1. Adaptive Skill Modeling
Advanced AI algorithms can more accurately infer a student’s evolving knowledge state, allowing cognitive tutors to tailor exercises that match the learner’s current skill mastery.
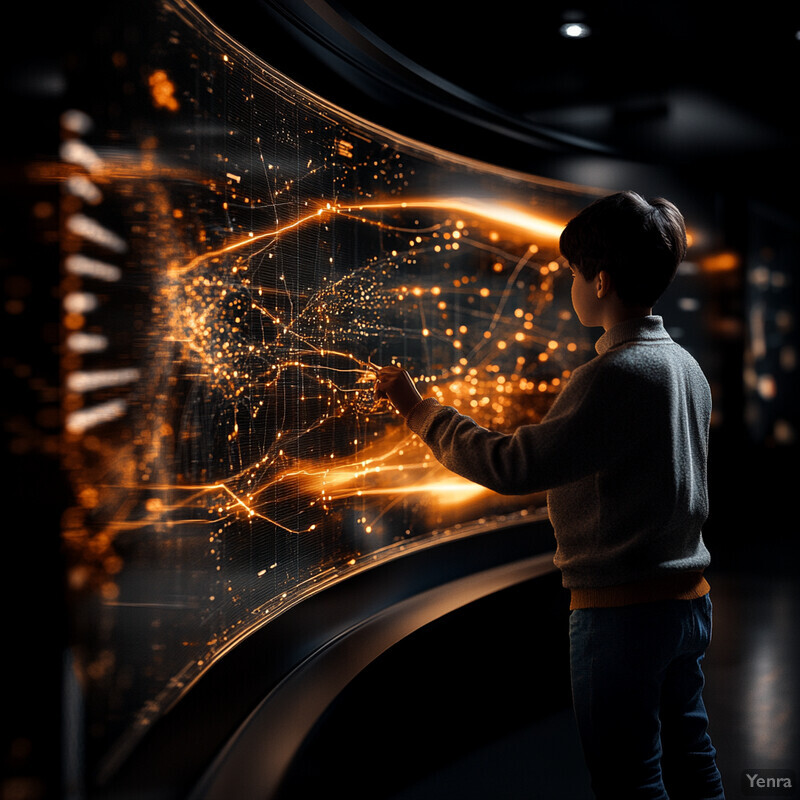
Advanced AI algorithms enable cognitive tutors to maintain highly granular models of a student’s knowledge state, capturing not only which concepts have been mastered but also the degree of fluency, error patterns, and areas of uncertainty. These models update in real-time as the learner progresses through tasks, integrating performance data, response times, and even patterns of misunderstandings. By doing this, the tutor can more accurately target instruction and practice opportunities. The result is a learning experience that dynamically adjusts difficulty and support to each student’s ever-changing skill level, promoting greater efficiency and depth in skill acquisition.
2. Personalized Learning Paths
By analyzing each student’s unique progress, cognitive tutors can dynamically generate individualized lesson sequences, ensuring that no two learners receive a one-size-fits-all curriculum.
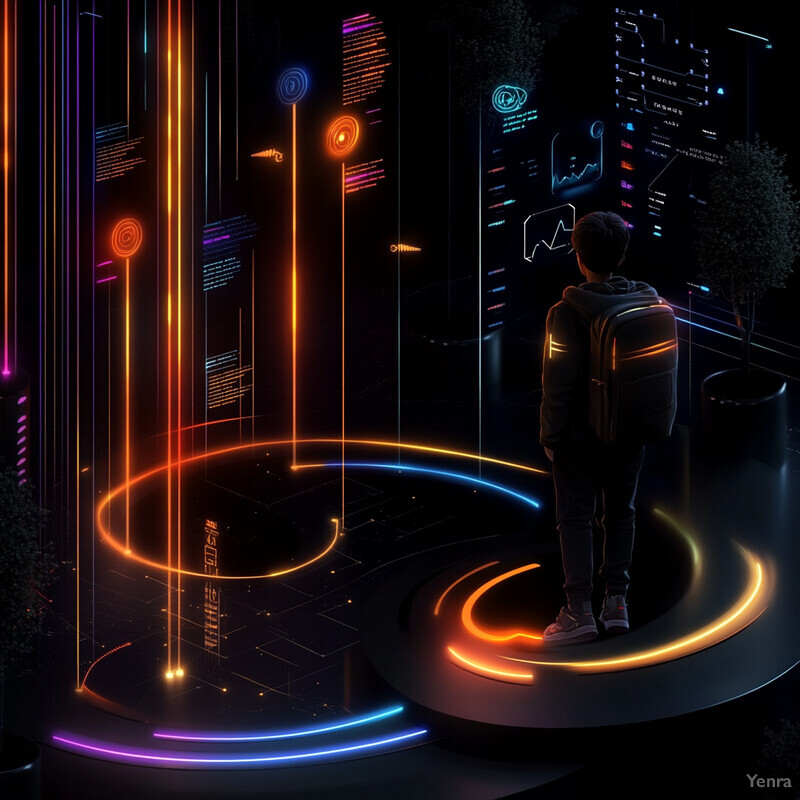
Traditional educational approaches often provide the same material to all learners, regardless of their individual needs. AI-enhanced cognitive tutors break this mold by crafting personalized sequences of lessons, exercises, and activities that align with a learner’s current understanding and pace of learning. These systems continuously monitor performance and adapt, offering remedial content to those who struggle while accelerating the path for more advanced students. By customizing the learning journey, students remain engaged, experience appropriately challenging material, and are less likely to feel bored or overwhelmed, ultimately fostering more meaningful and sustained learning outcomes.
3. Real-time Feedback Generation
Natural language processing and machine learning techniques enable tutors to provide immediate, context-sensitive hints, explanations, and suggestions in response to student inputs and errors.
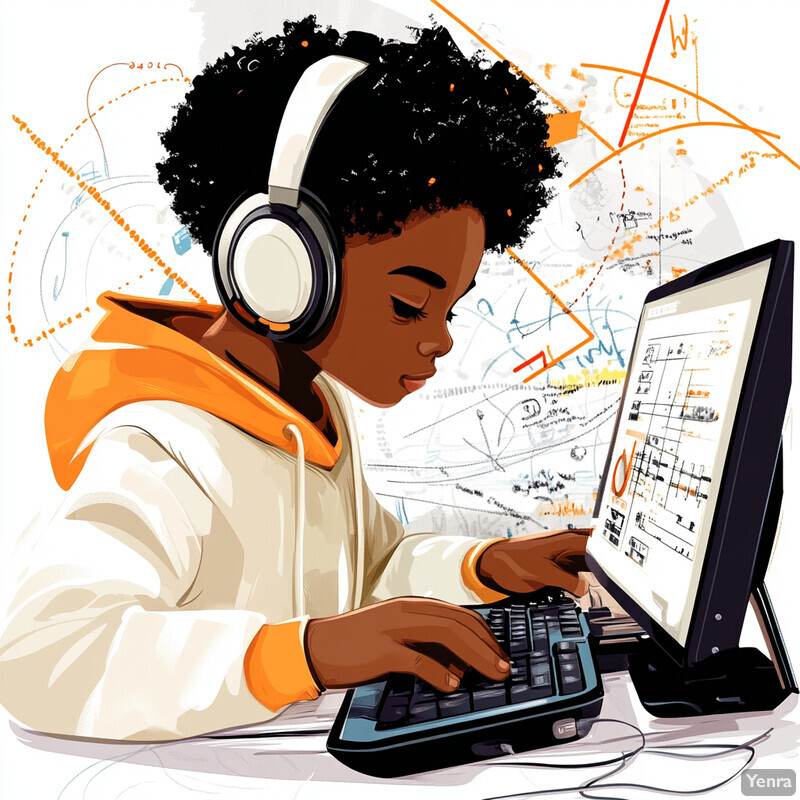
One of the most impactful features of AI-powered cognitive tutors is their ability to provide immediate, context-sensitive feedback. Rather than waiting for teacher grading or relying on static answer keys, AI uses NLP and advanced reasoning models to interpret a student’s input—be it a written explanation, a mathematical solution, or a coded program—and respond with targeted hints, clarifications, and corrective steps. This instantaneous support helps learners identify and rectify mistakes as they arise. Over time, such consistent, responsive guidance accelerates comprehension, builds confidence, and helps learners develop more refined problem-solving strategies.
4. Emotion and Affect Recognition
AI-driven sentiment analysis tools can gauge when students are frustrated, bored, or confused, prompting the tutor to adjust its teaching style, pace, or difficulty level accordingly.
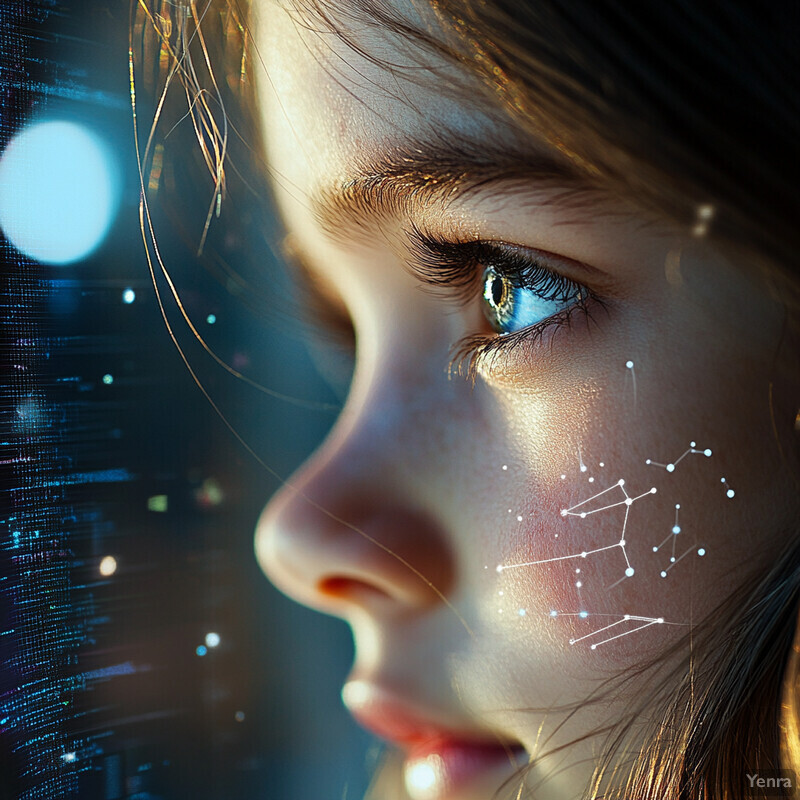
Beyond academic performance, AI-driven cognitive tutors increasingly consider the emotional and affective states of learners. Using techniques such as facial expression analysis, voice tone recognition, or physiological signals (when available), these systems can infer when a student is frustrated, anxious, bored, or particularly engaged. Recognizing these emotional cues allows the tutor to adjust instruction style, pacing, or complexity to maintain motivation. For instance, it might reduce difficulty to prevent discouragement or introduce more challenging problems if signs of boredom emerge. Addressing emotional factors helps create more supportive, empathetic, and effective learning experiences.
5. Predictive Analytics for Performance
Using historical data and predictive modeling, AI-powered tutors can anticipate where learners are likely to struggle, intervening with preemptive guidance before issues become entrenched.
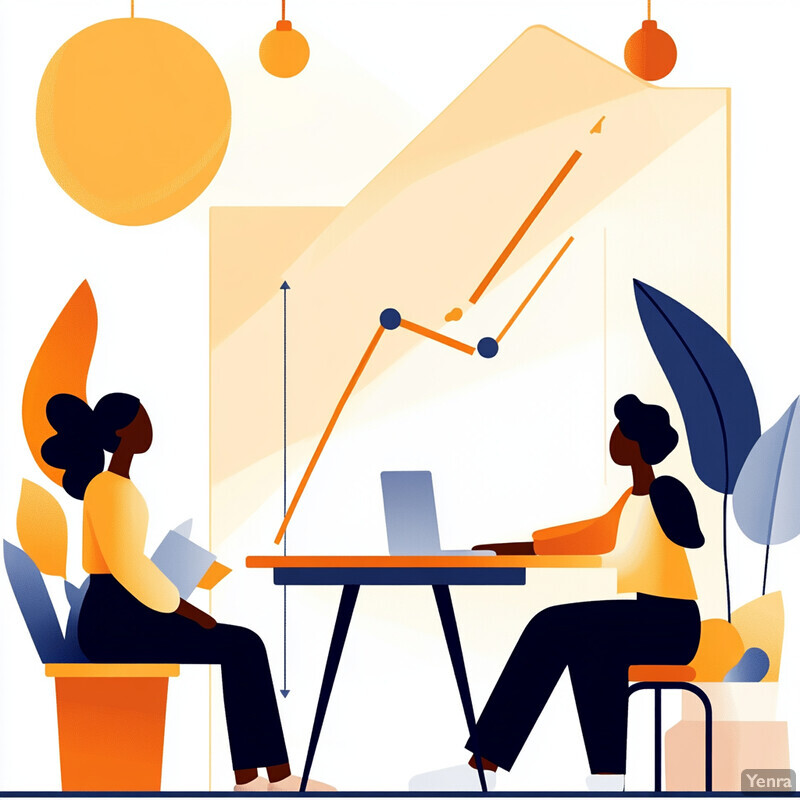
AI-powered tutors harness large volumes of student interaction data and apply machine learning models to predict future performance trends. By analyzing patterns of correct and incorrect responses, time on task, and the evolution of concept mastery, these tutors can forecast where learners are likely to struggle next. Armed with this insight, the system can intervene proactively, introducing targeted hints, supplementary materials, or alternative explanations before a knowledge gap solidifies. This data-driven anticipation of learning hurdles helps maintain steady progress, reduces anxiety associated with sudden difficulties, and encourages a smoother, more confident learning trajectory.
6. Continuous Formative Assessment
Advanced assessment engines can seamlessly evaluate a student’s comprehension at every step, ensuring instruction is always informed by the learner’s current understanding rather than relying solely on end-of-unit tests.
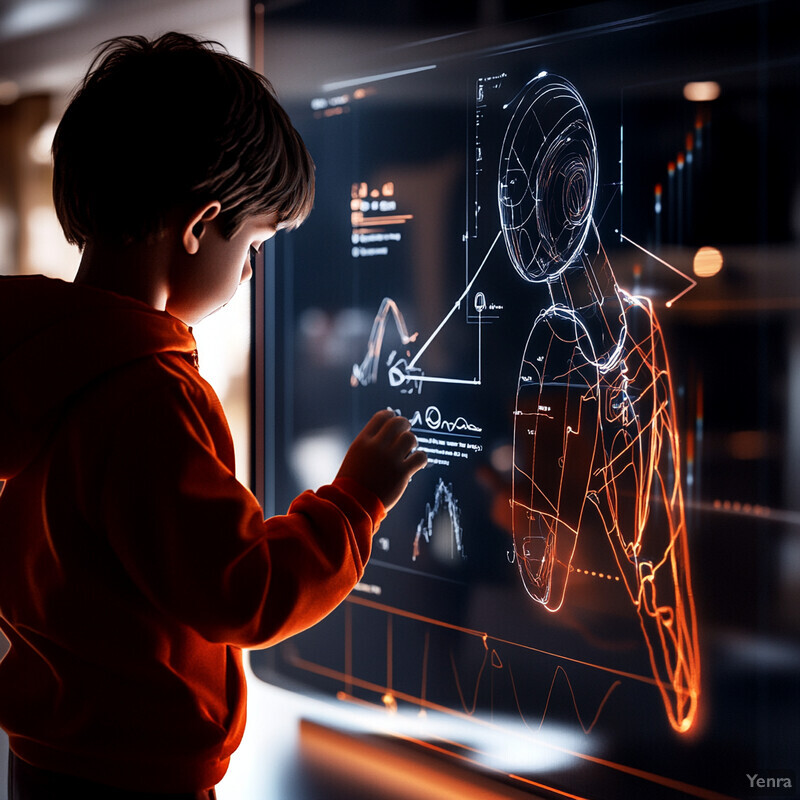
In contrast to traditional methods that rely heavily on periodic testing, AI-enhanced cognitive tutors integrate assessment into every moment of interaction. Each problem a student attempts and each explanation they provide contribute to a continuous measurement of understanding. As learners solve tasks, the tutor evaluates their reasoning and solution paths, updating its model of the student’s mastery. This ongoing formative assessment allows the tutor to adjust its instruction in the moment, ensuring that learning activities are always aligned with the student’s true skill level. The result is a more efficient, responsive, and learner-centered education process.
7. Intelligent Hint Policies
Reinforcement learning can optimize hint-timing and complexity, ensuring that help is neither given too early nor too late.
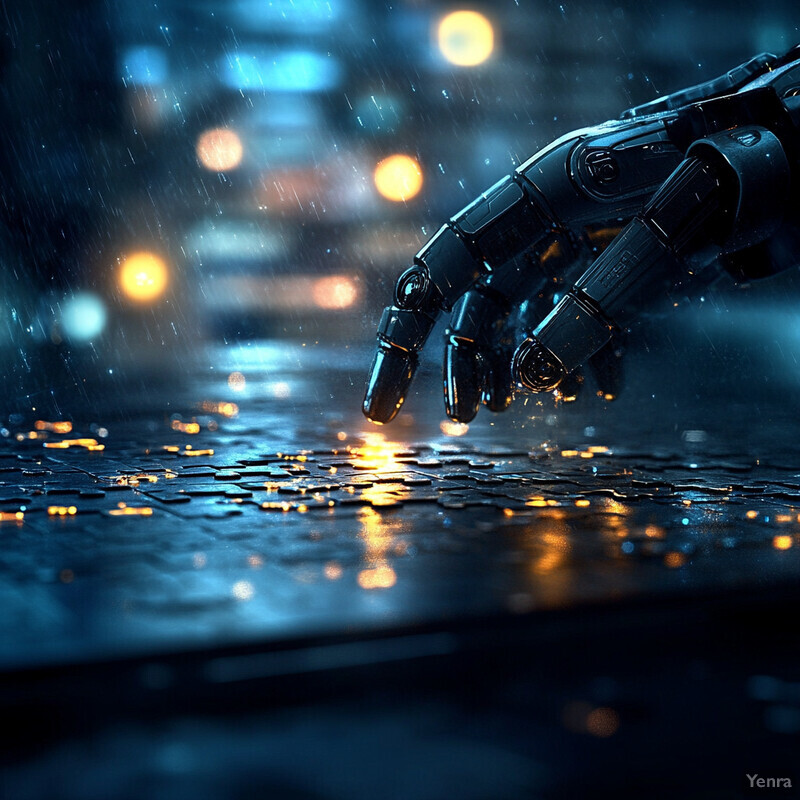
Determining when and how to provide help is an art that AI can refine. Using reinforcement learning and other optimization techniques, cognitive tutors can analyze data from many learners and identify the most effective hint-giving strategies. Instead of offering hints too early—which discourages persistence—or too late—which leads to frustration, the tutor adjusts its hint policies dynamically. It might give a gentle nudge after detecting multiple unsuccessful attempts or provide progressively more specific guidance. These fine-tuned interventions help students develop resilience, autonomy, and effective problem-solving habits, ultimately enhancing both motivation and learning outcomes.
8. Domain-general Reasoning
Rather than focusing on narrow tasks, modern cognitive tutors can leverage AI for broader domain reasoning, helping students transfer skills from one context or subject to another.
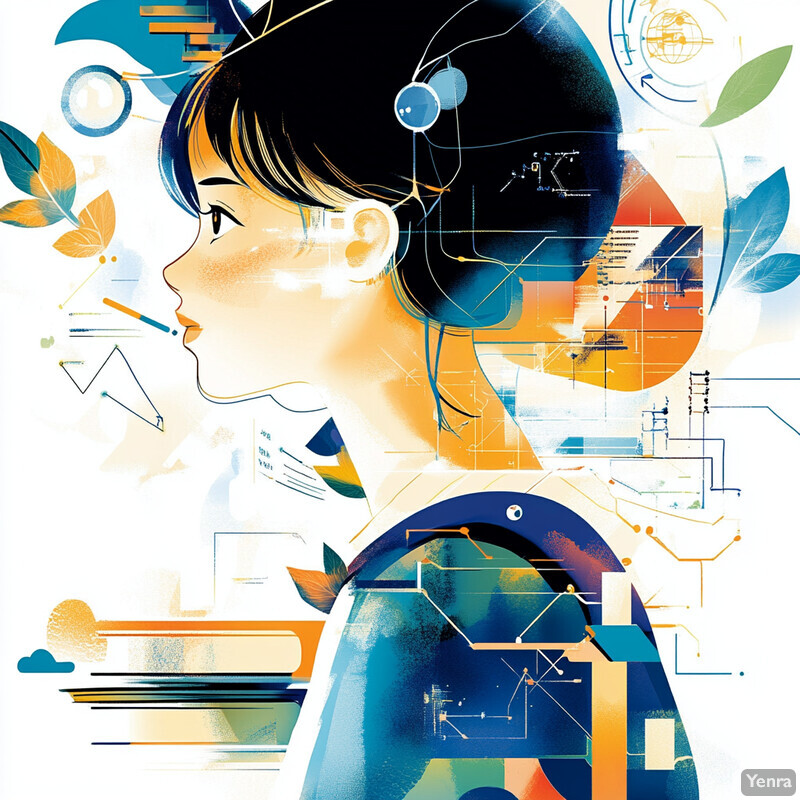
Early cognitive tutors often focused on narrow skill sets within a single domain—like algebraic manipulation. AI advancements now enable these systems to support domain-general reasoning skills that transfer across subjects. By integrating abstract logic, critical thinking, and metacognitive strategies into their instructional framework, these tutors can teach students how to learn and solve problems more broadly. This expands beyond rote memorization, helping learners understand underlying principles and apply their knowledge to novel contexts. Encouraging domain-general reasoning empowers students to become adaptable, independent thinkers capable of tackling complex, interdisciplinary challenges throughout their academic and professional lives.
9. Automated Content Generation
Deep learning models can generate new practice problems, scenario-based questions, and personalized examples, freeing educators from the burden of manual content creation.
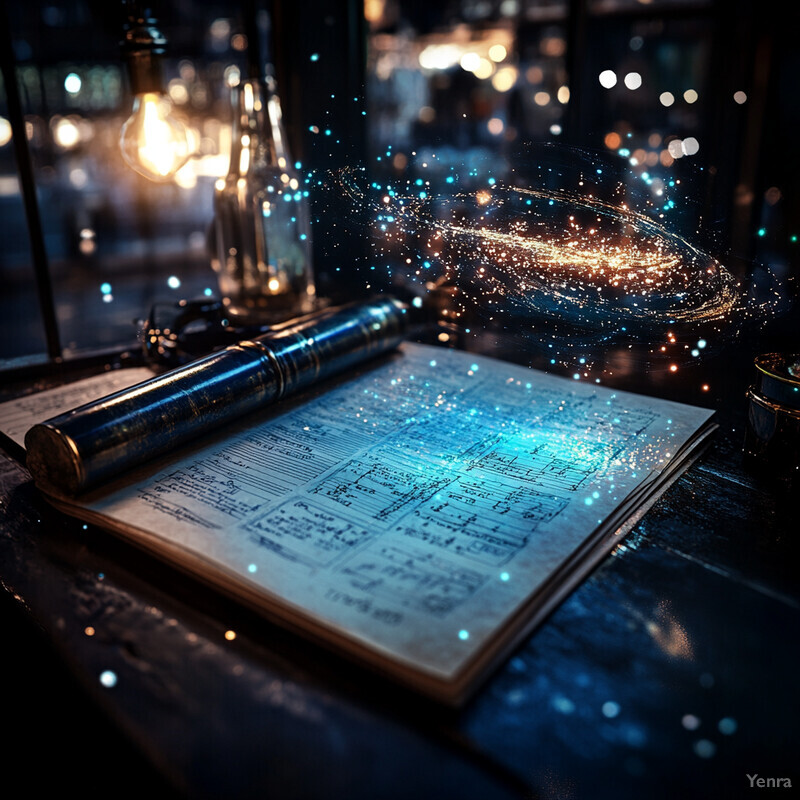
Creating new exercises, problems, and learning materials can be time-consuming for instructors. AI-driven cognitive tutors can automate much of this content generation, using deep learning models to produce high-quality, diverse problems tailored to various difficulty levels and instructional goals. By analyzing existing content and student performance data, the system identifies gaps and generates targeted exercises to strengthen weak areas. It can also offer more advanced challenges to skilled students. Automated content generation not only eases the burden on teachers but ensures a steady supply of fresh, relevant materials that maintain student engagement and growth.
10. Adaptive Scaffolding
AI can automatically adjust the level of step-by-step support based on the learner’s performance, gradually reducing assistance as the student gains confidence and competence.
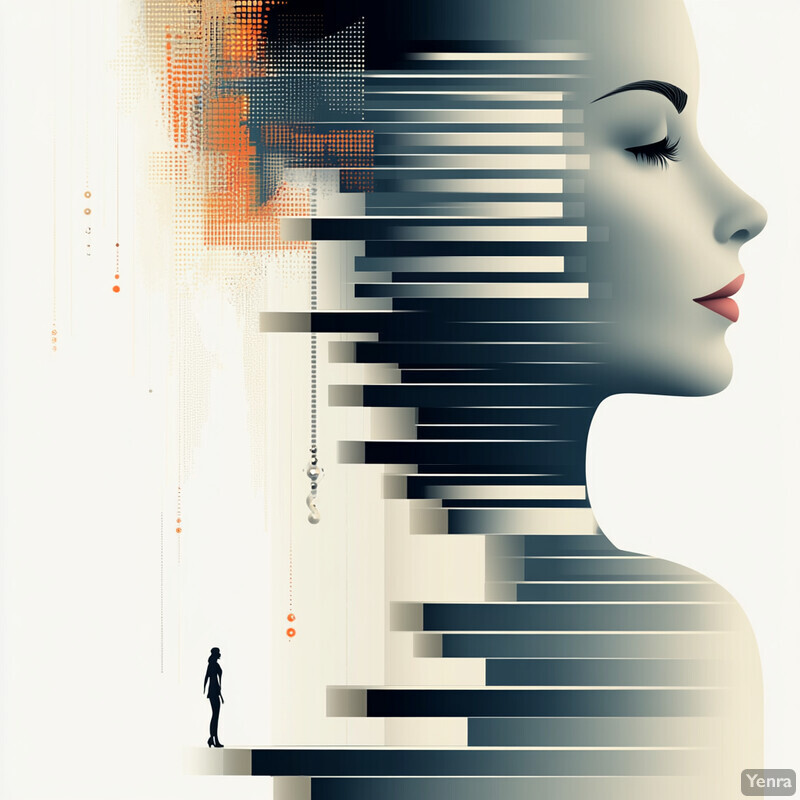
Scaffolding involves providing structured support to help learners bridge from their current ability level to more complex skills. AI-powered tutors dynamically adjust the type and amount of scaffolding based on student performance and confidence signals. Early on, a student might receive step-by-step instructions or worked examples. As their proficiency grows, the tutor gradually reduces these supports, encouraging more independent problem-solving. This careful calibration helps learners develop both competence and autonomy. Over time, students learn to rely less on external guidance and more on their own reasoning, fostering self-efficacy and long-term mastery.
11. Multi-modal Interaction
AI advancements allow tutors to incorporate speech, handwriting recognition, and visual understanding, enabling students to interact with the system more naturally.
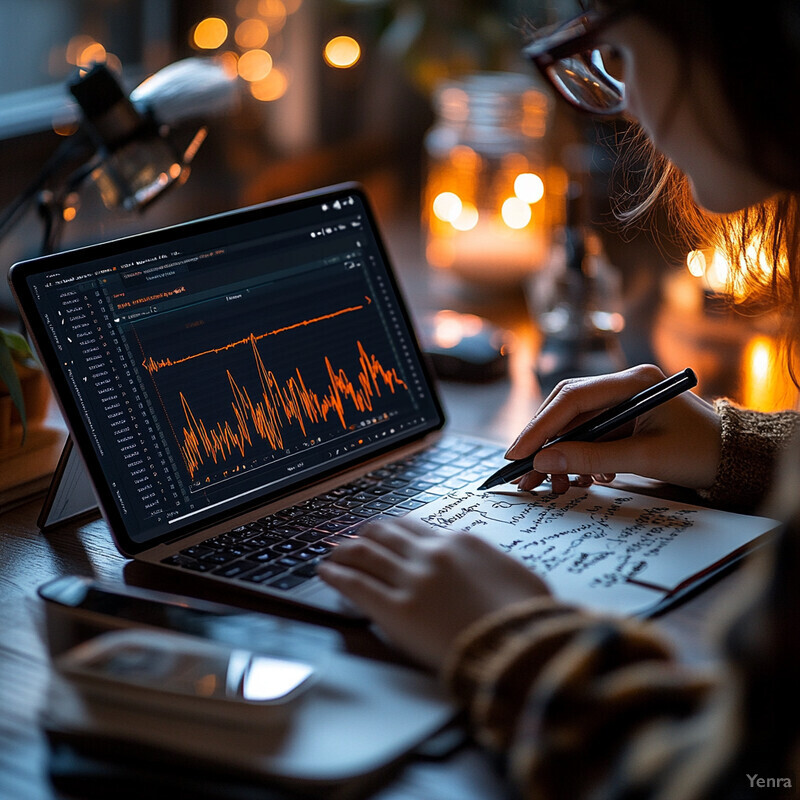
While traditional computer-based tutors relied heavily on text input and mouse clicks, modern AI technologies allow cognitive tutors to understand and respond to multiple forms of communication. Speech recognition lets students ask questions or explain reasoning verbally, while handwriting recognition and visual analysis handle diagrams or equations drawn on tablets. This multi-modal approach creates more natural, intuitive learning experiences that mirror the variety of communication modes used in classrooms. By accommodating different learning preferences and making the interface more engaging and accessible, these tutors reach a broader range of students and learning styles.
12. Longitudinal Learner Tracking
Intelligent tutoring systems can maintain a long-term profile of each student’s strengths, weaknesses, and learning styles, drawing from longitudinal data to guide long-term skill development.
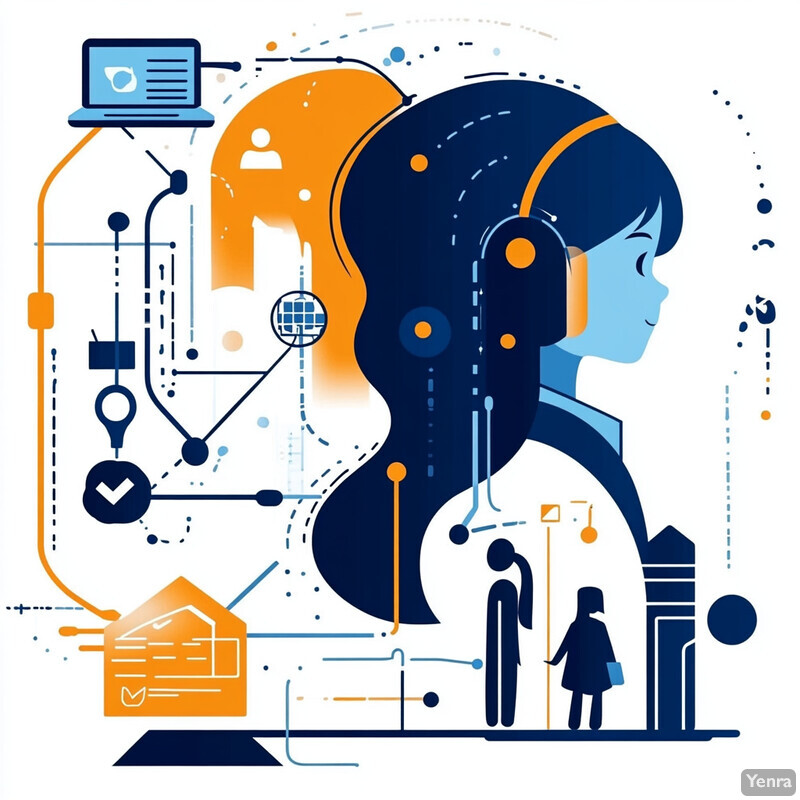
Over time, cognitive tutors accumulate rich data on individual learners, capturing growth, struggles, and patterns of concept mastery. AI can analyze this longitudinal data to inform long-term learning strategies. If a student consistently struggles with a certain concept year over year, the tutor can introduce targeted review sessions or different instructional methods. Conversely, identifying areas of strong competence can guide learners to more advanced topics, ensuring continuous intellectual challenge. By maintaining a long-term perspective, cognitive tutors become lifelong learning companions, supporting not just immediate mastery but sustained intellectual development.
13. Contextualized Learning Experiences
Cognitive tutors, enhanced by AI, can integrate real-world applications and simulations that adapt based on the student’s learning progress, making abstract concepts more concrete and relatable.
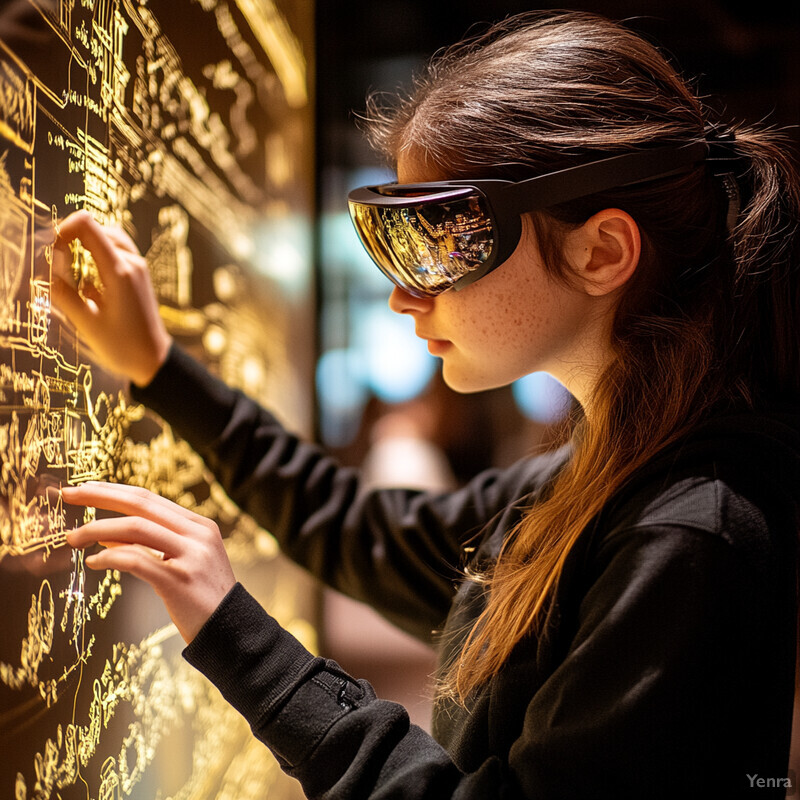
AI-powered tutors can go beyond abstract exercises by embedding learning in authentic, context-rich scenarios. Simulations, virtual labs, and problem-based learning activities can be automatically adapted to match a student’s skill level and interests. For example, a math problem might be framed in terms of engineering challenges or environmental data if the student has shown enthusiasm for those topics. By making learning more relevant and situating new concepts in real-world contexts, these tutors help students understand why they are learning something, which increases motivation, deepens comprehension, and encourages long-term retention.
14. Peer Learning Simulations
AI can model and simulate peer interactions, allowing students to practice collaborative problem-solving within the tutor environment, receiving guidance on teamwork and communication skills.
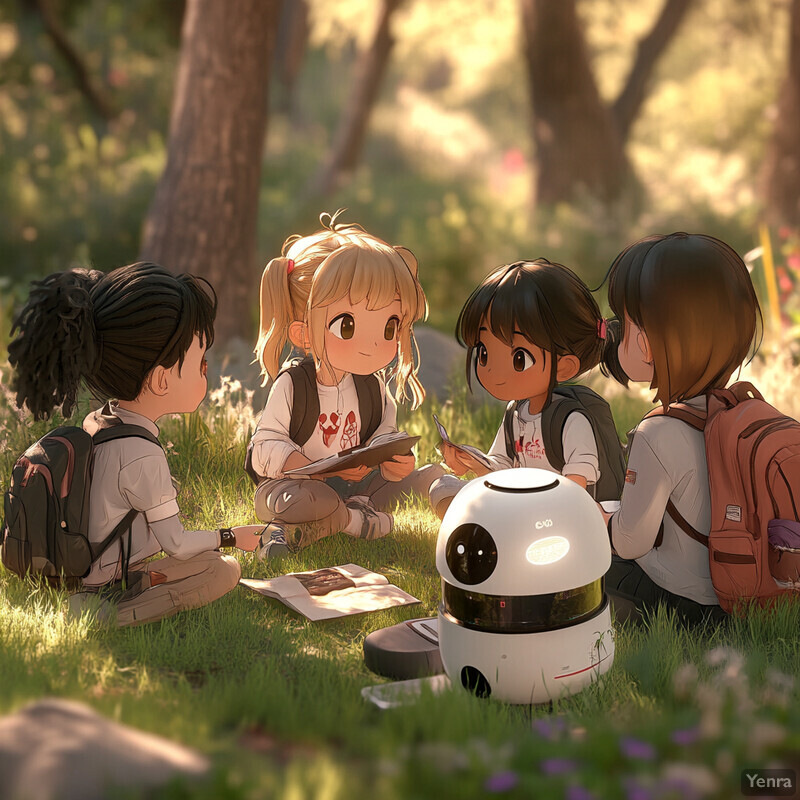
Collaboration and social learning are integral to education, yet traditional computer tutors often focus on individual tasks. AI-enhanced cognitive tutors simulate peer interactions, allowing learners to practice teamwork, communication, and problem-solving in virtual group settings. The system can model other “peers” who pose questions, propose partial solutions, or require explanation, prompting the learner to articulate their reasoning. Such simulations not only bolster cognitive skills but also teach vital social and collaborative competencies, bridging the gap between isolated practice and the cooperative, dialogue-rich learning that occurs in classrooms and professional environments.
15. Metacognitive Support
By analyzing patterns of student behavior, AI-driven tutors can help learners develop strategies for planning, monitoring, and evaluating their own learning, fostering stronger metacognitive skills.
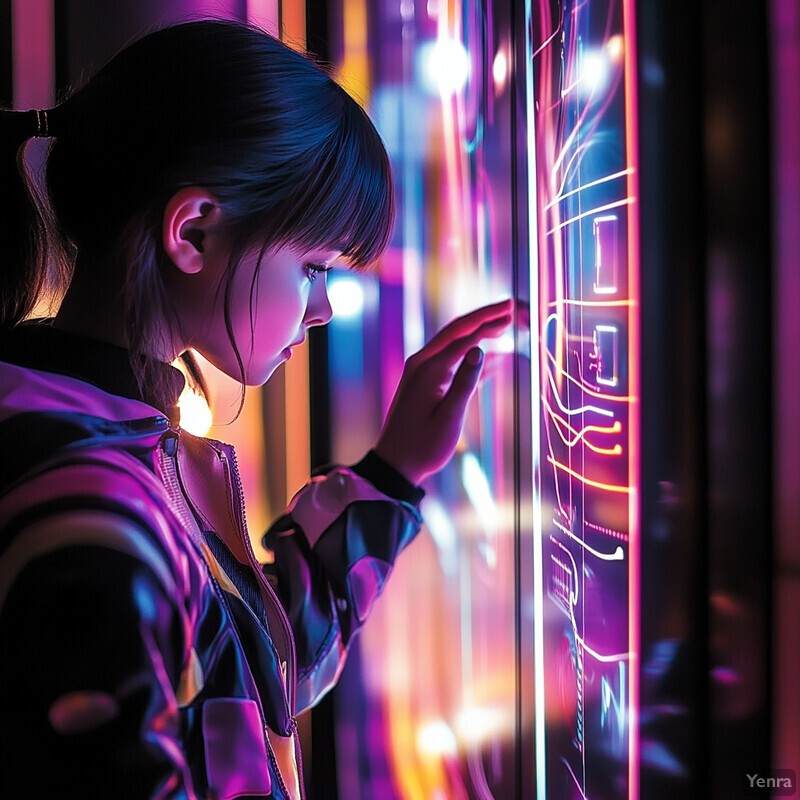
Strong learners are often those who understand their own thinking processes. AI-powered cognitive tutors can analyze patterns in a student’s work to identify their metacognitive strategies—or lack thereof. By noting when students plan their approach, check their answers, or reflect on mistakes, the tutor can provide prompts to improve these skills. Over time, learners gain insights into their own learning styles, strengths, and weaknesses. This self-awareness builds resilience, strategic thinking, and a growth mindset. In essence, the tutor not only teaches academic content but also empowers students to become more effective, independent learners.
16. Adaptive Pacing and Sequencing
AI can fine-tune the presentation order and timing of educational materials, ensuring learners are neither overwhelmed nor under-challenged, thus maintaining optimal cognitive load.
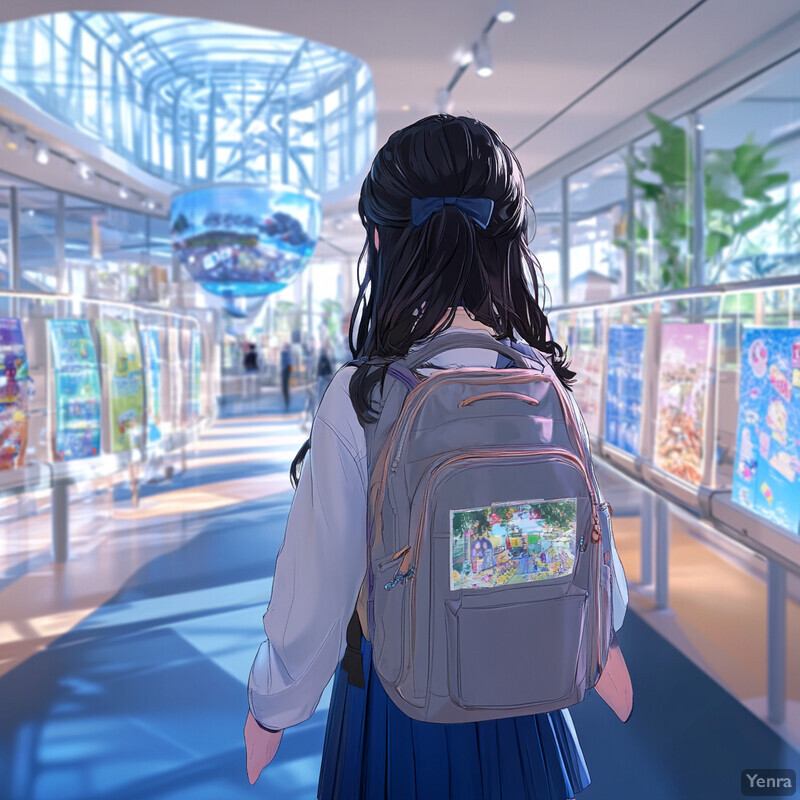
Striking the right pace and sequence of instruction is challenging, as students have varying needs and learning speeds. AI-driven cognitive tutors continuously analyze learner performance, adjusting the order and difficulty of tasks in real-time. If a student is excelling, the tutor can introduce more advanced materials sooner, while learners who need more support may receive additional practice or remedial explanations before moving on. This tailored pacing prevents boredom and frustration, keeps motivation high, and ensures that learners build a solid foundation before tackling more complex concepts, leading to a more efficient and rewarding learning journey.
17. Interoperability with LLMs and Chatbots
Integrating large language models allows cognitive tutors to hold richer, more natural dialogues with students, offering explanatory depth and responding flexibly to open-ended questions.
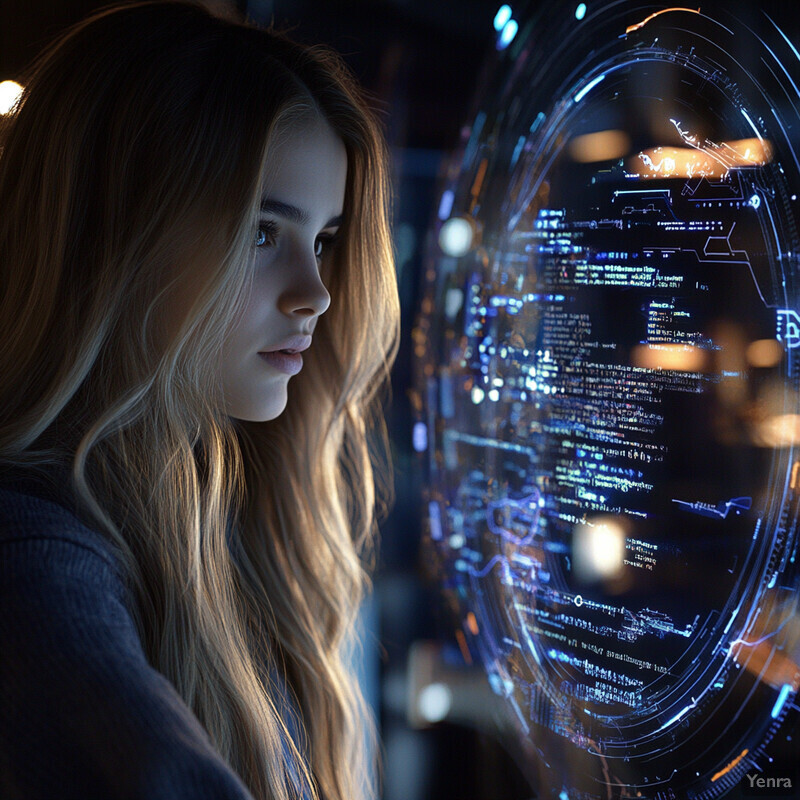
The integration of large language models (LLMs) and advanced chatbots into cognitive tutors unlocks new dimensions of interaction. Instead of being limited to structured question-answer formats, learners can engage in more open-ended dialogues with the tutor. The system can clarify concepts, respond to follow-up questions, or provide more elaborate examples—much like a human tutor would. This richer conversational capability allows students to explore topics more deeply, test their understanding by asking “what if” questions, and ultimately achieve a more nuanced and flexible grasp of the material.
18. Data-driven Curriculum Refinement
Educators and curriculum designers can draw from tutor analytics to identify common misconceptions and knowledge gaps across cohorts, refining course content for subsequent learners.
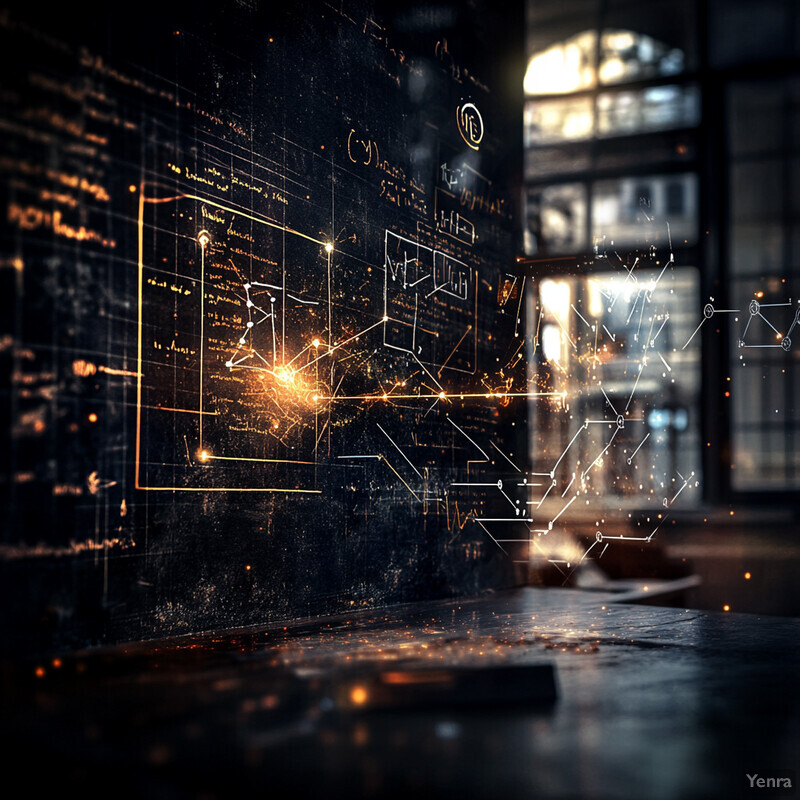
Cognitive tutors generate a wealth of data about how students interact with content, where they succeed, and where they struggle. Analyzing this data with AI tools helps educators and curriculum designers identify common misconceptions, inefficient teaching sequences, or content that’s too easy or too hard. In response, they can revise lessons, add or remove certain exercises, and reorganize the curriculum to better align with learners’ needs. Over time, this leads to an iterative improvement cycle where the curriculum continually evolves, ultimately becoming more effective and responsive to student outcomes.
19. Cultural and Linguistic Sensitivity
AI-enhanced tutors can adapt to different languages, dialects, and cultural contexts, ensuring accessible, inclusive learning experiences for diverse student populations.
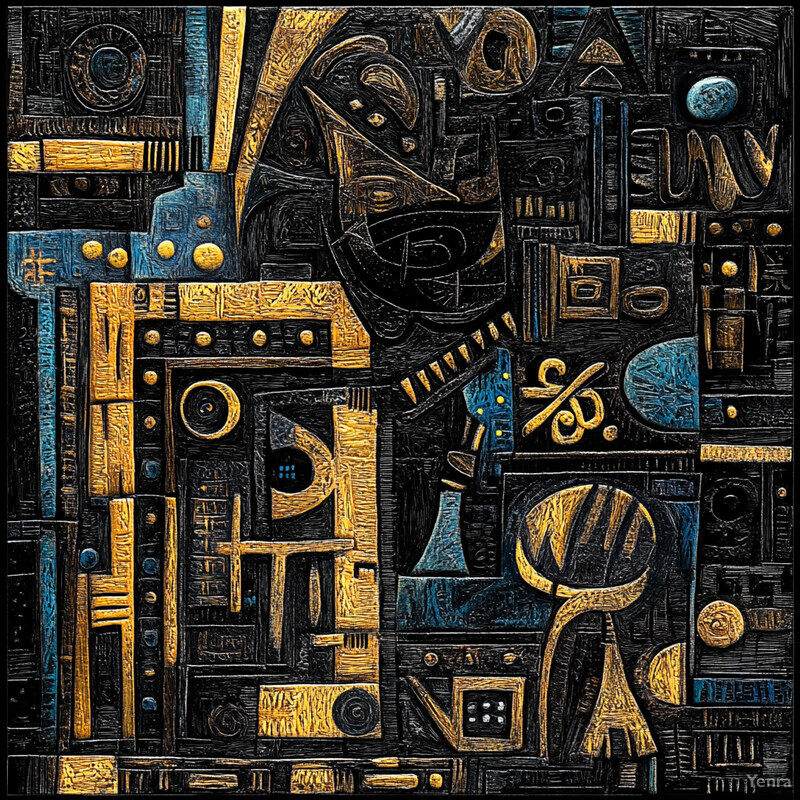
Global classrooms are increasingly diverse, with students from various linguistic and cultural backgrounds. AI-enhanced cognitive tutors can adapt their language, examples, and references to resonate with learners’ cultural contexts. They can translate content into different languages, respect local educational norms, and include culturally relevant scenarios or analogies. This sensitivity not only makes learning more accessible and inclusive but can also improve student motivation and comprehension. By acknowledging and valuing diversity, cognitive tutors help ensure that all learners receive equitable support tailored to their cultural identities and linguistic abilities.
20. Holistic Learner Profiles
By combining cognitive, behavioral, and affective indicators, AI allows cognitive tutors to treat learners as whole individuals, shaping instruction that aligns not just with skills, but also with motivation and engagement levels.
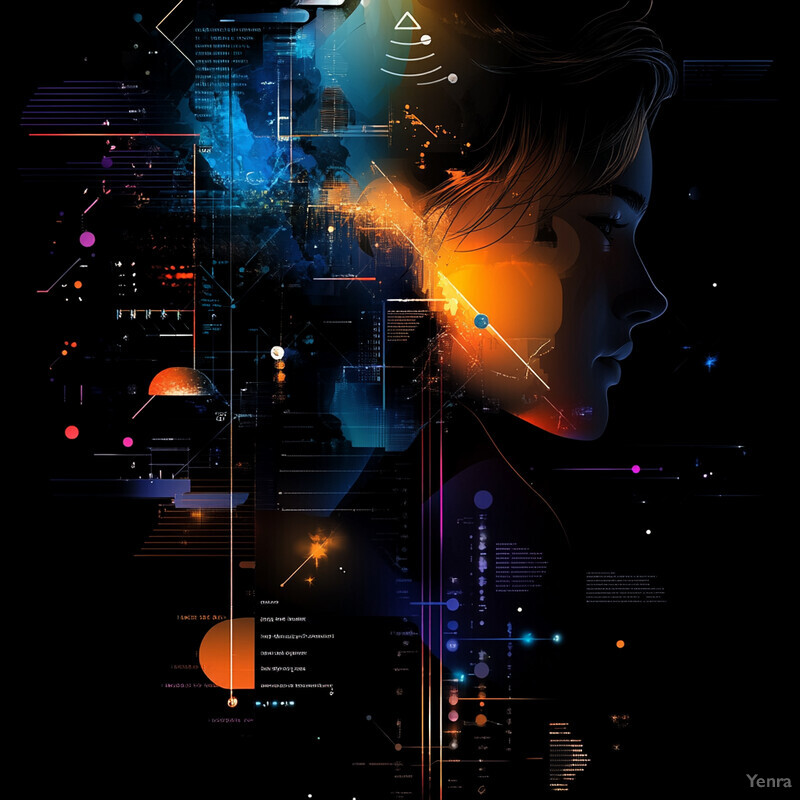
Education is not solely about academic aptitude; motivation, engagement, and personal interests also play critical roles. AI-powered cognitive tutors combine cognitive data (such as mastery of concepts) with affective and behavioral indicators (such as persistence, help-seeking patterns, or emotional states) to create a holistic learner profile. This integrated perspective allows the tutor to select strategies that address not just what a student knows, but how they feel and behave while learning. By considering the whole learner, cognitive tutors become more empathetic, responsive, and ultimately more effective in nurturing both intellectual growth and personal development.