1. Predictive Maintenance
AI algorithms can predict equipment failures before they occur by analyzing data from sensors and historical maintenance records, significantly reducing downtime and maintenance costs.
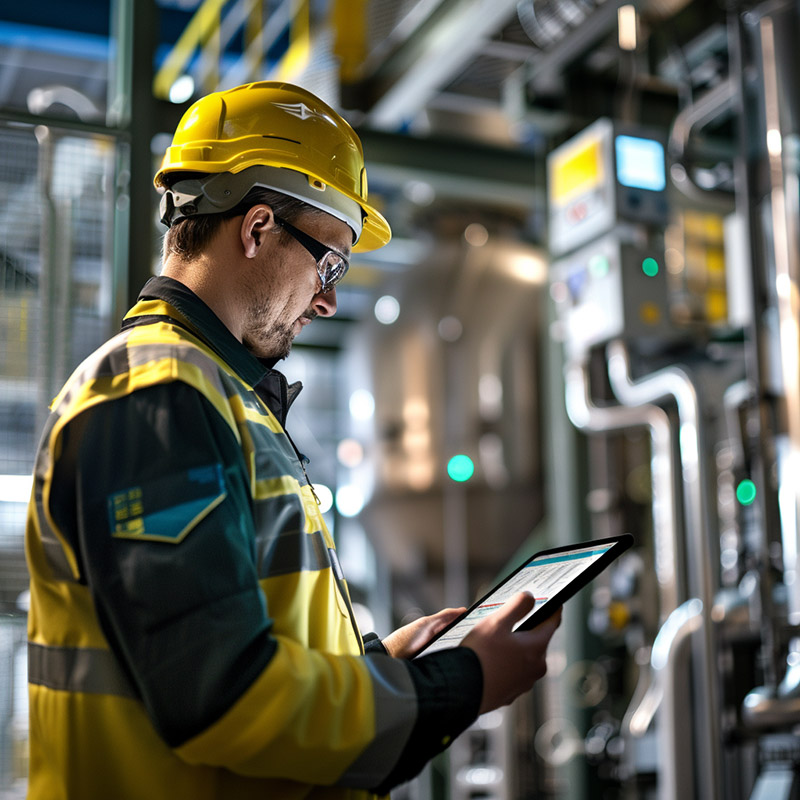
Maintenance Before AI
Traditional maintenance strategies often relied on scheduled maintenance or reacting to machine failures as they occurred. This approach led to unnecessary downtime for maintenance when it might not have been needed, or unexpected breakdowns that could halt production, resulting in lost time and increased costs.
Maintenance After AI
With AI-driven predictive maintenance, manufacturers can analyze data from sensors on machinery to predict failures before they happen. This means maintenance can be scheduled at just the right time, minimizing downtime and extending the lifespan of equipment. The precision of AI algorithms significantly reduces the risk of unexpected breakdowns, improving overall efficiency and reducing maintenance costs.
2. Quality Control Enhancement
AI-powered visual inspection systems can identify defects and quality issues more accurately and much faster than human inspectors, improving product quality and reducing waste.
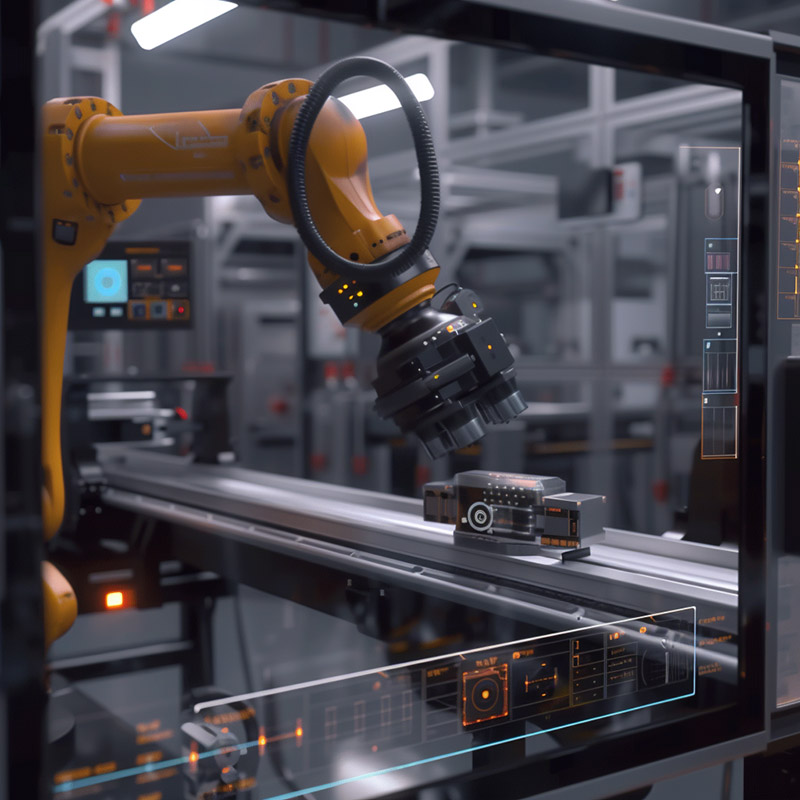
Quality Before AI
Quality control in manufacturing traditionally required manual inspection of products, a time-consuming and error-prone process. Inspectors might miss defects, leading to inconsistent product quality and potential recalls, damaging the brand's reputation and customer trust.
Quality After AI
AI-powered visual inspection systems can consistently and accurately identify even the smallest defects at high speeds. This improves product quality by ensuring defects are caught and addressed early in the production process. The result is a more reliable product output, enhanced brand reputation, and reduced waste and rework costs.
3. Supply Chain Optimization
AI can analyze vast amounts of data to predict supply chain disruptions, optimize inventory levels, and enhance logistics, ensuring materials are available when needed and products are delivered efficiently.
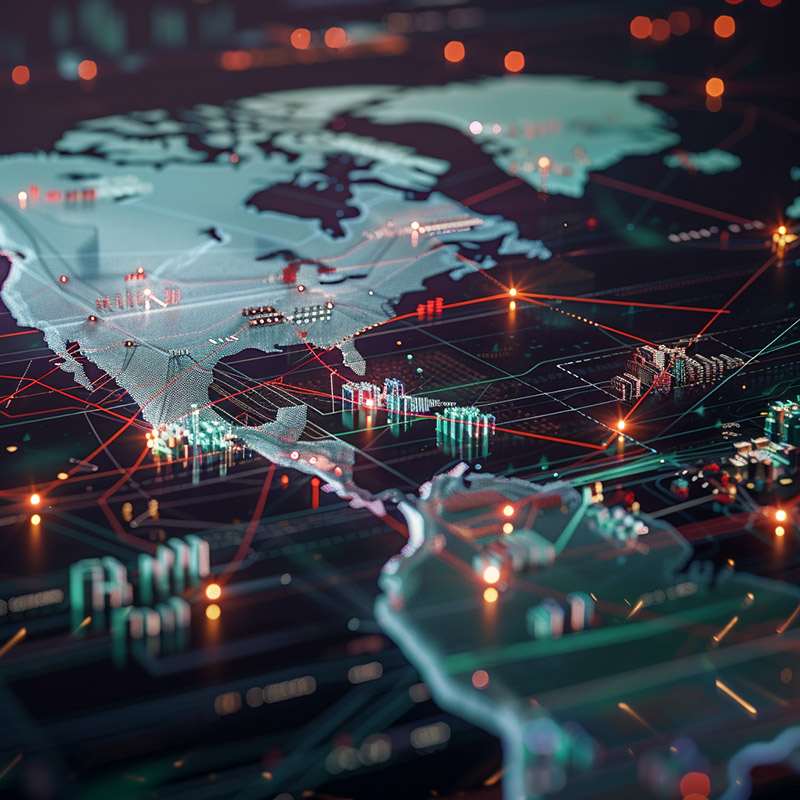
Supply Chain Management Before AI
Managing supply chains was often a complex task prone to inefficiencies due to the reliance on historical data and manual planning. This could lead to overstocking, stockouts, and an inability to quickly respond to disruptions, resulting in lost sales and increased holding costs.
Supply Chain Management After AI
AI enables dynamic supply chain optimization by analyzing real-time data from various sources, including market demand, logistics performance, and supplier status. This allows for more accurate forecasting, inventory management, and responsive logistics planning, significantly reducing costs and improving the ability to meet customer demand promptly.
4. Smart Robotics
Robots equipped with AI can perform complex tasks with high precision, adapt to new tasks through learning, and work safely alongside humans, increasing productivity and flexibility in manufacturing processes.
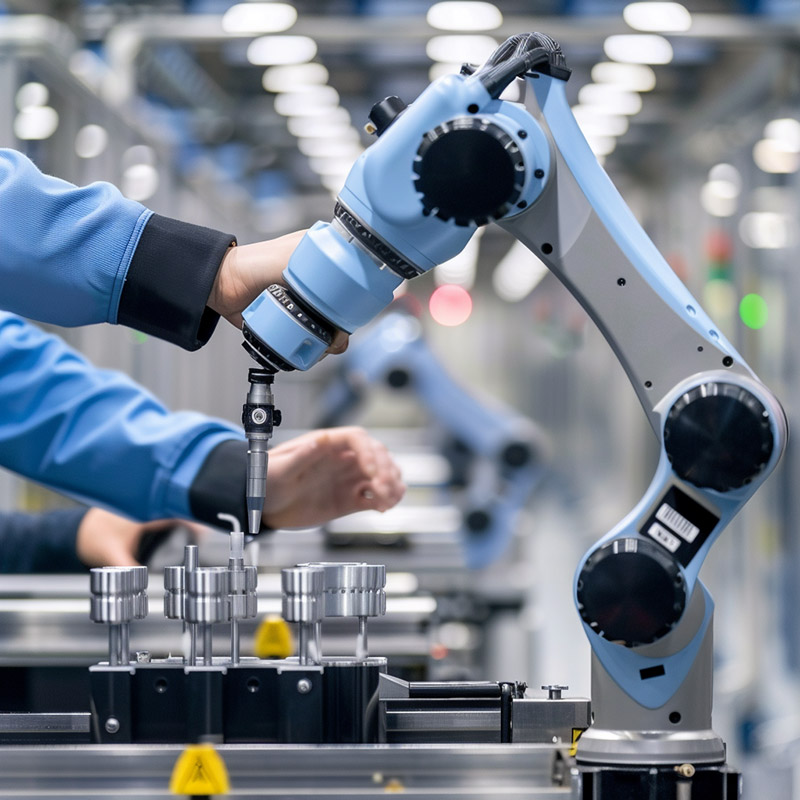
Robotics Before AI
Robots in manufacturing were typically limited to repetitive, predefined tasks, requiring significant reprogramming for new tasks. This limited their flexibility and required human intervention for more complex or variable tasks.
Robotics After AI
AI-infused robotics can learn from their environment and adapt to new tasks through machine learning, increasing their versatility and ability to work collaboratively with humans. This not only boosts productivity but also allows for more complex and creative applications of robotics in manufacturing, from assembly to packaging and quality control.
5. Design and Product Development
AI tools can accelerate the design process by generating and evaluating multiple design options based on specified criteria, leading to innovative products developed in shorter timeframes.
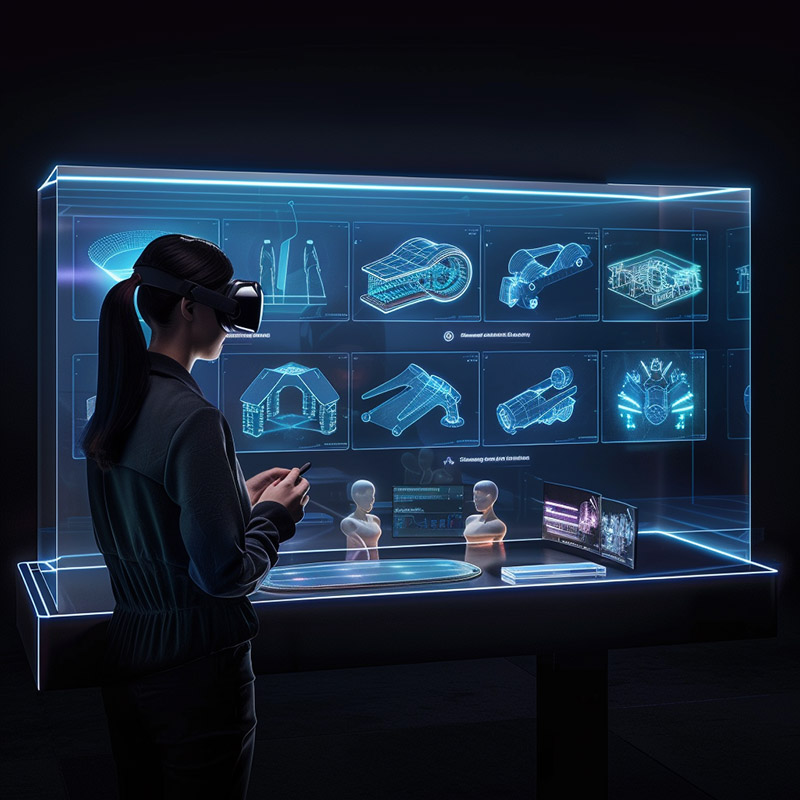
Design Before AI
Product design and development were often lengthy and iterative processes, constrained by human creativity and the manual testing of prototypes. This could delay product launches and lead to missed market opportunities.
Design After AI
AI accelerates the design process by quickly generating and evaluating a wide range of design alternatives based on specified criteria, including functionality, aesthetics, and manufacturability. This enables faster innovation cycles, reducing time-to-market and opening up new possibilities for customized and optimized products.
6. Demand Forecasting
By analyzing market trends, consumer behavior, and other external factors, AI can provide more accurate demand forecasts, helping manufacturers adjust production plans to meet market needs effectively.
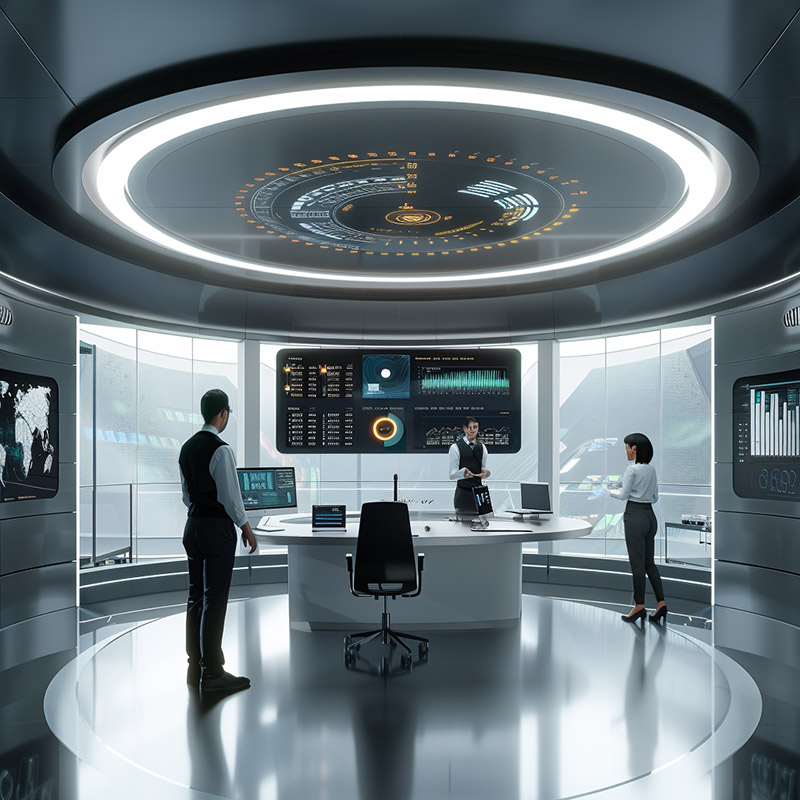
Forecasting Before AI
Forecasting demand was traditionally based on historical sales data, often failing to account for sudden market shifts or trends. This could result in either excess inventory or stock shortages, impacting customer satisfaction and financial performance.
Forecasting After AI
AI algorithms analyze vast datasets, including sales, market trends, and external factors, to provide accurate and timely demand forecasts. This allows manufacturers to adjust production plans proactively, optimizing inventory levels and meeting market demand more effectively.
7. Energy Efficiency
AI systems can monitor and control energy consumption across manufacturing facilities, identifying patterns and anomalies that suggest ways to reduce energy use and cut costs.
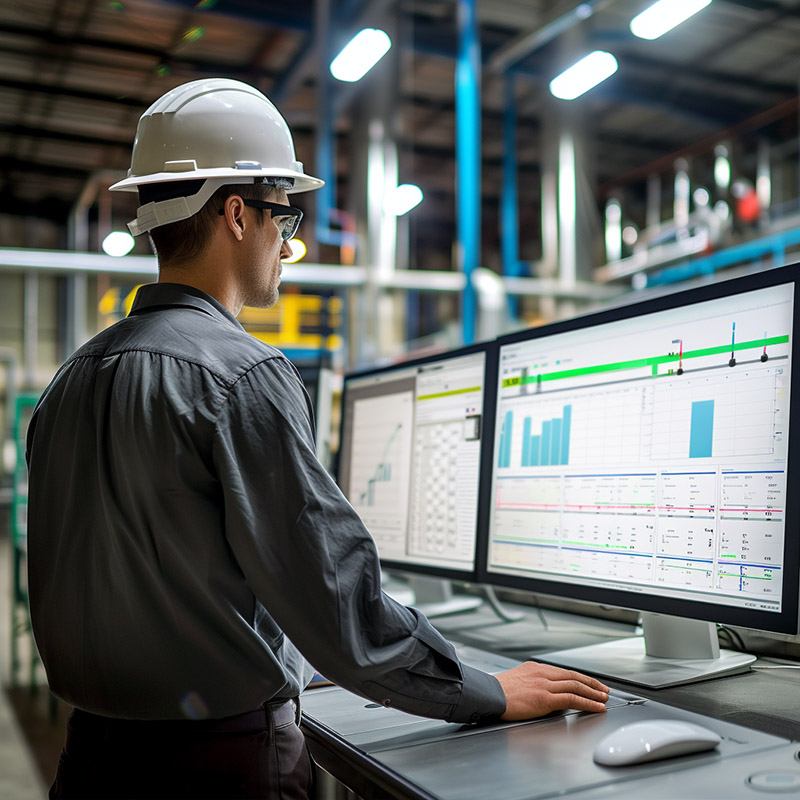
Energy Efficiency Before AI
Energy consumption in manufacturing plants was often monitored manually, if at all, leading to inefficiencies and unnecessarily high operational costs without a clear understanding of how to reduce them.
Energy Efficiency After AI
AI-driven energy management systems continuously monitor and analyze energy usage across a manufacturing facility, identifying inefficiencies and optimizing energy consumption. This not only reduces costs but also contributes to sustainability goals by minimizing the environmental impact of manufacturing operations.
8. Customization and Personalization
AI enables the cost-effective customization of products at scale by optimizing production processes for individualized products, meeting the growing consumer demand for personalized items.
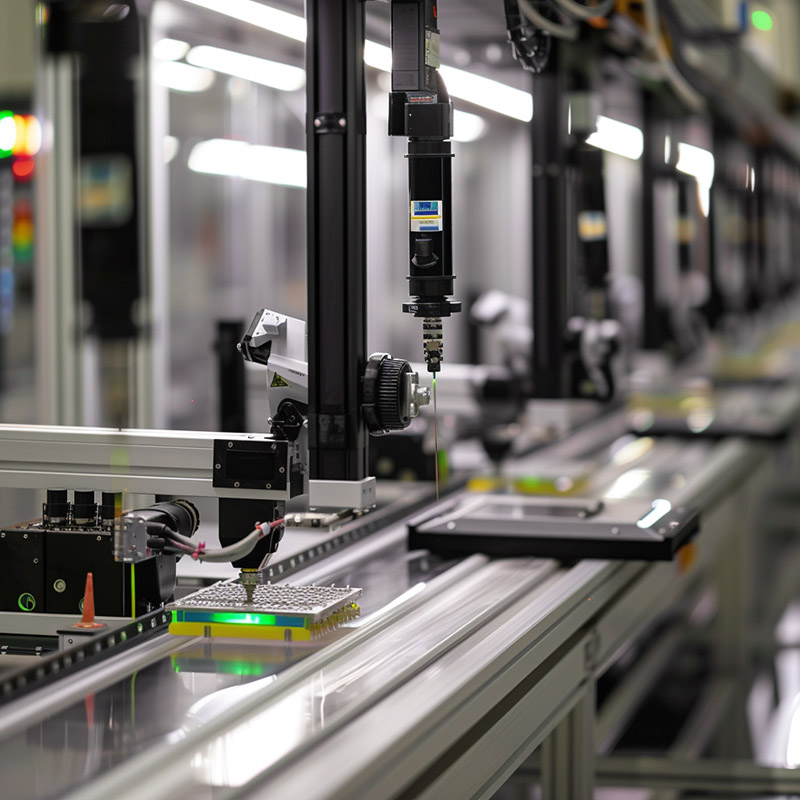
Personalization Before AI
Producing customized or personalized products was typically costly and time-consuming, limiting the ability of manufacturers to offer such options without significant markups.
Personalization After AI
AI enables efficient customization at scale by optimizing production processes for individualized orders. Manufacturers can now offer personalized products at competitive prices, meeting customer expectations for uniqueness without compromising on efficiency or cost.
9. Worker Safety and Ergonomics
AI-powered wearables and sensors can monitor the workplace environment and workers' physical condition, predicting potential safety incidents and suggesting improvements to reduce workplace injuries.
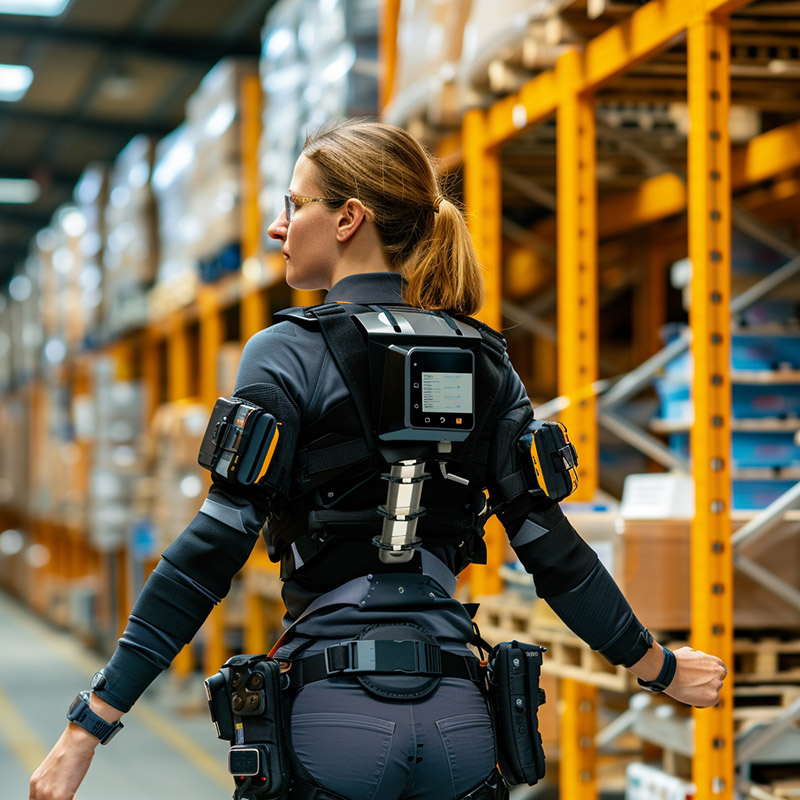
Safety Before AI
Ensuring worker safety and ergonomic working conditions often relied on manual assessments and training, which could overlook potential risks or fail to prevent all workplace injuries.
Safety After AI
AI-powered wearables and environmental sensors provide real-time monitoring of workers' physical condition and the workplace environment, predicting and preventing potential safety incidents before they occur. This proactive approach significantly improves worker safety and health outcomes.
10. Real-time Decision Making
Integrating AI across manufacturing operations allows for real-time analysis and decision-making, enabling managers to respond quickly to production issues, supply chain interruptions, or changes in demand.
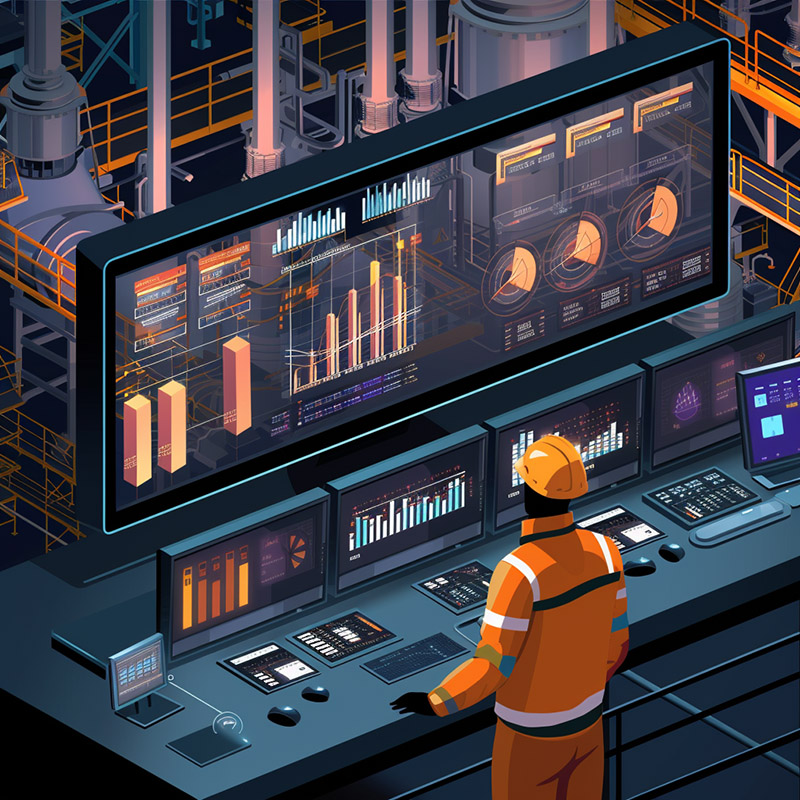
Decision Making Before AI
Decision-making in manufacturing was often reactive, based on periodic reports and historical data. This could delay responses to production issues, supply chain disruptions, or changes in demand, affecting operational efficiency and market responsiveness.
Decision Making After AI
Integrating AI across manufacturing operations enables real-time analysis and decision-making, allowing managers to quickly adjust to new information. This agility improves operational efficiency, reduces waste, and ensures that manufacturing processes can swiftly adapt to changing market conditions or production challenges.