1. Real-Time Data Fusion
AI algorithms integrate data streams from diverse sources—such as ground-based sensors, satellite imagery, weather stations, and traffic patterns—to create a more holistic and accurate representation of current air quality conditions.
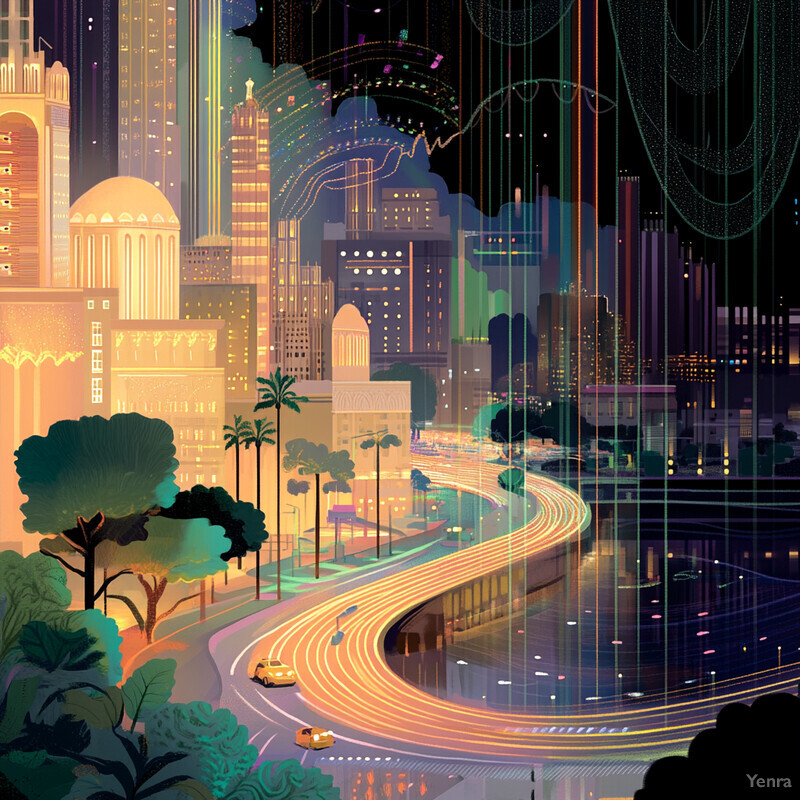
AI technologies integrate a wide range of data sources—ranging from ground-based sensor networks and satellite remote sensing to traffic patterns and meteorological stations—to create a unified and dynamic picture of air quality. By intelligently merging these disparate data streams, machine learning algorithms can reconcile differences in resolution, coverage, and measurement accuracy. This ensures that environmental agencies and research institutions gain deeper insights into spatial and temporal pollution trends. Real-time fusion of data also allows for immediate identification of emerging pollution hotspots and the ability to adjust monitoring strategies or issue public alerts as conditions evolve.
2. High-Resolution Spatial Modeling
Advanced machine learning models can downscale broad regional air quality data into hyperlocal forecasts, mapping pollution levels at neighborhood or even street-level resolution.
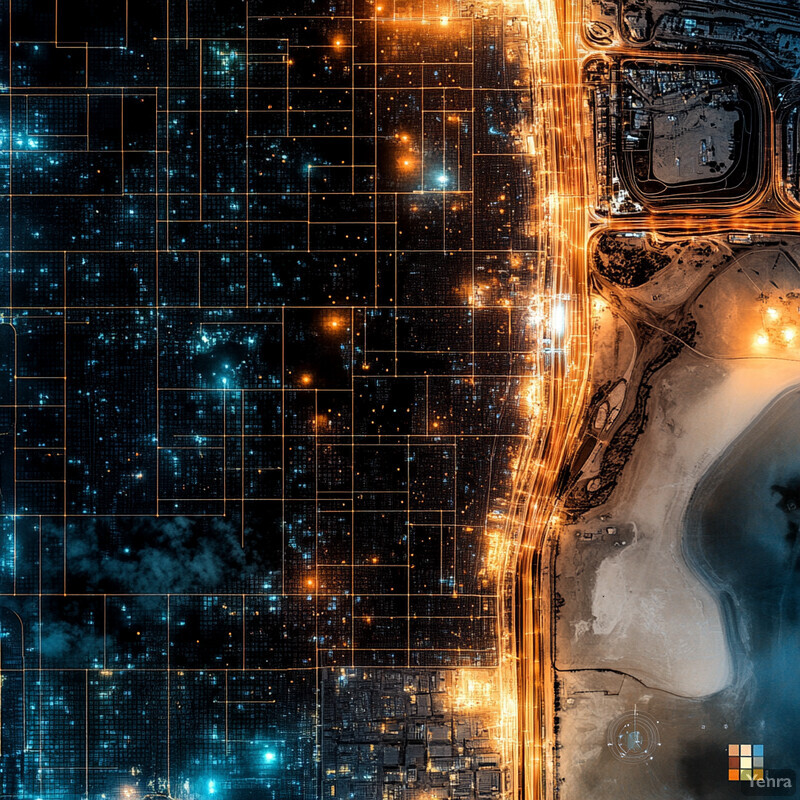
Traditionally, air quality data has been available at coarse geographical resolutions, providing only a limited understanding of pollution distribution. AI and advanced machine learning techniques enable downscaling of coarse data into high-resolution spatial maps at the street or neighborhood level. These fine-grained models account for variations in emissions sources, topography, and local meteorology. The result is an extremely detailed snapshot of pollution patterns that can help policymakers pinpoint problem areas and tailor mitigation efforts more effectively, ultimately reducing exposure for communities most at risk.
3. Temporal Forecasting
Deep learning architectures, like recurrent neural networks (RNNs) or long short-term memory (LSTM) models, are used to predict how pollutant concentrations will change over the coming hours, days, or weeks, aiding in proactive pollution management.
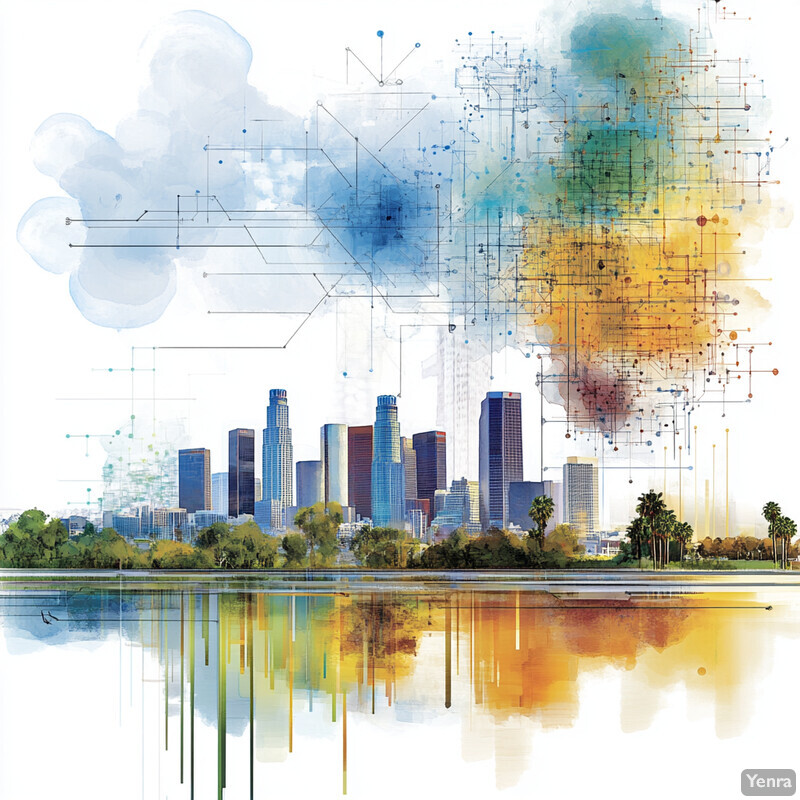
Predicting how air quality will change over time is a complex task influenced by weather patterns, emission rates, and chemical reactions in the atmosphere. AI-driven temporal forecasting models, including recurrent neural networks (RNNs) and long short-term memory (LSTM) architectures, use historical and current data to learn patterns and anticipate future pollution levels. These predictions enable city planners, environmental agencies, and the public to prepare for expected pollution episodes—whether hours, days, or weeks in advance—making it possible to implement timely interventions, advise sensitive groups, or adjust traffic flows preemptively to reduce emissions.
4. Intelligent Sensor Placement
AI-driven optimization algorithms identify the best locations to install new sensors or reposition existing ones, ensuring coverage of critical areas and reducing monitoring gaps.
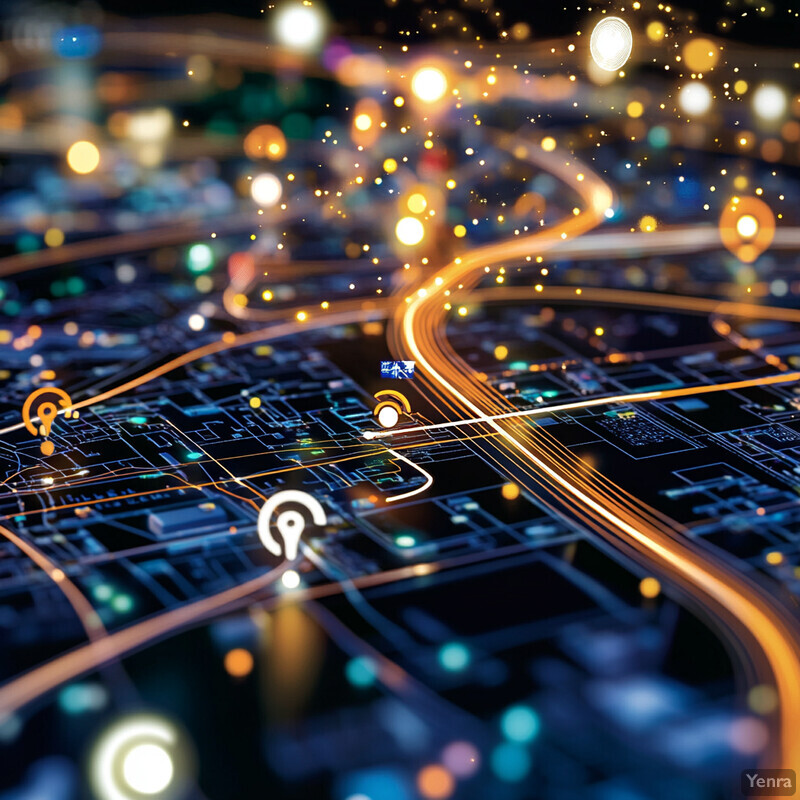
Determining where to place air quality sensors is critical for capturing accurate and representative data. AI-powered optimization tools leverage geographic, demographic, and historical pollution data to identify sensor placement strategies that maximize coverage and cost-effectiveness. By simulating different configurations, these algorithms find locations that reduce blind spots, capture complex pollution gradients, and increase the reliability of subsequent analyses. Intelligent sensor placement, therefore, ensures that monitoring networks provide a comprehensive view of air conditions, guiding data-driven policy and infrastructure decisions.
5. Anomaly Detection
Unsupervised learning methods detect unusual pollution spikes or sensor malfunctions, alerting authorities to unexpected events or the need for equipment maintenance.
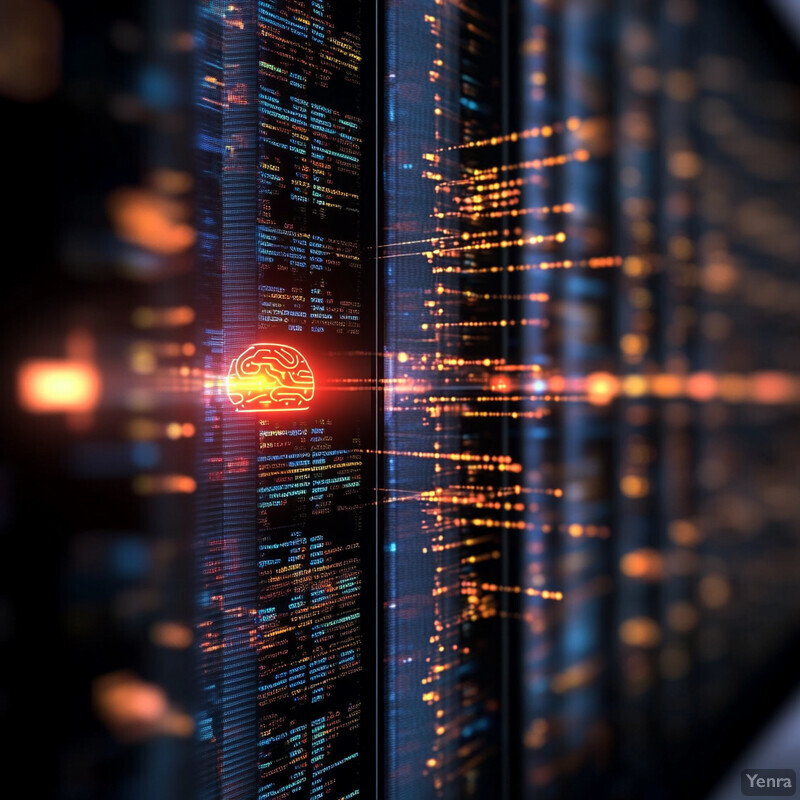
Environmental data can be erratic, influenced by sensor failures, power outages, weather extremes, or unexpected pollution spikes. Unsupervised machine learning methods, such as clustering and outlier detection algorithms, help identify anomalies in real-time data streams. By learning what “normal” looks like for a specific sensor or region, these models swiftly highlight deviations that might signal equipment malfunctions, unusual emission events, or spreading wildfires. Early detection of anomalies empowers agencies to intervene quickly—repairing faulty sensors, launching investigations, or issuing public health alerts—to prevent inaccurate data from skewing analyses or to mitigate health risks.
6. Data Gap Filling
Machine learning techniques can interpolate missing or corrupted data, improving the continuity and reliability of air quality records without costly additional monitoring infrastructure.
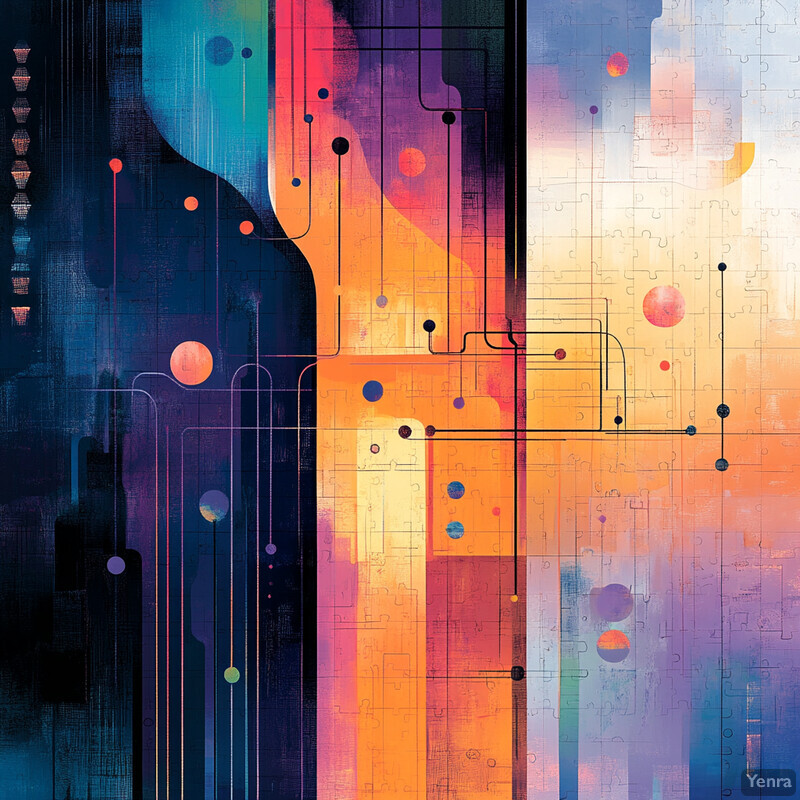
Air quality datasets often contain missing or corrupted values caused by sensor downtime, maintenance issues, or environmental interference. Machine learning techniques, including interpolation and data imputation methods, help fill these gaps without resorting to expensive manual corrections or additional sensor deployments. By training models on historical and complementary data, AI can seamlessly reconstruct missing information, thereby maintaining the continuity and quality of long-term records. Consistent data allows for more robust trend analysis, policy evaluation, and long-term environmental forecasting.
7. Emissions Source Attribution
By analyzing patterns in pollutant composition and flow, AI tools help isolate the most significant sources of emissions—factories, traffic, wildfires—informing targeted mitigation strategies.
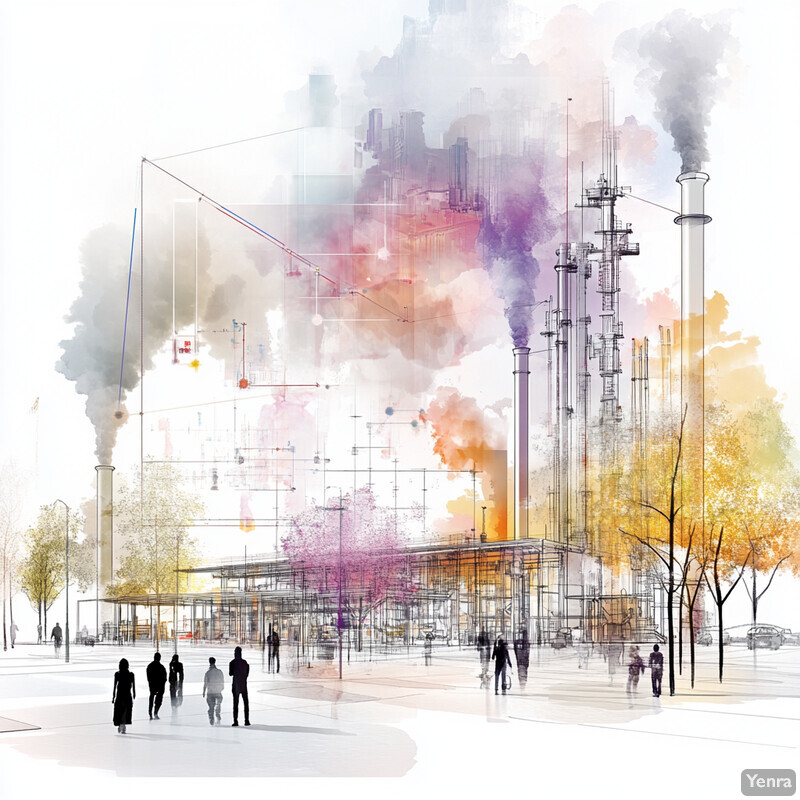
Pinpointing where pollutants come from—be it industrial facilities, vehicles, or natural events—can be challenging. AI-driven source attribution models analyze pollutant composition, atmospheric dispersion patterns, and wind trajectories to identify likely emission sources and their relative contributions. These models greatly enhance our understanding of local and regional pollution dynamics. By offering scientific evidence on the primary culprits behind poor air quality, policymakers can enact targeted controls and regulations, industry can adopt cleaner technologies, and the public can gain insight into how daily activities impact the air they breathe.
8. Predictive Analytics for Policy Impact
Simulation models enhanced with AI can test the future impact of proposed environmental policies, emission regulations, or changes in transportation infrastructure before they are implemented.
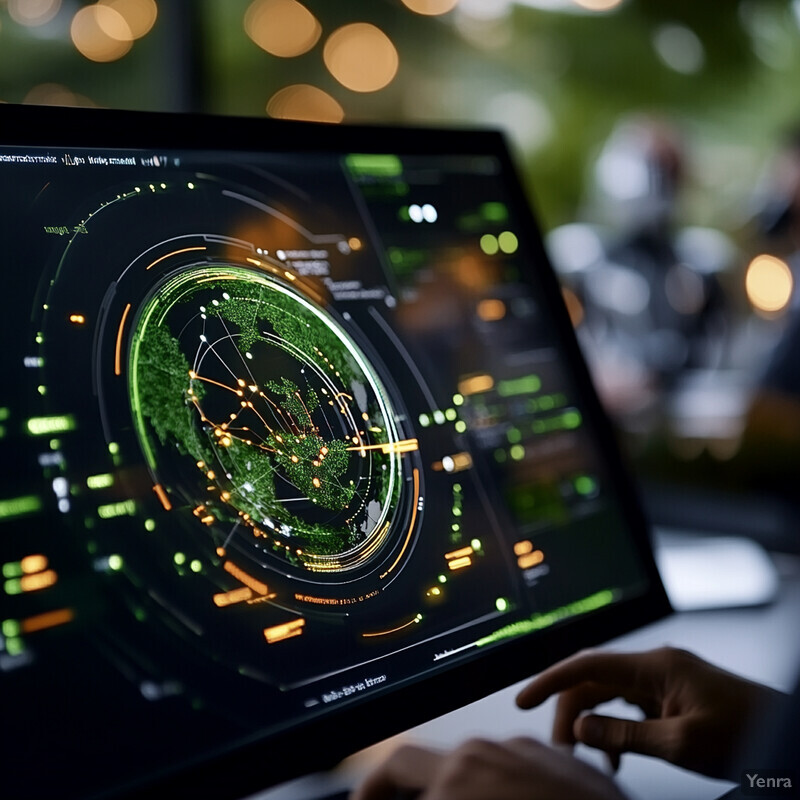
Developing environmental policies often involves complex trade-offs and long-term implications. AI-backed simulation models enable stakeholders to test different regulatory scenarios, emission standards, or transportation strategies before implementing them in reality. By forecasting how changes might influence pollutant concentrations, these predictive analytics tools help policymakers make evidence-based decisions. They can identify measures that yield the greatest environmental benefits, minimize economic costs, and lead to sustainable improvements in air quality—empowering governments and communities to craft more effective regulations.
9. Dynamic Air Pollution Alerts
Using real-time predictions, AI can issue timely warnings to the public when pollution is likely to exceed health thresholds, helping communities and individuals take preventive action.
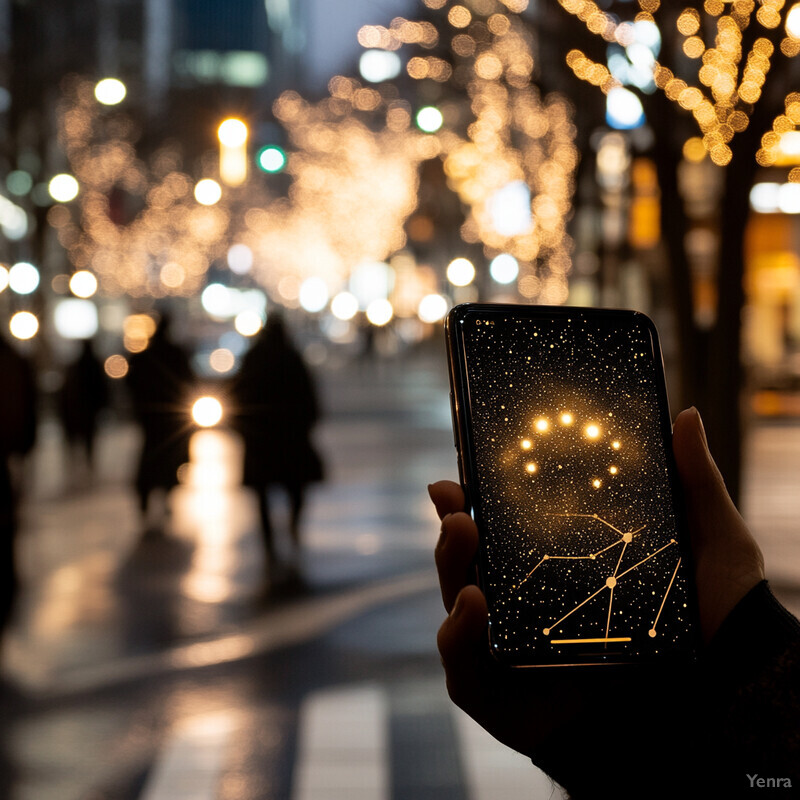
Real-time AI models assess current conditions and predict short-term changes in air quality, issuing alerts before pollution reaches hazardous levels. Automated alert systems allow city authorities and health agencies to warn vulnerable populations—such as children, the elderly, and those with respiratory conditions—to take precautions. Timely advisories, disseminated through mobile applications, websites, or social media, can recommend avoiding outdoor exercise, using protective masks, or adjusting commutes. Ultimately, dynamic pollution alerts help individuals make informed decisions and reduce exposure to harmful pollutants.
10. Integrating Traffic and Meteorological Data
AI-driven models correlate meteorological patterns and traffic dynamics to predict how changes in weather or rush-hour volumes will affect local air quality.
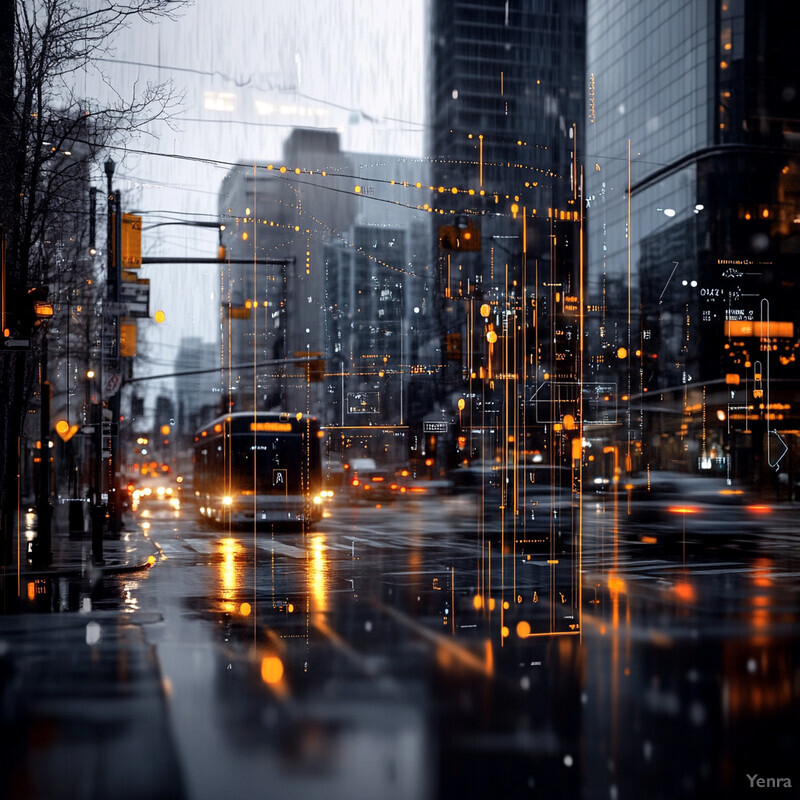
Traffic congestion, vehicle emissions, and weather patterns profoundly affect air quality. By integrating traffic flow data with meteorological variables like wind speed, temperature, and humidity, AI models predict how congestion patterns and weather changes influence pollution dispersal. As a result, transportation planners can develop strategies to alleviate congestion at critical times, environmental agencies can fine-tune monitoring efforts, and individuals can plan travel to minimize exposure. This integration creates a more nuanced understanding of the interdependencies between urban mobility, atmospheric conditions, and air quality.
11. Health Impact Forecasting
By linking pollution exposure data with epidemiological models, AI can forecast potential health outcomes—such as asthma attacks or hospital visits—helping healthcare systems prepare for pollution events.
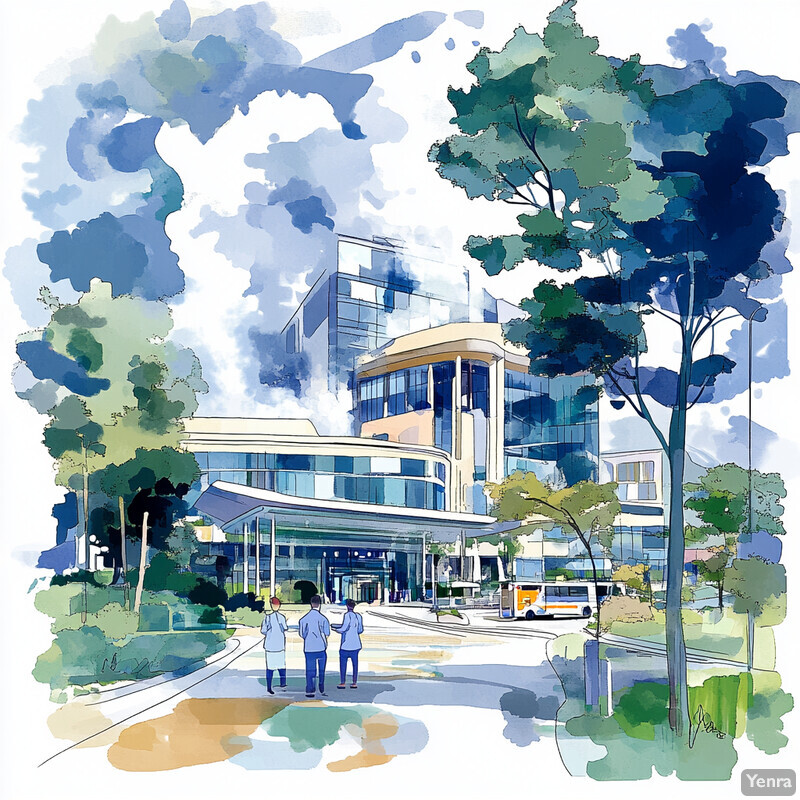
Air pollution exposure is linked to respiratory conditions, heart disease, and other adverse health effects. AI-driven health impact forecasting models integrate medical, demographic, and pollution data to predict the potential burden of illness during anticipated pollution events. These tools allow healthcare providers to prepare for increases in hospital admissions, pharmacies to stock essential medications, and public health officials to allocate resources more effectively. Over time, these models can also help evaluate the efficacy of interventions in improving population health outcomes.
12. Automated Compliance Checking
AI can assist regulators in quickly verifying whether industries are meeting emission standards, reducing manual oversight and increasing efficiency in environmental governance.
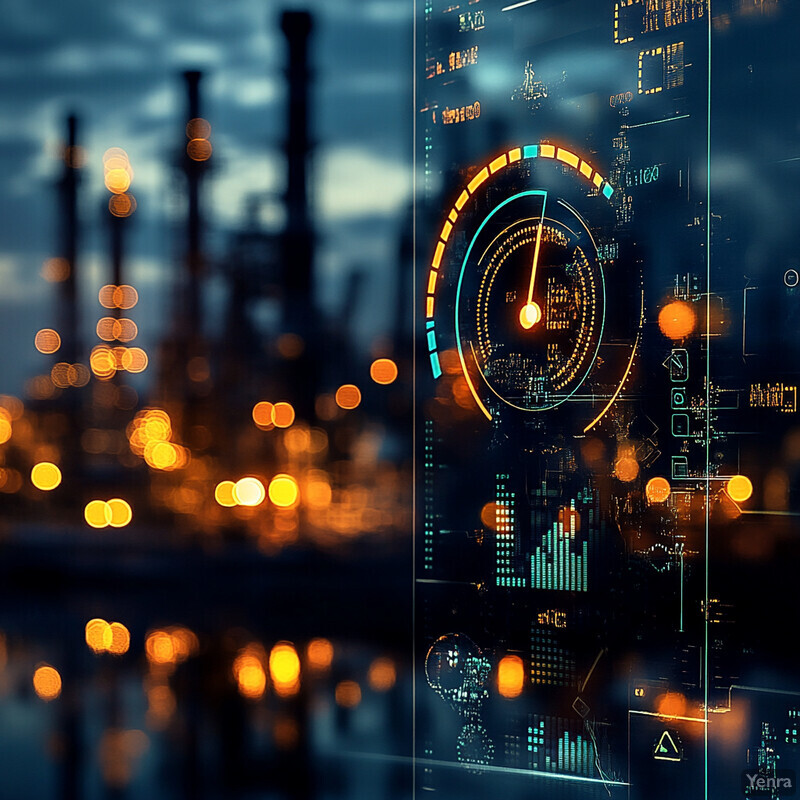
Regulatory agencies often face the challenge of ensuring industries adhere to emission standards. AI-powered compliance tools analyze continuous streams of emissions data from industrial facilities and compare them against legal thresholds. By spotting patterns of non-compliance in real time, these systems reduce the need for manual inspections and accelerate enforcement actions. This not only makes environmental regulation more efficient but also sends a strong message to polluters that violations will be swiftly identified and addressed.
13. Edge Computing for On-Site Analysis
AI models deployed directly on sensor devices (edge computing) enable real-time analytics without relying on continuous network connectivity, making remote or mobile monitoring more feasible.
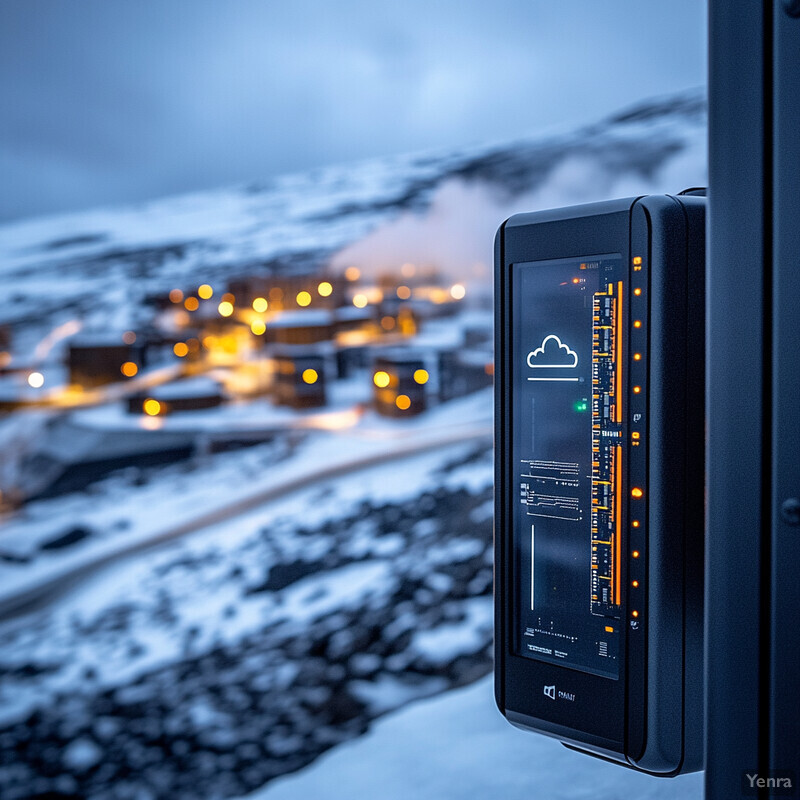
Not all air quality monitoring can rely on stable network connectivity. In remote or mobile monitoring scenarios—such as on ships, drones, or in underserved communities—devices equipped with edge computing capabilities run AI models directly on-site. These low-latency computations enable immediate decision-making, like adjusting sensor parameters or triggering localized alerts, without waiting for cloud-based processing. The result is more responsive and resilient air quality monitoring, especially in disaster-stricken areas or regions with limited communication infrastructure.
14. Satellite Image Analysis
Computer vision algorithms applied to satellite data help detect pollutant plumes, track smoke from wildfires, and estimate emissions in areas lacking ground sensors.
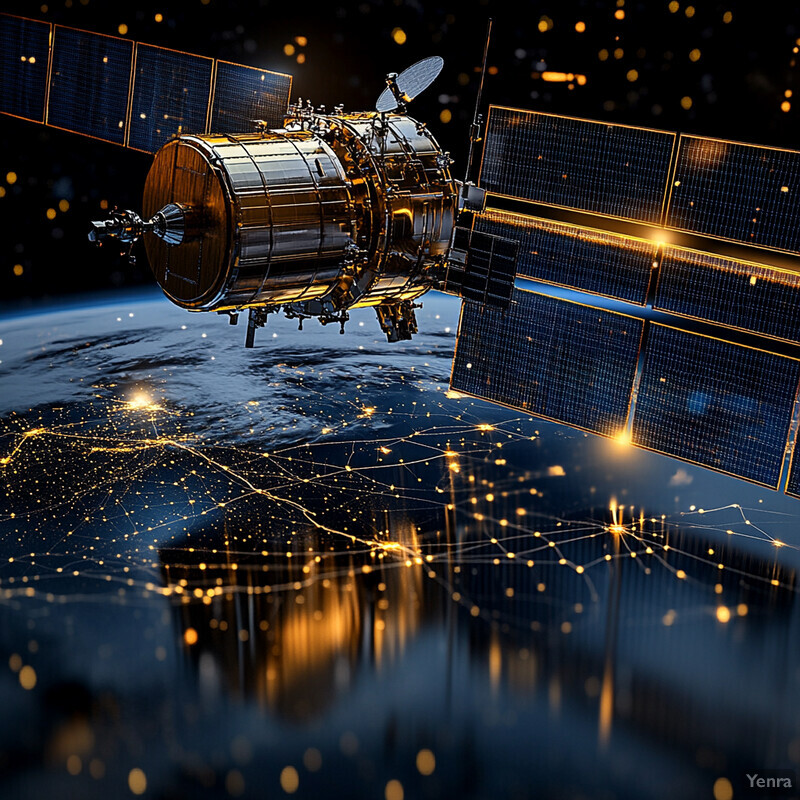
Satellites provide broad coverage of Earth’s atmosphere, capturing pollutant distributions on regional and global scales. Using computer vision and deep learning to interpret satellite imagery, AI models identify pollution plumes, track wildfire smoke, and estimate emissions for remote areas lacking ground-based sensors. These global snapshots enhance our understanding of cross-border pollution transport and long-term environmental changes. They also serve as a valuable supplement to terrestrial monitoring efforts, closing critical data gaps and improving modeling accuracy.
15. AI-Enhanced Source Modeling
Inverse modeling techniques supported by AI estimate not just where pollution is coming from, but in what quantity, supporting more informed and effective remediation measures.
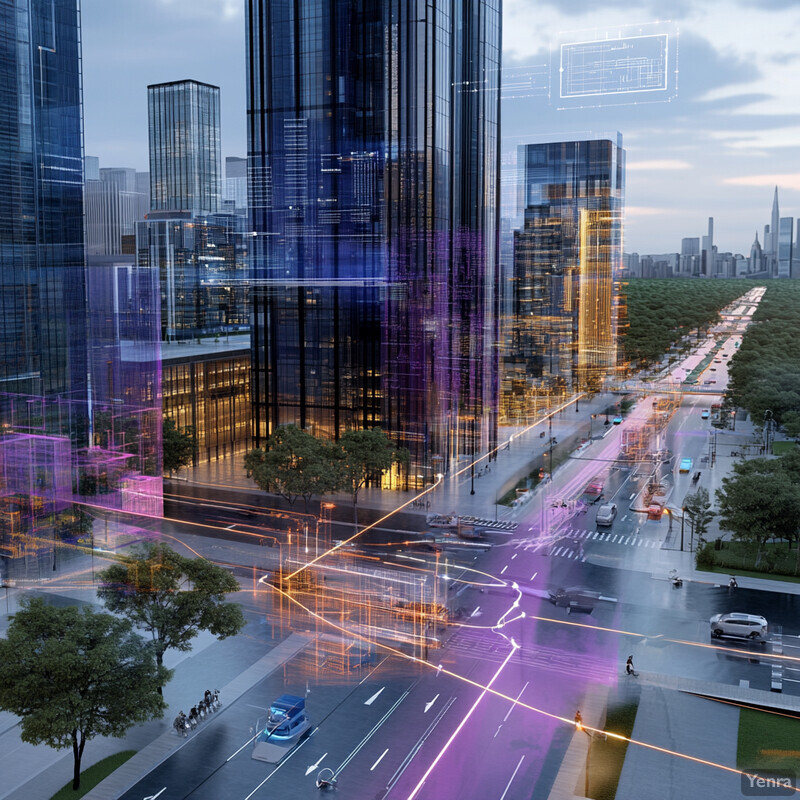
Inverse modeling—where observed pollutant concentrations are used to deduce emission rates and locations—is complex due to the intricate chemistry and physics at play. AI enhances these models by rapidly exploring multiple hypotheses and finding the configurations that best explain observed data. The result is more accurate quantification of emissions and better-informed decisions about where to implement mitigation measures. This improved source modeling capability ensures that interventions directly address the most significant pollution contributors, making mitigation efforts more cost-effective and impactful.
16. Adaptive Calibration of Sensors
Machine learning automatically recalibrates low-cost sensors over time to maintain accuracy despite drift, temperature changes, or component aging.
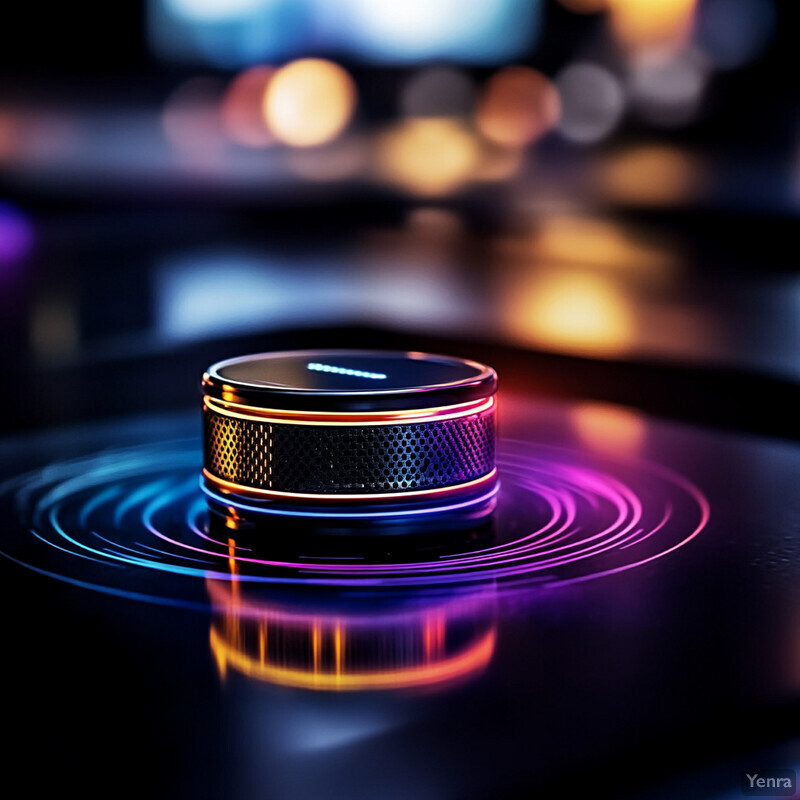
Over time, low-cost sensors, which are vital for dense monitoring networks, may drift away from their initial calibration due to temperature swings, humidity, and material aging. AI-driven calibration models continually learn from reference-grade sensors or known standards to adjust and correct sensor readings automatically. This adaptive approach maintains data quality without requiring constant human intervention or costly replacements. As a result, widespread low-cost sensor deployments remain viable and consistent, providing a stable foundation for larger-scale environmental analyses.
17. Urban Planning Optimization
City planners can use AI-driven insights to layout green spaces, redesign traffic flows, and optimize infrastructure placement to minimize pollutant hotspots.
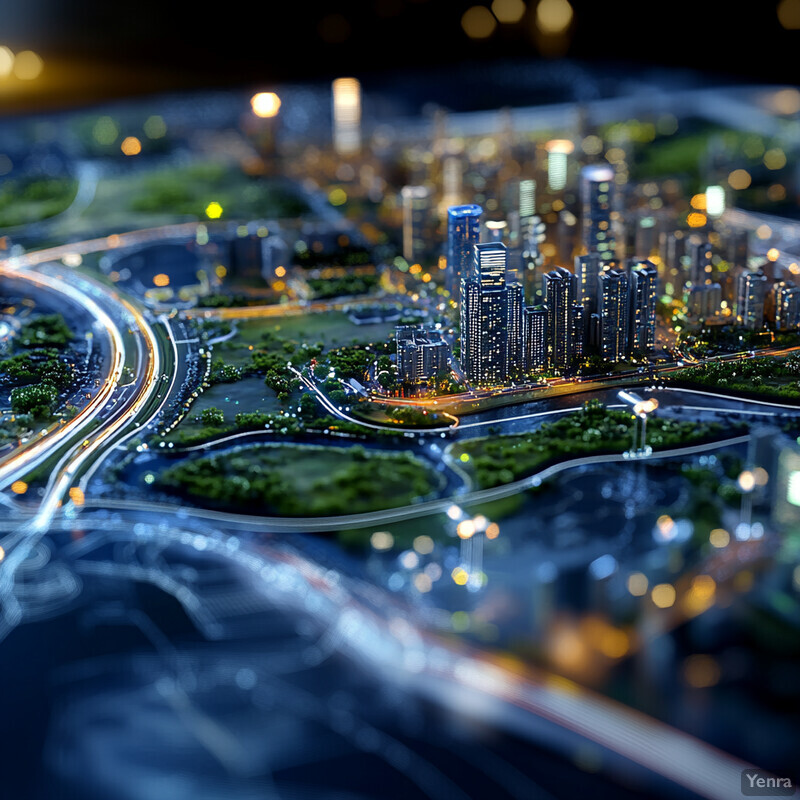
The placement of highways, parks, industrial zones, and green buffers influences local air quality. AI models can simulate various urban design scenarios, analyzing how infrastructure changes might alter pollution patterns. By identifying configurations that reduce pollutant hotspots—like adding greenery to intercept particulates or optimizing traffic flows around schools—urban planners can create healthier and more sustainable communities. This data-driven approach to city development ensures that future growth aligns with environmental and public health objectives.
18. Personalized Exposure Estimation
Mobile and wearable devices equipped with AI-driven predictive models provide individuals with personalized air quality assessments, guiding decisions like route selection to minimize inhalation of harmful pollutants.
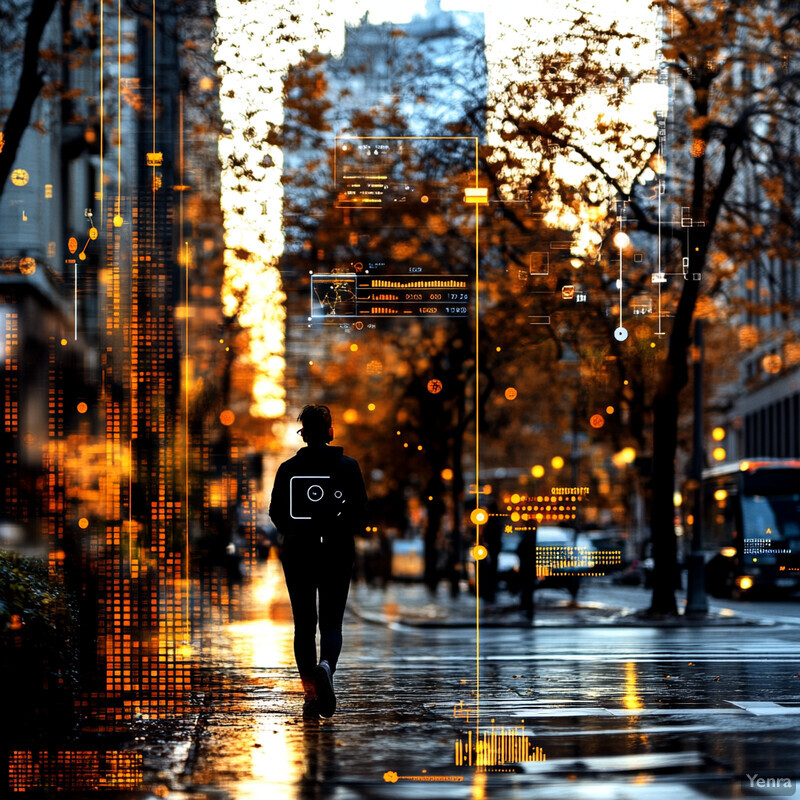
Personal exposure to pollutants can vary widely depending on one’s daily activities and routes. AI models that integrate wearable sensor data, location information, and environmental conditions can estimate how much pollution an individual is likely to encounter throughout the day. Such personalized forecasts empower citizens to make informed choices, such as selecting cleaner routes for walking or cycling, using protective equipment in high-exposure areas, or timing outdoor activities when air quality is better. Ultimately, personalized estimates help people minimize their health risks in polluted environments.
19. Scenario Analysis for Climate Change
AI-powered scenario modeling predicts how changing climate conditions—rising temperatures, altered precipitation patterns—might affect future air quality trends.
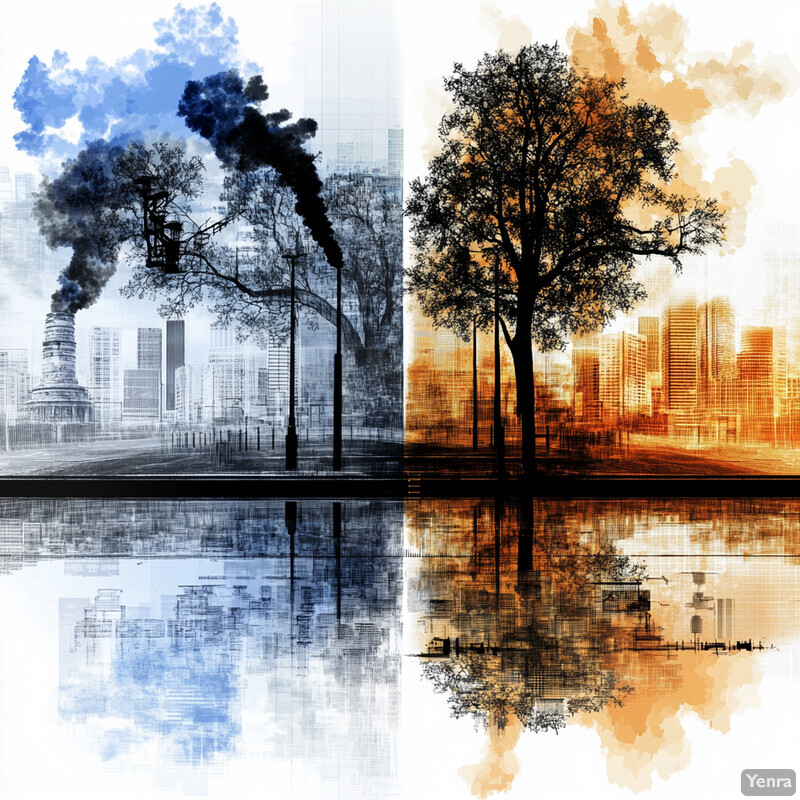
Climate change is altering weather patterns, affecting everything from wind speeds and precipitation to temperature inversions that trap pollutants. AI-driven scenario analysis examines how these evolving meteorological conditions may influence future pollution trends. Through climate models coupled with air quality simulations, researchers can anticipate new hotspots, seasonal shifts in pollution levels, or changes in pollutant chemistry. Understanding these long-term projections allows policymakers, scientists, and communities to plan climate adaptation strategies that protect public health and maintain livable cities.
20. Enhanced Public Engagement Tools
Intelligent visualization platforms and natural language summaries, powered by AI, make it easier for non-experts to understand air quality data and take informed actions, strengthening community involvement and awareness.
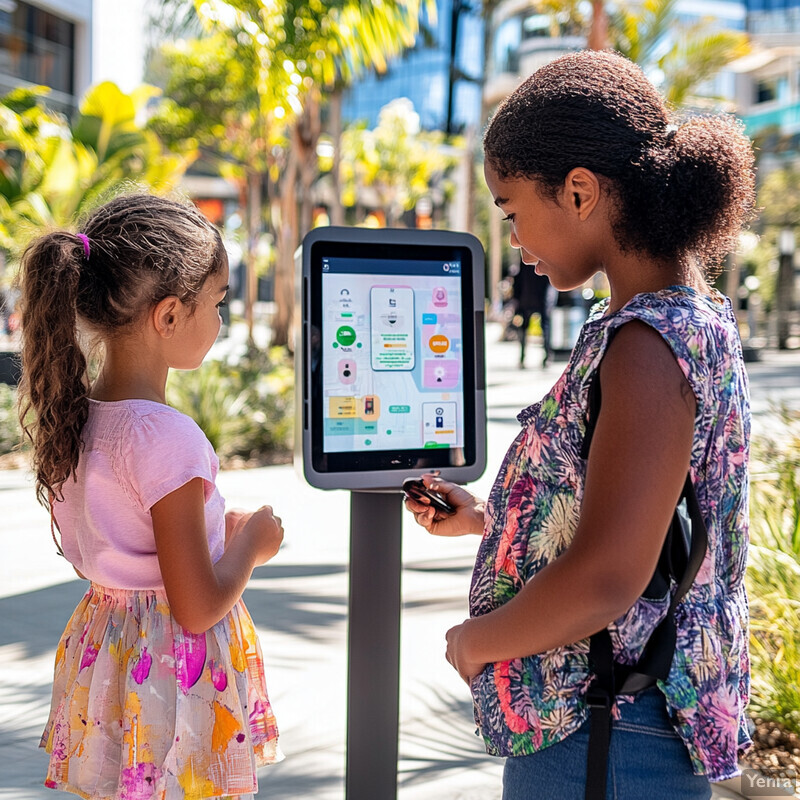
Data visualization and natural language processing (NLP) techniques powered by AI help translate complex air quality information into intuitive, user-friendly formats. Interactive maps, charts, and explanatory summaries break down technical details, allowing the public to understand local air pollution issues without needing specialized expertise. These accessible tools support community engagement, encourage environmental stewardship, and foster transparent communication between policymakers, scientists, and citizens. By making air quality data more comprehensible, these platforms empower individuals to participate in informed dialogues and collectively advocate for cleaner, healthier environments.