1. Data Integration from Diverse Sources
AI aggregates and synthesizes data from a wide range of sources, including polls, social media, and demographic statistics, to provide a more comprehensive view of voter sentiment.
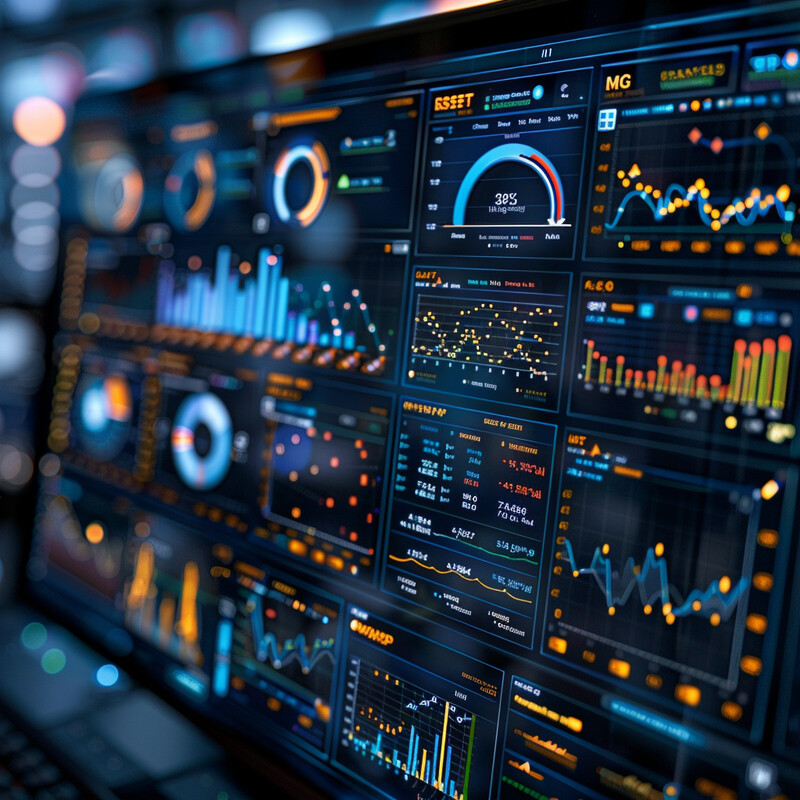
AI excels at integrating and synthesizing diverse data sources, including polling data, social media activity, economic indicators, and demographic statistics. By aggregating this information, AI can offer a comprehensive and nuanced view of the electoral landscape, which helps in creating more accurate and robust forecasting models.
2. Real-time Sentiment Analysis
AI analyzes social media and online content in real time to gauge public sentiment and political trends, enabling forecasters to see shifts in opinion as they occur.
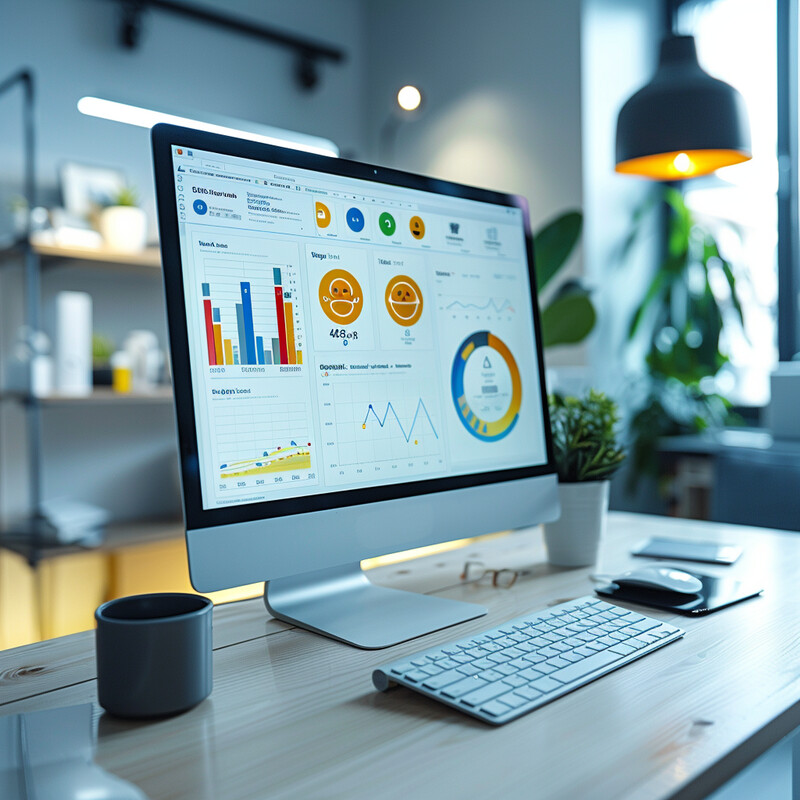
AI employs natural language processing (NLP) to analyze sentiments expressed in social media posts, news articles, and online discussions in real-time. This continuous sentiment tracking allows analysts to detect shifts in public opinion quickly and adjust forecasts accordingly, capturing the dynamic nature of voter preferences.
3. Voter Behavior Prediction
AI uses historical data and current trends to predict voter behaviors, such as turnout and party allegiance, enhancing the accuracy of election predictions.
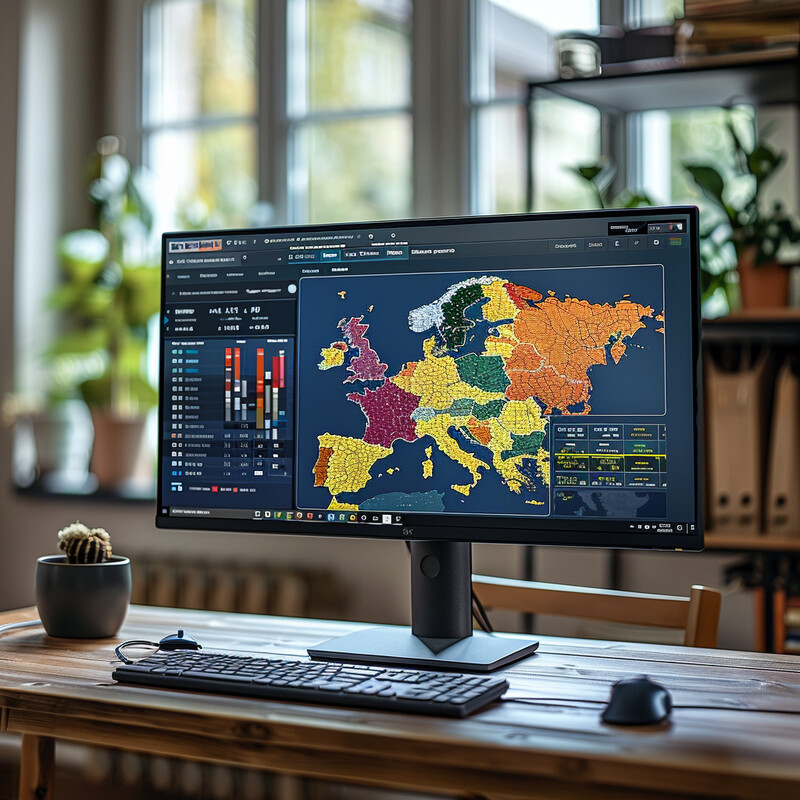
Using machine learning algorithms, AI analyzes patterns in historical voting data and current trends to predict voter behaviors such as turnout rates and party preferences. This predictive capability helps forecasters estimate election outcomes more accurately by understanding how different factors influence voter decisions.
4. Simulation of Electoral Scenarios
AI runs simulations based on various inputs and hypothetical situations to predict potential outcomes, helping strategists and analysts explore different electoral scenarios.
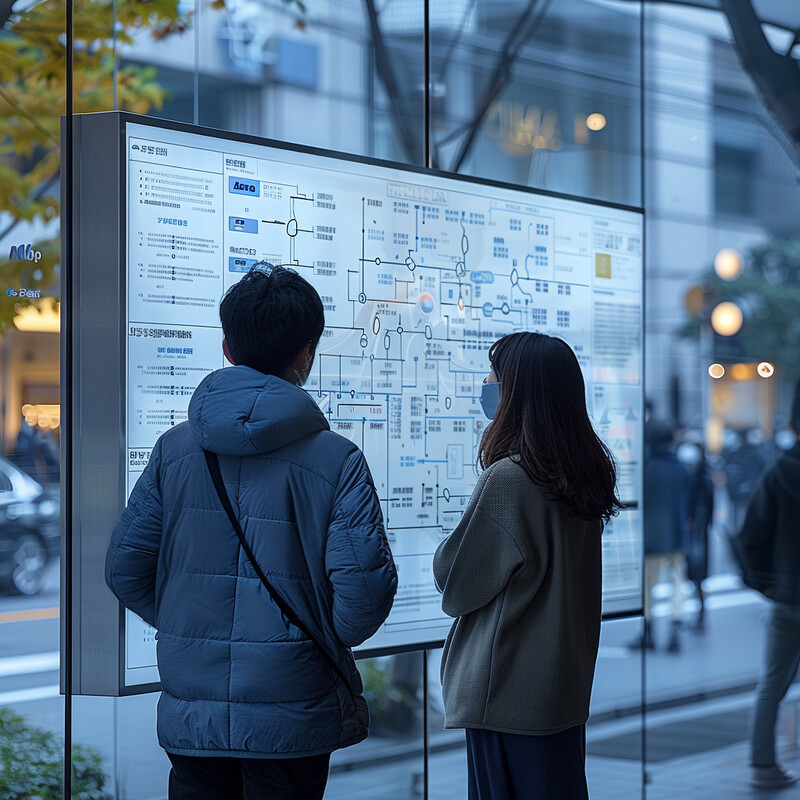
AI can simulate multiple electoral scenarios based on varying inputs such as changes in voter turnout, demographic shifts, or candidate popularity. These simulations allow analysts to explore and prepare for a range of possible outcomes, aiding strategic decision-making in political campaigns.
5. Targeted Poll Analysis
AI enhances poll analysis by identifying biases and weighing polls according to their historical accuracy and methodology, leading to more precise forecasting models.
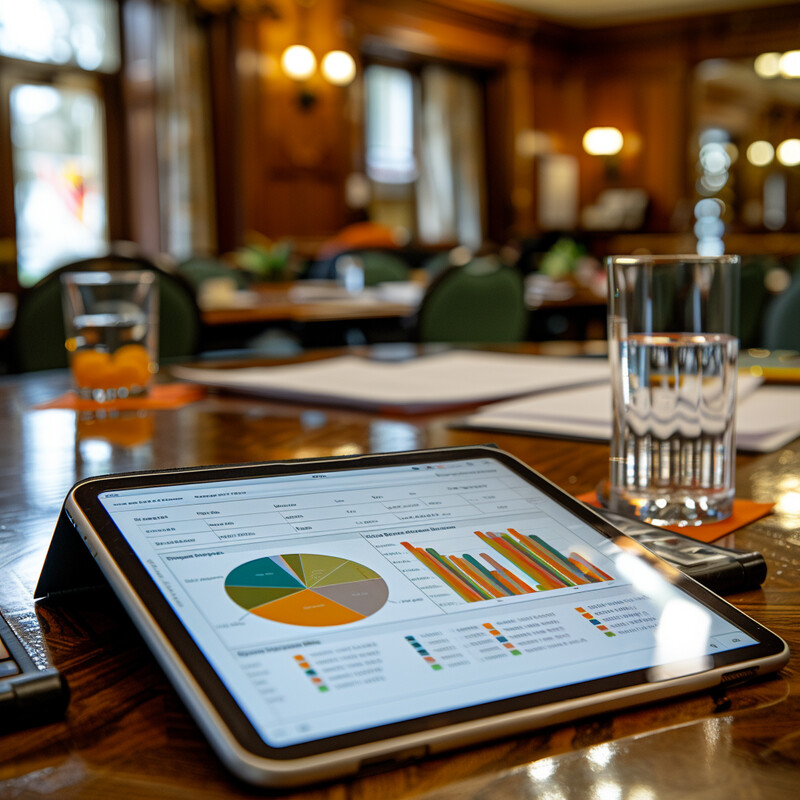
AI improves the reliability of poll-based forecasts by analyzing the methodology, historical accuracy, and potential biases of different polls. It then weights these polls accordingly in the forecasting model, refining the prediction accuracy by emphasizing more reliable sources.
6. Geospatial Analysis
AI incorporates geographic information systems (GIS) to analyze voting patterns by location, highlighting regional trends and potential swing areas crucial for election strategies.
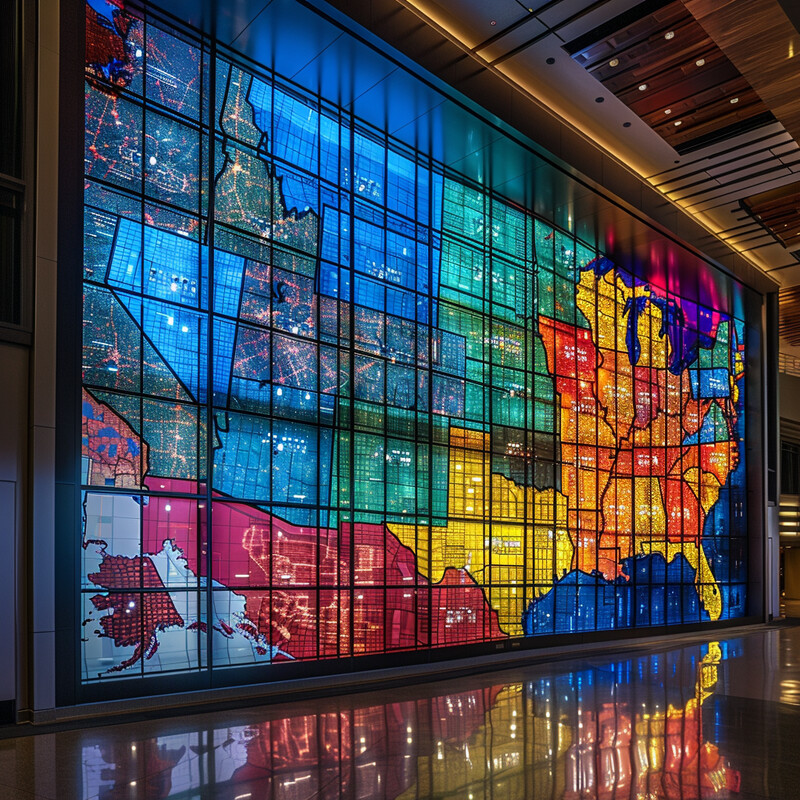
Incorporating geographic data, AI analyzes voting patterns across different regions and districts using geographic information systems (GIS). This geospatial analysis helps identify key battleground areas and regional voting trends, which are critical for targeting campaign efforts and predicting election results.
7. Early Detection of Disinformation Campaigns
AI tools detect and analyze patterns of disinformation, helping to mitigate their impact on voter perception and forecast integrity.
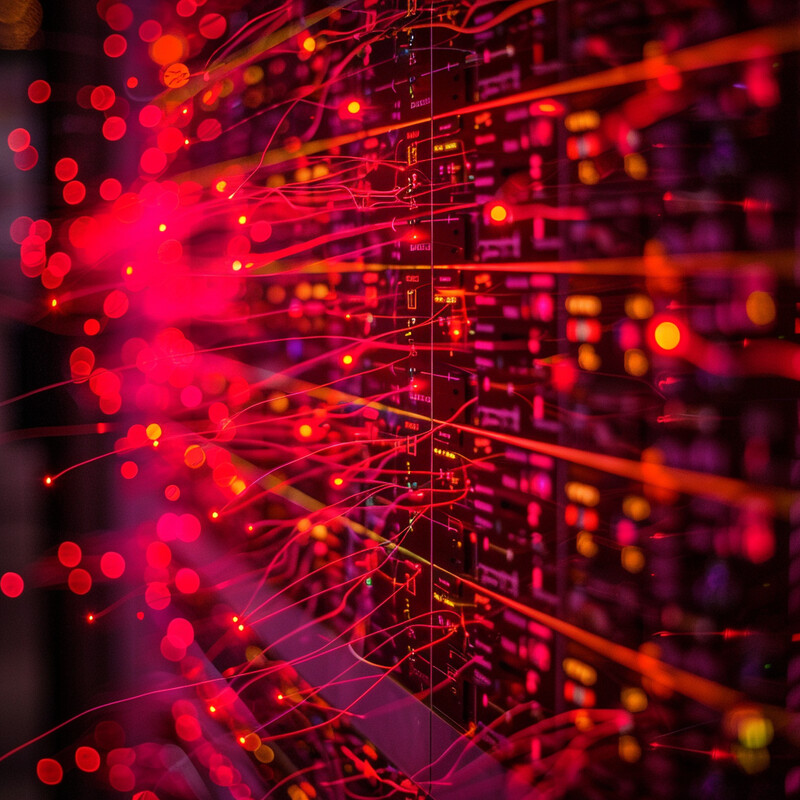
AI algorithms detect and analyze unusual patterns and spikes in online activity that may indicate disinformation campaigns. By identifying these early, AI helps mitigate their potential impact on public opinion and election integrity, ensuring a fairer electoral process.
8. Enhanced Demographic Analysis
AI drills down into demographic data to understand voting trends among specific population segments, improving the understanding of how different groups may influence election outcomes.
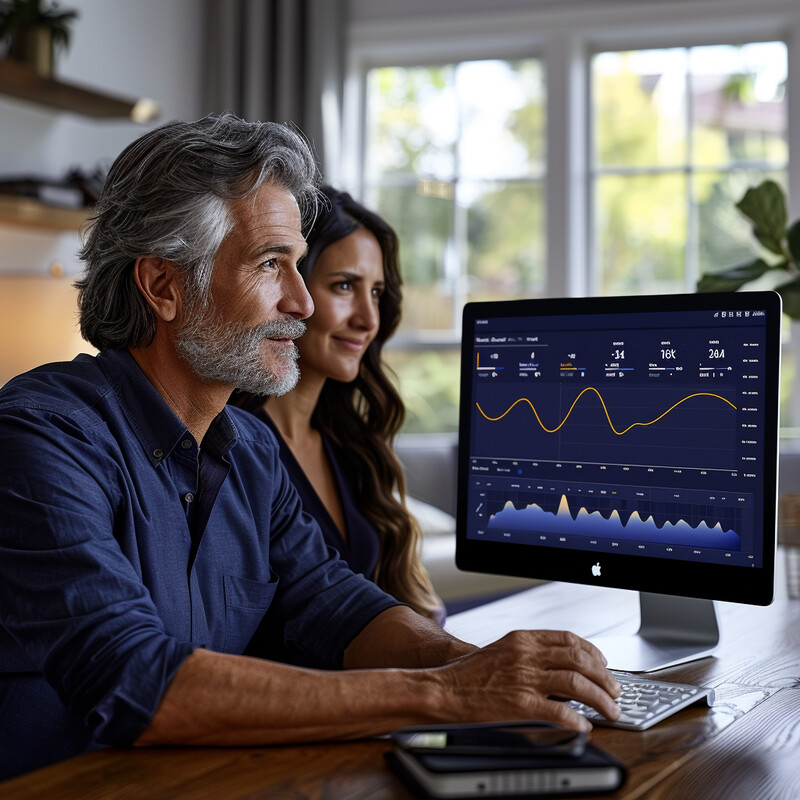
AI drills into demographic data to dissect voting trends among specific groups based on age, ethnicity, income level, education, and other factors. This detailed analysis helps forecasters understand the electoral influence of different demographic segments, improving the granularity of election predictions.
9. Automated Reporting and Visualization
AI automates the creation of visualizations and reports that make complex election data more accessible and understandable to analysts and the public.
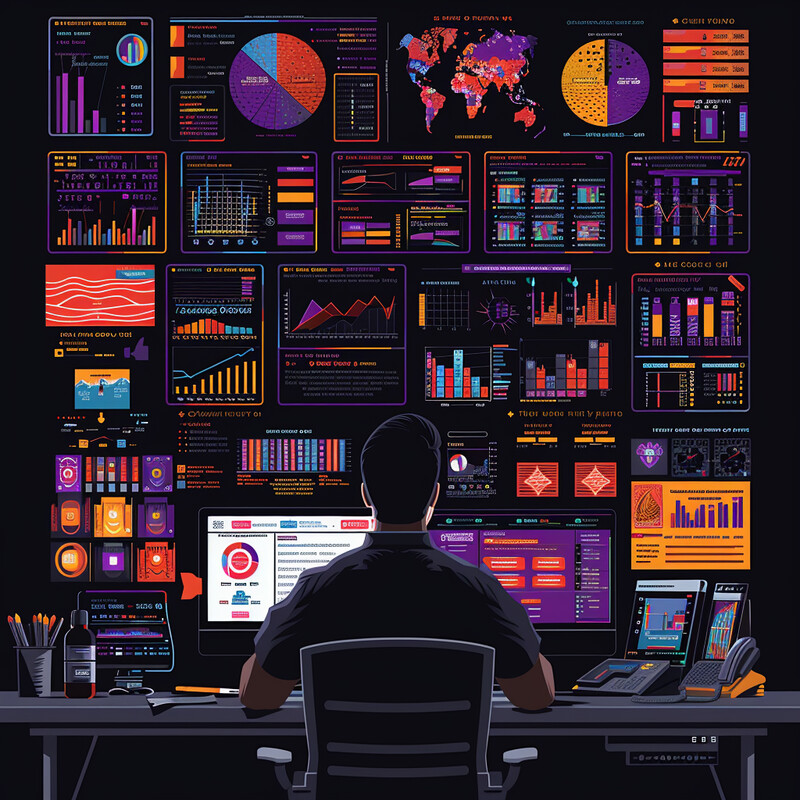
AI automates the generation of data visualizations and detailed reports, transforming complex electoral data into accessible and understandable formats. This automation enhances the communication of forecast insights to campaign strategists, media, and the public, facilitating informed discussion and decision-making.
10. Predictive Analytics for Long-term Trends
AI not only forecasts immediate election outcomes but also analyzes long-term political trends, providing insights into future electoral shifts and policy impacts.
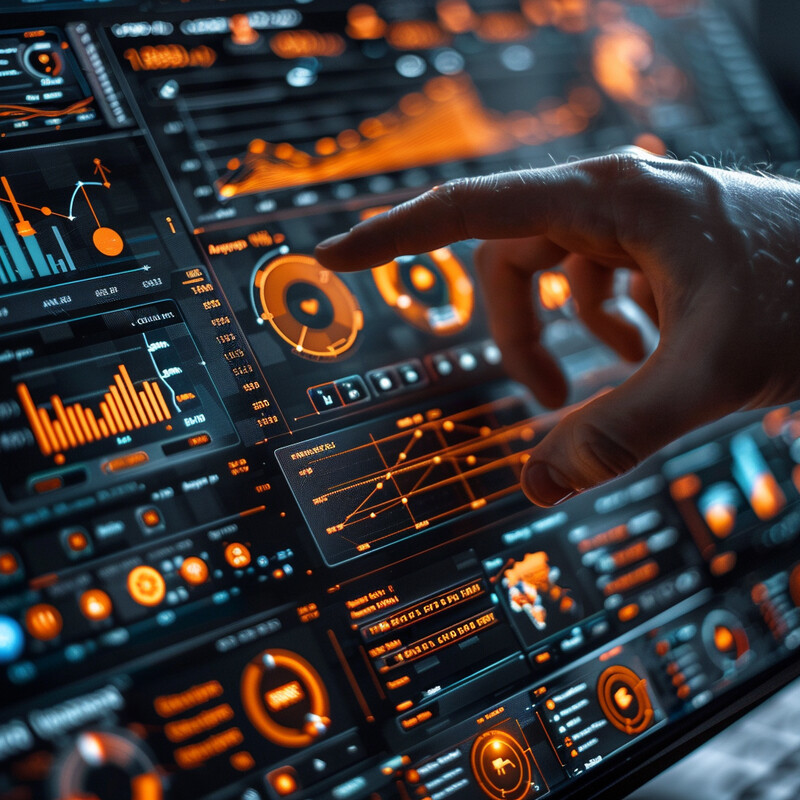
Beyond immediate elections, AI analyzes data over extended periods to identify and predict long-term political trends. This analysis can forecast shifts in political alignments, voter priorities, and policy impacts, providing valuable insights for future electoral strategies and governance planning.