1. Feedstock Composition Prediction
Advanced machine learning models analyze incoming waste streams, predicting moisture content, calorific value, and composition variability, enabling operators to adjust parameters for more efficient combustion.
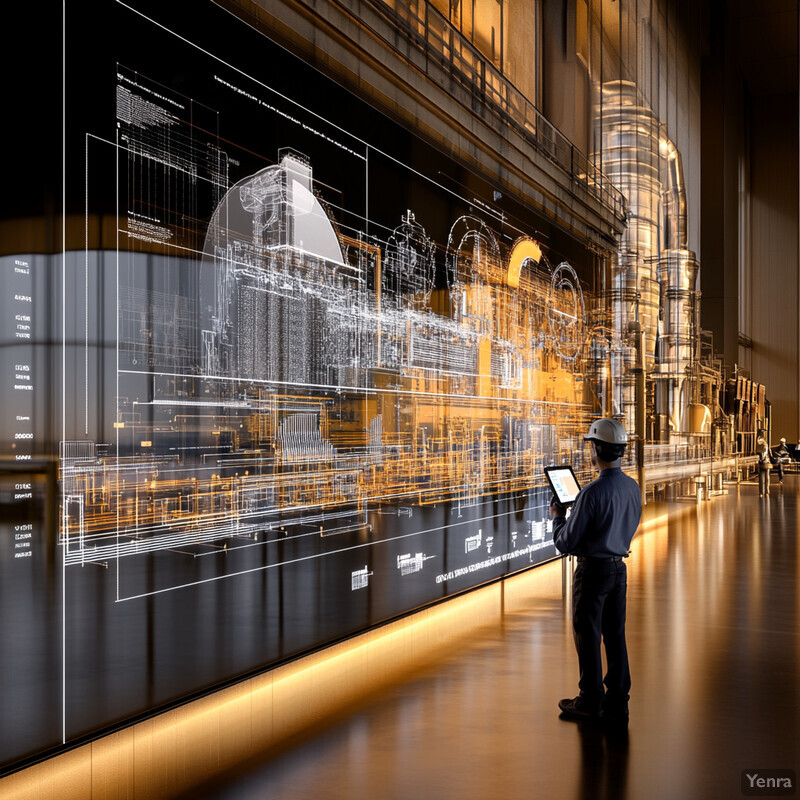
By employing machine learning models trained on historical waste data, sensors, and external factors such as weather conditions, AI systems can accurately predict the physical and chemical composition of incoming waste streams. This includes anticipating variations in moisture content, energy density, and the presence of contaminants. When operators know in advance that a certain batch of waste has unusually high moisture or lower calorific value, they can adjust furnace temperatures, airflow rates, and grate speeds accordingly. Such proactive measures lead to more stable combustion conditions, improved thermal efficiency, and reduced emissions. The result is a smoother and more cost-effective conversion of waste into energy while minimizing operational uncertainties and downtime.
2. Dynamic Fuel Blending
AI tools can determine the ideal mix of various waste types in real time, optimizing burn rates, improving combustion stability, and enhancing overall energy recovery.
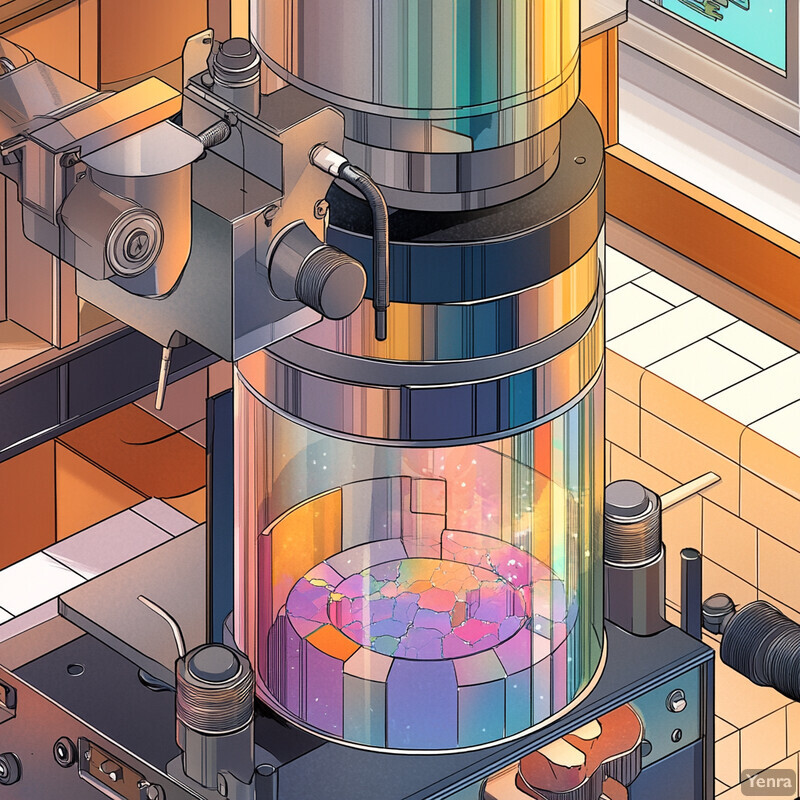
AI-driven optimization platforms can determine the ideal mixture of diverse waste streams in real time, ensuring that the fuel entering the boiler consistently meets energy and quality targets. Since waste composition often varies widely, blending low-calorific-value waste with more energy-dense feedstock optimizes combustion efficiency and heat recovery. These systems account for multiple constraints, including plant throughput, emission limits, and available fuel sources. The result is a balanced and stable combustion process that avoids spikes in emissions, reduces slag formation, and enhances the reliability and performance of the plant’s thermal conversion process.
3. Adaptive Combustion Control
By continuously monitoring key parameters (temperature, oxygen levels, and steam pressure), AI systems adjust grate speed, air flows, and other variables to maintain optimal combustion conditions, reducing fuel use and emissions.
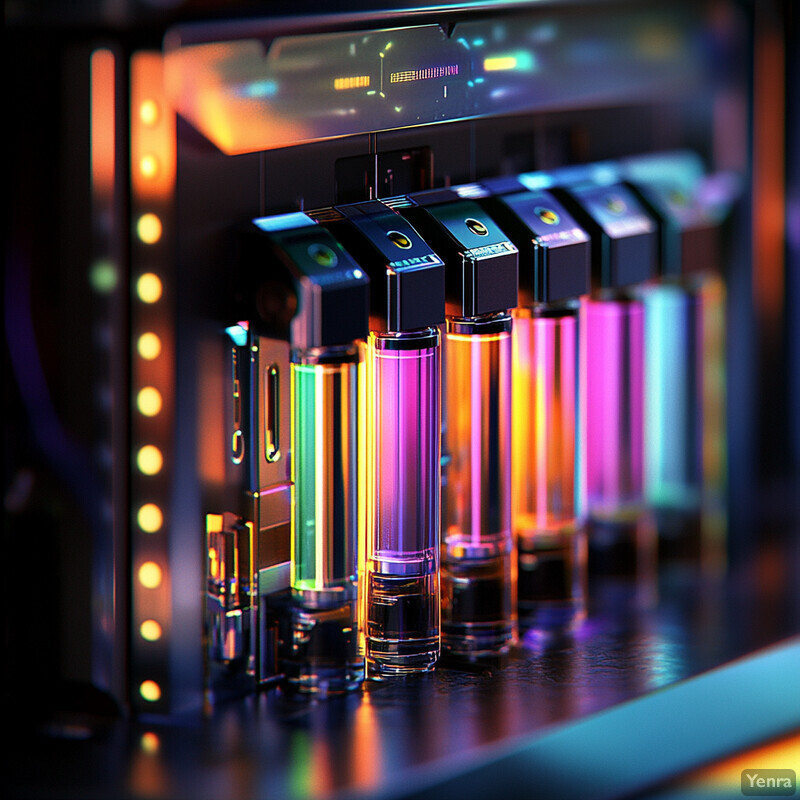
Advanced control algorithms leverage sensor data, including temperature readings, oxygen concentrations, and steam pressures, to regulate combustion in real time. These AI-driven solutions can anticipate changes in waste composition and automatically adjust furnace grate speeds, primary and secondary air supply, and burner configurations. By constantly fine-tuning the combustion parameters, the system maintains optimal flame stability and heat release. This leads to lower fuel consumption, more efficient heat transfer, and fewer harmful byproducts. It also allows for smoother operation with less operator intervention, ensuring a more consistent production of steam and electricity.
4. Real-Time Emissions Monitoring and Control
AI-driven analytics can detect subtle changes in flue gas composition, proactively adjusting operating conditions to maintain compliance with environmental regulations and minimize pollutant output.
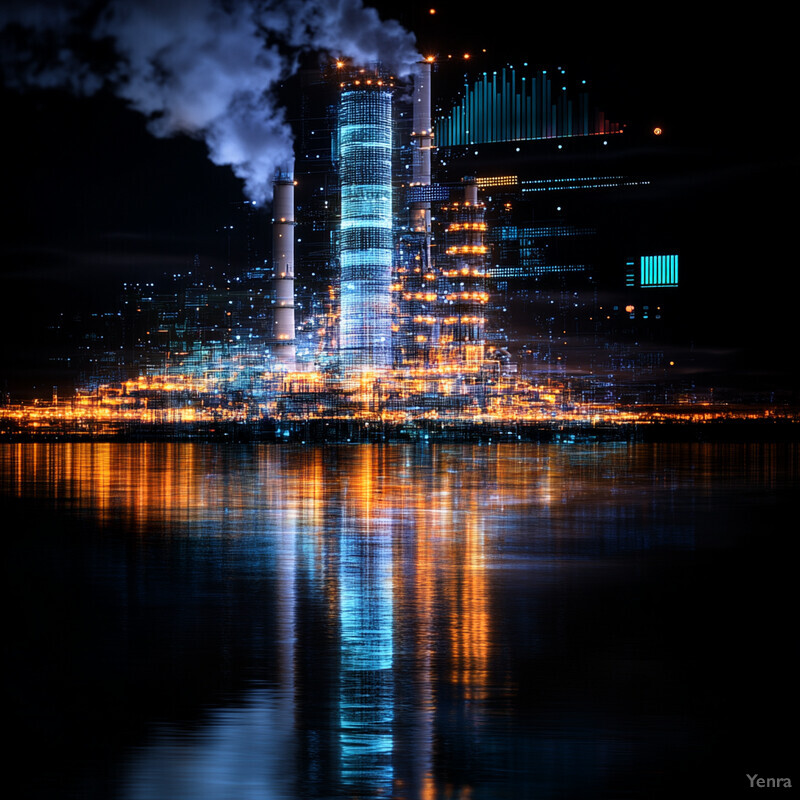
AI-powered analytics platforms integrate data from continuous emissions monitoring systems (CEMS) and other environmental sensors to detect even subtle shifts in flue gas composition. By correlating these findings with operational data—such as combustion temperature or fuel feed rates—the system can identify the root causes of any pollutant spikes. With this information, operators can proactively tweak process parameters, introduce or reduce reagent dosing in the flue gas treatment system, or adjust combustion conditions to minimize emissions. This ensures ongoing compliance with regulatory limits, protects the plant’s environmental reputation, and reduces the risk of costly fines or shutdowns.
5. Predictive Maintenance and Equipment Health Monitoring
Machine learning models can forecast equipment failures or performance degradation by analyzing vibrations, temperature trends, and historical operational data, ensuring timely maintenance and reducing unplanned downtimes.
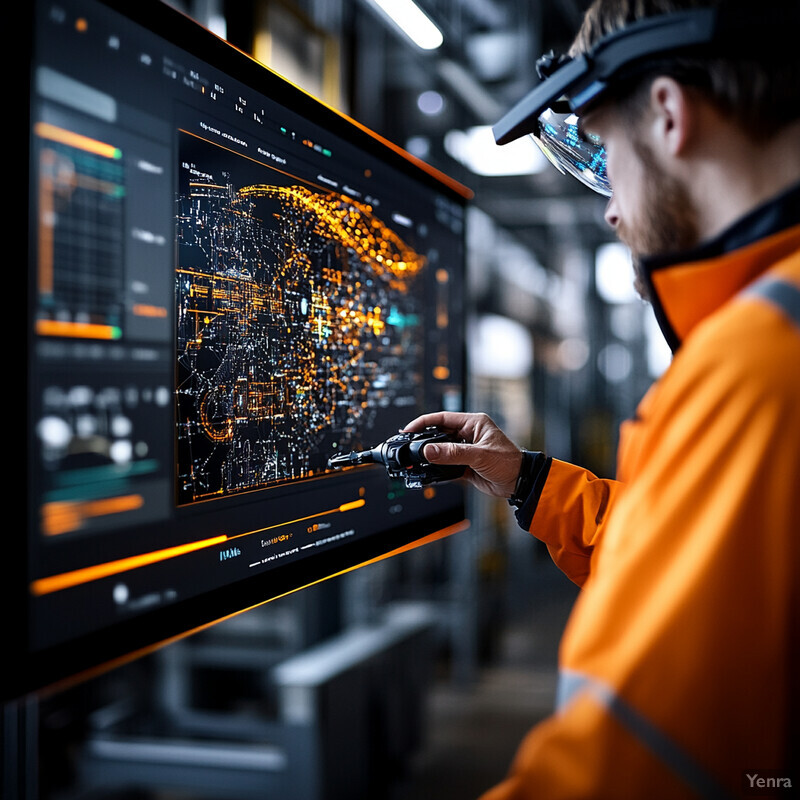
Utilizing sensor data on equipment vibrations, acoustic signatures, lubrication quality, and temperature profiles, AI-based maintenance platforms can predict when critical machinery—like turbines, boilers, or conveyors—is likely to fail or experience performance degradation. By establishing patterns from historical data, these models trigger early alerts for maintenance crews, allowing for planned interventions before breakdowns occur. This proactive approach reduces unplanned downtime, extends equipment life, lowers maintenance costs, and ensures that the plant runs at peak efficiency for longer periods. It also helps maintain consistent energy production and revenue streams.
6. Intelligent Ash Management
AI can predict ash composition, helping operators tweak furnace conditions to improve ash quality and recovery rates of valuable metals, reducing waste disposal costs and generating additional revenue streams.
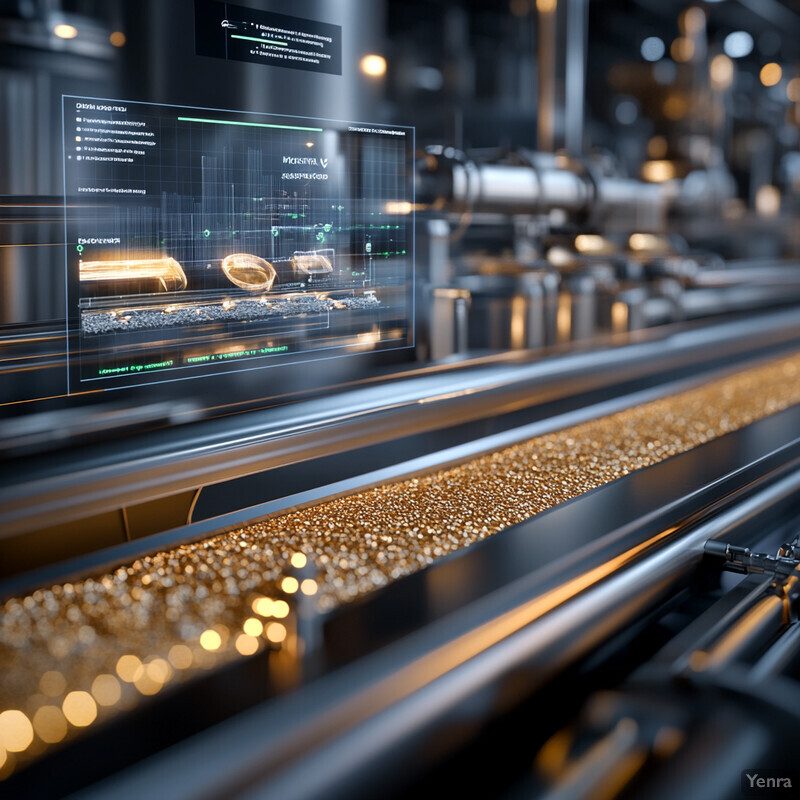
The leftover ash from combustion processes can contain valuable metals and other materials worth recovering. AI algorithms analyze operational data, chemical samples, and process parameters to predict ash composition and identify the optimal operating conditions for improving ash quality. By adjusting furnace temperatures, fuel blend ratios, and other variables, the plant can maximize the extraction of valuable byproducts and reduce the volume of non-recyclable ash sent to landfills. This not only creates a secondary revenue stream but also contributes to the circular economy and enhances the overall sustainability of waste-to-energy operations.
7. Automated Scheduling for Throughput Optimization
AI-driven scheduling algorithms can balance incoming waste deliveries with plant throughput capacity, ensuring continuous and steady feedstock availability without bottlenecks.
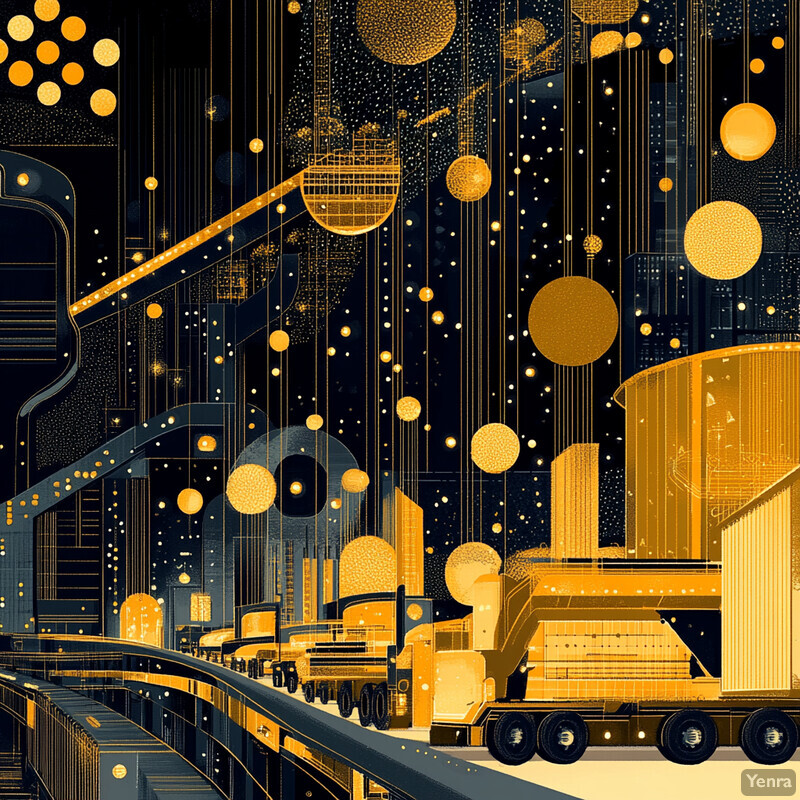
Ensuring that the right amounts of waste are delivered, stored, and processed at the right times is a complex logistical challenge. AI scheduling algorithms consider factors such as transportation schedules, seasonal fluctuations in waste generation, and real-time plant capacity to streamline intake and processing. By balancing incoming waste volume against the plant’s combustion rate, they prevent feedstock shortages or surpluses. This steady feeding of waste into the system helps maintain optimal thermal conditions, reduces the risk of downtime due to fuel shortages, and ensures more predictable, uninterrupted energy output.
8. Enhanced Boiler and Turbine Efficiency
Data-driven insights help fine-tune boiler feedwater temperatures and turbine load distributions, improving thermal efficiency and prolonging component life.
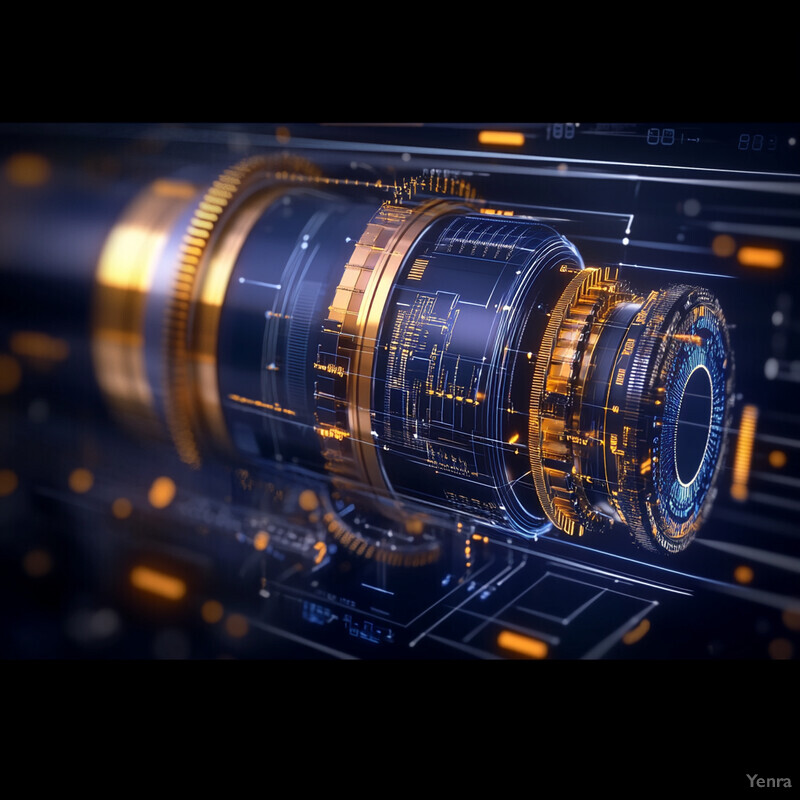
Boilers and turbines operate best within specific temperature, pressure, and flow ranges. AI-driven analytics help fine-tune these conditions by correlating thousands of operational variables with performance metrics. For instance, the system might recommend adjustments to feedwater temperature, optimize steam pressures, or modulate turbine load distributions to squeeze out extra efficiency. Over time, these incremental improvements compound, leading to higher overall plant efficiency, reduced fuel consumption per unit of energy produced, and extended component lifespans by avoiding stress and wear caused by suboptimal operations.
9. Demand Forecasting and Load Management
Integrating AI with energy market data and grid conditions allows WTE plants to ramp up or down production to match market demand and peak electricity prices, maximizing revenue.
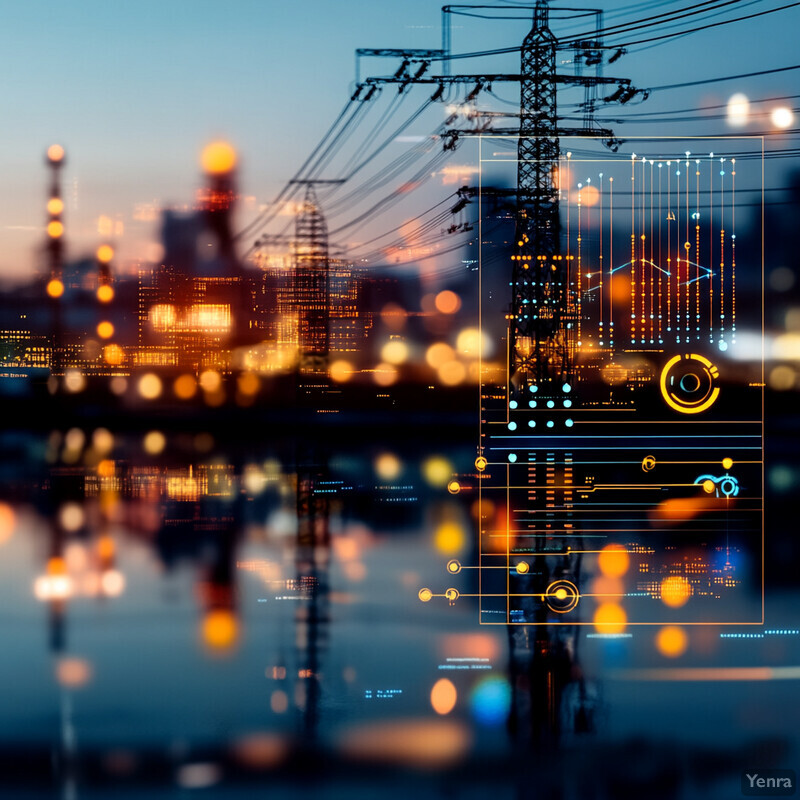
AI models can integrate data from electricity markets, weather patterns, and grid conditions to predict short-term and long-term energy demand. Using these insights, a waste-to-energy plant can plan its output more effectively, timing production to coincide with peak energy prices or adjusting generation during periods of low demand. This flexible approach maximizes revenue while reducing stress on the grid. In addition, the plant can harmonize its generation with intermittent renewable sources, contributing to more stable and resilient energy systems.
10. Continuous Process Tuning via Digital Twins
Digital twin simulations, powered by AI models, can test process changes virtually before implementation, helping engineers optimize operating parameters and reduce trial-and-error in the physical system.
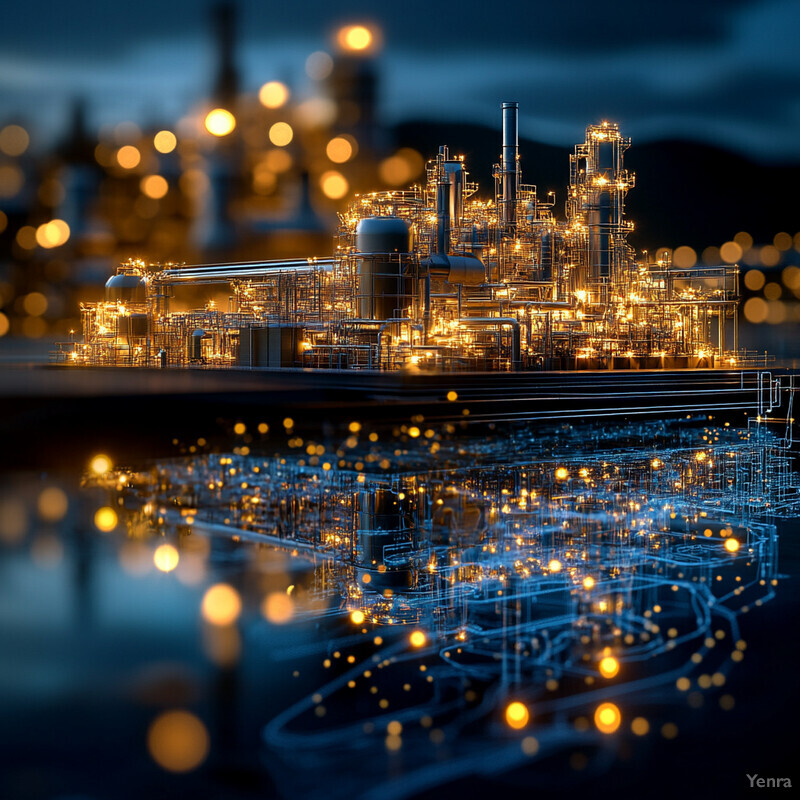
A digital twin is a virtual replica of the plant’s physical systems, updated continuously with real-time operational data. AI-driven simulations run within this digital environment to test the effects of process adjustments—such as altering combustion parameters or upgrading a component—before implementing changes in the real plant. This “what-if” analysis allows engineers to identify the best operational parameters, reduce trial-and-error experimentation, and minimize the risk of negative consequences. The digital twin accelerates innovation, improves decision-making, and leads to more efficient, reliable operations.
11. Optimal Use of Auxiliary Fuels
When needed, supplementary fuels can be added more intelligently, guided by AI models that predict when and how much auxiliary fuel improves stability or boosts output, while minimizing excess costs.
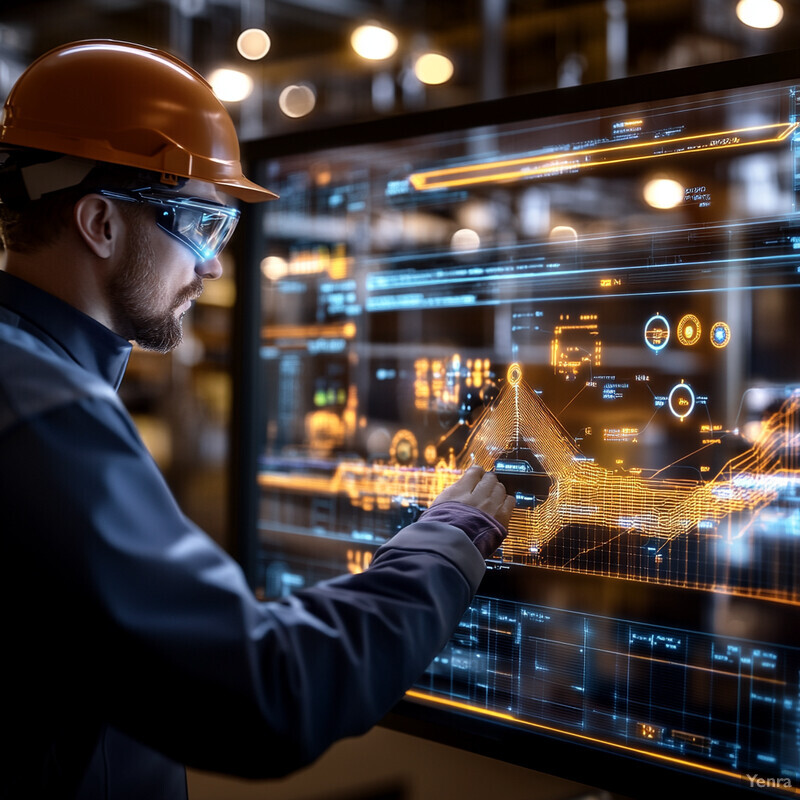
Sometimes, to maintain stable combustion or achieve desired temperatures, auxiliary fuels like natural gas or fuel oil may be introduced. AI systems can predict when and how much auxiliary fuel is needed based on real-time feedstock analysis and operational metrics. By using auxiliary fuels more judiciously, plants reduce unnecessary costs, maintain stable heat and power output during challenging feedstock conditions, and limit over-reliance on fossil fuels. This approach supports operational resilience, energy cost reduction, and continuous compliance with environmental standards.
12. Refined Waste Sorting and Pre-Treatment
AI-driven vision systems and robotic sorters help ensure that feedstock is free of non-combustible contaminants, improving overall plant efficiency and protecting processing equipment.
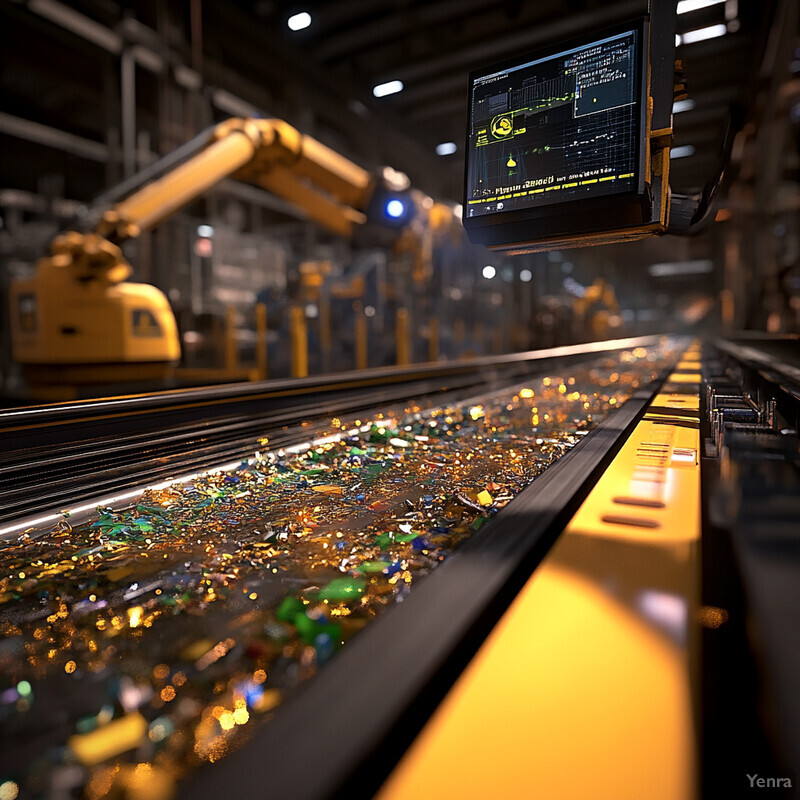
Before waste enters the furnace, it must be free of large non-combustible items and ideally uniform in particle size and composition. AI-driven vision systems and robotic sorters powered by advanced image recognition can detect and remove unwanted materials, such as metals, glass, or large plastics. By providing consistently suitable fuel, these systems improve combustion efficiency, reduce damage and wear to equipment, and ensure a safer working environment. They also enhance the recovery of recyclables, further improving the plant’s environmental and economic performance.
13. Supply Chain and Logistics Optimization
AI tools can optimize waste collection routes, scheduling, and inventories, ensuring that the right quantity and quality of waste arrives at just the right time to the WTE plant.
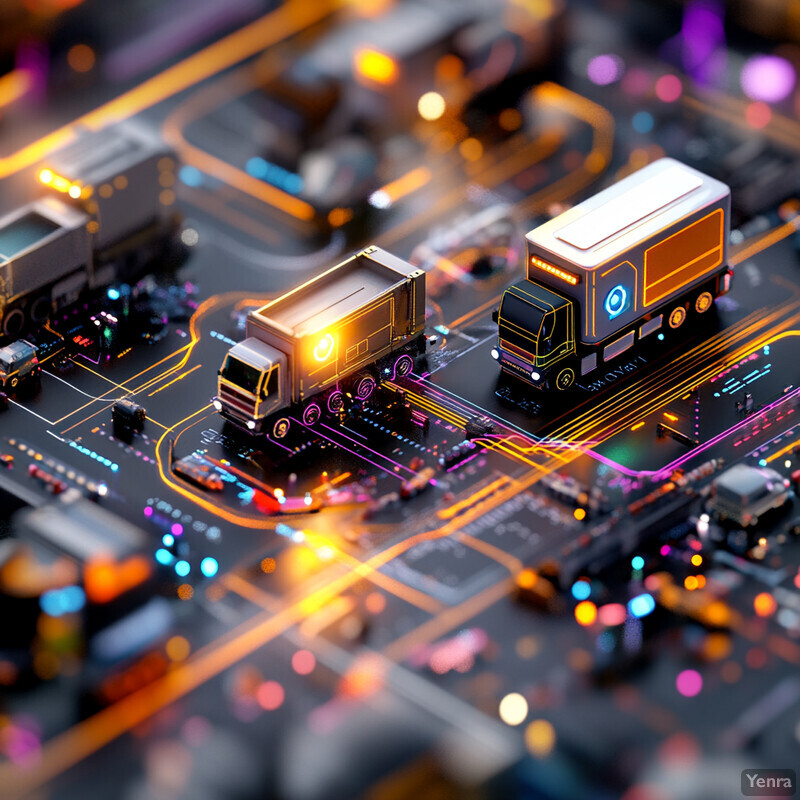
Waste-to-energy plants rely on a steady supply of waste from multiple sources, including municipal collection routes and industrial facilities. AI-based logistics platforms can optimize collection schedules, transportation routes, and transfer station operations. By ensuring timely and cost-effective delivery of fuel to the plant, these tools minimize delays, reduce transportation costs, and help maintain optimal inventory levels. The outcome is a more streamlined supply chain that supports consistent energy production and reduced operational expenditure.
14. Resource Recovery Strategies
By analyzing operational and sensor data, AI can suggest process modifications that improve the recovery of metals, inert materials, and other recyclables from combustion residues, adding to plant revenue streams.
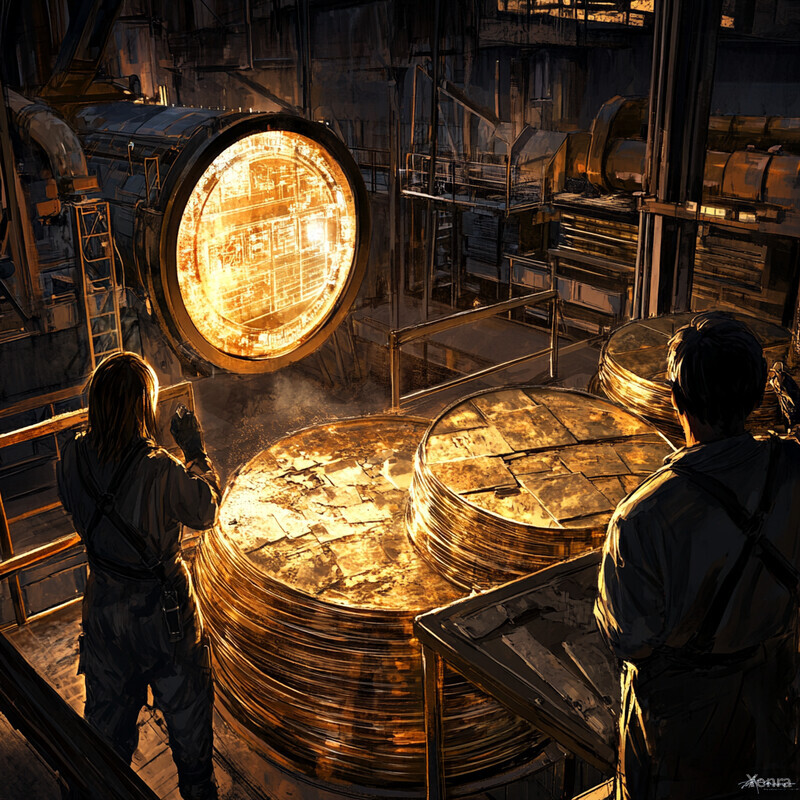
Beyond generating energy, waste-to-energy plants can also recover valuable materials from combustion residues. AI systems analyze operational data and residue characteristics to find the ideal conditions for extracting metals, minerals, and other reusable materials. By systematically adjusting combustion parameters, ash handling processes, and separation techniques, operators can enhance material recovery rates. This not only creates an additional income stream but also reduces the volume of final waste, contributing to better environmental stewardship and a circular economy approach.
15. Odor and Pollution Mitigation
Predictive models can identify operating conditions that lead to odor or off-gas spikes, allowing operators to intervene early with better air treatment strategies and adjustments in waste handling.
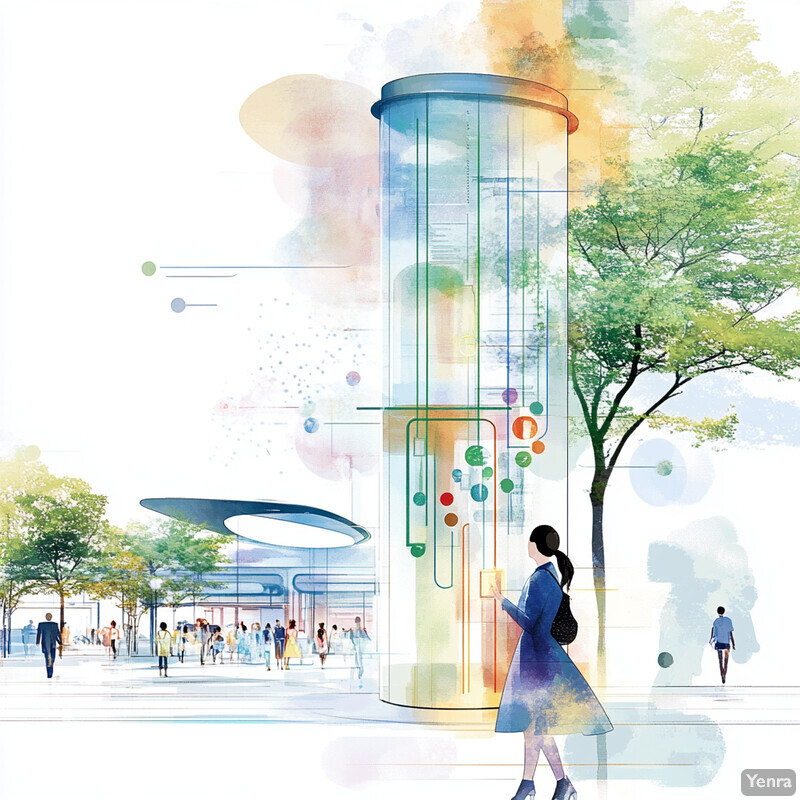
Waste handling areas and certain process steps can produce unpleasant odors or localized emissions. AI-powered predictive models analyze factors like waste composition, storage conditions, and ventilation rates to forecast when odor or emissions spikes might occur. Operators can then adjust air handling systems, alter waste loading practices, or schedule maintenance tasks to head off such problems. By mitigating odor and pollution issues proactively, plants improve their relationship with surrounding communities, avoid regulatory penalties, and maintain a healthier work environment.
16. Adaptive Control of Flue Gas Cleaning Systems
AI-enabled controllers can dynamically adjust scrubber reagent dosing, filter parameters, and other flue gas cleaning steps, reducing chemical use and costs while ensuring emissions remain below regulatory limits.
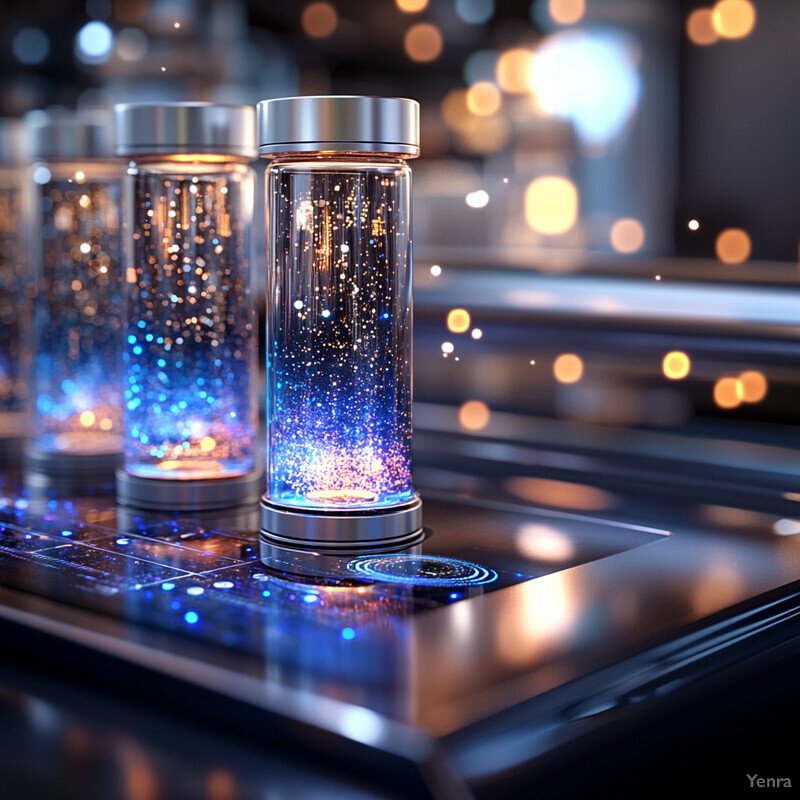
Flue gas treatment involves multiple steps, such as dosing reagents to neutralize acidic gases, filtering out particulates, and scrubbing pollutants. AI controllers dynamically calibrate these steps based on real-time emissions readings, fuel quality, and process conditions. By automatically fine-tuning reagent injection or adjusting filter configurations, the system reduces chemical consumption and operational costs while still meeting or exceeding regulatory thresholds. This responsive, data-driven approach ensures that environmental safeguards remain effective without unnecessary waste of resources.
17. Multi-Objective Optimization (Cost, Efficiency, & Compliance)
Advanced algorithms can simultaneously consider multiple goals—such as minimizing costs, maximizing energy output, and adhering to environmental standards—identifying the best trade-offs in real time.
-0.jpg)
Waste-to-energy plants must juggle numerous goals, such as maximizing efficiency, minimizing costs, and adhering to emissions standards. AI-based optimization algorithms consider all these factors simultaneously, providing balanced operational strategies that do not compromise one objective at the expense of another. By quantifying the trade-offs between efficiency, cost, and environmental compliance, the plant’s operators can make well-informed decisions that improve overall performance. This holistic approach leads to more sustainable operations and long-term viability in a changing energy landscape.
18. Process Anomaly Detection and Root Cause Analysis
AI models can detect subtle deviations from normal plant operation and identify root causes, enabling quick corrective actions that restore optimal conditions and prevent extended downtime.
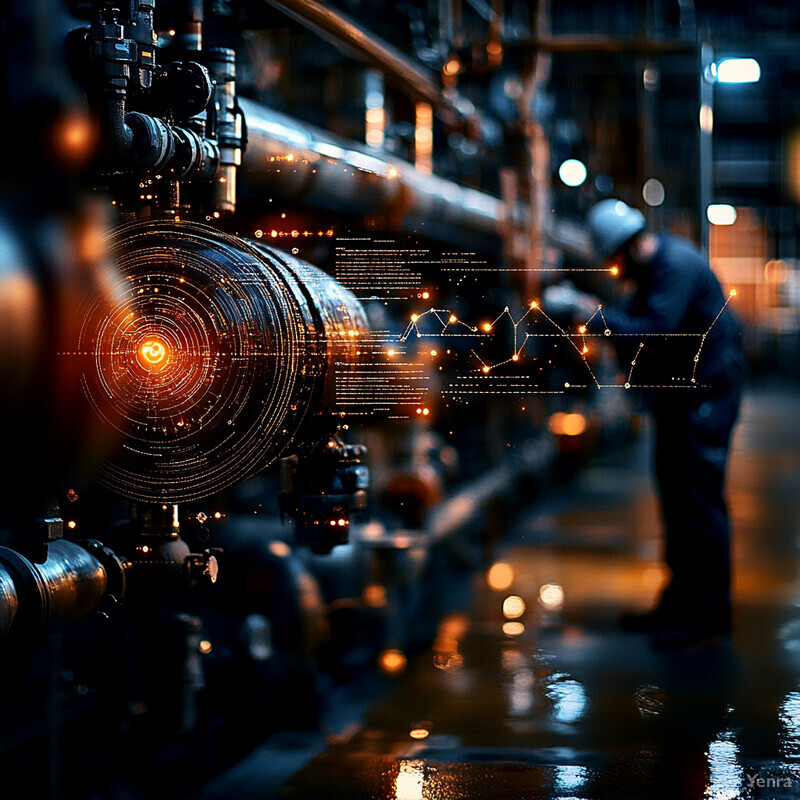
Complex mechanical and thermal processes often experience small deviations that can, if unchecked, lead to major operational issues. AI-powered anomaly detection tools continuously monitor thousands of sensor signals, flagging unusual patterns or outliers before they escalate. When an anomaly is identified, the system uses historical data and machine learning techniques to suggest likely root causes. This rapid, automated diagnostic capability helps maintenance teams address problems more quickly, preventing extended downtime, mitigating equipment damage, and maintaining stable, efficient energy production.
19. Energy Storage Integration
With AI’s forecasting capabilities, plants can integrate batteries or thermal storage more effectively, ensuring stable energy delivery and improved grid balancing during fluctuating supply and demand scenarios.
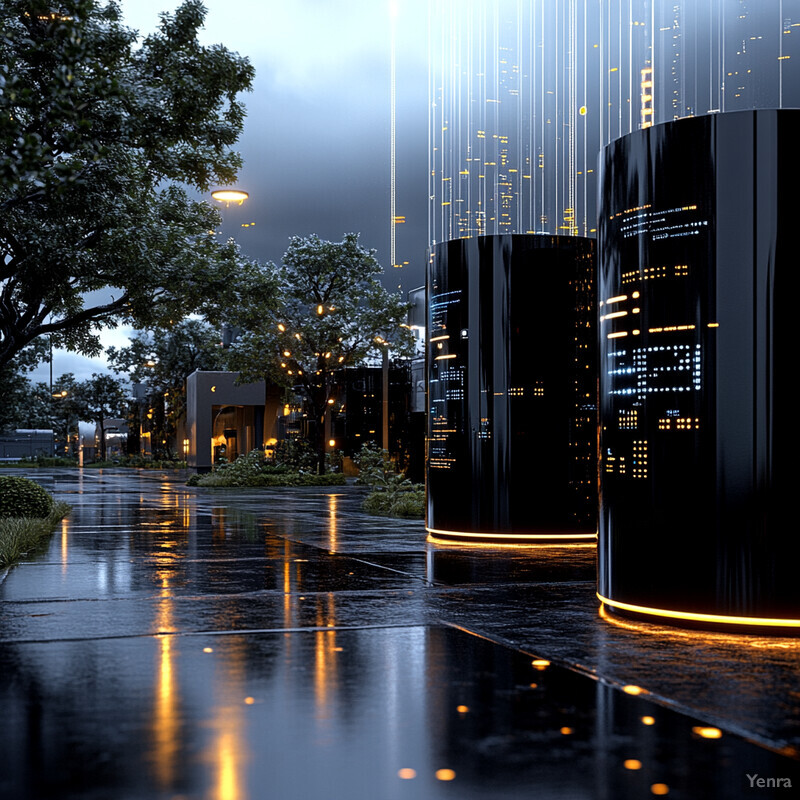
As renewable energy sources like solar and wind integrate into the grid, supply volatility becomes a concern. AI forecasting models that predict waste feedstock variability, plant output, and grid conditions can guide when and how to use on-site energy storage, such as batteries or thermal storage systems. By optimally charging or discharging these storage assets, the plant can stabilize its energy supply, respond to peak demand pricing, and provide ancillary services like frequency regulation. This flexibility enhances the plant’s profitability, reliability, and value to the overall energy system.
20. Long-Term Strategic Planning
Over time, AI-driven analytics incorporate historical data, evolving market conditions, and future emission standards to guide long-term investment decisions, capacity expansions, and technology upgrades for sustained competitiveness.
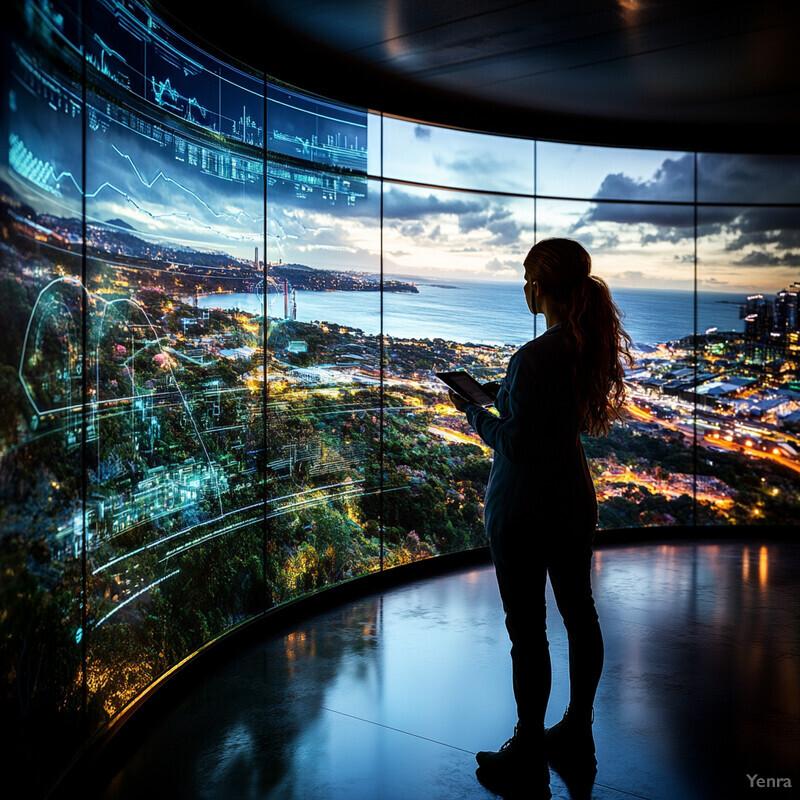
Over time, changes in regulations, market demands, technology advancements, and waste streams can affect the viability of a waste-to-energy plant. AI-driven forecasting tools and scenario planning models help decision-makers anticipate these changes and adjust strategies accordingly. By analyzing historical trends, potential regulatory shifts, evolving waste compositions, and future energy market conditions, the system guides long-term investments in equipment upgrades, capacity expansions, and new technologies. This strategic foresight ensures that the plant remains competitive, profitable, and aligned with environmental and societal goals for years to come.