, or install preventive measures—such as improved lighting or CCTV—in these high-risk areas. This approach not only increases the efficiency of officers’ time on the street but also helps reduce overall crime rates by disrupting potential incidents before they occur.
2. Resource Allocation Optimization
Machine learning tools help departments strategically deploy officers and resources based on data-driven risk assessments, ensuring more efficient use of limited manpower.
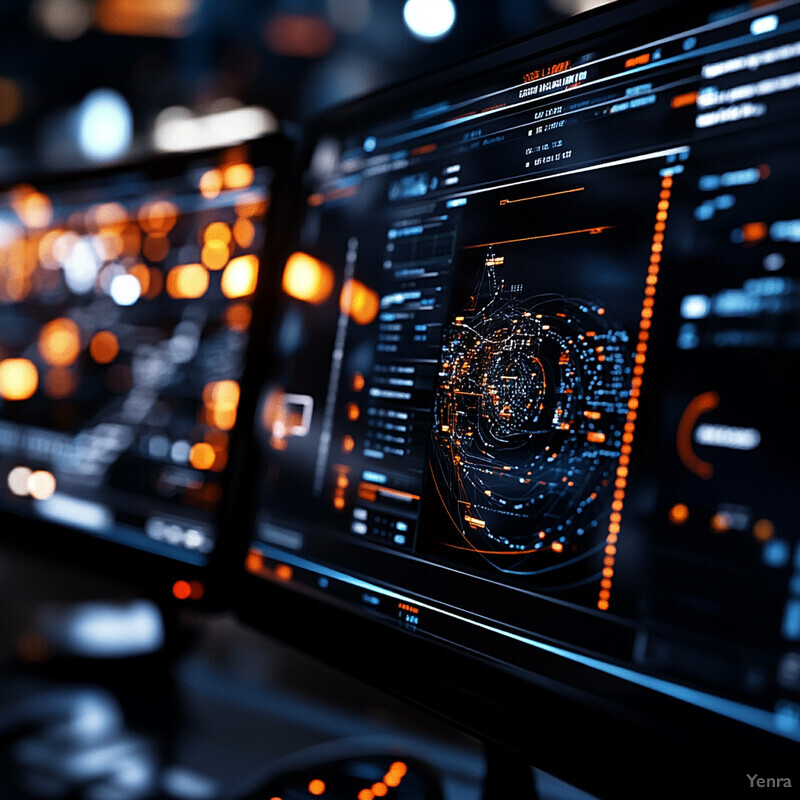
AI-driven tools use data integration and machine learning to determine how best to deploy limited law enforcement resources. By considering factors such as crime type, neighborhood-specific data, seasonal patterns, and officer availability, these systems help police departments find the optimal distribution of patrols, vehicles, and technological assets. Over time, agencies can identify which interventions yield the greatest results, ensuring that resources—from personnel to equipment—are used in a cost-effective and impactful manner. This data-informed approach bolsters the department’s ability to serve the community more comprehensively and transparently.
3. Early Intervention Systems for Officers
AI can analyze officer behavior, use-of-force incidents, and complaint patterns to identify early warning signs and guide interventions or additional training, improving community trust.
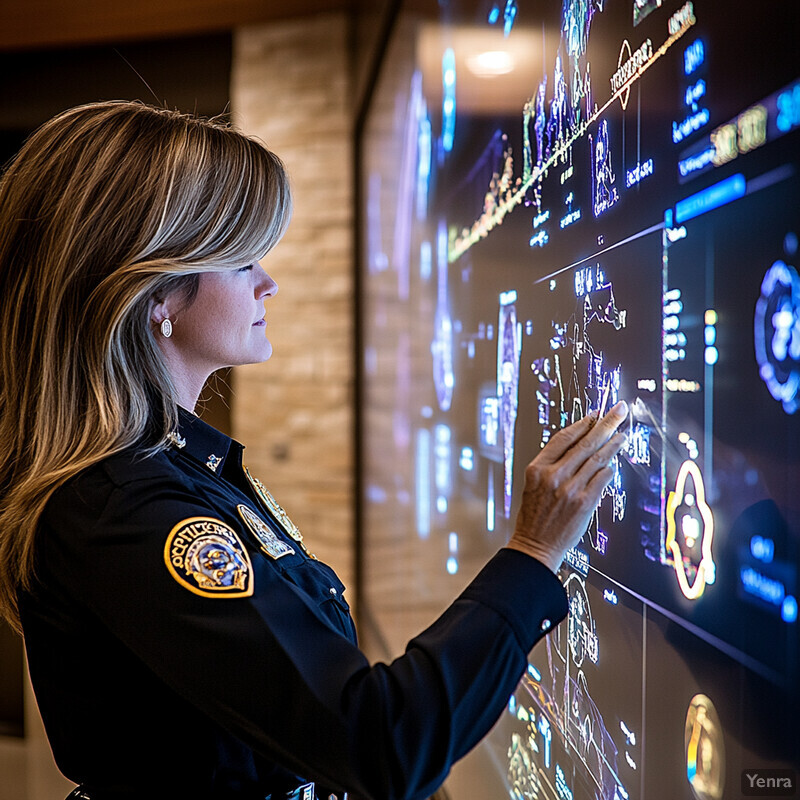
Law enforcement agencies increasingly rely on AI-based early intervention systems to monitor officer performance and behavior. These systems scrutinize a range of indicators—complaints from community members, frequency and context of use-of-force incidents, response times, and disciplinary records—to detect subtle warning signs of stress, bias, or misconduct. When concerning patterns emerge, supervisors can take proactive steps, such as offering additional training, counseling, or mentorship. By correcting issues before they escalate, departments can improve officer accountability, community trust, and overall professionalism within the force.
4. Intelligent Video Analytics
Advanced computer vision algorithms enable real-time screening of surveillance footage to detect suspicious activities—such as loitering, trespassing, or abandoned packages—and alert officers promptly.
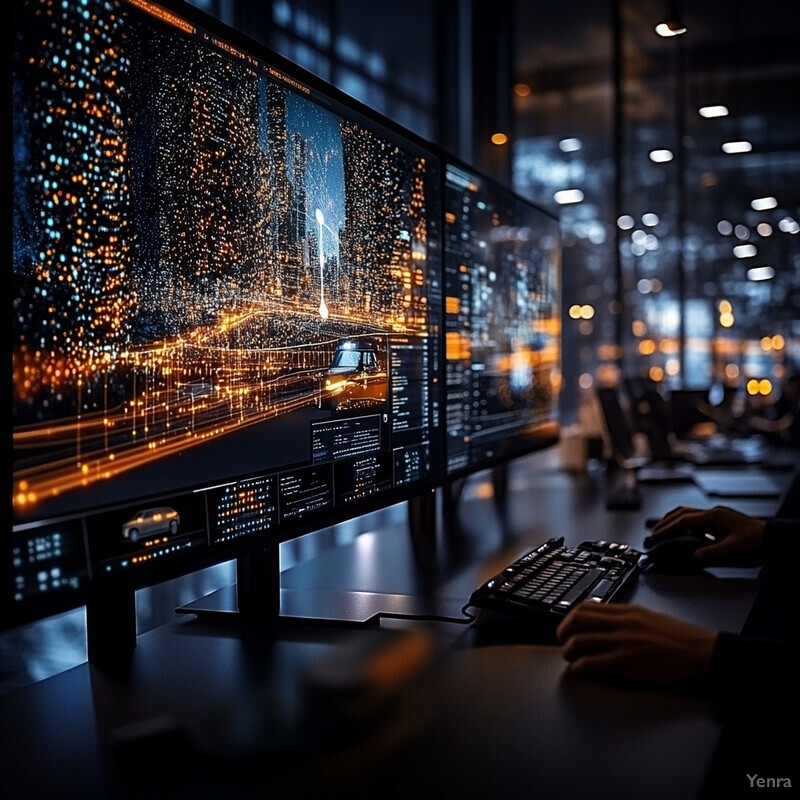
Modern policing now benefits from AI-enhanced video surveillance systems capable of recognizing patterns and alerting human operators to suspicious activities. Computer vision algorithms can detect unusual behaviors—loitering near restricted areas, abrupt crowd dispersals, or unauthorized access attempts—and immediately flag these instances for review. Such intelligent filtering greatly reduces the time analysts spend combing through footage. As a result, officers can respond more quickly to potential threats, prevent crimes in progress, and maintain a heightened sense of situational awareness that enhances community safety.
5. Automated License Plate Recognition (ALPR)
AI-powered camera systems scan vehicle plates to quickly identify stolen cars, wanted suspects, and vehicles linked to ongoing investigations, enhancing patrol efficiency.
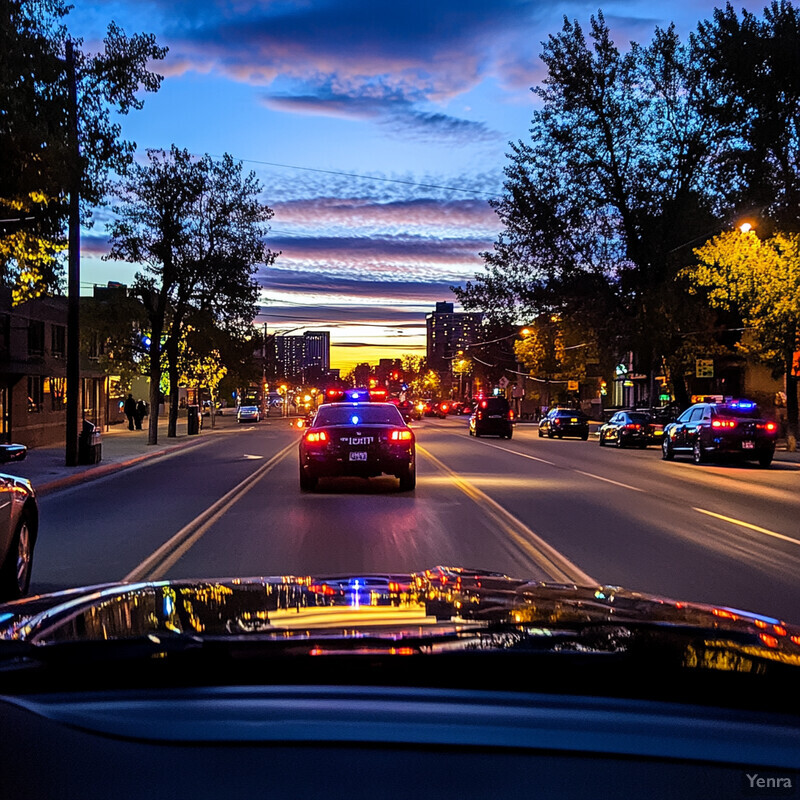
ALPR systems deploy AI to quickly and accurately identify vehicle license plates, enabling officers to spot stolen cars, track suspects’ movements, and verify driver identities in near real-time. By automating what was once a labor-intensive process, this technology helps agencies swiftly confirm whether a vehicle is associated with known offenders or outstanding warrants. The immediate data feedback streamlines investigations, reduces human error, and improves officers’ ability to apprehend suspects. This not only makes traffic stops safer and more efficient but also adds another layer of deterrence for would-be criminals on the move.
6. Facial Recognition to Find Missing Persons
Responsible and regulated facial recognition can assist in identifying missing persons, victims of human trafficking, or abducted children, improving community safety outcomes.
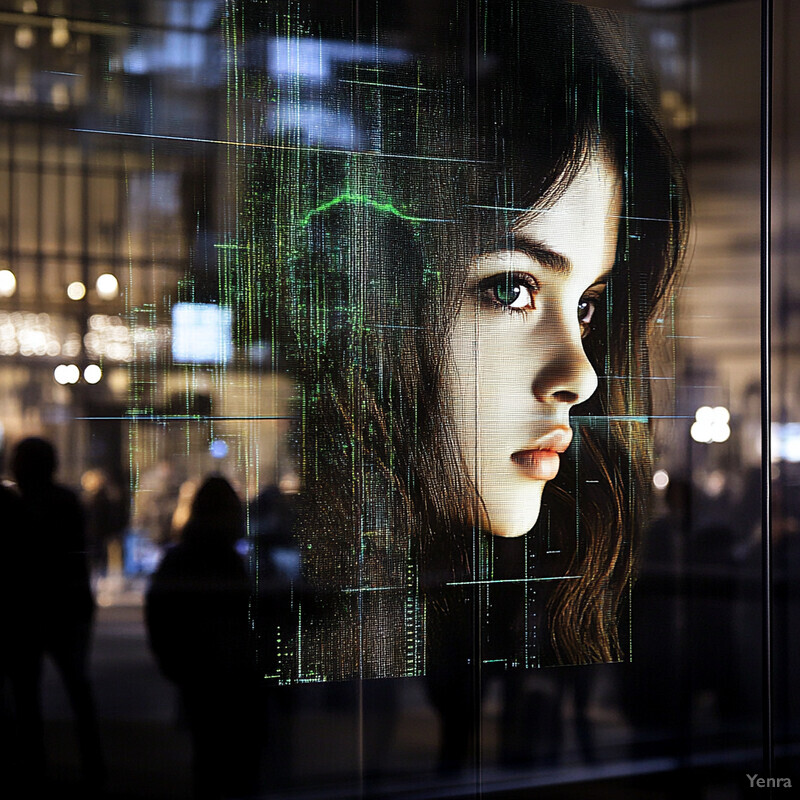
When used responsibly and with proper oversight, facial recognition technology can dramatically speed up the identification of missing persons, kidnapping victims, or at-risk individuals. By comparing real-time images captured at transportation hubs, public events, or crowded streets against databases of missing persons, authorities can locate individuals who need help more quickly. This tool assists not only in closing cases more efficiently but also in bringing peace of mind to families and communities. Its success lies in maintaining a balanced approach that respects privacy rights while leveraging technology for public good.
7. Gunshot Detection and Localization
AI-powered acoustic sensors can instantly identify gunshots, differentiate them from fireworks or other noises, and pinpoint their location, allowing for rapid response to violent incidents.
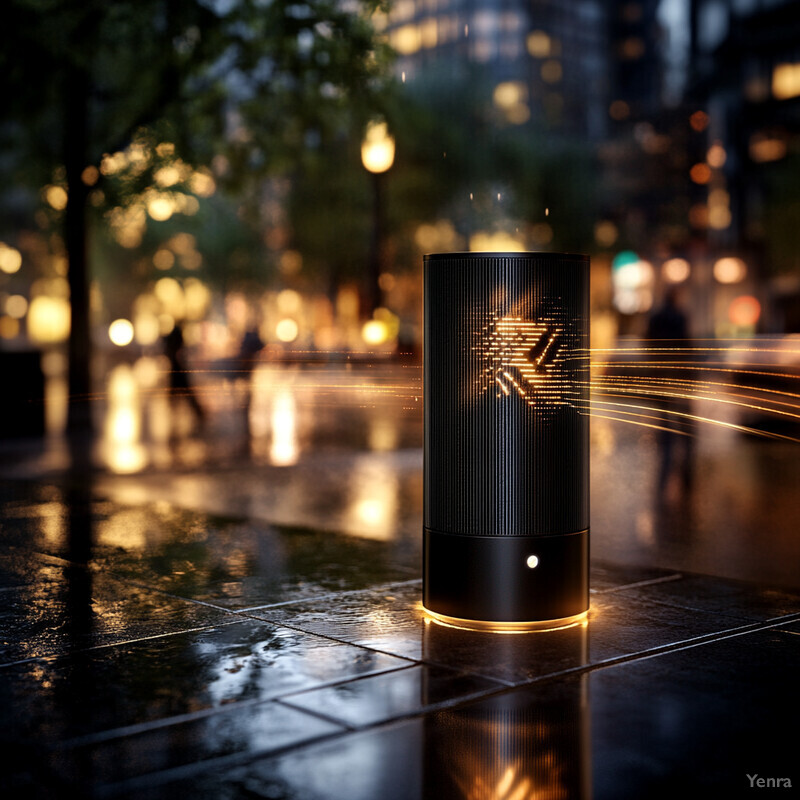
Acoustic sensors equipped with AI-based sound classification algorithms can discern the unique audio signature of gunfire amidst urban noise. Once detected, these systems triangulate the source of the gunshot and relay that information directly to dispatch centers and patrol units. The speed and accuracy with which responders are alerted often save valuable minutes, allowing them to reach a scene before critical evidence is lost. Prompt intervention can prevent further violence, increase the chances of apprehending perpetrators, and reassure neighborhoods that law enforcement is attentive to their safety.
8. Social Media Monitoring for Threat Detection
AI-driven natural language processing (NLP) can scan social media posts and public online forums for indicators of gang recruitment, violent extremism, or planned criminal activities, allowing proactive interventions.
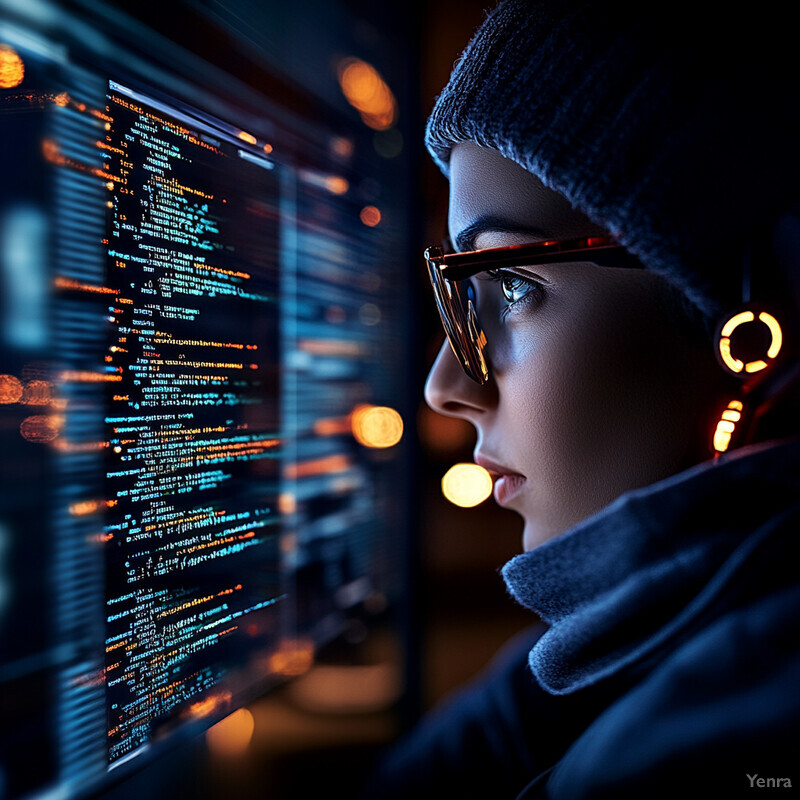
With so much public conversation occurring online, AI-driven linguistic and sentiment analysis can identify early indicators of potential criminal plots, hate crimes, gang activity, and violent extremism. By scanning public social media posts, blogs, and online forums, these systems highlight suspicious patterns—such as increased mentions of certain locations, coded language, or explicit threats. Law enforcement can then act preemptively, investigating leads or working with community groups to diffuse tensions. This proactive stance aims to address dangerous situations before they manifest into real-world harm, balancing privacy concerns with public safety.
9. Sentiment Analysis of Community Feedback
By analyzing comments, surveys, and social media posts, AI can gauge community sentiment toward law enforcement, helping agencies address concerns, build trust, and enhance public relations strategies.
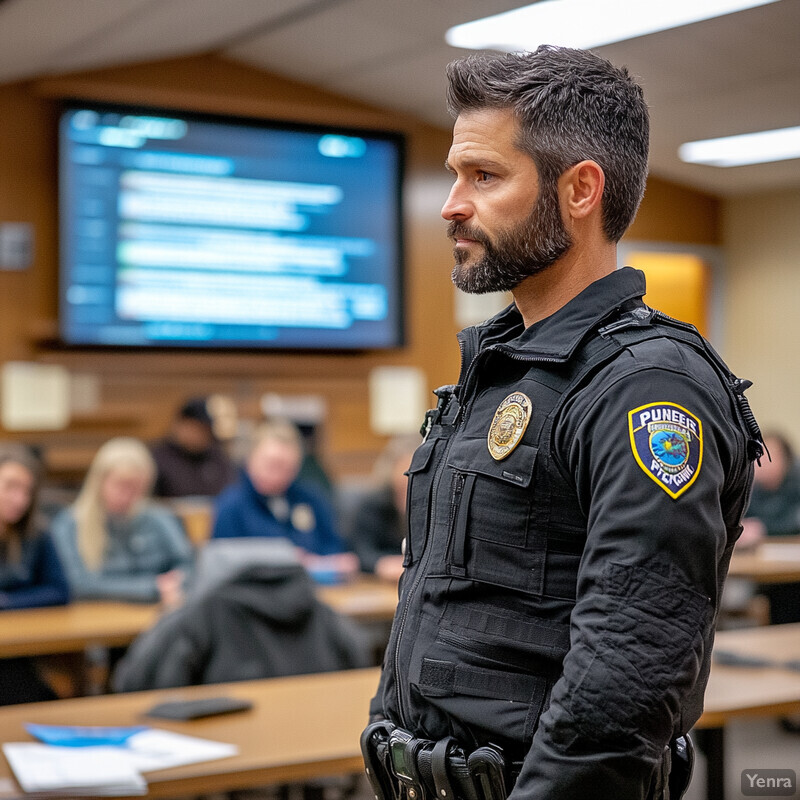
Beyond crime statistics, AI can gauge community trust and satisfaction by analyzing communication channels—surveys, social media comments, email feedback, or neighborhood forum discussions. Sentiment analysis tools help leaders understand public perception of policing methods, identify areas where the community feels underserved, and highlight potential sources of friction. With these insights, departments can refine training, community outreach programs, and policy reforms, ensuring that policing strategies evolve in response to citizens’ legitimate concerns. In turn, this feedback loop strengthens relationships between law enforcement and the public they serve.
10. Predictive Models for Repeat Offenders
Machine learning can identify individuals at higher risk of reoffending, guiding community-based rehabilitation programs, probation strategies, and tailored supervision efforts.
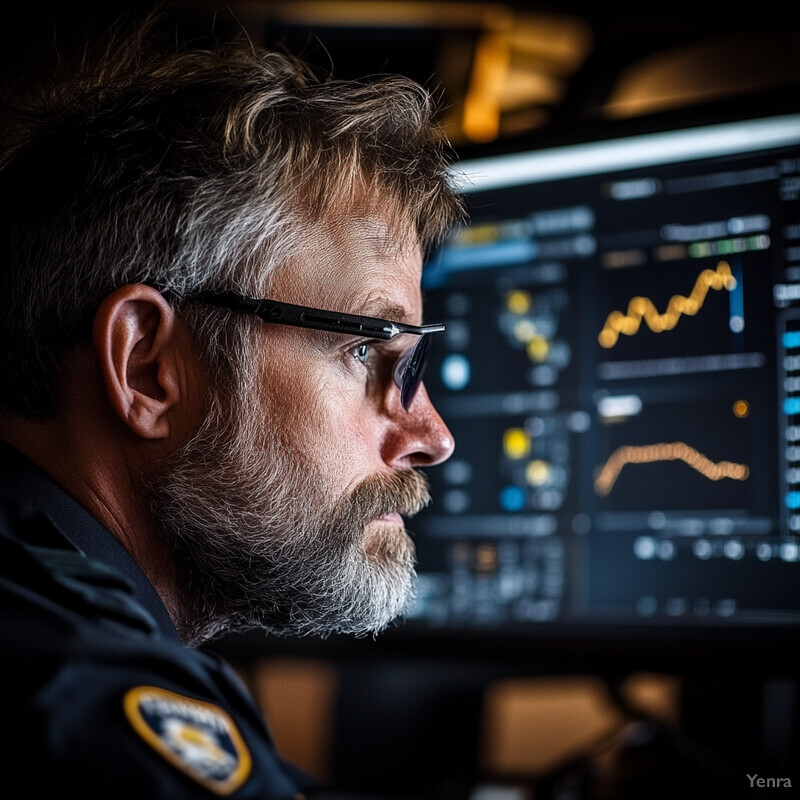
Machine learning models analyze arrest records, behavioral profiles, environmental factors, and other data points to predict the likelihood of reoffending. Such insights enable law enforcement and allied agencies—like social services and probation departments—to identify at-risk individuals and tailor interventions accordingly. Perhaps this means increased supervision, rehabilitative counseling, job placement assistance, or targeted education programs. By addressing root causes and tailoring support, the community can help break cycles of crime and reduce recidivism, ultimately contributing to a safer and more hopeful environment.
11. Real-Time Translation and Language Assistance
AI-driven translation tools help officers communicate effectively with non-English speakers, reducing misunderstandings and building stronger relationships with diverse communities.
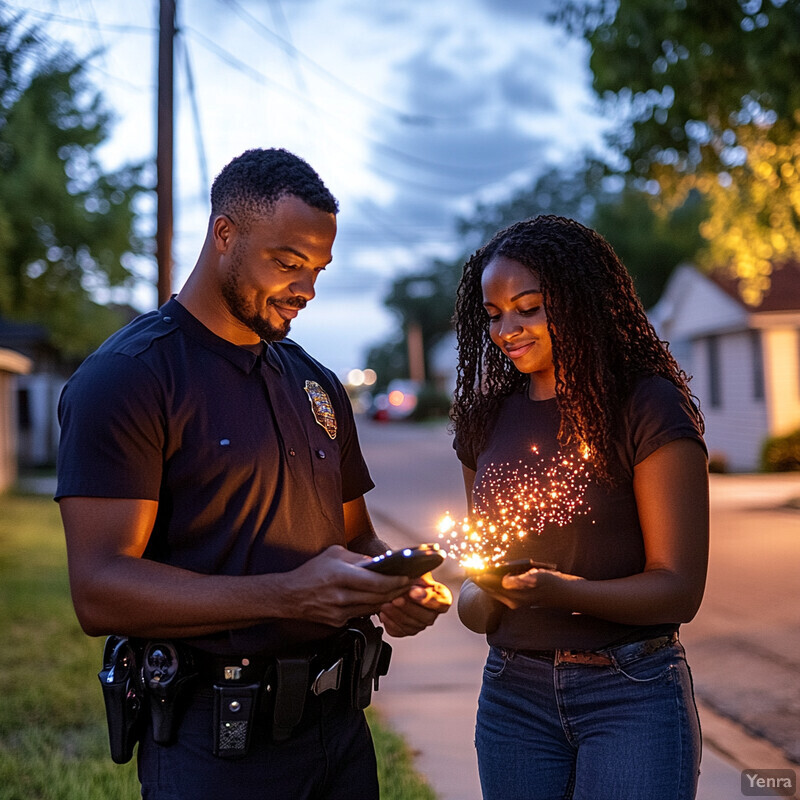
In multilingual communities, communication barriers can impede effective policing and community trust. AI-powered translation applications and speech recognition tools allow officers to communicate accurately and efficiently with residents who speak different languages. Officers can better understand citizens’ concerns, interview witnesses more effectively, and provide instructions without confusion. By facilitating clear dialogue, this technology helps build trust, encourages cooperation in investigations, and ensures that all community members feel equally heard and protected.
12. Forensic Pattern Recognition
AI-assisted forensic software can detect patterns in crime scene evidence, linking seemingly unrelated cases to serial offenders more quickly and accurately than manual review.
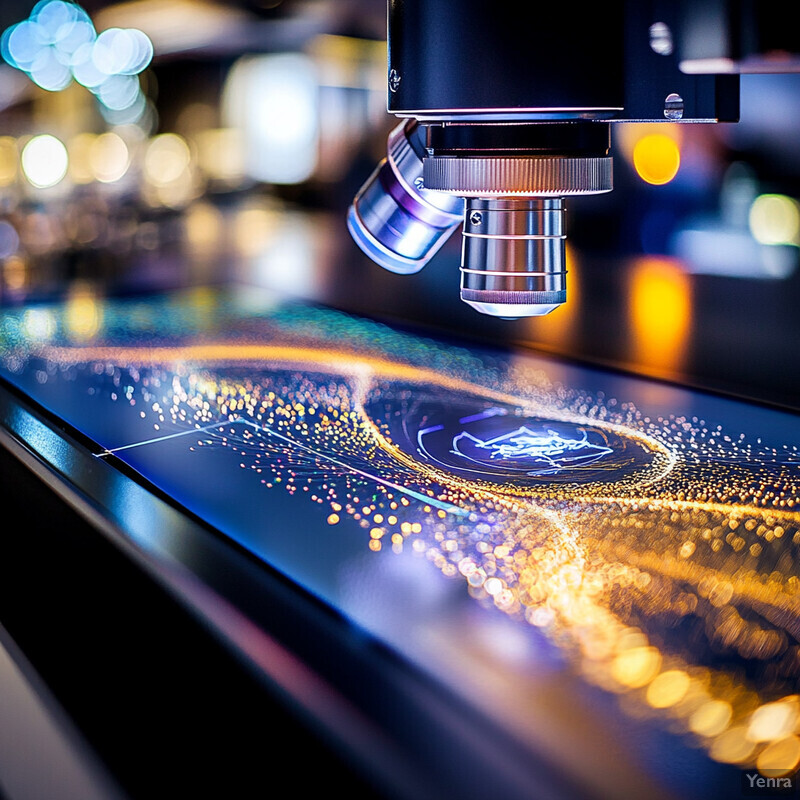
Advanced AI algorithms can analyze forensic evidence—fingerprints, DNA samples, ballistic trajectories, shoe prints, or tool marks—with extraordinary speed and precision. Linking such evidence across multiple crime scenes, the system can detect patterns that might indicate serial offenders or related cases. By identifying these connections sooner, investigators can focus their efforts more effectively, prevent further criminal acts, and bring suspects to justice more quickly. In doing so, these technologies contribute to a more efficient, scientific, and impartial investigative process.
13. Fraud and Cybercrime Detection
Specialized AI tools analyze digital transactions, emails, and network traffic to identify suspicious patterns of fraud, identity theft, or cyberattacks that threaten community members.
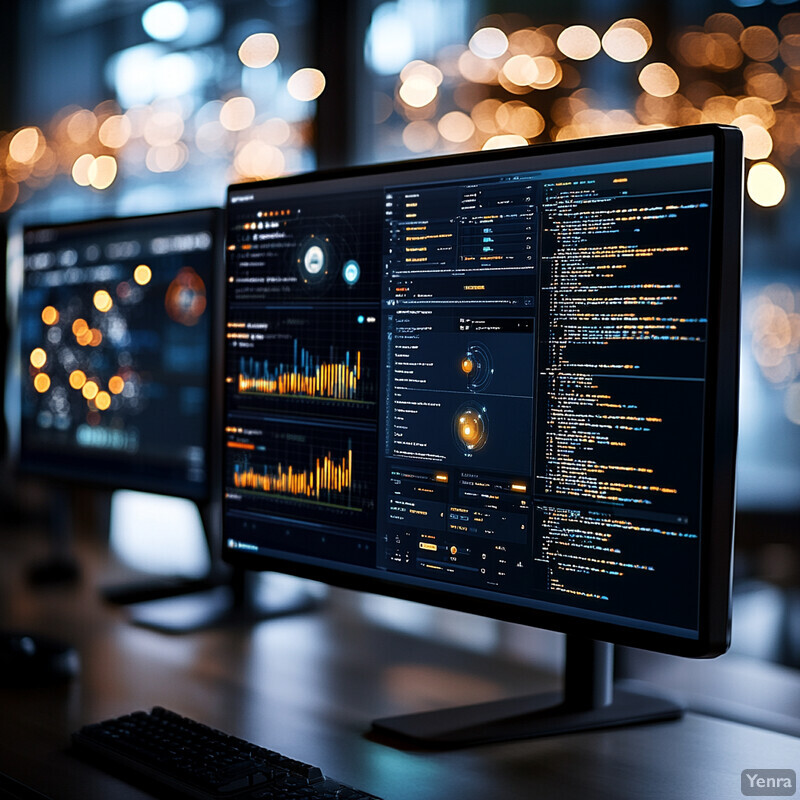
With the rise of digital transactions, AI has become crucial in identifying anomalous patterns that suggest fraud, identity theft, or ransomware attacks. Machine learning algorithms continuously learn from historical data to detect suspicious behavior—unusual spending patterns, account breaches, or phishing schemes—and alert authorities in real time. This proactive approach helps safeguard individuals’ financial security and personal information, thus maintaining community confidence in their local institutions. By catching cybercriminals early, law enforcement prevents economic harm and encourages a safer digital environment for everyone.
14. Anonymous Tip Analysis
AI can process and prioritize community-submitted tips—whether text-based, voice messages, or online forms—to highlight credible leads and ensure faster follow-up by investigators.
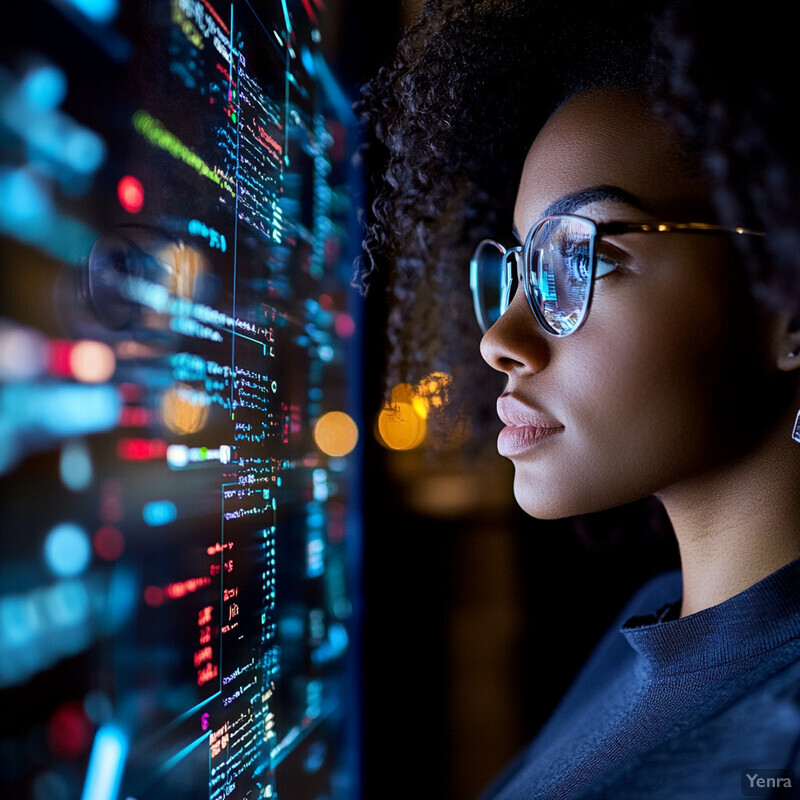
Community policing thrives on trust, and anonymous tip lines have long played a role in gathering valuable information. With AI, these tips—whether voice messages, text submissions, or online forms—are rapidly analyzed and prioritized. Natural language processing helps classify the urgency, credibility, and relevance of each lead, ensuring that investigators act quickly on the most actionable intelligence. This streamlined approach improves case resolution rates, fosters community participation, and reassures the public that their input is both valued and impactful.
15. Predictive Analytics for At-Risk Youth
Collaborative efforts with social services enable AI to identify youth at risk of gang involvement or criminal behavior, prompting early community interventions, mentorship, and support programs.
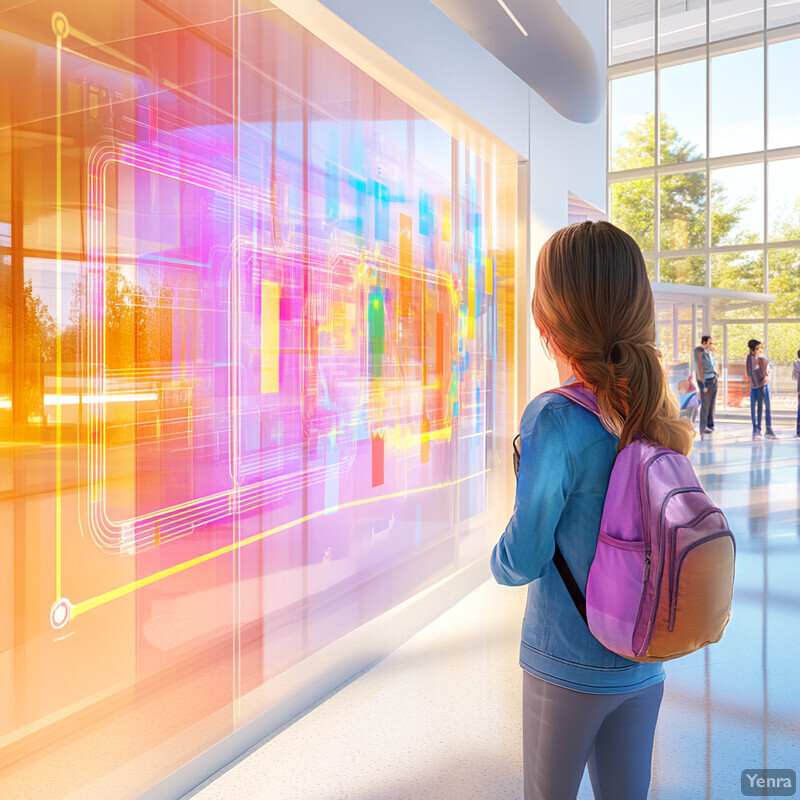
Some AI tools assist in the early identification of young individuals who may be vulnerable to gang recruitment, substance abuse, or other pathways into criminal activity. By drawing on data from schools, social services, and community organizations, these predictive models help guide preventative support, mentoring programs, and afterschool initiatives. The aim is to intervene before these youths become involved in crime, breaking negative cycles and redirecting them toward positive opportunities. This holistic approach emphasizes compassion, long-term safety, and the well-being of future generations.
16. Public Safety Chatbots and Hotlines
AI chatbots can assist residents by providing immediate information on local resources, how to report crimes, and guidance on safety precautions, improving community outreach.
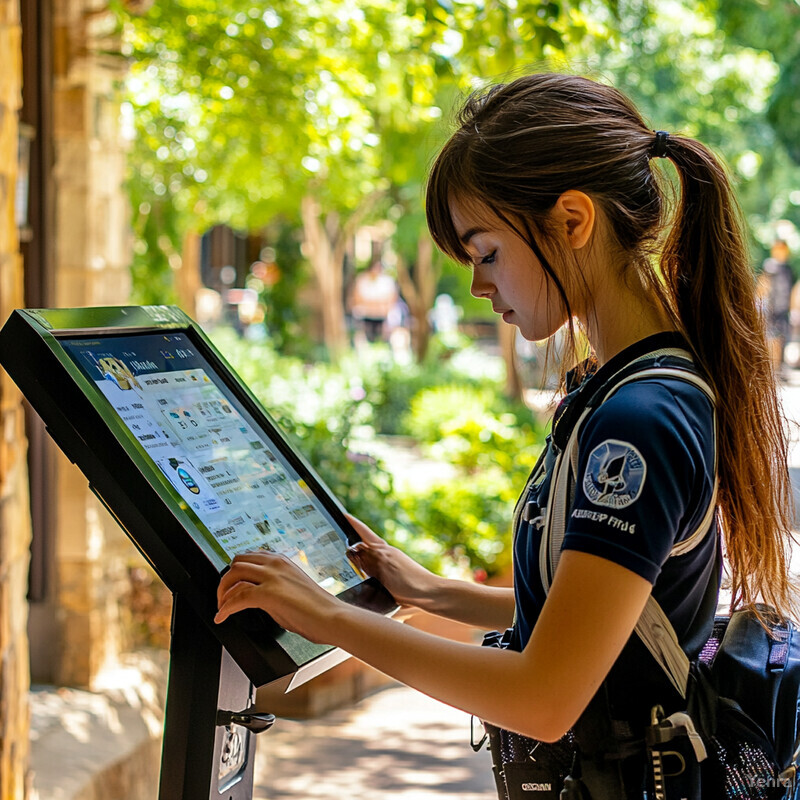
AI-powered chatbots and virtual hotlines provide residents with immediate, round-the-clock access to information, guidance, and resources. Whether they need to report a crime, find victim assistance programs, learn about neighborhood watch groups, or understand legal rights, these digital assistants offer prompt and accurate responses. By easing the workload on dispatchers and providing self-service options, community members feel more supported. In turn, this accessibility encourages proactive engagement, fosters transparency, and ensures help is always at residents’ fingertips.
17. Intelligence-Led Policing Support
Machine learning integrates crime data, offender profiles, and community intelligence to help detectives and analysts identify criminal networks, enabling more targeted, less intrusive policing methods.
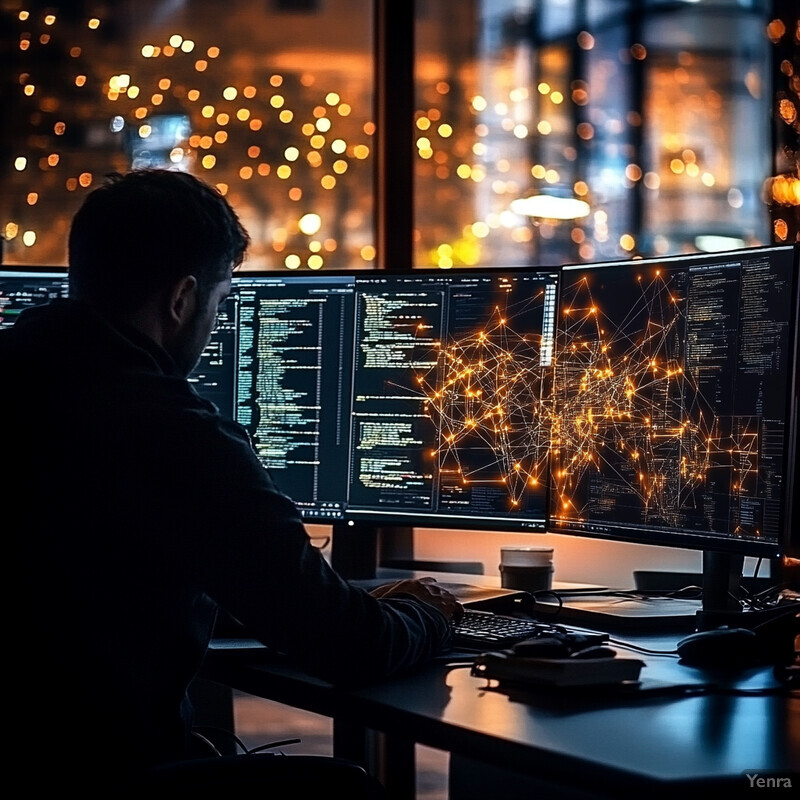
Machine learning consolidates crime data, case files, and intelligence reports into cohesive, searchable insights that inform strategic decision-making. Analysts can identify criminal networks, understand evolving tactics, and predict where resources should be concentrated. By coupling data analysis with human expertise, intelligence-led policing zeroes in on the core drivers of crime, taking measured, evidence-based actions rather than broad, reactive measures. This refined focus can reduce unnecessary stop-and-frisks, build public confidence, and ultimately foster a more effective partnership between law enforcement and communities.
18. Event and Crowd Management
AI can analyze data from CCTV, drones, and social media to help authorities maintain safety during large gatherings, protests, or festivals, minimizing risk and building public confidence in law enforcement.
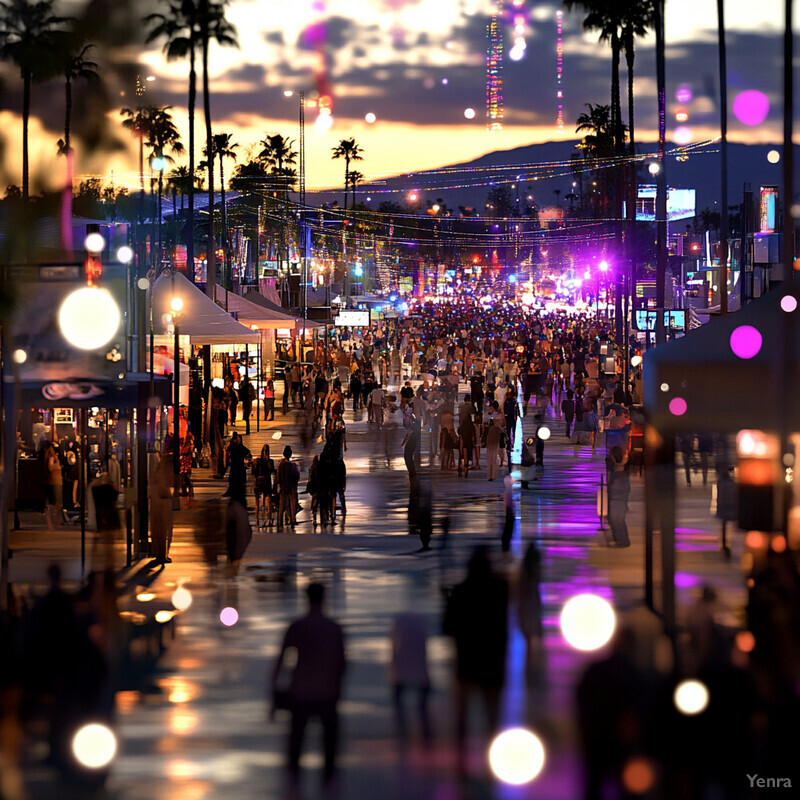
At large gatherings—be they concerts, sporting events, protests, or cultural festivals—AI-enhanced surveillance and drone imagery help officers monitor crowd density, movement flows, and unusual activity. With real-time analysis, law enforcement can detect potential safety hazards—stampedes, sudden disruptions, or tensions between groups—and respond decisively. Strategic interventions maintain the balance between public freedom and security, reassuring attendees that their well-being is a priority. This intelligent management of public spaces strengthens community trust and supports the right of people to gather safely.
19. Crime Linkage Analysis
Advanced algorithms can find hidden correlations between cases—like distinctive modus operandi or weapon types—enabling detectives to close cases more efficiently and prevent future offenses.
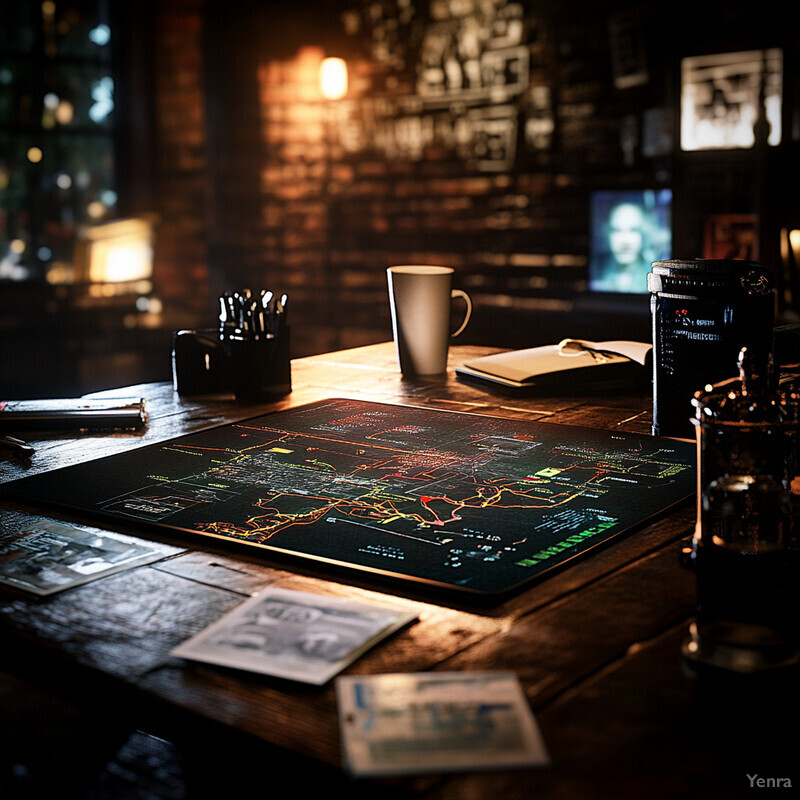
AI tools excel at sifting through massive archives of criminal data to find hidden connections among crimes that may span neighborhoods, jurisdictions, or even countries. By identifying patterns in suspects’ methods, weapon usage, or target selection, these systems assist detectives in linking unsolved cases to known offenders. Accelerated case closures not only deliver justice to victims and their families but also deter criminals who see that technology significantly reduces their chances of evading detection. Thus, such linkage analysis upholds the rule of law and enhances overall community confidence in the justice system.
20. Continuous Training and Simulation
AI-driven virtual reality training scenarios allow officers to practice de-escalation tactics, cultural sensitivity, and community engagement skills in realistic simulations, ultimately fostering a more community-oriented policing approach.
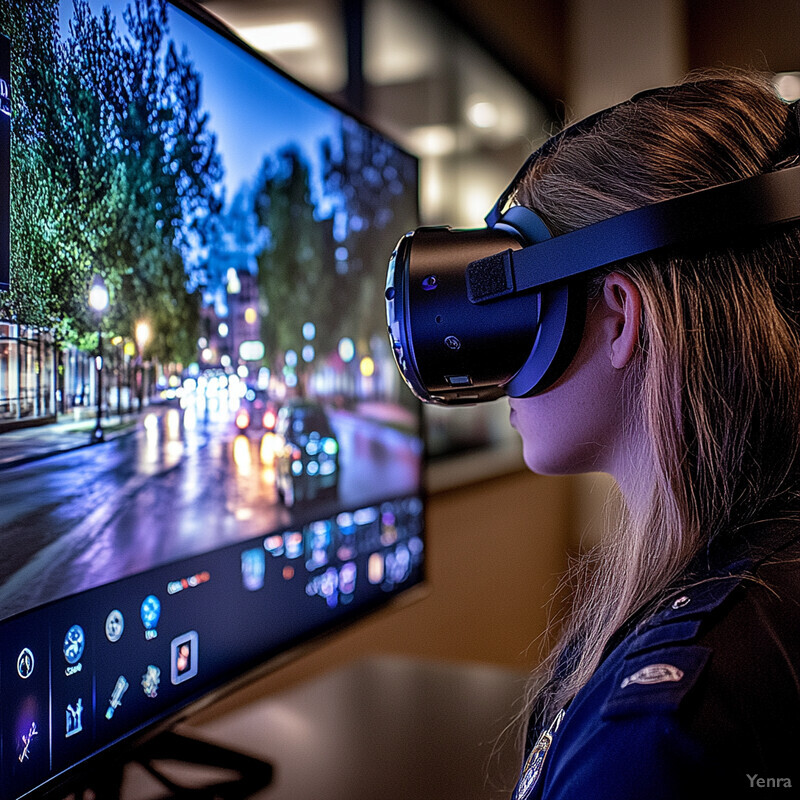
AI-driven virtual reality (VR) and simulation platforms offer law enforcement officers realistic, evolving training scenarios. They can practice de-escalation techniques, cultural competency, effective communication, and community outreach methods in diverse, lifelike situations. By learning to handle complex challenges—from language barriers to mental health crises—officers become more adept and empathetic in the field. This immersive training ultimately results in more professional, community-oriented policing, leading to stronger relationships, reduced conflicts, and better outcomes for all community members.