1. Improved Crop Yield Prediction
AI models can correlate spectral data, vegetation indices, soil characteristics, and weather patterns from satellites to accurately predict future yields, enabling better harvest planning and supply chain adjustments.
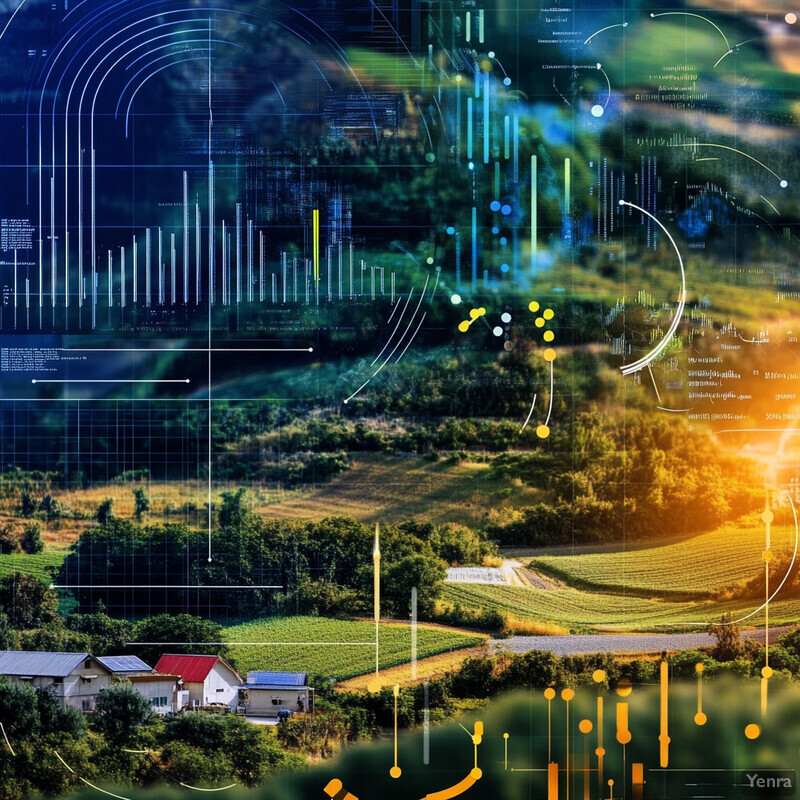
By integrating satellite-derived indicators—such as Normalized Difference Vegetation Index (NDVI), Enhanced Vegetation Index (EVI), and thermal infrared data—with ground-based agronomic, meteorological, and historical production records, AI-driven models can accurately forecast future crop yields well in advance of harvest. These predictive models leverage machine learning algorithms to uncover subtle correlations among spectral signatures, soil moisture levels, plant health conditions, and weather patterns. As a result, farmers and agricultural stakeholders can make more informed decisions about planting dates, seed varieties, fertilizers, and resource allocation, while traders and policymakers gain valuable insights for managing inventory, ensuring market stability, and formulating proactive agricultural policies.
2. Automated Land Cover Classification
Advanced machine learning algorithms classify land use and land cover at scale, distinguishing between crop types, forested areas, and water bodies, reducing manual labeling costs and improving mapping accuracy.
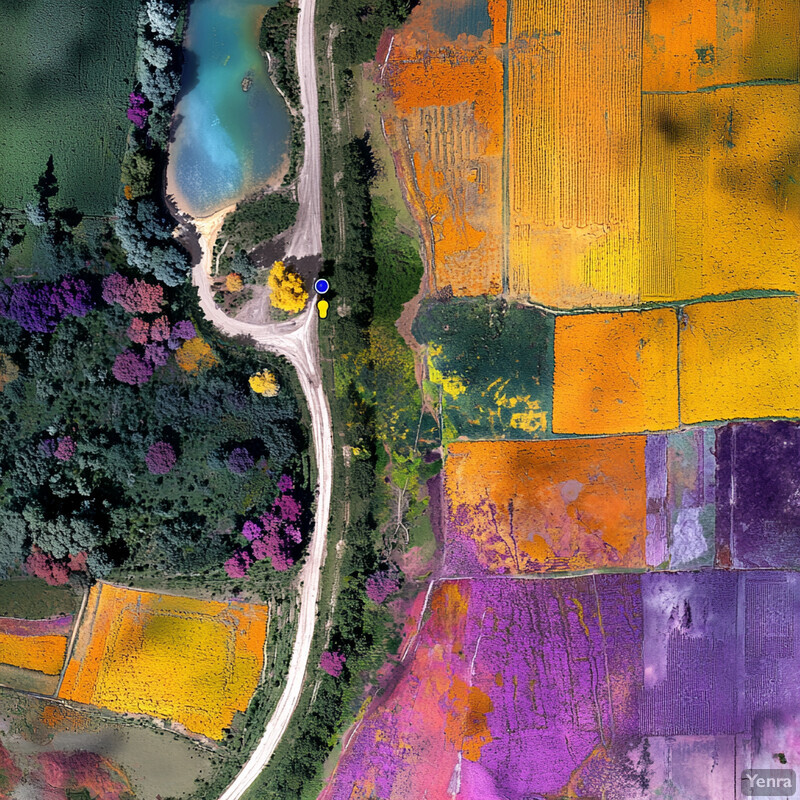
AI-powered remote sensing techniques have revolutionized the classification of agricultural landscapes. Traditional methods of mapping land cover types—such as forest, cropland, pasture, or water—were often labor-intensive and error-prone. Now, machine learning and deep learning algorithms process large volumes of high-resolution satellite imagery to automatically discern land cover categories. By comparing pixel-level spectral and textural information, these algorithms can differentiate between crop types, detect fallow fields, and track changes in land use over time. This information assists farmers, land managers, and governments in better understanding land distribution, verifying agricultural subsidies, monitoring environmental compliance, and planning sustainable land-use strategies.
3. Dynamic Crop Health Monitoring
By analyzing multispectral and hyperspectral imagery, AI can detect subtle changes in plant reflectance patterns to identify early signs of stress, diseases, or nutrient deficiencies, prompting timely interventions.
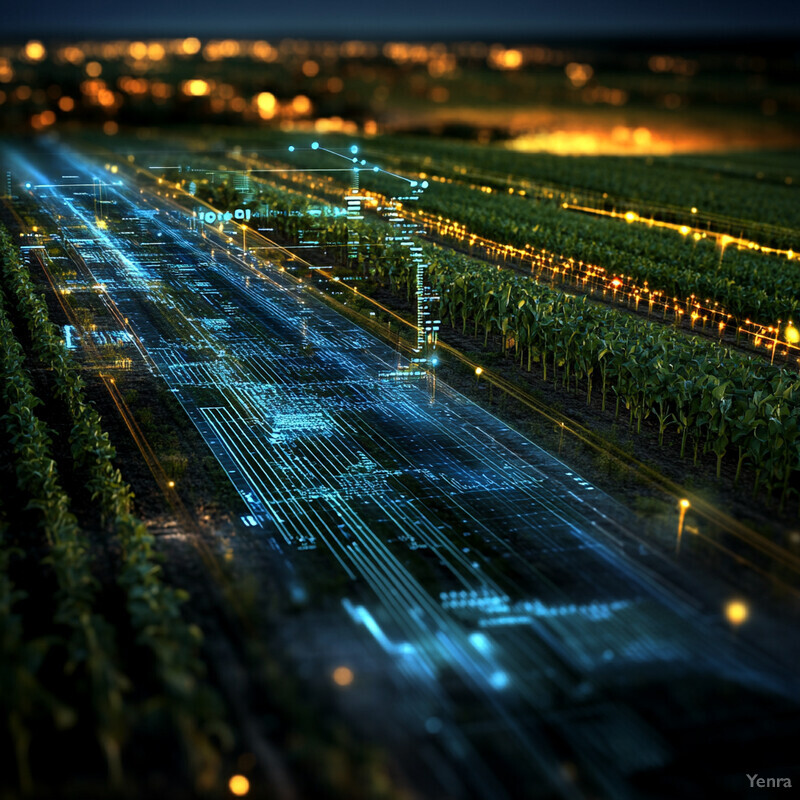
AI enhances the detection and monitoring of crop health through continuous analysis of spectral and thermal data from satellites. Early signs of stress—whether from nutrient deficiencies, water scarcity, pests, or pathogens—manifest in subtle changes in reflectance patterns that machine learning models can detect before they become visually apparent. These insights enable precision interventions, such as targeted fertilizer application, efficient irrigation, or timely pesticide treatments, minimizing both crop losses and environmental damage. As a result, farmers can maintain healthier fields, increase yield stability, and reduce the overall costs associated with broad-based or reactive interventions.
4. Soil Moisture Estimation
Deep learning techniques integrate thermal bands, radar backscatter data, and optical signals from satellites to estimate soil moisture content, informing irrigation scheduling and improving water resource management.
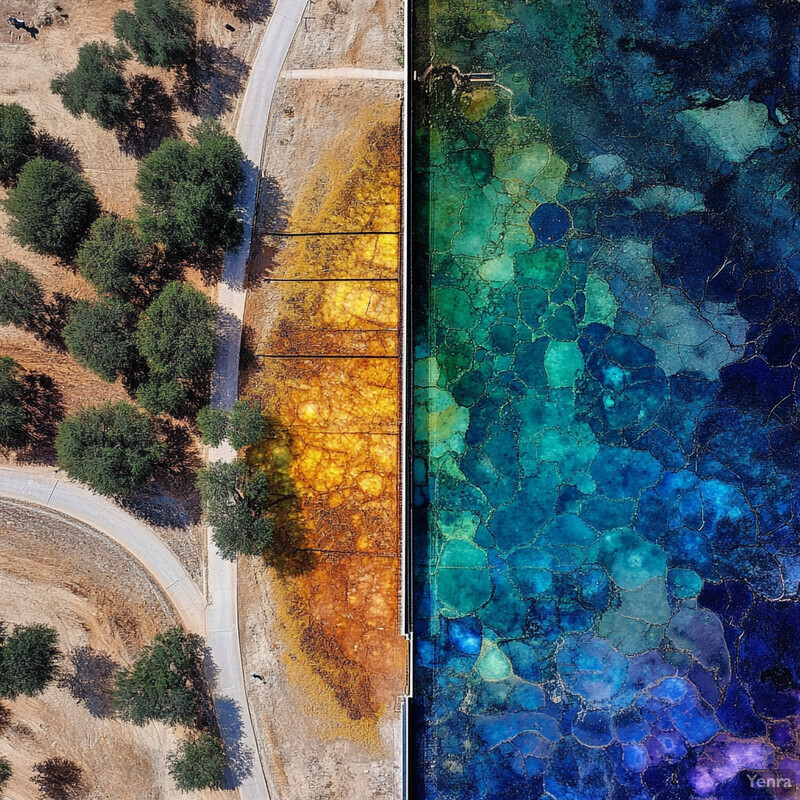
Timely and accurate soil moisture information is essential for irrigation planning and efficient water use. AI models now integrate optical, radar, and thermal satellite data with local weather forecasts and historical records to estimate soil moisture at different depths and scales. By doing so, they help farmers understand the best times and amounts of irrigation required. This synergy of data sources informs precision agriculture techniques, ensuring that water is conserved without compromising crop growth. It also helps mitigate the impacts of drought, reduces water wastage, and leads to more sustainable farming practices, particularly in regions with scarce freshwater resources.
5. Precise Pest and Disease Outbreak Alerts
AI models trained on extensive libraries of historical crop health imagery can identify patterns that precede pest or disease outbreaks, enabling farmers to take preventive steps before the problem escalates.
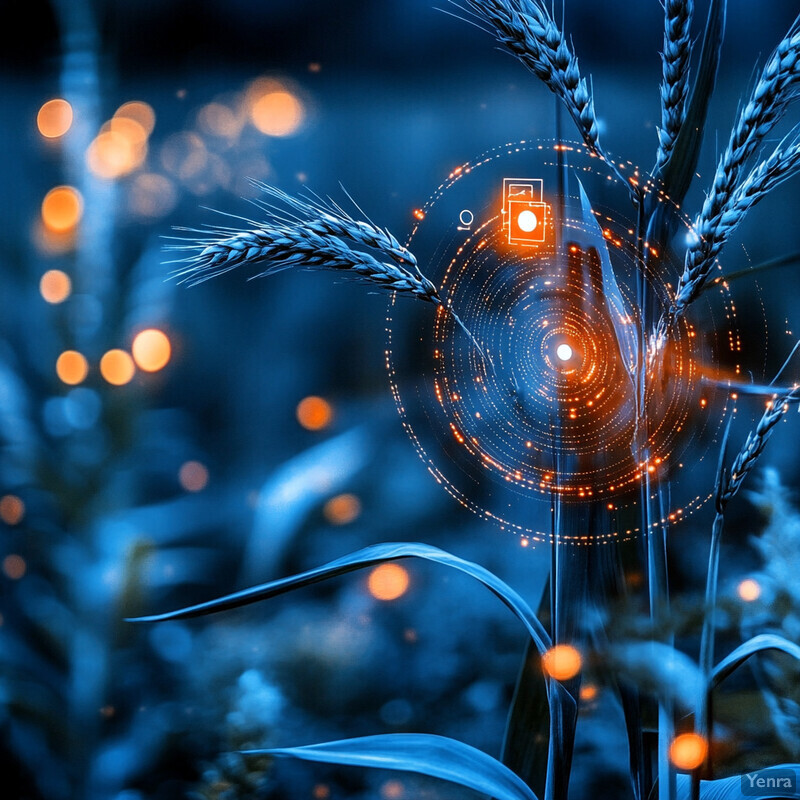
AI tools can identify patterns in historical and current satellite-based vegetation indices that correlate with impending pest or disease outbreaks. Through pattern recognition and anomaly detection, machine learning models pinpoint areas where plant stress indicators—such as leaf discoloration or abnormal growth—could signal the onset of pests or pathogens. By providing early warnings, farmers gain the opportunity to implement preventive measures—like targeted pesticide applications, biological controls, or quarantines—before pests spread and cause extensive yield losses. This proactive approach reduces crop damage, supports integrated pest management strategies, and minimizes the environmental footprint of chemical interventions.
6. Weed Detection and Differentiation
Using high-resolution satellite imagery and convolutional neural networks, AI can distinguish weeds from crops at scale, helping in targeted herbicide application or mechanical removal strategies.
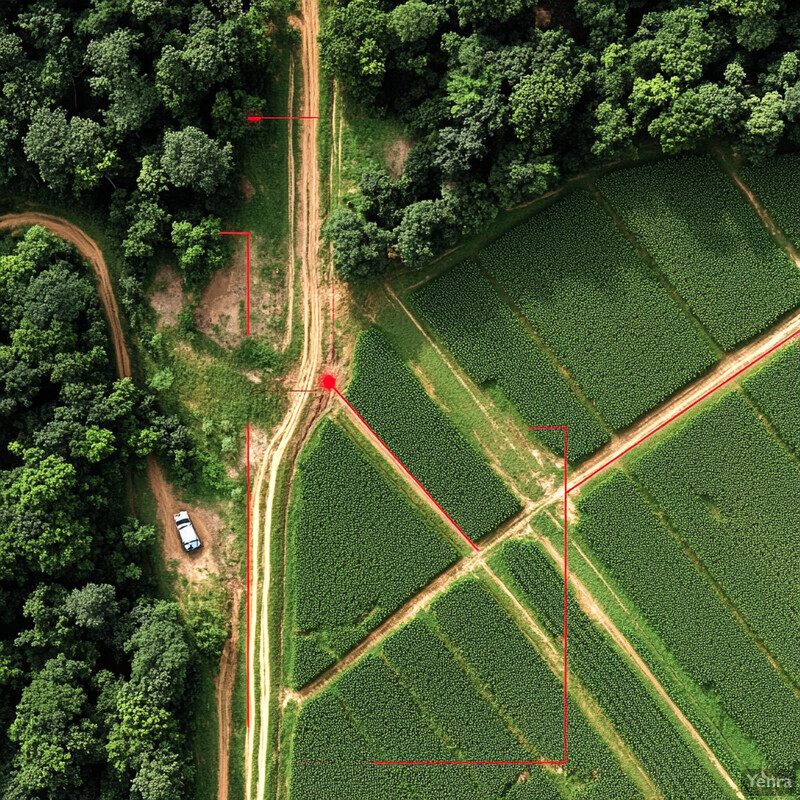
Weeds compete with crops for nutrients, water, and light, and timely removal is crucial for maintaining high yields. AI-based weed detection leverages high-resolution satellite imagery to distinguish weeds from the desired crop canopy. Using convolutional neural networks (CNNs) trained on large image datasets, the technology identifies patterns of leaf shape, texture, and spectral response. As a result, farmers can apply herbicides more selectively or employ mechanical weeding techniques with pinpoint accuracy. By reducing broad-spectrum spraying, farmers lower their chemical usage and operational costs, while also contributing to healthier soils and surrounding ecosystems.
7. Optimized Fertilizer Application
By correlating plant nutrient uptake patterns with satellite-derived vegetation indices, AI tools help farmers apply fertilizers only where needed, improving efficiency and reducing environmental impact.
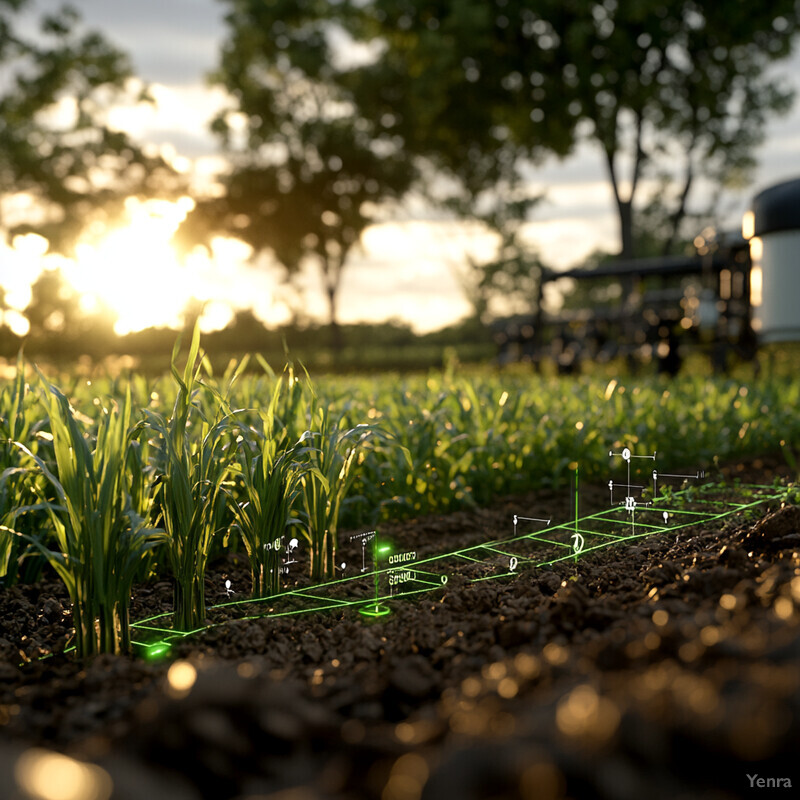
Fertilizer misapplication wastes resources, increases costs, and can harm the environment. AI models mitigate these risks by using satellite data to map variations in plant health and nutrient uptake across a field. By correlating vegetation indices with soil nutrient profiles, these models highlight areas that require more or less fertilization. Farmers can then adjust the type, timing, and volume of fertilizer to match the crop’s needs. This precision approach improves nutrient use efficiency, bolsters yield quality, and lessens runoff and leaching into water systems, fostering both economic and environmental benefits.
8. Accurate Yield Estimation at Various Scales
Machine learning models combine satellite data with agronomic and meteorological inputs to generate accurate yield forecasts from plot-level to regional scales, assisting policy makers and agribusinesses in strategic planning.
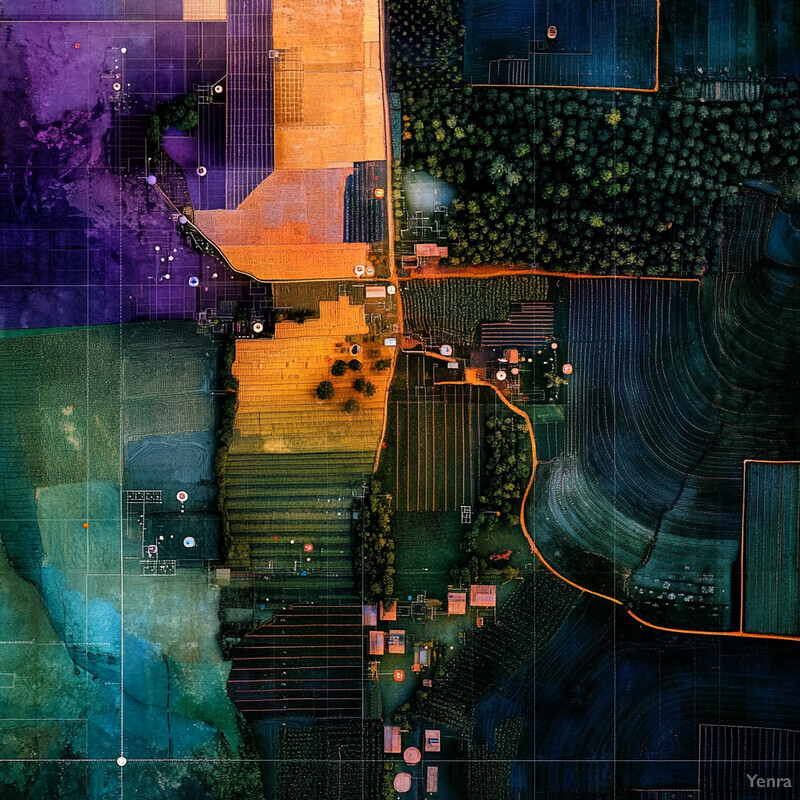
From individual fields to entire regions, accurate yield estimation informs policy decisions, supply chain management, and commodity market projections. AI systems integrate a multitude of data—satellite-derived vegetation indices, climate records, soil data, and on-ground observations—to model yield outcomes across spatial scales. These models can estimate harvest quantity, guide infrastructure planning (like storage and transport), and inform insurance models and risk assessments. Improved yield estimation bolsters food security by enabling better inventory planning, price stabilization, and efficient resource distribution, ensuring that supply chains remain resilient in the face of environmental uncertainties.
9. Field Boundary and Farm Plot Detection
AI-driven segmentation algorithms can identify exact field boundaries from satellite imagery, aiding in farm registration, subsidies, compliance monitoring, and farmland valuations.
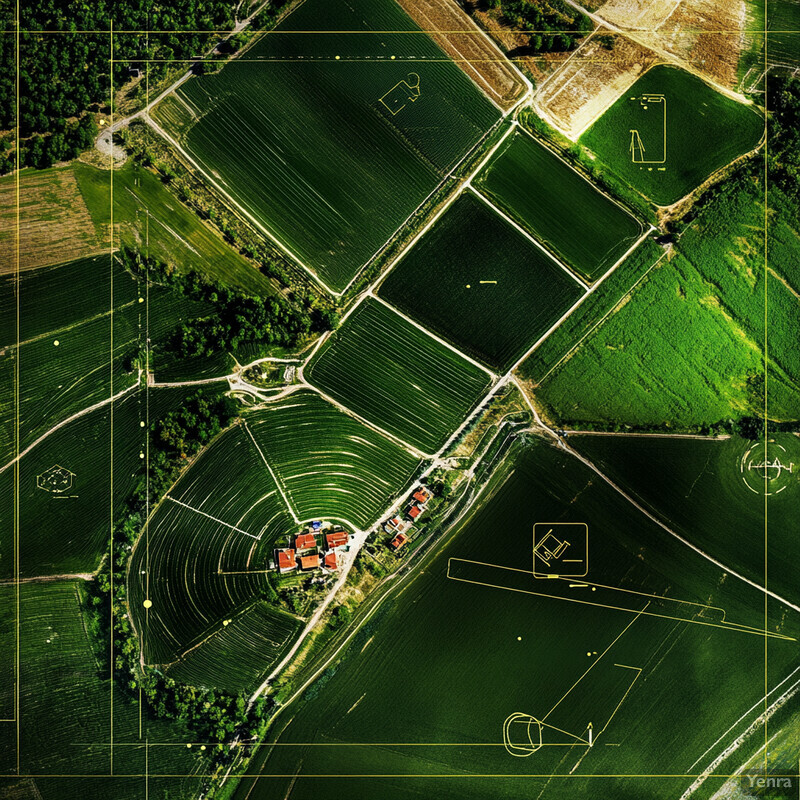
Accurate delineation of field boundaries is critical for land registration, subsidy allocation, and monitoring agricultural practices. AI-driven segmentation algorithms, using satellite imagery, can identify and map farm plots to a high degree of accuracy. By recognizing edges defined by differences in crop type, vegetation density, or soil conditions, these tools replace manual digitization methods that are costly and prone to human error. The clear mapping of plots facilitates precision agriculture applications, ensures fair and transparent allocation of government subsidies, and streamlines auditing and regulatory compliance procedures.
10. Evaluating Crop Rotation and Soil Health Patterns
By examining historical imagery, AI can identify the most effective crop rotation patterns and analyze long-term soil health trends, guiding sustainable land management practices.
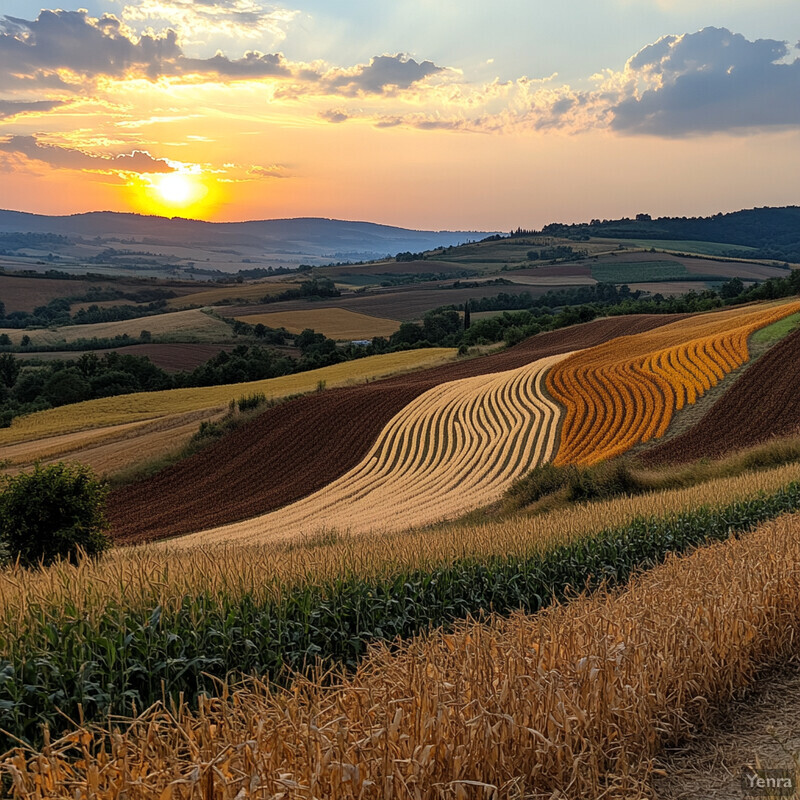
Sustainable agriculture hinges on well-planned crop rotations that maintain soil fertility and prevent disease buildup. By analyzing multi-year satellite imagery, AI algorithms can detect patterns in crop sequences and correlate them with soil health indicators—such as organic matter content, erosion rates, or nutrient availability. This historical perspective helps farmers identify the most beneficial rotational strategies, balanced planting frequencies, and complementary crop combinations. Over time, informed rotation decisions lead to healthier soils, increased biodiversity, enhanced crop resilience, and more stable long-term yields.
11. Real-Time Drought Monitoring
Using satellite-based measures of evapotranspiration, soil moisture, and vegetation stress, AI systems can produce near real-time drought risk maps, helping stakeholders allocate resources effectively.
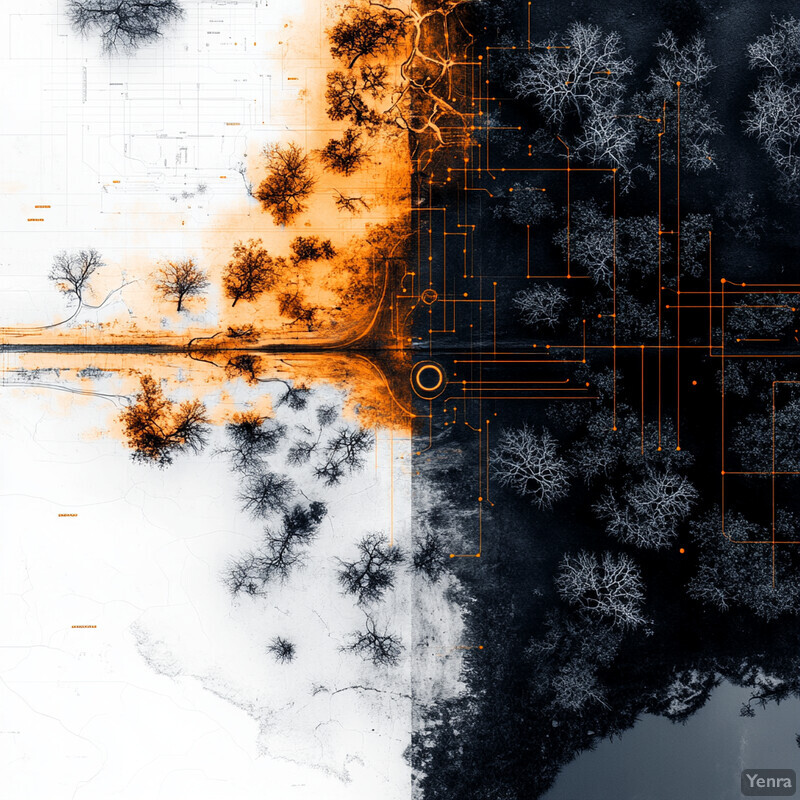
Drought conditions can emerge swiftly, severely impacting crop production and water resources. AI-enhanced satellite analysis offers near real-time assessments of vegetation stress, soil moisture depletion, and evapotranspiration rates. By integrating these factors into drought indices, machine learning models produce timely maps indicating which regions or fields are at greatest risk. Stakeholders—ranging from farmers to emergency planners—use this information to prioritize irrigation, allocate relief funds, implement water-saving measures, and develop contingency plans. Early warnings and spatially explicit drought insights mitigate economic losses and support more resilient agricultural systems.
12. Enhanced Food Supply Chain Traceability
By connecting remote sensing data to GIS-based farm management systems, AI can track crop growth stages and harvesting dates, improving supply chain transparency and food traceability.
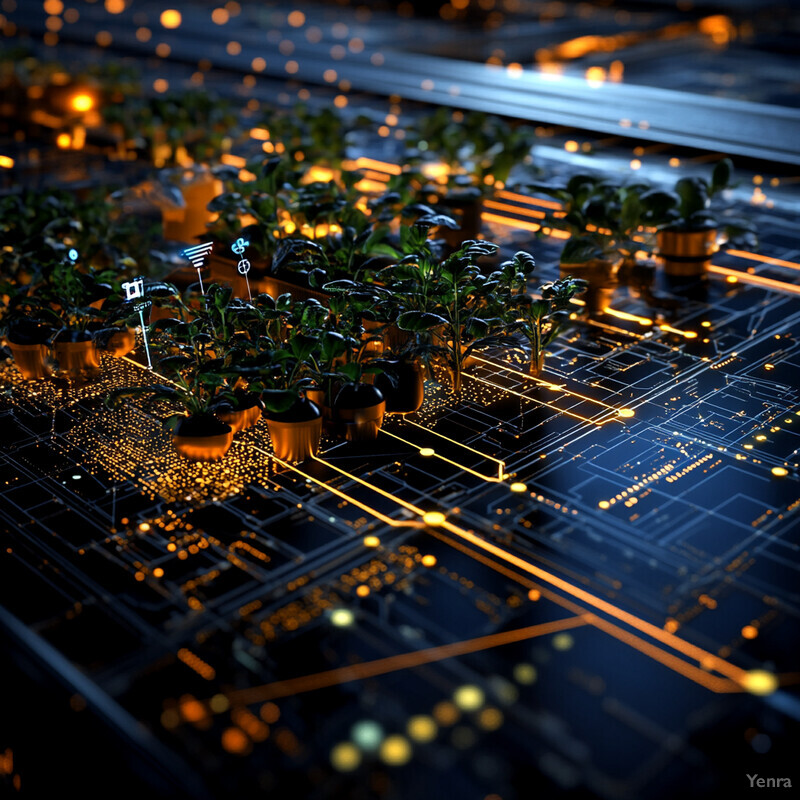
Global supply chains rely on accurate, transparent information about the origins and conditions of agricultural produce. AI-driven satellite analytics, when linked to geographic information systems and farm management software, can verify where crops were grown, track their growth stages, and estimate harvest windows. This level of traceability builds consumer trust, meets regulatory compliance, and enables rapid responses to quality issues or contamination events. It also empowers retailers and distributors to optimize logistics, reduce waste, and ensure consistent product quality, thus fortifying trust and sustainability throughout the food supply chain.
13. Estimating Carbon Sequestration in Agricultural Lands
Through AI-driven analysis of biomass trends and vegetation changes, satellite data can help estimate carbon stocks and monitor practices that enhance soil carbon sequestration, supporting climate-smart agriculture.
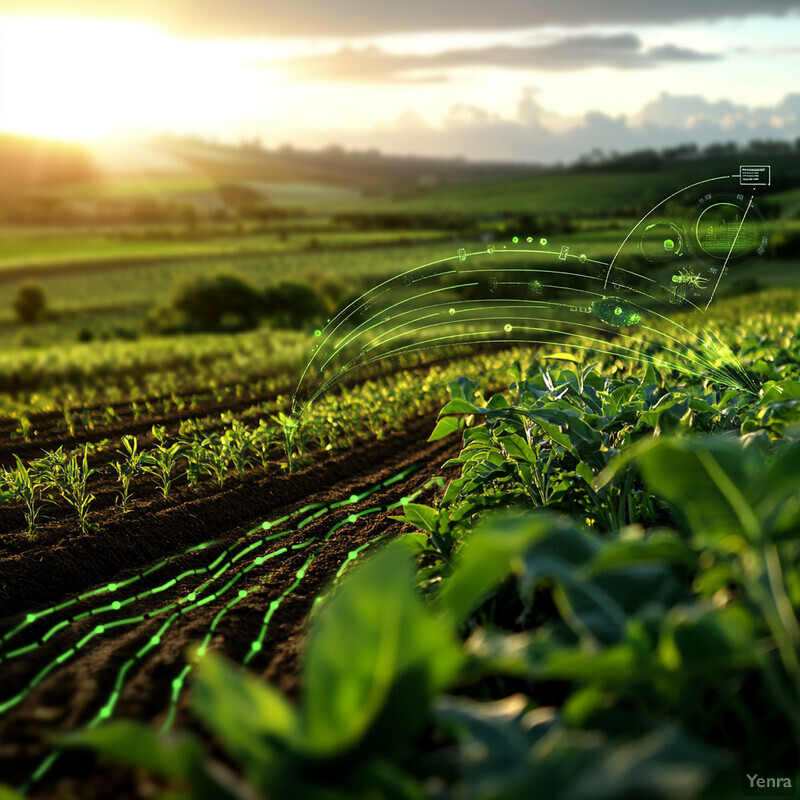
As agriculture is both a source and sink for greenhouse gases, AI-supported analysis of satellite data is instrumental in estimating carbon sequestration potential. By monitoring biomass accumulation, vegetation changes, and soil organic matter indicators derived from spectral signatures, AI models can approximate how much carbon farmland stores over time. This quantification assists in designing and validating climate-smart agriculture practices such as cover cropping, reduced tillage, and agroforestry. Policy makers and environmental groups can use these estimates to inform carbon credit systems, incentivize sustainable practices, and track progress toward climate mitigation goals.
14. Forecasting Market Trends and Pricing
Combining satellite-derived yield forecasts with economic models, AI can help predict market fluctuations and guide pricing strategies for commodities, benefiting farmers, traders, and policymakers.
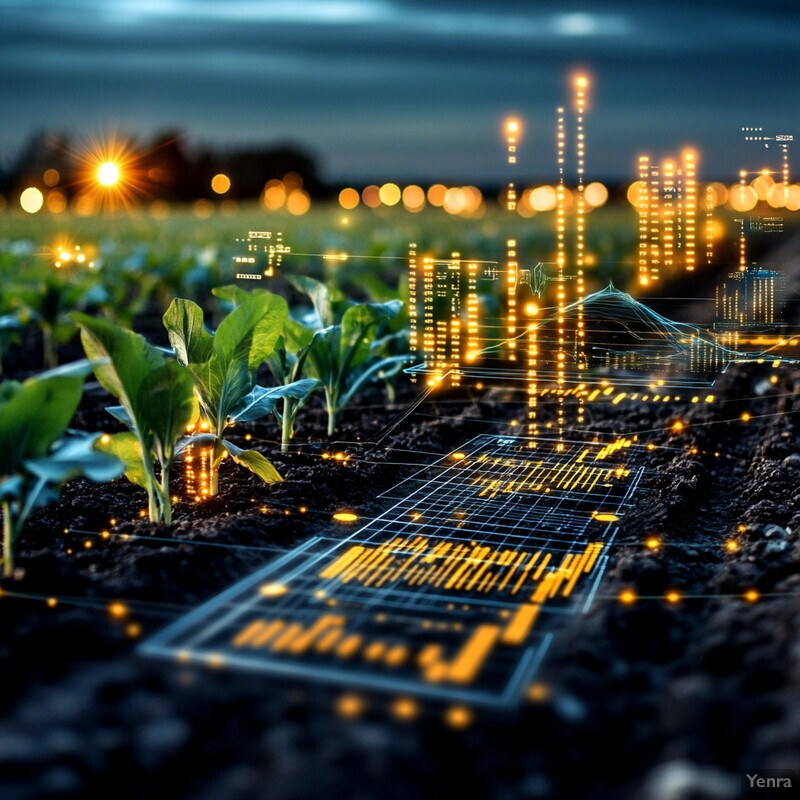
By merging yield forecasts generated from satellite data with economic and historical market variables, AI models can anticipate supply fluctuations and influence crop prices. These projections help farmers decide when to sell their produce, guide traders in managing inventories, and inform policymakers about impending supply surpluses or shortages. Early price signals also enable governments and cooperatives to develop strategic food reserves and stabilize markets. As a result, stakeholders can mitigate financial risks, encourage fair pricing, and maintain stability in both local and global agricultural markets.
15. Precision Irrigation Management
By fusing data on soil moisture, crop water needs, and weather predictions, AI can produce irrigation schedules optimized for conserving water while ensuring optimal plant growth.
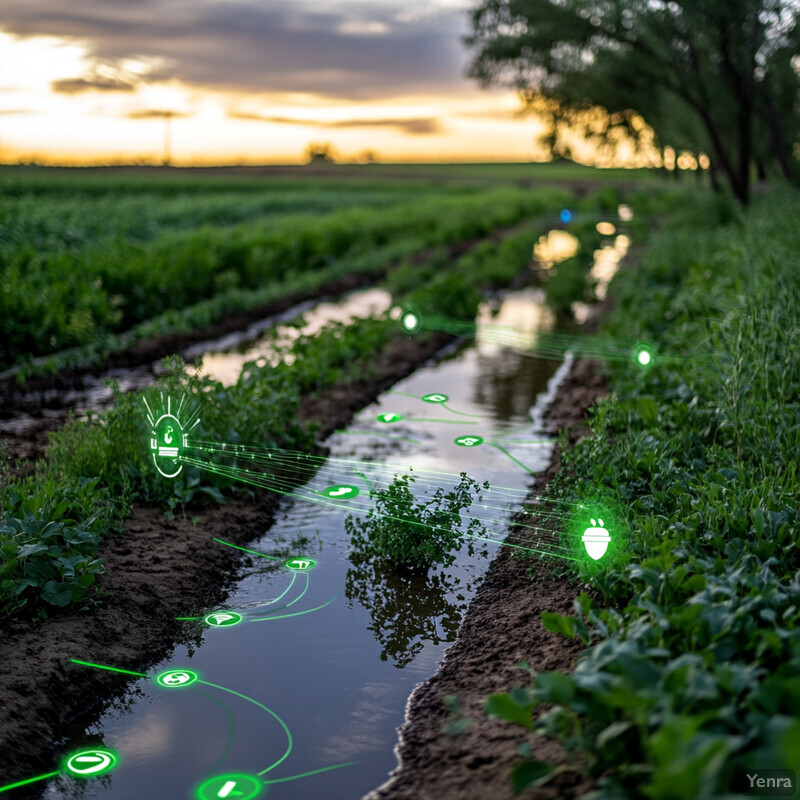
In regions facing water scarcity or erratic rainfall, precision irrigation ensures that crops receive the exact amount of water they need, no more and no less. AI systems integrate satellite-based soil moisture estimates, rainfall predictions, and vegetation indices to inform optimal irrigation timings and volumes. This approach helps farmers conserve water, reduce energy consumption, and maintain consistent crop performance. Beyond environmental benefits, precision irrigation lowers operational costs, mitigates drought impacts, and supports sustainable intensification—growing more with fewer resources.
16. Identifying and Monitoring Agricultural Inputs
AI systems can detect patterns in satellite imagery to gauge where and when inputs like fertilizers or pesticides were applied, improving compliance monitoring and reducing misuse.
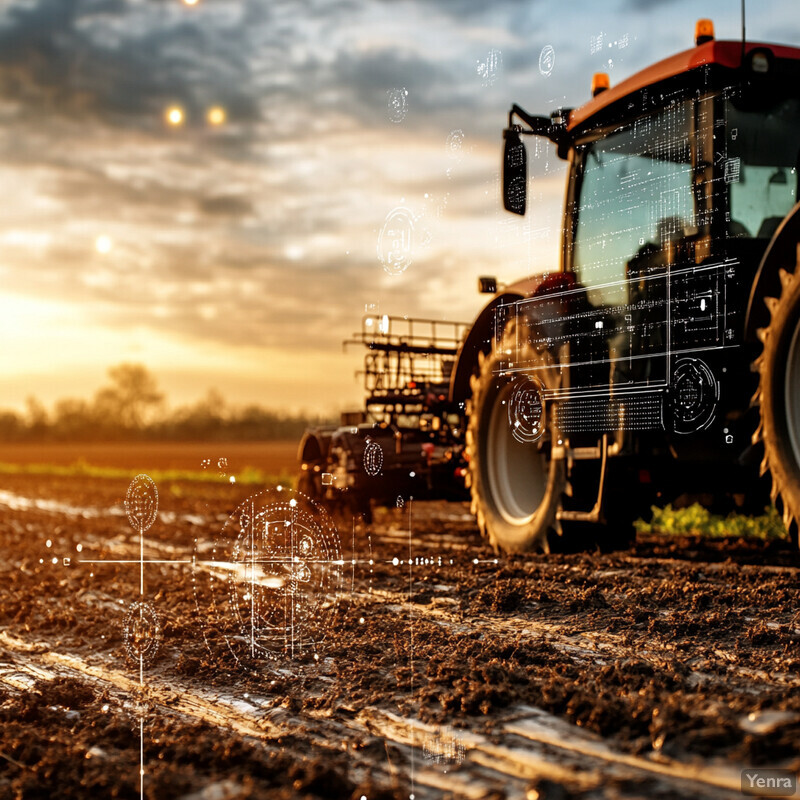
Tracking the use of inputs like fertilizers and pesticides is essential for quality assurance, environmental protection, and regulatory compliance. AI-powered analysis of temporal satellite imagery can detect patterns in vegetation response that correlate with input application events. By identifying these signals, authorities and supply chain partners verify responsible resource management, discourage overuse or misuse of chemicals, and ensure product integrity. This level of monitoring helps maintain soil fertility, safeguards water quality, and upholds standards for organic and sustainable certifications, ultimately benefiting both producers and consumers.
17. Early Detection of Soil Salinity Issues
Satellite-based reflectance and thermal data, combined with AI models, can identify increasing soil salinity before it becomes severe, prompting preventative soil remediation measures.
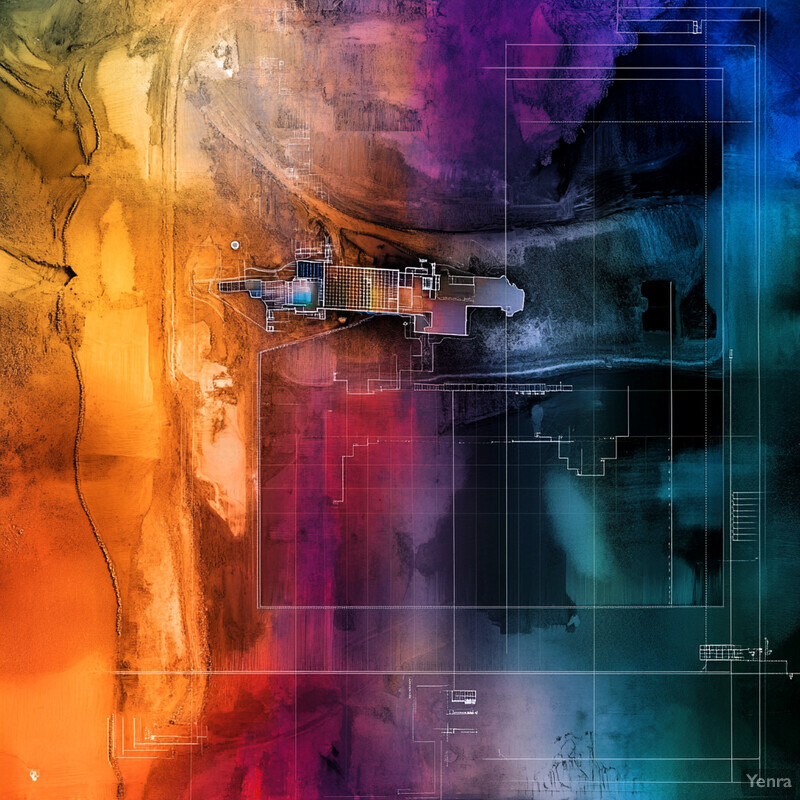
Soil salinity, if undetected, can drastically reduce crop yields and limit long-term agricultural viability. AI models leverage hyperspectral and thermal satellite data to identify early spectral signatures of salinity stress on vegetation and soil surfaces. By catching these subtle changes before they become severe, farmers have the opportunity to implement remedial strategies such as improved drainage, soil amendments, or alternative cropping choices. Early intervention not only saves current harvests but also preserves soil health and productivity for future seasons, contributing to long-term agricultural sustainability.
18. Quantifying Impact of Agricultural Practices on Biodiversity
By analyzing land cover changes and habitat fragmentation, AI can help assess how different farming methods affect local ecosystems, aiding in biodiversity conservation efforts.
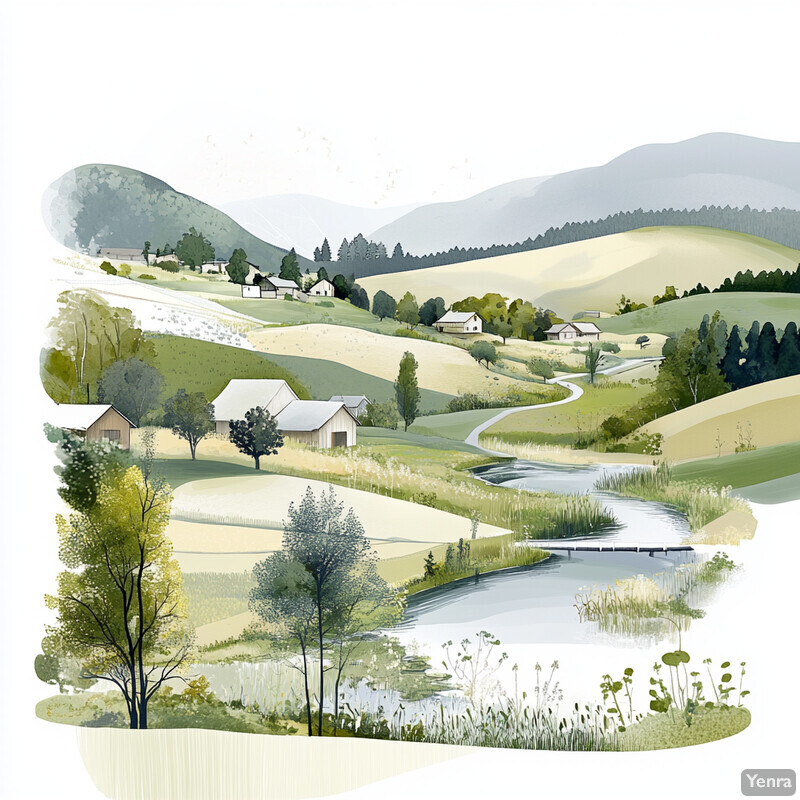
As concerns over biodiversity loss intensify, it’s increasingly important to understand how agriculture shapes local ecosystems. AI-driven land cover analysis from satellite imagery can highlight shifts in habitat connectivity, changes in vegetation structure, and patterns of land use that impact wildlife. By quantifying these ecological changes, stakeholders can assess the effects of tillage, pesticide use, or monocultures on pollinators and other beneficial organisms. Armed with this information, farmers and environmental organizations can adopt practices that balance agricultural productivity with biodiversity conservation, fostering resilient agroecosystems.
19. Near-Real-Time Flood Risk Assessment for Fields
AI-powered interpretation of radar and optical satellite imagery can detect standing water and flood patterns, providing early warning to farmers and enabling quick response to mitigate crop losses.
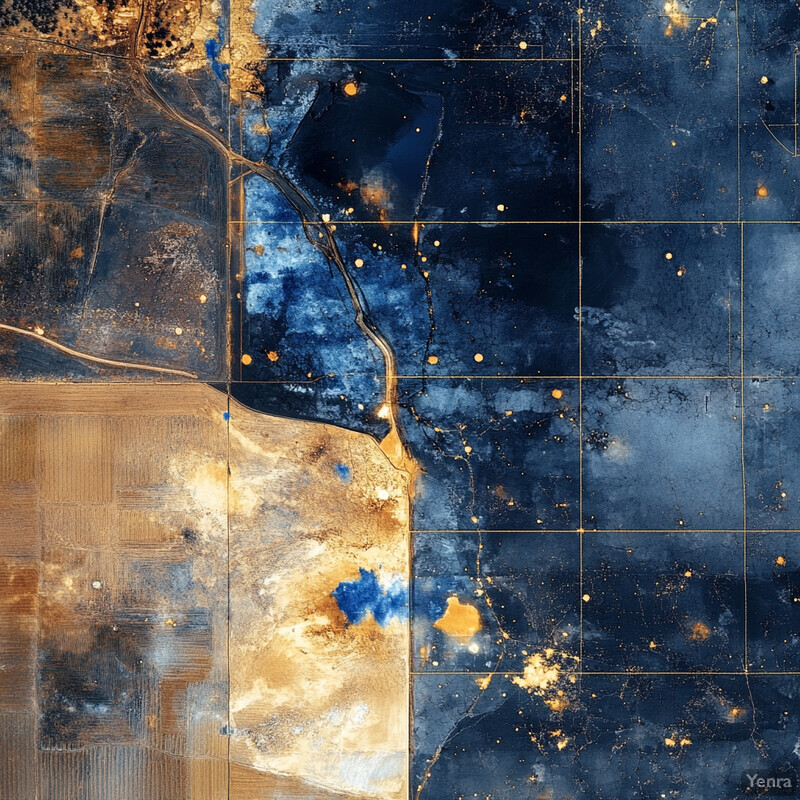
Flooding represents a major threat to agricultural lands, destroying crops, eroding soils, and disrupting planting schedules. AI-enhanced analysis of radar and optical satellite imagery detects standing water, identifies flood extents, and predicts water movement through landscapes. When combined with topographic and meteorological data, these models offer near-real-time flood risk assessments. Farmers and emergency managers can quickly enact protective measures such as relocating equipment, adjusting planting dates, implementing drainage solutions, or procuring insurance coverage. Timely warnings reduce damage and economic losses, safeguarding livelihoods and ensuring more stable food production.
20. Automated Compliance with Agricultural Regulations
Satellite data integrated with AI can verify adherence to policies such as buffer zones, crop rotations, or no-till practices, simplifying compliance checks and enabling sustainable agriculture incentives.
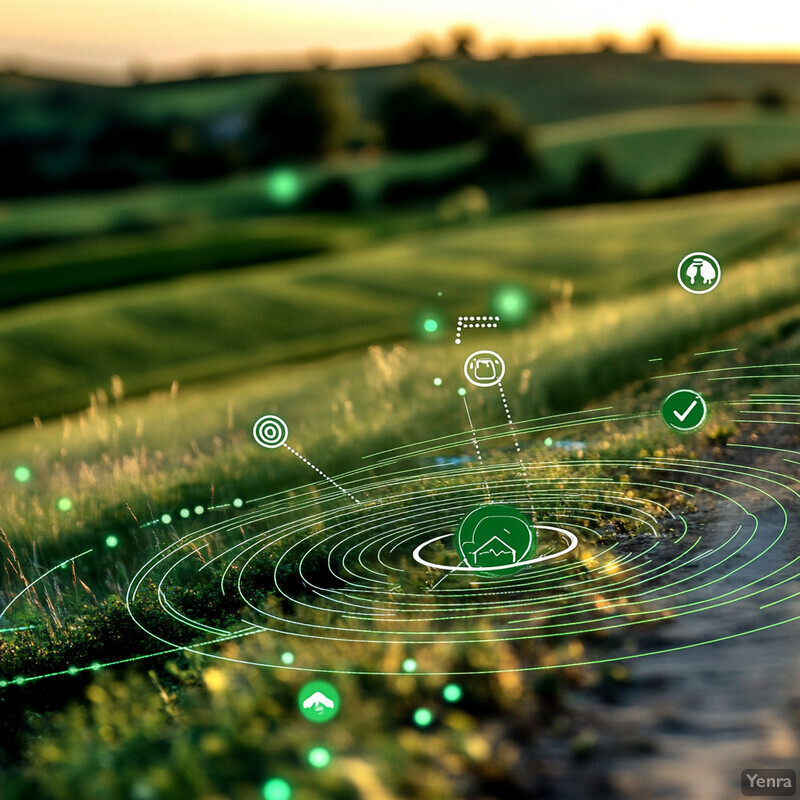
With increasing environmental and safety regulations in agriculture, ensuring compliance can be complex. AI-powered satellite analytics provide objective, consistent, and scalable methods for verifying practices like maintaining buffer zones, adhering to crop rotation requirements, or respecting no-till mandates. By comparing current-year imagery against historical baselines and regulatory maps, these tools flag deviations and compliance breaches. Farmers benefit by avoiding fines, while governments can more efficiently enforce standards and incentivize good stewardship. Ultimately, automated compliance checks reinforce sustainable farming practices, preserving natural resources and public trust in the agricultural sector.