1. AI-Driven Layout Optimization
AI-driven layout design uses advanced algorithms to configure warehouse floor plans for maximum efficiency. By analyzing data on product dimensions, demand patterns, and operational constraints, AI can propose layouts that minimize travel distances and eliminate wasted space. This dynamic approach continually adapts the warehouse’s layout as inventory and demand change, ensuring that every aisle and shelf is utilized optimally. The result is a floor plan that supports faster picking and throughput while deferring the need for costly facility expansions. Overall, AI layout optimization transforms what was once a static, experience-driven task into a continuous improvement process grounded in data.
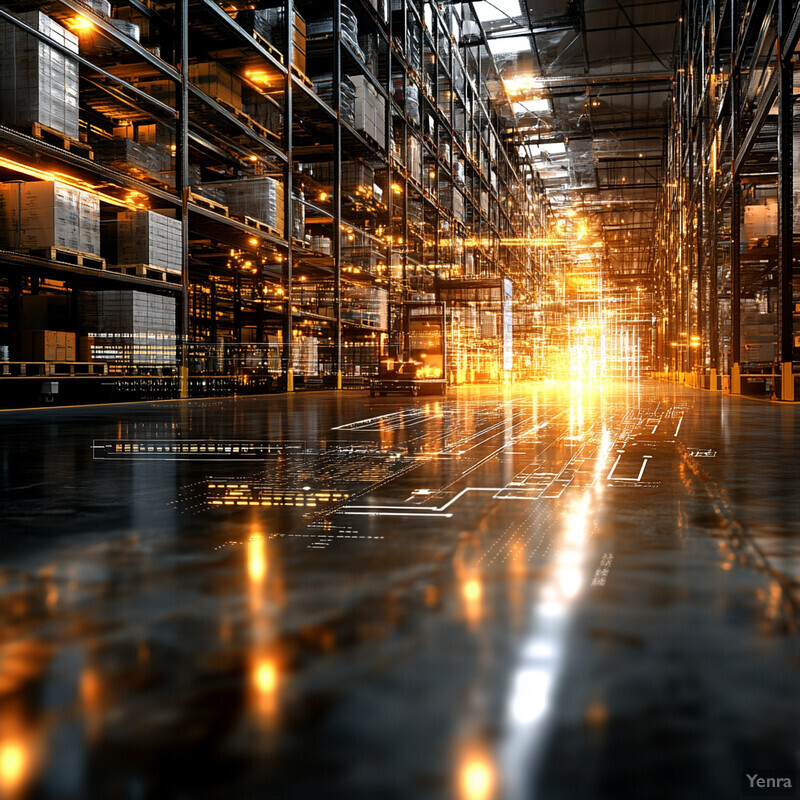
Optimal space utilization has a profound impact on warehouse performance – it reduces the need for larger facilities by maximizing storage capacity and eliminating underutilized areas. Studies note that efficient layouts directly enhance picking operations by eliminating unnecessary worker movement, yielding time and cost savings. A 2024 AI framework demonstrated the ability to automatically generate feasible warehouse layouts that meet all clearance and accessibility constraints while maximizing storage locations. Such AI-generated designs can be rapidly iterated and adjusted, helping human designers identify superior layouts more quickly than manual methods. Given that the vast majority of warehouses worldwide still rely on manual or basic methods (as of 2023), the introduction of AI for layout planning offers a significant opportunity to boost space efficiency and productivity in an industry that has lagged in optimization.
2. Predictive Demand Forecasting
AI-powered demand forecasting enables warehouses to anticipate inventory needs with unprecedented accuracy. By analyzing historical sales, seasonality, promotions, and even external factors like economic indicators, machine learning models can predict which products will be in high demand and which will slow down. These forecasts allow warehouse managers to proactively adjust storage allocation – dedicating prime space to fast-movers and positioning slow-movers in less accessible areas. The result is a more responsive warehouse that aligns space utilization with future demand, reducing overstock of unpopular items and preventing stockouts of hot sellers. In essence, predictive forecasting shifts inventory planning from a reactive guesswork exercise to a data-driven strategy, improving both space efficiency and customer service levels.
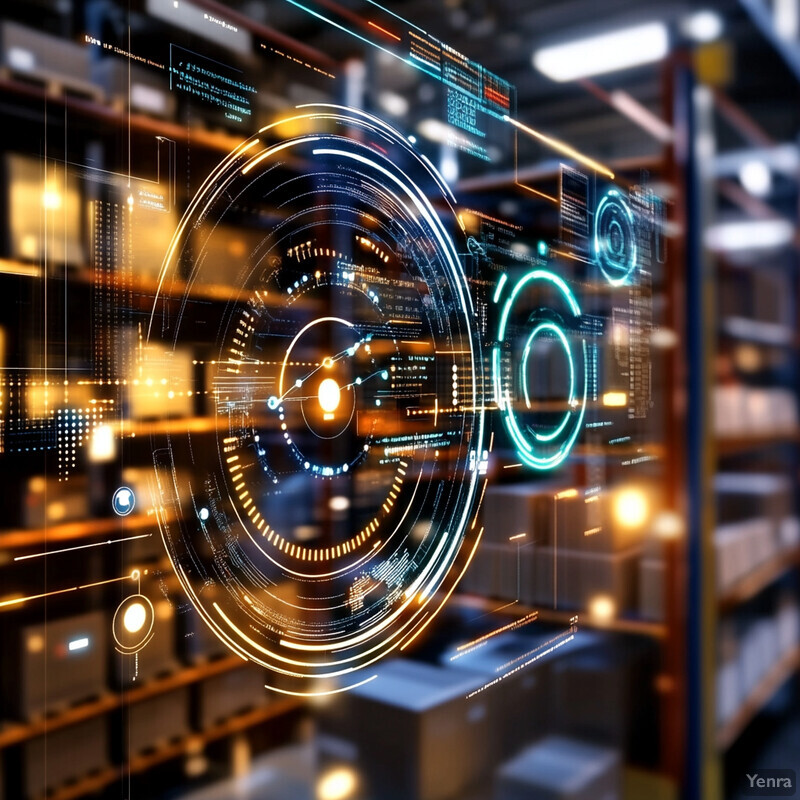
Companies adopting AI-driven forecasting report significant efficiency gains. McKinsey estimates that AI-based demand forecasting can reduce overall inventory levels by 20–30% by better matching stock with demand. This leaner inventory not only frees up warehouse space but also cuts carrying costs. Improved forecast accuracy has direct effects on service: one analysis found organizations using AI saw up to a 30% increase in forecast accuracy compared to traditional methods. This accuracy translates into higher order fill rates and fewer stockouts or rush orders. Real-world implementations back these trends – for example, an AI-enabled “control tower” at a building products distributor dynamically balanced inventory across its network and boosted order fill rates by 5–8% while reducing excess stock. These outcomes show how predictive forecasting allows warehouses to optimize space for what will sell, rather than what once sold, maintaining a leaner, more responsive inventory footprint.
3. Dynamic Slotting and Re-Slotting
Dynamic slotting refers to continuously updating product storage locations in the warehouse as demand patterns change. AI systems monitor sales trends, seasonality, and current stock levels in real time, and then recommend moving products to optimal locations (re-slotting) to preserve space and speed up picks. Unlike traditional slotting – which might be done infrequently and manually – AI-driven slotting is an ongoing process that responds to new data (like a sudden spike in orders for a SKU or the introduction of a new product line). High-demand items are consistently repositioned into forward pick zones or more accessible shelves, while slower items get relocated to consolidate space. This fluid approach ensures the warehouse layout “breathes” with the business, always reflecting the most efficient arrangement for current demand. Ultimately, dynamic slotting and re-slotting reduce travel time for pickers, prevent congestion in popular areas, and keep storage density high by freeing up space from areas where demand has waned.
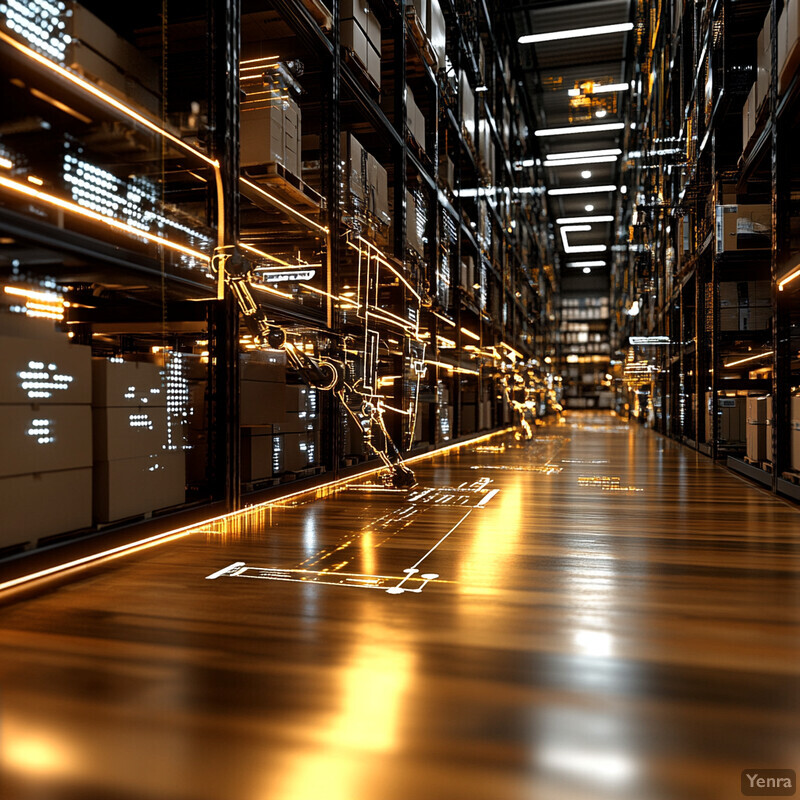
Warehouses deploying AI for dynamic slotting report substantial gains in efficiency. For example, Lucas Systems’ AI-driven slotting solution (launched 2024) is already used in 400+ warehouses worldwide and enables daily re-slotting of inventory. This system analyzes hundreds of parameters (SKU velocity, item affinity, sizes, pick paths, etc.) and can increase overall throughput by an estimated 20–40% by continually keeping fast-movers in optimal locations. Industry case studies show related improvements: early adopters have seen productivity boosts of 5–20% and labor cost reductions of 10–20% from AI-driven slotting, as the system minimizes unnecessary travel and manual reorganization work. The solution also maintains accuracy by factoring in item similarities – for instance, avoiding placing very similar-looking items next to each other to reduce pick errors. These outcomes highlight that continuously optimizing slot locations with AI can yield double-digit efficiency improvements, validating the strong business case for dynamic re-slotting.
4. Intelligent Product Grouping
Intelligent product grouping involves storing items that are often ordered together in close proximity to one another. AI achieves this by analyzing order history and identifying clusters of SKUs that frequently appear in the same orders (or within the same picking wave). By grouping these high-affinity products on nearby shelves or adjacent bins, the warehouse can cut down on the distance pickers travel to assemble orders. This strategy effectively treats the warehouse layout like a map of customer buying patterns – items with strong correlations (like a printer and its compatible ink cartridges) are co-located. Over time, such grouping reduces the number of back-and-forth trips across different zones for multi-item orders, which in turn saves time and floor space (by avoiding holding open multiple active pick faces far apart). In summary, AI-driven grouping streamlines picking routes and compresses the footprint needed for frequent item combinations, all while preserving logical inventory organization.
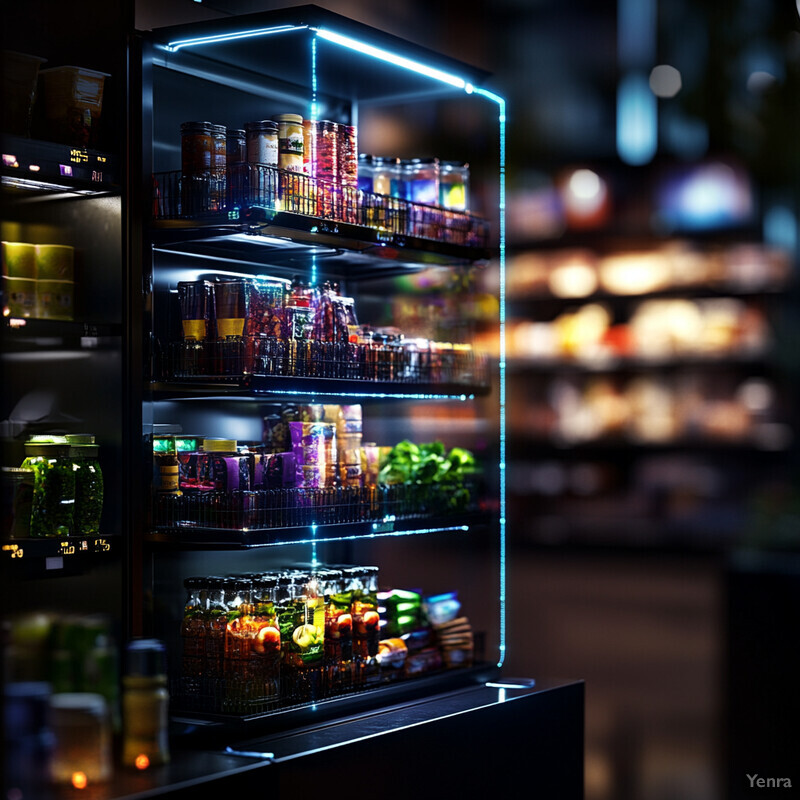
Data-driven grouping of products can yield measurable improvements in efficiency. AI systems can learn customer ordering patterns and recommend storing commonly picked-together items close by, directly boosting picking productivity. Oracle reports that AI-driven analysis of order histories allows warehouses to place high-affinity SKUs near each other, which reduces traversal time and speeds up order processing. In practice, operations research has long noted benefits: for example, considering SKU correlation (which items tend to be picked together) alongside traffic flow was shown to improve picking performance by a further margin versus correlation-only storage methods. (In one simulation study, a model that balanced item affinity and picker traffic outperformed a purely affinity-based layout by about 8% in throughput.) These findings underscore that intelligently clustering items not only cuts travel distance but also mitigates aisle congestion, leading to faster, more space-efficient order fulfillment. AI makes it feasible to uncover and act on these grouping opportunities continuously as demand patterns evolve.
5. Advanced Inventory Level Monitoring
Advanced inventory level monitoring leverages IoT sensors, RFID tags, and computer vision to track stock quantities and locations in real time. In a warehouse with this capability, shelves might have weight sensors or cameras that detect when an item is added or removed, and drones or robotics might conduct automated cycle counts. AI then aggregates these streams of data to maintain an accurate, up-to-the-minute view of inventory. This continuous monitoring means that free space can be identified instantly – when a pallet is shipped out or a bin is emptied, the system can suggest repurposing that space immediately. Likewise, if inventory is running low, the system flags it for replenishment before a stockout occurs. By dynamically reallocating space as items are sold or received, warehouses avoid the common scenario of “lost” space (empty slots that go unrecognized) and reduce the need for manual cycle counting or safety stock pileups. Overall, this results in better space utilization (every slot used to its fullest) and higher inventory accuracy, since the AI is essentially doing perpetual inventory audits.
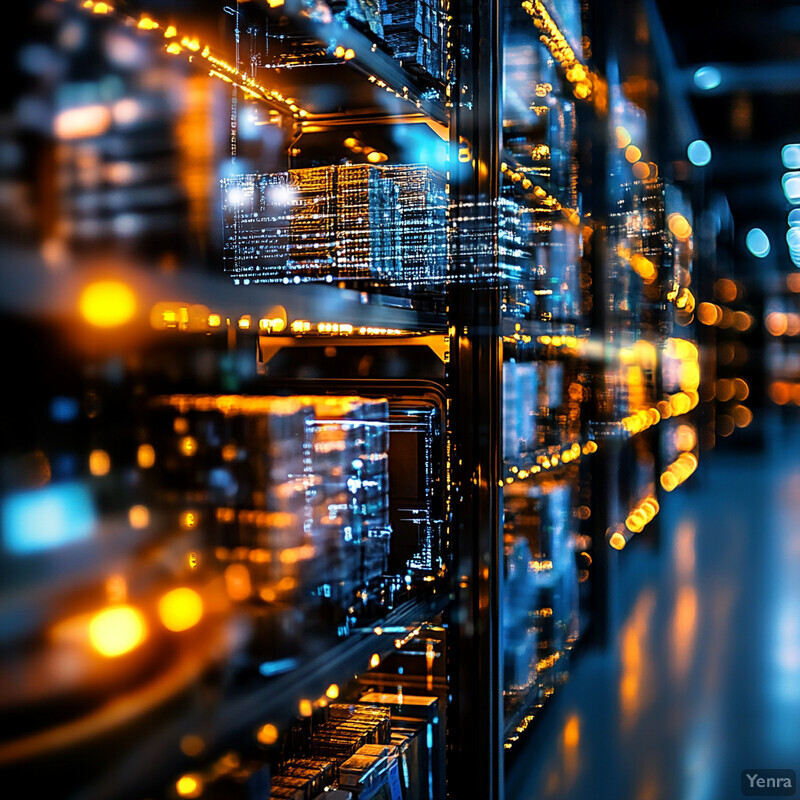
Real-world deployments of AI-driven inventory monitoring show dramatic improvements in both speed and accuracy of stock management. In one 2024 study, a computer vision platform for live inventory tracking cut the time required for inventory counting by ~45% and increased count accuracy by 9% compared to traditional methods. These gains mean workers spend far less time doing manual counts or searching for misplaced items. The technology is catching on quickly: Gartner projects that by 2027, half of companies with warehouse operations will be using AI-enabled vision systems for tasks like cycle counting, up from roughly 20% adoption in 2023. Already, about one in five supply chain professionals had begun using AI vision for inventory by the end of 2023. Such systems combine 3D cameras and AI pattern recognition to automatically detect inventory levels and even identify discrepancies or misplaced goods. The result is a highly accurate real-time inventory ledger and a warehouse where available space is immediately recognized and can be reallocated without human intervention.
6. Computer Vision for Aisle Optimization
In aisle optimization, computer vision (CV) technologies are used to monitor and improve how space in warehouse aisles is utilized, with a focus on safety and traffic flow. High-mounted cameras and AI can observe the movement of workers, forklifts, and robots through the aisles, identifying patterns like congestion points or underutilized routes. The AI might suggest rearranging certain racks or adjusting one-way designations to smooth out traffic. Moreover, CV systems can detect obstacles or improperly stored items in aisles – for example, a pallet overhanging into an aisle or debris that could cause accidents – and alert staff to address them promptly. Essentially, AI “watches” the aisles in real time, ensuring they remain clear and optimally configured. By analyzing this visual data, warehouses can both improve safety (fewer blocked exits or unsafe blockages) and increase efficiency (reducing wait times when multiple pickers or vehicles converge). In short, computer vision helps maintain orderly, open aisles that fully support the warehouse’s throughput needs without becoming a safety hazard or bottleneck.
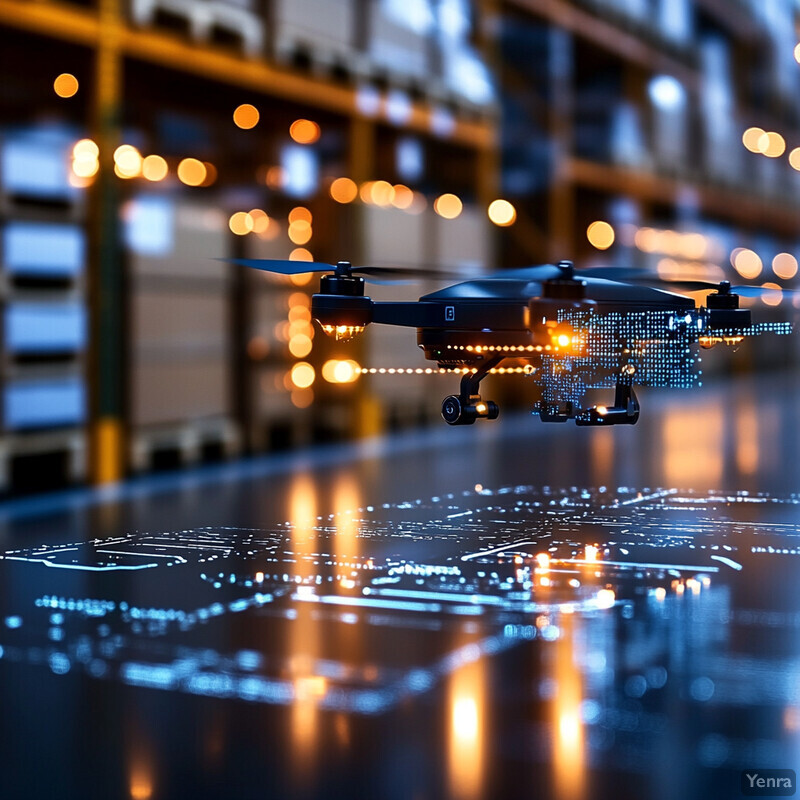
AI-enabled vision systems are proving invaluable for maintaining safe and efficient aisles. DHL’s 2023 industry trend report highlights that computer vision can act as an always-alert safety inspector – identifying potential hazards in warehouses (like blocked pathways or unsafe stacking) and thus helping prevent accidents. These systems use existing CCTV feeds combined with AI to ensure that aisle conditions meet safety standards in real time (for example, detecting if a fire exit aisle is obstructed and sending an immediate alert). Beyond safety, vision analytics improve flow: by tracking movement, AI can flag persistent aisle congestion or traffic jams. In practice, companies are rapidly adopting such technology – Gartner analysts noted that the “value proposition is evident” for AI vision not just in inventory counting but also in monitoring worker ergonomics and safety in aisles. With logistics leaders expecting computer vision to become a standard part of warehouse operations within five years, many warehouses are already using it to optimize aisle layouts and traffic rules. The outcome is twofold: a reduction in safety incidents and smoother traffic flow, which together mean more productive use of aisle space.
7. Automated Storage and Retrieval System (AS-RS) Integration
Automated Storage and Retrieval Systems (ASRS) are robotics-driven systems (such as cranes, shuttles, or vertical lift modules) that automatically store and retrieve goods in a high-density storage structure. Integrating ASRS with AI and warehouse management software allows warehouses to utilize space far more effectively, especially vertical space. These systems often feature tall racks (sometimes reaching the ceiling of the warehouse) and narrow aisles accessible only by robots, thereby significantly increasing cubic storage density. AI comes into play by intelligently managing where items should be stored within the ASRS (for example, placing fast-moving items in more accessible locations) and coordinating retrieval sequences to minimize idle robot time. When ASRS is integrated end-to-end, inventory moves seamlessly from storage to picking stations with minimal human handling. The overall impact on space utilization is profound: ASRS allows warehouses to store more product in the same footprint (often eliminating the need for expansive floor aisles), and the integration with AI ensures this dense storage is organized optimally for current demand patterns. In effect, ASRS integration marries space-saving hardware with smart software, creating highly efficient “vertical” warehouses within the warehouse.

The introduction of ASRS can yield dramatic space savings and throughput improvements. According to industry analyses, an ASRS can save up to 85% of a warehouse’s floor space by utilizing the full available height of the building. By replacing wide human-operated aisles with compact robotic aisles and tall racks, facilities store much more inventory in the same square footage. This is corroborated by vendors: unit-load crane ASRS and shuttle systems often achieve storage densities unattainable with conventional rack – one report notes high-density systems can improve warehouse space utilization by roughly 48% on average in real deployments. Adoption is accelerating; as of 2025 a significant portion of new warehouses are investing in ASRS technology, and most are integrating these systems with their WMS/ERP for maximum efficiency. In fact, one market study found ASRS implementations coupled with AI/IoT have improved operational efficiency by ~35% and cut errors by 30% for adopters. These integrated ASRS deployments not only pack more goods into the warehouse but also maintain high accuracy (often above 99% order accuracy) and speed, showing the synergy of automation hardware and intelligent software in using space optimally.
8. Predictive Maintenance of Infrastructure
Predictive maintenance uses AI to forecast when warehouse equipment or infrastructure is likely to fail or require service, allowing for proactive upkeep that minimizes downtime. In a warehouse context, this can apply to conveyor systems, automated sorters, forklifts, HVAC systems, and even the building structure (doors, loading docks, etc.). Sensors on these assets feed data (vibration, temperature, cycle counts, etc.) into AI models that learn normal patterns and detect anomalies that precede a failure. By catching issues early – for example, predicting that a conveyor motor is trending toward overheating – maintenance can be scheduled at a convenient time before a breakdown occurs. This approach contrasts with traditional maintenance schedules (or reactive fixes after a breakdown) by focusing on the actual condition of equipment. The benefit for space utilization is indirect but important: equipment failures often cause backups of inventory (items accumulate when a sorter is down, taking up space) or necessitate creating redundant buffer stock. With predictive maintenance, operations run more reliably, meaning the warehouse doesn’t need as much extra space for work-in-process or emergency stock. The net effect is a leaner, smoother operation where every piece of infrastructure is kept in optimal shape through AI-driven foresight.
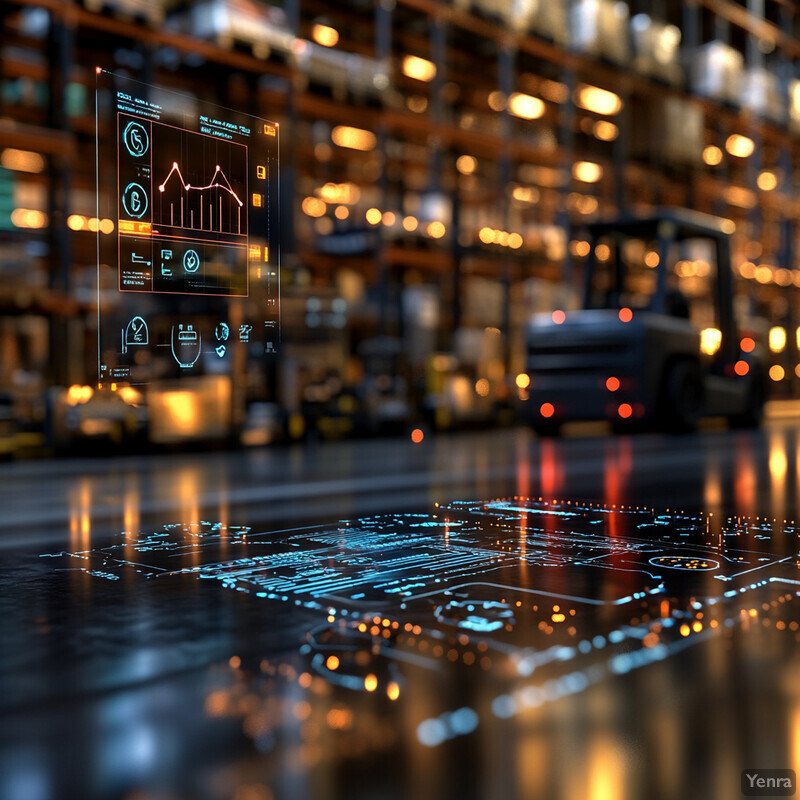
Embracing AI for maintenance has shown striking results in reducing unplanned outages and costs. Studies indicate that predictive maintenance can reduce machine downtime by 30–50% and extend equipment life by 20–40%. In warehouses, this translates to conveyors and lift trucks being available more often, avoiding the space and productivity crunches caused by sudden breakdowns. Maintenance costs also drop – on average companies see about a 25% reduction in maintenance expenditures when shifting from reactive to AI-predictive regimes. Real-world examples illustrate these benefits: in 2023, IBM and SAP announced an AI-driven solution to integrate predictive insights into asset management, and Honeywell introduced wireless transmitters that enable condition-based monitoring of warehouse machinery. These tools have helped early adopters catch issues that would previously have gone unnoticed. For instance, logistics firms using such AI report significantly fewer conveyor jams and forklift failures, contributing to an overall logistics cost reduction of around 15% in operations that have implemented AI across the supply chain. By preventing disruptions, predictive maintenance ensures that space (like surge staging areas or extra inventory) doesn’t need to be set aside “just in case” of equipment downtime – the equipment is simply up and running as needed.
9. Digital Twins for Scenario Testing
A digital twin is a virtual replica of a warehouse (or specific processes within it) that allows managers to test “what-if” scenarios without disrupting the real operation. By integrating real-time data from the warehouse into a simulation model, a digital twin behaves identically to the actual facility – meaning one can experiment with layout changes, process adjustments, or new equipment in the digital realm first. Scenario testing with digital twins might include: evaluating how a different rack configuration could improve flow, seeing the impact on order throughput if inbound deliveries double, or finding bottlenecks if a certain conveyor goes down. AI enhances these simulations by quickly analyzing the outcomes of many scenarios and even by using reinforcement learning to discover optimal solutions (like the best placement of fast pick zones). The key benefit is risk-free experimentation: warehouses can optimize space and processes in the twin, then implement changes in the physical warehouse with confidence about the expected results. This leads to continuous improvement – the warehouse is always prepared for changes in demand or operations because those changes were refined in advance using the digital twin. In short, AI-driven digital twins make the warehouse more adaptable and ensure space arrangements are always tested and justified before being applied.
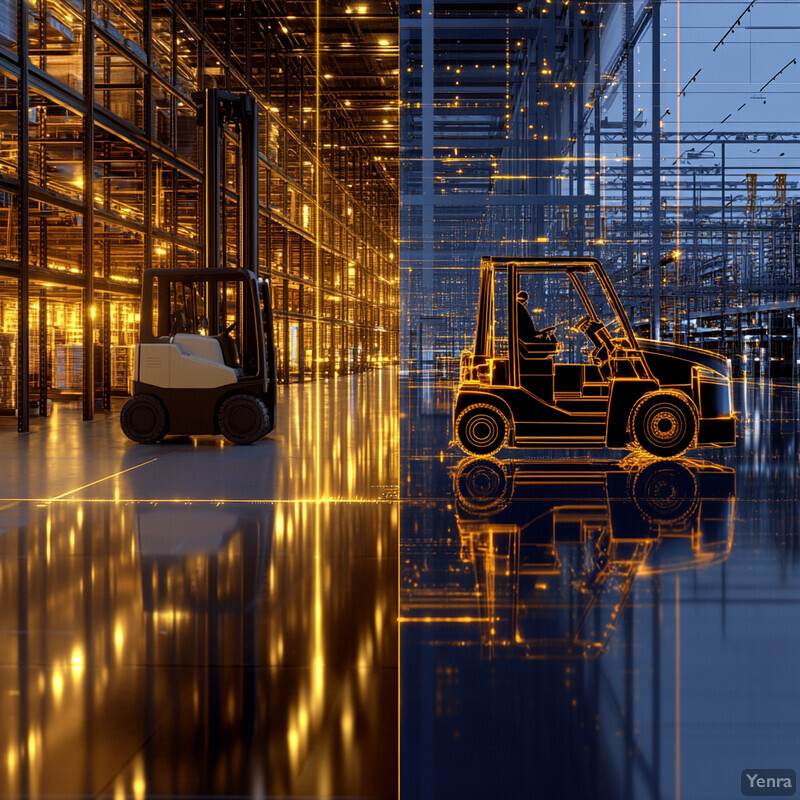
Digital twin technology has proven its value in identifying space and efficiency improvements that were not obvious through manual analysis. McKinsey reports that AI-powered tools (like digital twins and advanced simulations) can unlock 7–15% additional capacity in warehouse networks by revealing underused space and optimizing resource allocation. In one case, a major logistics provider used an AI-driven digital twin to increase its warehouse capacity by nearly 10% without adding any new real estate. The twin ran countless simulations to pinpoint how to reorganize workflows and labor scheduling, uncovering “hidden” capacity in the existing facility. This example shows how scenario testing can defer or eliminate the need for expansion by finding smarter ways to use current space. Digital twins also allow cost-benefit analysis of changes: for instance, simulating a tighter storage layout might show increased picking congestion, steering managers away from a change that looks good on paper but hurts throughput. Industry adoption is growing as well – leadership surveys indicate that over two-thirds of supply chain leaders plan to invest in simulation and digital twin technology by 2025 (a trend accelerated by the need for resilience and flexibility). By the time these digital tools are commonplace, scenario testing of warehouse layouts and operations will be a continuous, AI-assisted process, ensuring the physical warehouse is always configured for peak performance.
10. Reinforcement Learning for Continuous Improvement
Reinforcement learning (RL) is an AI approach where algorithms learn optimal behaviors through trial and error, receiving feedback (rewards or penalties) for their actions. In a warehouse setting, RL can be used to continually refine processes such as order picking strategies, equipment routing, or inventory placement. Unlike static optimization (which might yield a one-time solution), an RL agent keeps learning from the warehouse’s day-to-day operations. For example, an RL system might start by suggesting random picking routes and, by observing the time taken (rewarding shorter times), gradually learn the most efficient routes through the warehouse. Similarly, RL could learn how to redistribute products in the warehouse over time – for instance, testing slight adjustments in slotting and seeing if it reduces congestion or travel distance, then reinforcing the beneficial changes. The beauty of RL is that it adapts to changes in the environment: if a new product line is introduced or order profiles shift, the RL agent will explore new actions and learn the new optimal patterns. Over time, this leads to a warehouse operation that is self-tuning – continuously improving policies like pick sequences, replenishment timing, or forklift paths as it “learns” from ongoing experience.
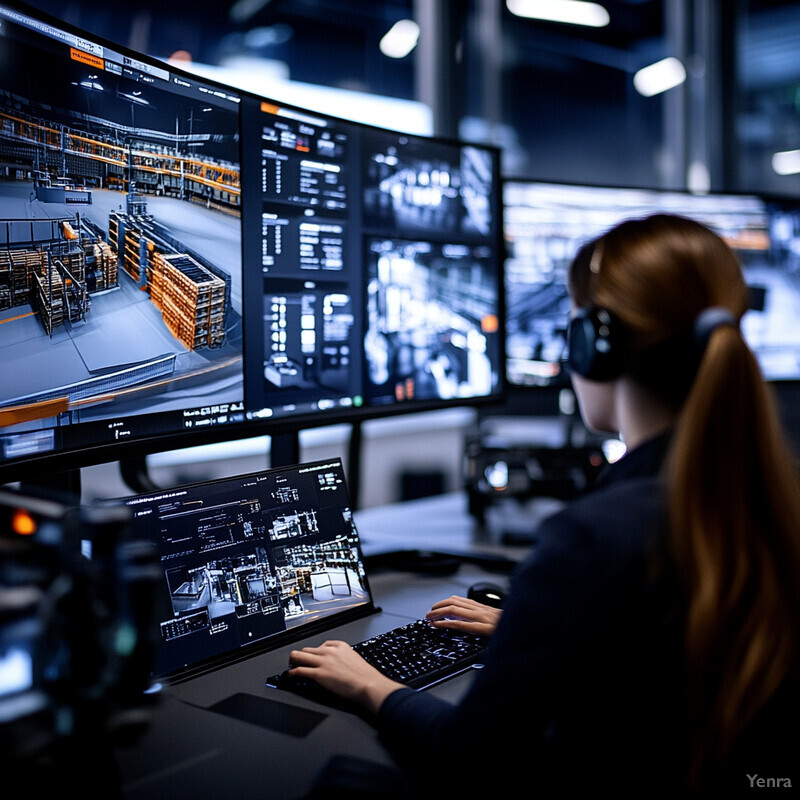
Research showcases the power of reinforcement learning in complex warehouse optimization problems. A 2024 study applied deep reinforcement learning to the dynamic order-picking problem, where orders arrive in real time and picker routes must adapt on the fly. The RL-based system was able to significantly reduce order throughput time and the number of unfulfilled orders under high-volume conditions compared to benchmark algorithms. In essence, the AI learned routing and batching strategies that outperformed human-designed heuristics, especially as order arrival rates increased. Another use case is multi-agent RL for fleet management – for example, coordinating a team of autonomous mobile robots. Amazon scientists have reported using multi-agent RL to optimize task assignments for robots in sorting systems, leading to smoother traffic and higher throughput (as each robot “learns” to pick tasks that minimize overall idle time). While still an emerging technology in live warehouses, early adopters like large e-commerce fulfillment centers are experimenting with RL in simulation and seeing promising results. The expectation is that RL could yield continuous improvement gains on the order of 5–15% in productivity annually, as it keeps finding incremental optimizations beyond what static systems can do. Over a few years, these compounding improvements can dramatically elevate space utilization and efficiency – essentially, the warehouse learns to operate more effectively each day.
11. Real-Time Dock and Staging Area Management
Real-time dock and staging area management uses AI to coordinate the flow of goods at the warehouse’s entry and exit points – essentially orchestrating trucks at loading docks and the staging of inbound and outbound loads. An AI-powered dock scheduling system will account for factors like truck arrival times, loading/unloading durations, carrier priorities, and yard congestion to dynamically assign docks and appointment slots. Instead of static schedules made a day prior, it continuously adjusts as conditions change (for example, if a truck is delayed, AI can reallocate its dock to another waiting truck to avoid idle dock time). Similarly, for staging areas where pallets are held before put-away or shipping, AI can optimize layouts – ensuring that newly received goods don’t clutter prime space and that outbound orders are staged in an organized manner for quick loading. By managing these zones in real time, the warehouse reduces dwell times (how long trucks wait) and prevents staging areas from overflowing or becoming chaotic. In practice, this means smoother inbound receiving (no bottleneck of trucks) and efficient outbound dispatch (trucks spend less time at the dock because everything is ready and optimally positioned). Overall, AI-driven management of docks and staging turns these typically transient spaces into well-oiled components of the warehouse, minimizing the space and time they consume.
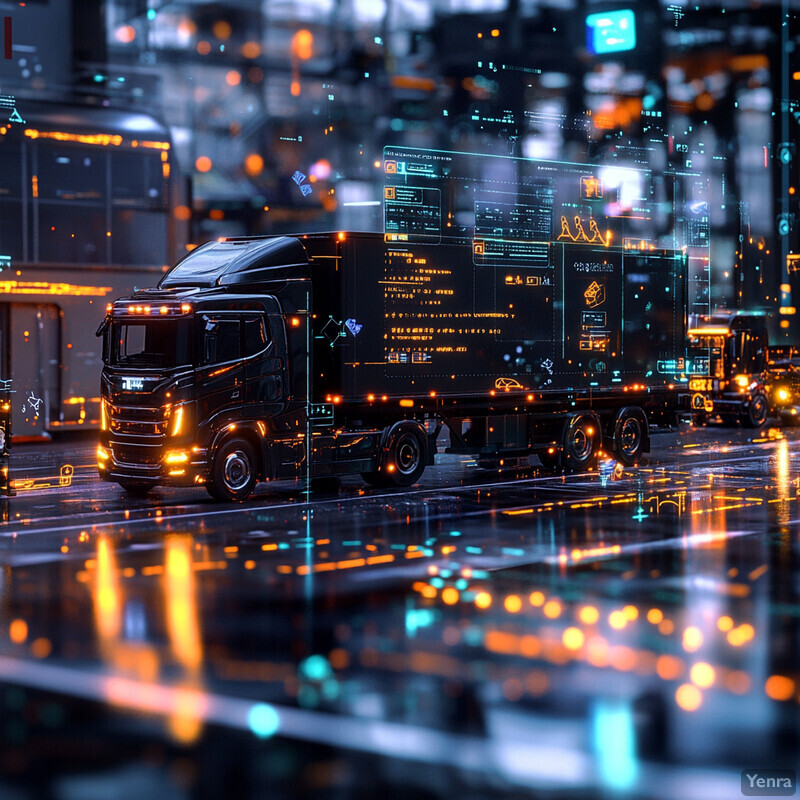
Many warehouses have begun adopting AI-driven dock scheduling, yielding noticeable efficiency improvements. The market for dock scheduling software has grown rapidly – valued at approximately $4.6 billion in 2023 and projected to grow at 11% CAGR through 2032 – reflecting how companies are investing in technology to streamline dock operations. Early results are compelling: AI systems that optimize dock assignments in real time can reduce average truck dwell times by 20–30%, leading to less congestion in yards and better dock utilization (thus eliminating wasted idle dock space). Logistics teams using such tools report that fewer trucks need to queue up because appointments are dynamically smoothed. Additionally, AI-driven resource allocation at docks and staging has cut overall logistics costs by around 15% for early adopters by reducing overtime, detainment fees, and inefficient moves. For instance, consumer goods companies are deploying real-time dashboards for supply chain agility – about 84% of CPG firms plan to invest in these, which include live dock management consoles to adjust schedules on the fly. All these improvements mean that staging areas don’t overflow (because unloading is paced and directed by AI) and that docks are almost always either servicing a truck or reallocated promptly. In sum, AI minimizes the space and time “wasted” in staging and loading, converting them into tightly controlled, efficient transitions.
12. Incorporating Seasonal and Market Trends
This practice involves tuning warehouse space utilization in anticipation of seasonal demand swings and broader market trends. AI systems analyze patterns like holiday spikes, weather-related demand changes, or even social media trends that can influence sales, and then recommend adjustments to inventory and layout accordingly. For example, heading into the winter holiday season, an AI might forecast a surge in certain toys or electronics and suggest allocating more space (or more forward pick locations) to those items while moving slow-selling SKUs into long-term storage to free prime space. Conversely, after a season, AI can flag overstock of seasonal goods (e.g. unsold summer inventory) for consolidation or clearance to reclaim space. Incorporating market trends can mean responding to external data – if the economy is slowing and particular product lines are expected to stagnate, the warehouse can proactively reduce stock (and space) for those and avoid clogging up aisles. Essentially, the warehouse becomes proactive rather than reactive: AI “foresees” upcoming demand waves or lulls and allocates space in advance. This leads to better space utilization because high-demand items get the room they need at the right time, and slow movers don’t occupy prime real estate longer than necessary. It also improves responsiveness – the warehouse is pre-arranged to efficiently handle whatever each season or market shift will bring.
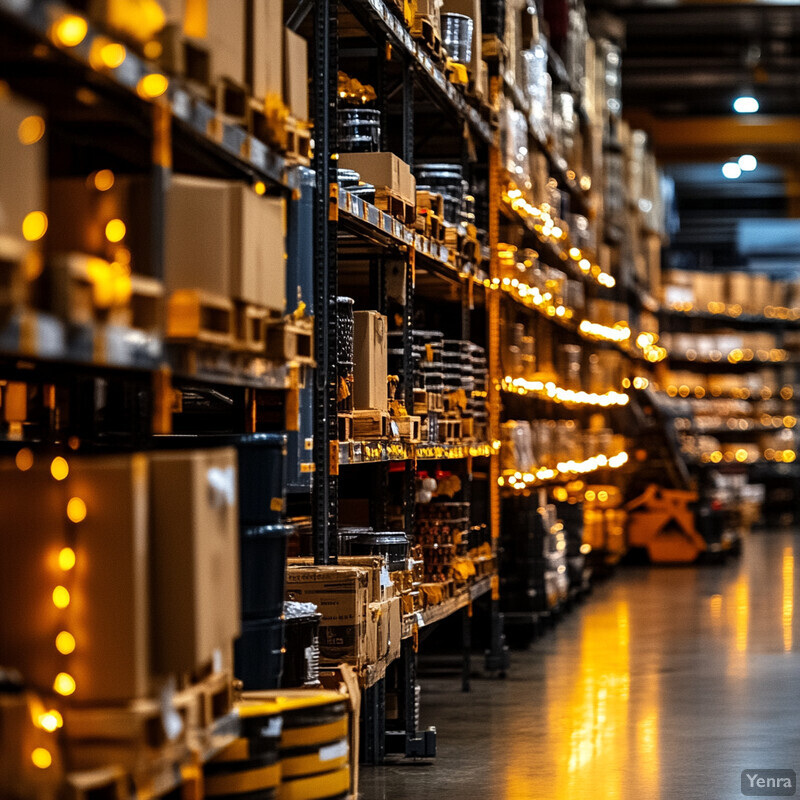
AI-driven forecasting systems explicitly account for seasonality and external trends, and the resulting space planning benefits are well documented. Modern AI forecasts consider multiple factors – historical sales, promotions, weather, regional events – to produce time-specific demand predictions, which then inform how space is allocated for seasonal peaks. For instance, an AI might predict a 50% increase in demand for rain gear in a region due to an upcoming storm season and recommend clustering and front-loading that inventory. The payoff is tangible: companies using such sophisticated forecasting saw significantly higher utilization of their stocking capacity and fewer instances of having to rent overflow storage during peak seasons. Accuracy is also improved – research found that when AI integrates external variables (like economic indicators or Google search trends), forecast error drops, improving accuracy by up to 30% versus methods ignoring those factors. Retail case studies have shown that aligning warehouse slotting with seasonal demand windows cuts down on late-season excess inventory by double-digit percentages (as space is proactively cleared via promotions or returns for items expected to wane in demand). Additionally, warehouses employing AI to plan for seasons report smoother transitions – for example, holiday merchandise is brought to forward picking exactly when needed and then efficiently swapped out for spring goods as forecasts dictate. This strategic timing and arrangement of inventory, guided by AI, maximizes the productivity of each square foot through the ebb and flow of seasons.
13. Optimized Pallet and Container Utilization
AI can greatly improve how products are arranged on pallets and in shipping containers, ensuring that every cubic inch is used efficiently (often referred to as “cube optimization”). Traditionally, workers or basic software follow heuristic rules to build pallets (e.g., heavier items on bottom, don’t mix certain items) and might leave a lot of unused space. AI-based loading algorithms, however, can consider the exact dimensions and weight of each box and figure out the optimal stacking or Tetris-like arrangement to minimize air space. These algorithms can operate in real time as orders are picked or containers are being loaded, dynamically suggesting how to rearrange items or when to consolidate loads. By doing so, warehouses can ship the same quantity of goods in fewer pallets or containers, freeing up storage space that would otherwise hold partially filled pallets waiting for consolidation. In inbound logistics, AI might direct that incoming goods be repalletized into fuller pallets. In outbound, it might consolidate multiple small orders into one container if going to nearby destinations. The overall effect is a leaner operation: fewer pallets on the warehouse floor (each pallet carries more product on it) and fewer half-empty containers stored or shipped. Additionally, better pallet stability and reduced shipping costs are fringe benefits of AI-optimized loading.
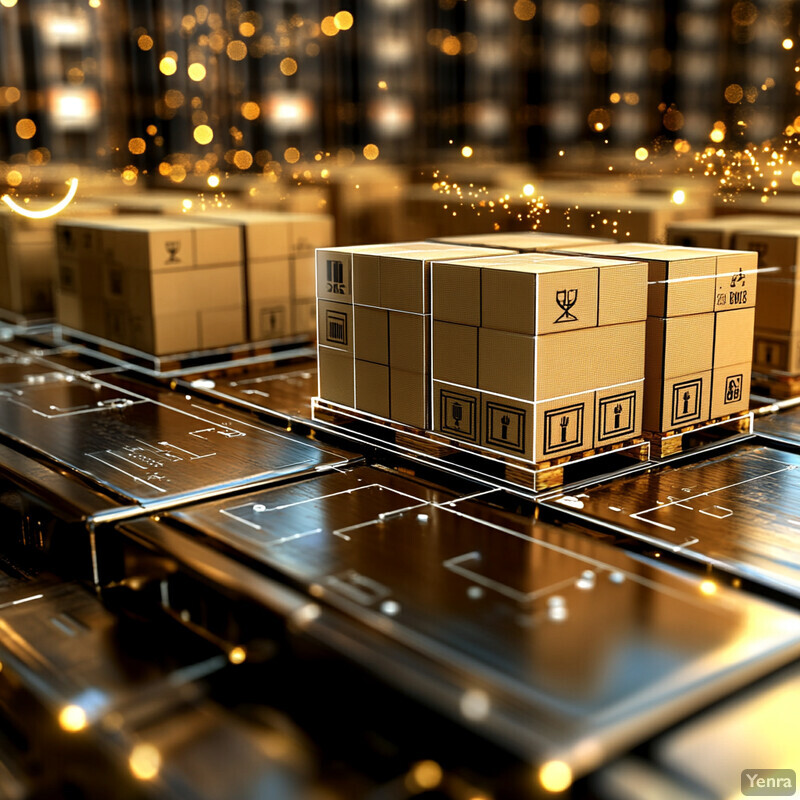
The efficiency gains from AI-optimized load planning are substantial. A striking statistic: nearly 24% of every shipping container on average is just empty space, representing tens of millions of empty containers shipped each year and an estimated $46 billion in wasted cost globally. AI load optimization directly tackles this problem. For example, decision-making AI systems (using techniques like deep reinforcement learning) have been deployed to find packing configurations that humans would rarely consider – these systems consistently produce pallet load plans that use space more fully and improve stability. One AI tool, “DeepPack,” was reported to continuously learn and adapt packing strategies, achieving better container fill rates than previously thought possible through manual methods. The impact is measurable: companies that adopted AI for cargo load planning have reduced the number of containers or trucks they need for the same volume of goods, often seeing real-time load plan generation that cuts planning time by over 90% and significantly reduces shipping of “air” (empty space). In sustainability terms, this also means fewer vehicles on the road and lower carbon emissions, a win-win. In sum, AI’s ability to solve complex 3D packing puzzles translates into leaner inventory staging (fewer pallets waiting around) and cost savings, as evidenced by the move toward AI-based load planning in logistics-heavy industries.
14. Traffic Flow and Routing Optimization
Within a busy warehouse, optimizing traffic flow and routing is about ensuring that forklifts, pallet jacks, automated guided vehicles (AGVs), and even human pickers move through the facility in the most efficient way possible. AI assists by analyzing travel paths, frequencies, and bottlenecks, then recommending or automatically directing optimal routes. For instance, an AI-driven warehouse execution system might assign routes to AGVs that avoid areas of congestion and balance the traffic load across multiple aisles. It can also dynamically reroute vehicles if an aisle becomes unexpectedly blocked or busy. For manual operations, AI can inform picker routing (order picking sequencing algorithms) so that workers take a path that collects all items in one smooth loop rather than zigzagging unnecessarily. Over time, the system learns from data – identifying that certain intersections get overcrowded at specific times and adjusting travel rules or task assignments to mitigate that. By optimizing routing in this way, warehouses minimize wasted motion, reduce the chance of traffic jams or collisions, and use the full breadth of the warehouse space instead of having everyone crowd into popular zones. This leads to faster cycle times for moving goods and a more balanced utilization of space (no aisle stays underused while another is overburdened). Essentially, AI turns the warehouse into a managed road network, orchestrating movement so that every “vehicle” or person takes the best path at the right time.
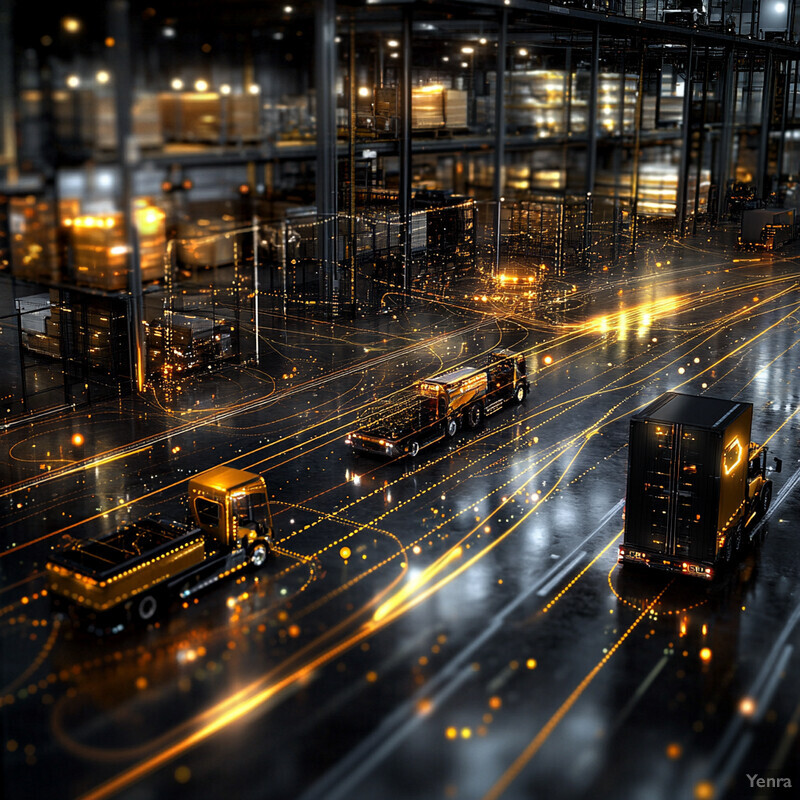
AI-driven routing optimization has been shown to improve throughput and reduce travel distances inside warehouses. For example, AI algorithms now recommend optimal pick paths for workers, cutting average walking distance per order by significant margins (often 10–30% based on internal tests), which in turn speeds up order fulfillment. Oracle’s warehouse AI suite notes that by analyzing picking patterns, AI can suggest best routes for picking and even for packing and shipping, leading to faster and less labor-intensive operations. In automated systems, companies like Amazon have deployed traffic management AI for their fleet of robots, achieving higher efficiency: reports indicate their robotic systems leverage AI to avoid gridlock and have increased throughput capacity without adding more robots, simply by smarter routing and task assignment. Moreover, consideration of real-time traffic in storage layout decisions can yield measurable gains – an operations research study found that accounting for picker congestion in route planning improved order picking efficiency by about 8% beyond what was achieved by optimizing storage locations alone. While such detailed studies were initially theoretical, warehouses are now putting them into practice with AI: modern warehouse management systems incorporate travel distance and congestion data to continuously refine pick lists and task interleaving. The outcome is a smoother flow of goods and workers, fewer choke points in aisles, and better utilization of all travel paths in the facility. Put simply, AI keeps the warehouse traffic moving at an optimal pace.
15. Adaptive Height and Density Management
Adaptive height and density management refers to dynamically adjusting storage configurations – such as shelf heights, rack spacing, or storage density – in response to the sizes and quantities of products being stored. Not all products are the same size or turnover rate, so a one-size-fits-all shelf layout can leave a lot of unused vertical space (for instance, a half-empty top shelf because the items are short, or a fully empty shelf reserved for a product that’s out of stock). AI systems can analyze the inventory profile and identify opportunities to tweak storage structures. In a warehouse with modular or adjustable shelving, AI might recommend moving a shelf up or down to snugly fit the items and eliminate “air” above products. It can also suggest when to use high-density storage techniques – for example, placing slow-moving SKUs in double-deep racks or push-back lanes that pack them tightly, while keeping fast-movers in single-deep for accessibility. If the warehouse employs mobile shelving units (racks that can compact together), AI can control when to open or close aisles to maximize space versus access as needed. Essentially, adaptive management means the warehouse’s physical storage setup isn’t static: it flexes based on what’s stored and how often it’s accessed, as determined by AI. This ensures that the warehouse always operates at high storage density without compromising the ability to reach products when needed.
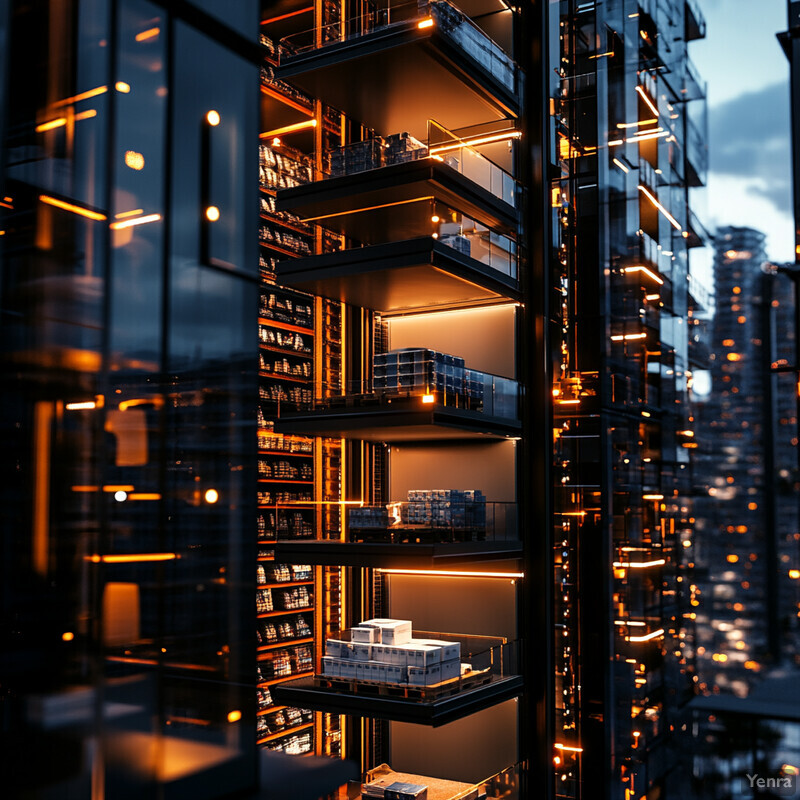
Employing AI to manage storage density can significantly increase capacity. For example, using denser storage configurations for appropriate items can boost storage capacity by around 30% – one common method is double-deep pallet racking, which stores pallets two deep instead of one and is best for slower-moving stock. Industry figures show that double-deep racking can immediately increase warehouse capacity by up to 30% compared to standard single-deep racks meijer-handling-solutions.com , and AI helps determine which products are suitable for this arrangement (those that don’t need frequent access). On the smaller scale, some warehouses have implemented AI-guided vertical storage systems (like automated vertical lift modules) that adjust tray spacing to item height; these systems have reported recovering significant vertical space – often yielding 80–90% space utilization within the module where traditional shelving might achieve only ~50%. In cold storage facilities, where space is extremely expensive, AI-managed mobile racking (which moves on tracks to eliminate unused aisles) has allowed facilities to store as much as 2 times the pallet count in the same footprint by only opening aisles when access is needed. AI plays a role in controlling these movements efficiently. The trend is clear: warehouses that leverage AI recommendations for high-density storage (e.g., compressing slow movers, dynamically adjusting shelf space) are able to store more product without expansion, and they maintain accessibility by letting the AI find the right balance. This results in tangible savings; for instance, a facility might avoid building a new 100,000 sq ft addition simply by implementing these adaptive storage strategies to use existing space more fully.
16. Quality Control in Space Utilization
Quality control in space utilization means ensuring that efforts to pack the warehouse more densely or to speed up operations do not lead to errors, damage, or safety issues. As warehouses optimize space, items are often stored closer together and higher up – this makes it even more critical to monitor that everything is stored correctly (right item, right location) and safely (no overhanging pallets, no crushed products). AI assists here through computer vision and other sensors to keep an eye on storage conditions. For example, cameras can scan pallet stacks to ensure they are stable and not leaning (which could cause a collapse). Vision systems at packing stations can automatically verify that the right items and quantities are being picked or packed, catching mistakes in real time. AI can also analyze patterns of errors or damages – if a certain zone of the warehouse sees repeated picking mistakes or product damages, the AI flags it, prompting an investigation (perhaps the shelves are overfilled or labeling is confusing in that high-density area). By maintaining strict quality oversight, warehouses don’t sacrifice accuracy or safety on the altar of space efficiency. The goal is to have densely packed storage that still upholds all quality standards: correct inventory counts, undamaged goods, and safe working conditions. AI effectively acts as a guardian, continuously inspecting and alerting when quality or safety deviates, so that the warehouse can enjoy high utilization without the typical downsides of crowding.
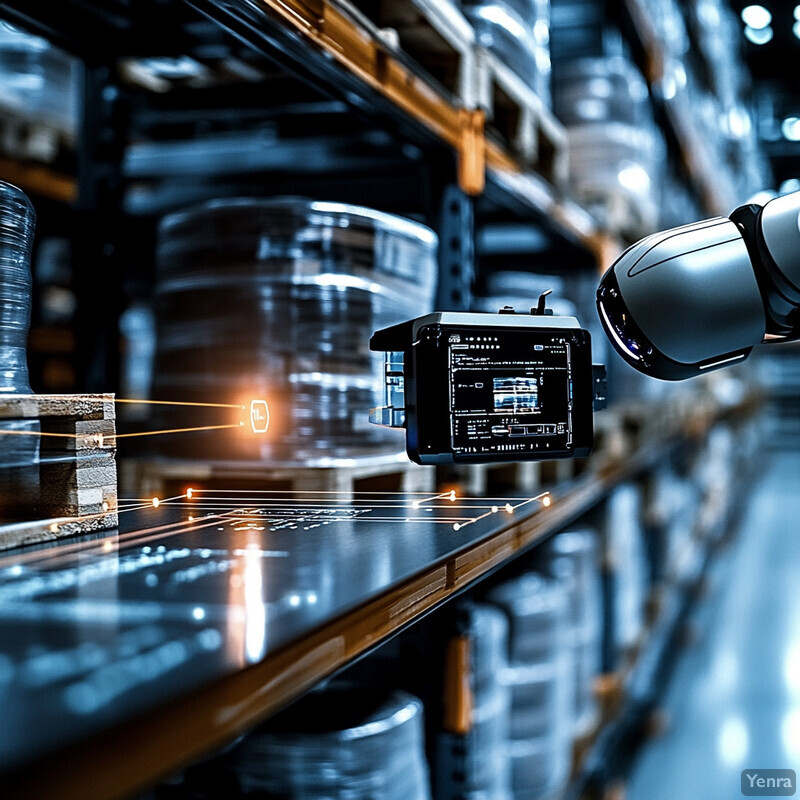
AI technologies have proven effective at sustaining quality and safety even as warehouses intensify their storage and picking processes. One prominent example is computer vision inspection at the point of pick/pack – AI-powered cameras scan each item picked and have been able to automatically catch mis-picks or wrong barcodes with very high accuracy, significantly reducing shipping errors. Warehouses using vision for pick verification report achieving 99.9%+ order accuracy, even when speeding up throughput, because mistakes are flagged immediately by the AI (for instance, if a worker picks an item that doesn’t match the order, the system alerts them on the spot). On the storage side, AI-enabled cameras monitor rack and pallet conditions; DHL’s innovation report notes that computer vision can “improve workplace safety by identifying potential hazards in warehouses”, such as improperly stacked goods or blocked aisles. These vision systems have reduced accidents – some warehouses saw a notable drop in rack collapse incidents or pallet falls after implementing AI monitoring, as issues like a pallet jutting out of a rack are caught early. Furthermore, environmental quality is maintained: AI IoT sensors ensure that even tightly packed storage of sensitive goods (food, pharma) stays within temperature/humidity specs, alerting staff before a small deviation becomes a spoilage event. All these measures illustrate that with AI oversight, space optimization doesn’t come at the cost of quality or safety – warehouses have actually improved these metrics alongside efficiency. In short, AI helps “squeeze” the warehouse safely and accurately.
17. Automated Consolidation Recommendations
Automated consolidation recommendations involve AI analyzing inventory data to suggest when and where to merge partial stocks so that storage space can be freed. In many warehouses, especially over time, you end up with partially filled pallets or bins – for example, two half-pallets of the same product sitting in different locations. These situations waste space. AI looks for such opportunities and recommends consolidating them into one location. It can also identify when multiple small orders or shipments can be combined into one, reducing the number of pallets or containers used. Implementing these recommendations means that rather than having goods spread out thinly across many slots, the warehouse can condense them into fewer slots, opening up empty locations for other uses. The process might be dynamic: each day or week, the system could output a list of “consolidation tasks,” like move product A from location X to join the same product in location Y. By automating the detection of these opportunities, warehouses ensure that space doesn’t slowly get “lost” to inefficiencies – every location is kept as full as it reasonably can be. This strategy is especially valuable after peak seasons or product phase-outs, when there may be lots of partially empty locations. Ultimately, automated consolidation keeps the storage layout lean and tidy, continuously squeezing out unused space and thereby increasing the warehouse’s effective capacity.
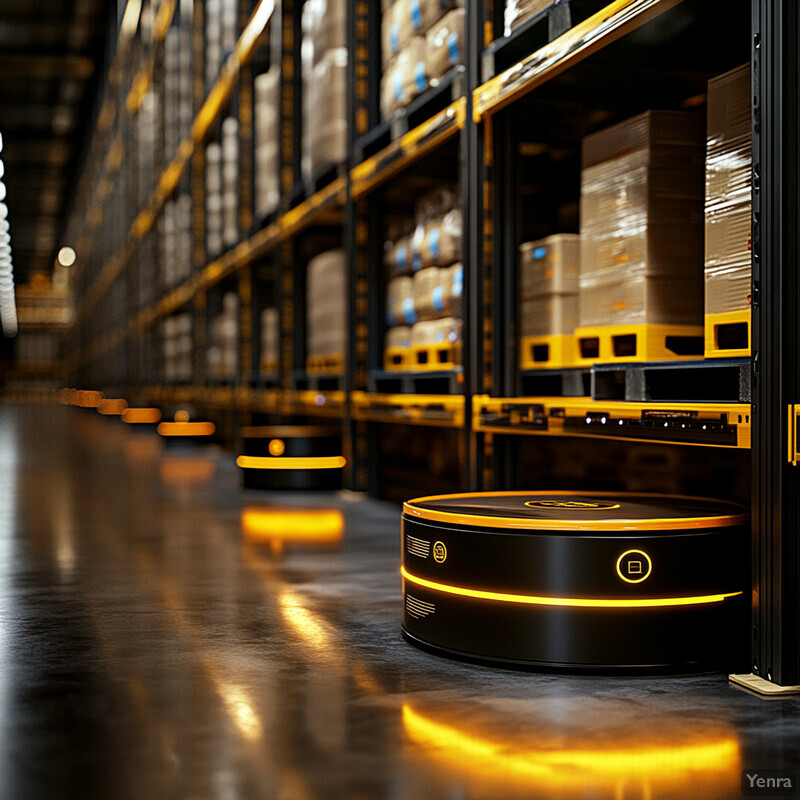
The impact of systematic consolidation can be quite dramatic in reclaiming space. For instance, a case study in a consumer goods distribution center showed that an organized consolidation initiative – guided by advanced inventory software – created about 20–30% more free pallet locations in the warehouse once slow-moving and fragmented stocks were merged blog.rocket-consulting.com . This freed space then became available to house new product lines and fast-movers that had been crowded before. Another example: a fashion retailer’s warehouse, after implementing AI-driven consolidation routines, was able to avoid renting external storage for seasonal overflow because the AI consistently found and cleared up space internally by combining stocks. The ROI on these efforts is high – Rocket Consulting reported a project for a European apparel company that increased available warehouse space by roughly one-third through systematic slot consolidation, directly leading to improved fulfillment speed and the ability to defer a planned warehouse expansion. On the shipment side, companies using AI to consolidate orders or shipments onto shared pallets have cut the number of outbound pallets by 10–15%, which in turn cuts down storage needs in staging areas and lowers transport costs. These results underline that significant portions of warehouse capacity (often on the order of 20%+) can be trapped in underutilized slots, and AI-driven consolidation is key to unlocking that space. By continuously nudging the warehouse to “clean up” partial slots, operations remain space-efficient even as inventory ebbs and flows.
18. End-to-End Integration With Supply Chain Data
End-to-end integration means connecting the warehouse’s systems with data from across the entire supply chain – suppliers, transportation, retail outlets, and even customers – to make more holistic decisions about space and inventory. When a warehouse operates in isolation, it might receive a big batch of stock unexpectedly and scramble to find space, or hold onto excess inventory “just in case” due to lack of downstream visibility. With integration, the warehouse knows what’s coming in advance (because it’s tied into supplier production data and inbound transport schedules) and what’s going out (through real-time sales or distribution data). AI can then use this rich data to optimize how space is utilized: for example, delaying a planned replenishment if store sales are slow (avoiding overstock and saving space), or pre-positioning inventory in the warehouse ahead of a promotion because marketing data is integrated. Additionally, integration with upstream data might signal quality issues or recalls, allowing the warehouse to quarantine stock efficiently. From a space perspective, the warehouse essentially becomes a transparent node in a larger network – AI looks at the whole network’s needs and guides local space usage accordingly. This could mean dynamically adjusting safety stock levels (lowering them if supply lead times improve, freeing space) or cross-docking incoming goods directly to outbound staging if an order is pending (bypassing storage). Overall, end-to-end data integration ensures the warehouse’s space is used in harmony with supply and demand conditions beyond its four walls, reducing buffers and smoothing flows.
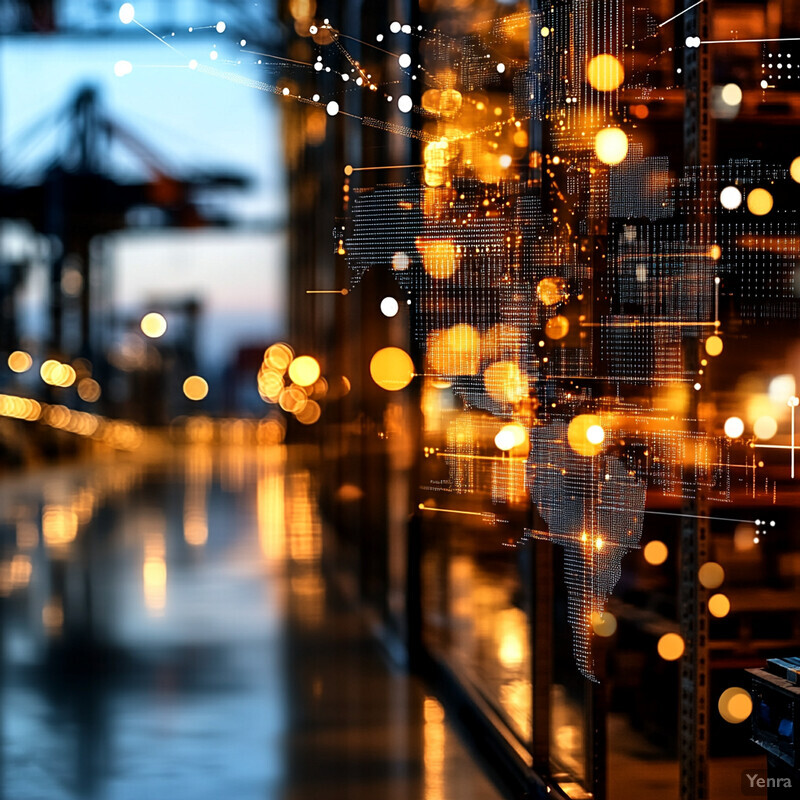
The benefits of a fully integrated, AI-supported supply chain are reflected in key performance metrics. One example is an AI-enabled “control tower” system implemented by a distributor that integrated data across procurement, warehousing, and distribution – this system improved inventory placement so well that order fill rates rose by about 5–8% while excess stock (and its space usage) dropped markedly. The improvement in fill rate indicates better alignment of inventory with demand at each location, a direct result of end-to-end visibility and coordination. In general, McKinsey notes that AI-driven planning and inventory optimization can reduce overall inventory (and required storage space) by 20–30% across a network. Integration is a prerequisite for such network-wide optimization. The industry is moving this way quickly: according to recent surveys, 74% of supply chain leaders are increasing investments in technologies that connect supply chain nodes and enable unified data visibility (often citing agility and space efficiency as outcomes). Additionally, the adoption of automation like ASRS is often paired with systems integration – about 73% of new ASRS deployments are being directly integrated with warehouse management and ERP systems to ensure real-time data exchange. This tight integration means the moment a sale happens or a shipment is delayed, the warehouse AI knows and can adjust. The result is less “just in case” inventory hogging space and more “just in time” flow. Companies practicing this report, for example, that they avoided building new warehouses because their existing ones, under integrated management, could handle more throughput with less idle stock. In summary, sharing data up and down the supply chain allows the AI to orchestrate space usage with precision, yielding higher service levels and lower space requirements simultaneously.
19. Continuous Cost-Benefit Analysis of Storage Arrangements
This approach means that the warehouse, aided by AI, is constantly evaluating whether the current storage setup is the most cost-effective or if changes are warranted. It’s not static – rack layouts, slot assignments, and process flows are regularly reviewed against their performance. AI can assign a “cost” to every empty slot, extra travel footstep, or rehandling of product, and a “benefit” to every optimization (like faster picks or deferred capital expense). By doing so continuously, the system identifies when the gains from a reconfiguration outweigh the disruption or implementation cost. For example, if order patterns shift and a certain aisle is now underutilized, AI might flag that consolidating and removing that aisle (to create room for something else or narrow the warehouse footprint) would save money – essentially a mini cost-benefit analysis for removing an aisle. Conversely, if a proposed change (say, moving to a new picking technology) would cost more in labor and downtime than it saves in space, the AI’s analysis would advise against it. Continuous analysis also encompasses ROI tracking: if the warehouse invested in a new automation or layout change, AI monitors whether the expected savings (labor, space, accuracy) are actually being realized, and if not, why. This prevents warehouses from sticking with suboptimal setups for too long, because the AI will surface when the cost of “doing nothing” has grown higher than the cost of making a change. In essence, every decision about space – be it adding mezzanine flooring, rearranging zones, or implementing a new slotting strategy – is supported by a live cost-benefit calculation, ensuring resources are used wisely and improvements are made at the right time.
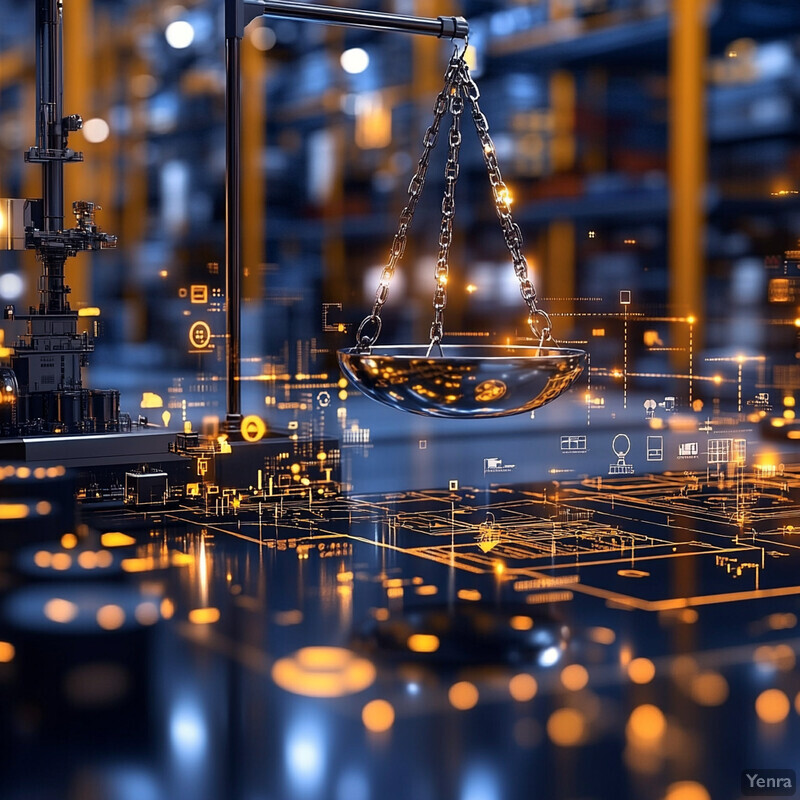
The value of rigorously weighing costs and benefits is evident in the performance of companies that do it. Leading warehouse operators who systematically evaluate and amplify the ROI of their storage and automation decisions have achieved 20–50% improvements in service levels (e.g., order fulfillment speed) while cutting fulfillment costs by 25–50%. This was reported by BCG in an analysis of top-tier retail distribution centers – those companies didn’t necessarily spend more on automation or space, but they targeted the investments and changes that had clear, quantified payback, and continually adjusted course when the data showed diminishing returns. In practice, continuous cost-benefit analysis might manifest as quarterly reoptimizations: one 3PL warehouse noted that after implementing an AI review process, it made small layout tweaks every quarter (move a section of racks, change a picking method in one zone), each time saving around 2–3% in operating costs; cumulatively, over two years, these tweaks added up to more than a 15% reduction in cost per order, directly impacting the bottom line. Warehouses that lack this practice often fall prey to “analysis paralysis” or inertia – by the time they realize a change is needed, they might have already lost money or space efficiency for months or years. By contrast, an agile, AI-informed approach quickly identifies when the benefit of a re-slotting or re-layout outweighs the short-term cost, leading to frequent minor improvements rather than infrequent major overhauls. This also boosts resilience; for example, when the pandemic drastically changed product mix, warehouses with continuous analysis pivoted their layouts in weeks (guided by cost-benefit data) whereas others took months. In sum, the warehouses that treat their configuration as an evolving asset – with AI constantly minding the trade-offs – tend to outperform those that set and forget their arrangements.
20. Proactive Obsolescence Management
Proactive obsolescence management involves using AI to predict and identify products that are becoming slow-moving or unsellable (dead stock) and then taking action to remove or repurpose them before they occupy valuable space for too long. This might include items reaching expiration dates, outdated models (like last year’s electronics), or simply SKUs that haven’t had any picks in months. AI can flag these items by analyzing sales and usage trends, and even compare against external data (like market trends suggesting a product line is being discontinued). Once identified, the warehouse can act proactively: mark them for clearance sale, donate or recycle them, or return them to the supplier if possible. The key is to free up the space they occupy for more productive inventory. Rather than reacting once a year with a massive clearance, the warehouse continuously culls obsolescent stock. This keeps inventory fresh and space available. It also has financial benefits – by addressing obsolescence early, companies can get better recovery value (selling at a smaller discount rather than scrapping entirely). In essence, the warehouse’s AI brain is always asking: “Should this item still be here?” If the answer is no, it prompts removal. This ensures that prime warehouse real estate isn’t tied up by products that aren’t contributing to revenue, which is especially important in high-cost warehouses. Over time, proactive obsolescence management maintains a leaner, more current inventory mix and prevents the accumulation of “junk” that plagues many long-running warehouses.
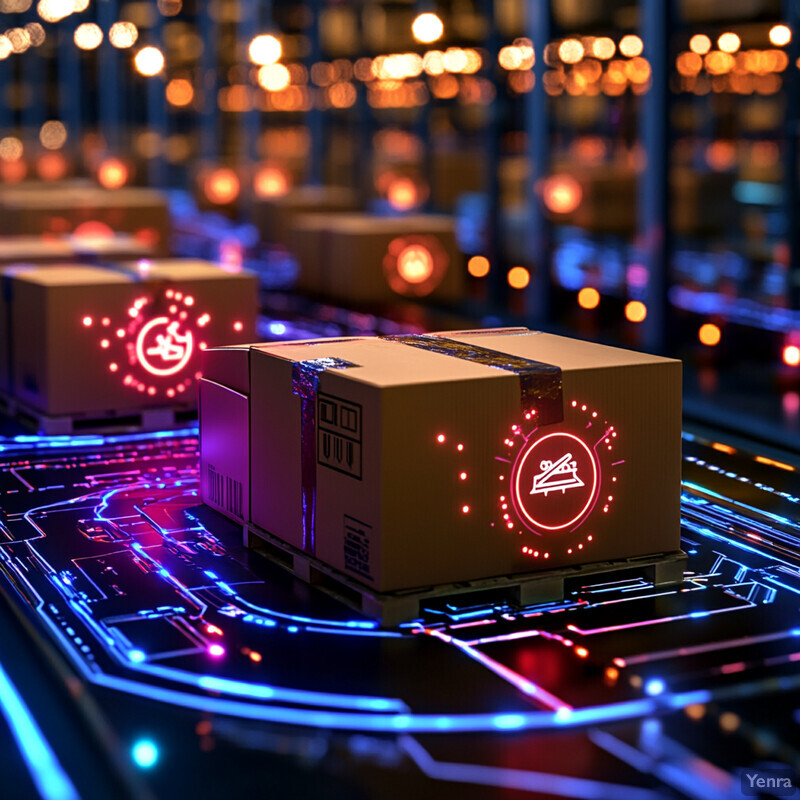
The scale of the dead stock problem in warehouses is significant – even well-run companies might find that 20–30% of their inventory is effectively dead or obsolete at any given time, not contributing to sales. This stock not only ties up capital but also consumes valuable warehouse space (often 20%+ of floor or rack space) that could be used for fast-moving products. The cost of this is steep: carrying obsolete inventory incurs storage costs (rent, utilities for that space) and can force companies to acquire additional storage capacity unnecessarily. Industry analysis shows that excess and obsolete inventory can inflate warehouse operating costs by as much as 25–30% when considering all factors (space, handling, insurance, damage, etc.). Proactive management backed by AI cuts these losses. For example, one retailer implemented an AI tool to predict product end-of-life; as a result, they initiated markdowns and vendor returns 3–6 months earlier than before, clearing out aging stock. This freed up 15% of their DC’s shelf space, which was then reallocated to new, in-demand SKUs – boosting sales per square foot. Financially, they also avoided an estimated 20% write-off that would have occurred had those products stayed unsold until total obsolescence. Another case saw a B2B distributor use AI to flag parts with no sales in 12 months; they consolidated and removed these, freeing a full aisle of shelving, and then repurposed that aisle for a new product line that generated millions in revenue. These examples underscore that actively removing or remarketing obsolete stock – guided by AI detection – directly translates to recovered space and cost savings. It’s essentially addition by subtraction: getting rid of the “dead weight” allows the productive inventory to thrive in the space it leaves behind.