1. Enhanced Disease Surveillance and Early Detection
AI-driven algorithms can process real-time data from medical records, social media, and other sources to identify unusual patterns, enabling earlier detection of disease outbreaks and informing preemptive policy measures.
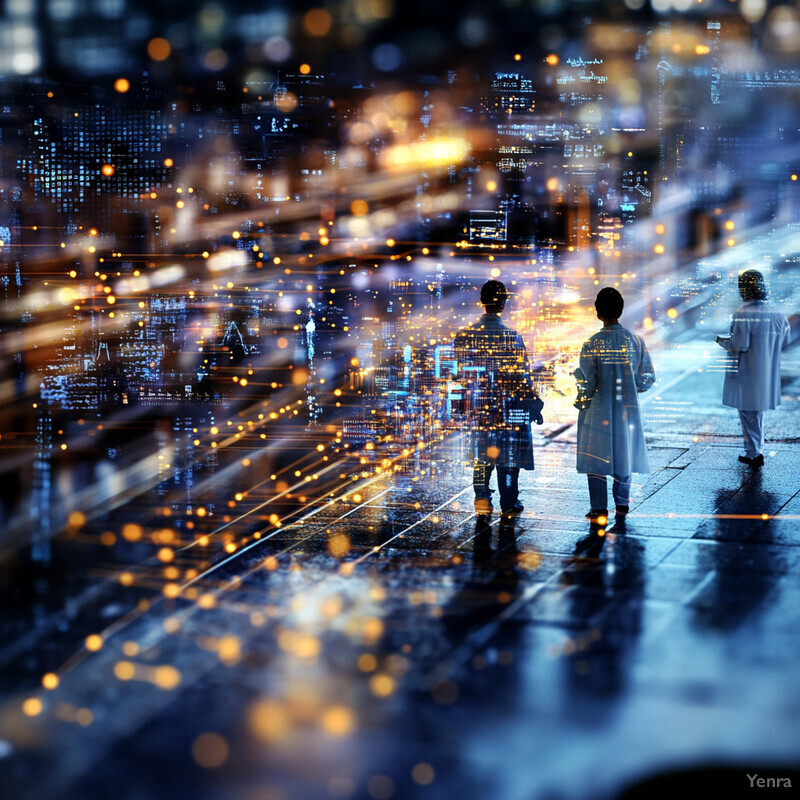
AI algorithms excel at processing large volumes of data in real-time, which allows for continuous surveillance of health indicators from various sources such as electronic health records, over-the-counter medication sales, social media signals, and even internet search queries. By detecting anomalies—like an unusual uptick in hospital visits for certain symptoms—AI can trigger early alerts that help public health authorities investigate potential outbreaks before they spread widely. This prompt detection fosters timely interventions such as targeted testing, quarantine measures, or vaccination campaigns. Over time, these enhanced surveillance capabilities can lead to more effective containment strategies and ultimately reduce the incidence and severity of outbreaks.
2. Predictive Modeling for Resource Allocation
Advanced machine learning models can forecast disease prevalence and healthcare demands, guiding policymakers in more strategic distribution of vaccines, medical supplies, and healthcare professionals.
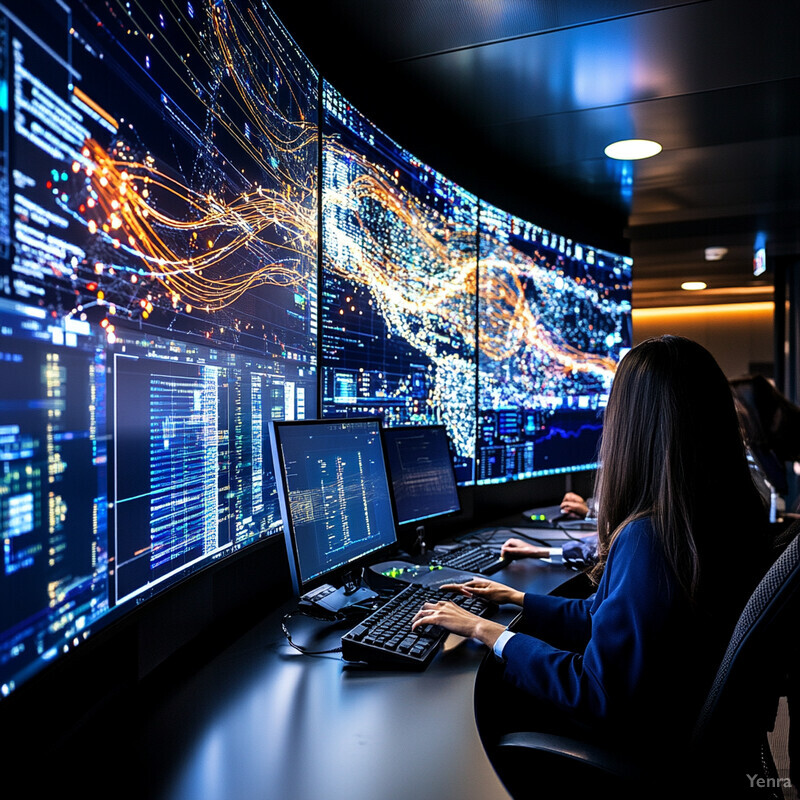
Effective public health policy often hinges on anticipating where and when resources—such as vaccines, personal protective equipment, or skilled healthcare workers—will be needed most. AI-driven predictive models use historical data, demographic statistics, disease transmission trends, and other indicators to generate forecasts of healthcare demand. Policymakers can leverage these forecasts to allocate funds and resources more strategically, ensuring communities are better equipped to respond to health crises. By improving the accuracy and reliability of these predictions, AI can help minimize waste, lower costs, and improve overall preparedness in public health systems.
3. Improved Targeting of Public Health Interventions
By analyzing large datasets, AI can pinpoint geographic areas and demographic groups at highest risk, allowing policies and interventions to be tailored more effectively to those populations.
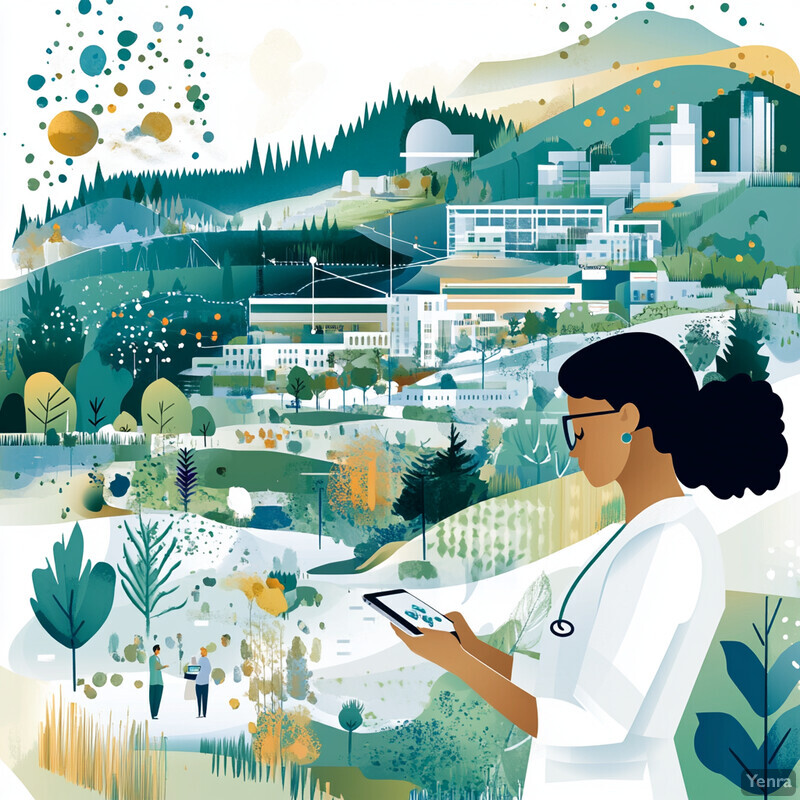
Not all populations face the same health risks; certain communities may be disproportionately affected by specific diseases due to environmental, genetic, or socioeconomic factors. AI can analyze large and complex datasets—covering everything from geographic infection patterns to lifestyle and behavioral data—and identify which groups are most vulnerable. By highlighting these high-risk clusters, policymakers can focus interventions like vaccination drives, health education programs, or screening campaigns more efficiently. This targeted approach ensures that limited resources have the greatest possible impact, ultimately improving health equity and outcomes on a population level.
4. Data Integration from Multiple Sources
AI tools can merge disparate data—clinical records, environmental variables, socioeconomic indicators—into unified dashboards, giving policymakers a comprehensive picture of community health and resource needs.
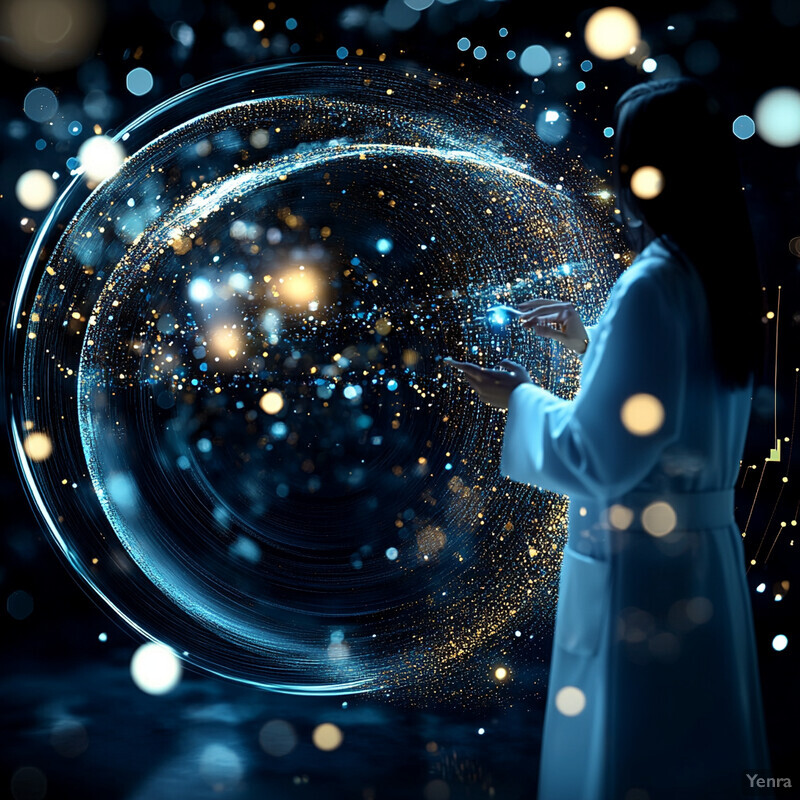
Public health data traditionally resides in separate silos: hospitals maintain medical records, environmental agencies track pollution, and social services collect information on housing conditions. AI can act as a powerful integrator, harmonizing disparate data sources—clinical, environmental, socioeconomic—into a single cohesive framework. Through machine learning-driven data fusion, policymakers gain a more holistic understanding of community health determinants. This comprehensive perspective enables the formulation of evidence-based policies that take into account the multifaceted nature of health, leading to interventions that address underlying root causes rather than just symptoms.
5. Cost-Effectiveness Analysis of Policies
Machine learning algorithms can evaluate various policy options and simulate their financial impacts, enabling more cost-effective decision-making in public health program planning.
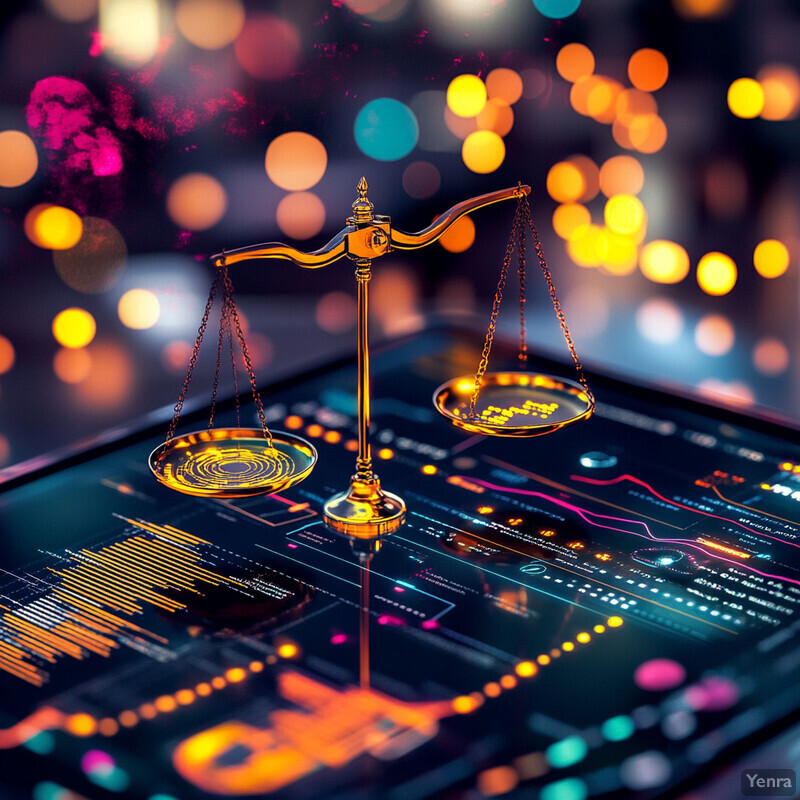
In an era of limited funding and rising healthcare costs, it’s vital to ensure that every dollar spent on public health yields maximal benefit. AI-driven simulation models and cost-effectiveness analyses can evaluate policy options—such as investing in preventive screenings, altering insurance coverage strategies, or implementing taxation on harmful substances—and quantify their fiscal impact alongside health outcomes. By comparing scenarios, policymakers can choose interventions that offer the best balance between cost and effectiveness, thereby promoting fiscal responsibility, improving health system sustainability, and ultimately delivering more value to the public.
6. Behavioral and Social Determinants Analysis
Natural Language Processing (NLP) and machine learning can help identify patterns in social factors—like housing conditions, education levels, and employment status—that influence health outcomes, guiding more holistic and equity-focused policies.
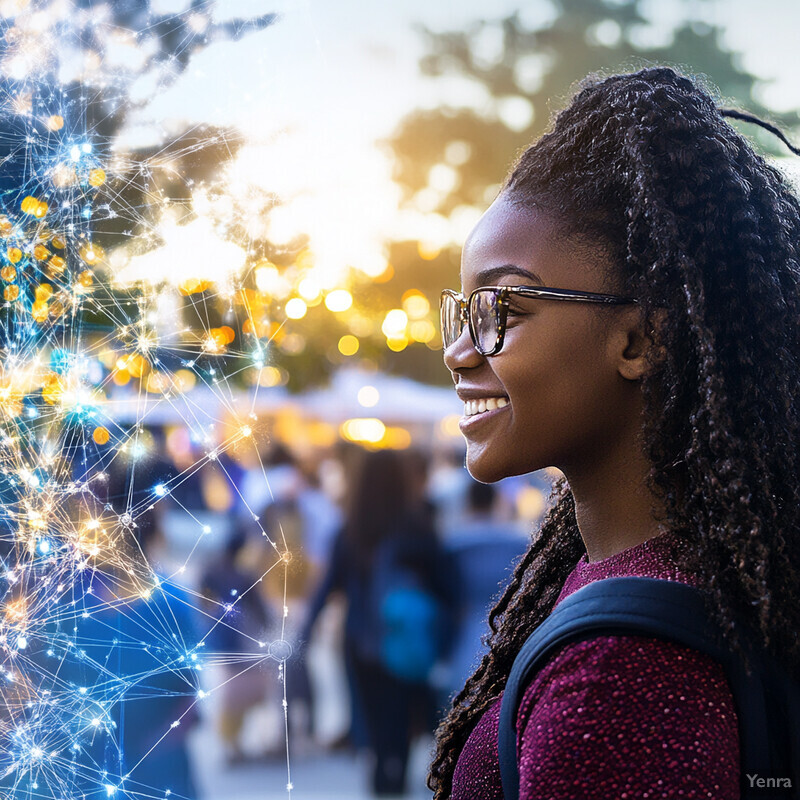
Health outcomes are influenced not only by genetics and pathogens but also by a wide array of social and behavioral factors. AI, particularly natural language processing and machine learning, can sift through large datasets that include social media posts, survey responses, lifestyle indicators, and community-level socioeconomic statistics to uncover patterns that contribute to health disparities. By quantifying these social determinants—like poverty, education, or food insecurity—public health officials gain insights into how best to address underlying issues. In turn, policies can be designed to tackle the structural and systemic factors that prevent individuals from achieving their highest attainable level of health.
7. Enhanced Contact Tracing and Epidemic Control
Automated AI-driven contact tracing using mobile phone and sensor data can streamline the identification of transmission chains, helping formulate timely containment policies.
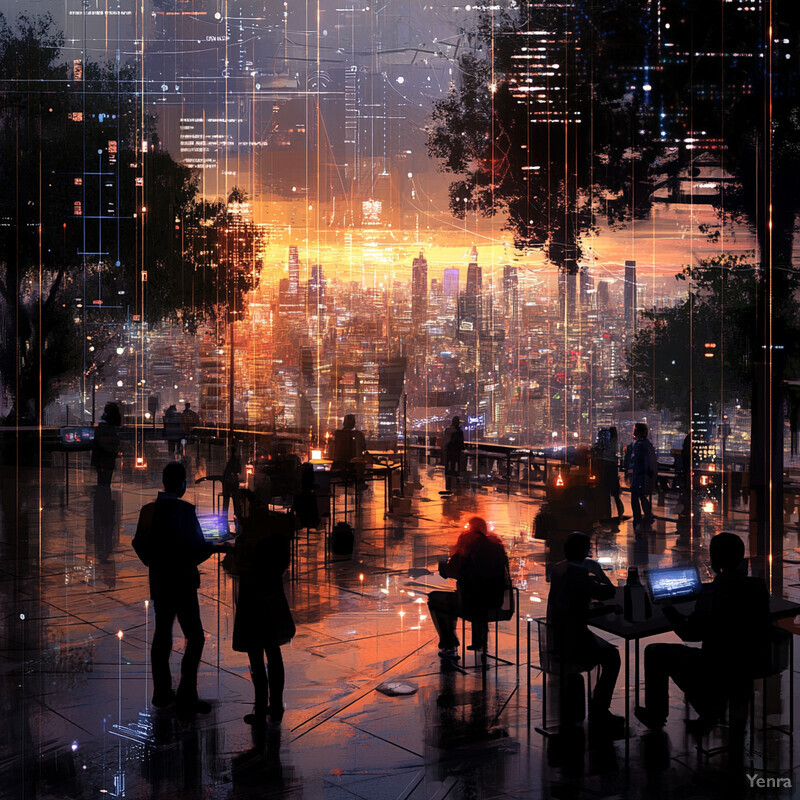
Traditional contact tracing methods can be time-consuming and prone to human error, but AI offers more efficient solutions. Automated contact tracing apps and AI-driven analysis of mobility data can help identify individuals who have been in close proximity to infected persons. By identifying transmission chains swiftly and accurately, policymakers can implement more targeted measures—quarantine orders, testing mandates, and localized lockdowns—to contain outbreaks. This precision in epidemic control reduces disruption to daily life, conserves critical resources, and limits the economic fallout often associated with broad, indiscriminate public health restrictions.
8. Early Warning Systems for Food and Water Safety
AI can continuously analyze supply chain and environmental monitoring data to flag potential contamination events, informing preventative regulations and safety standards.
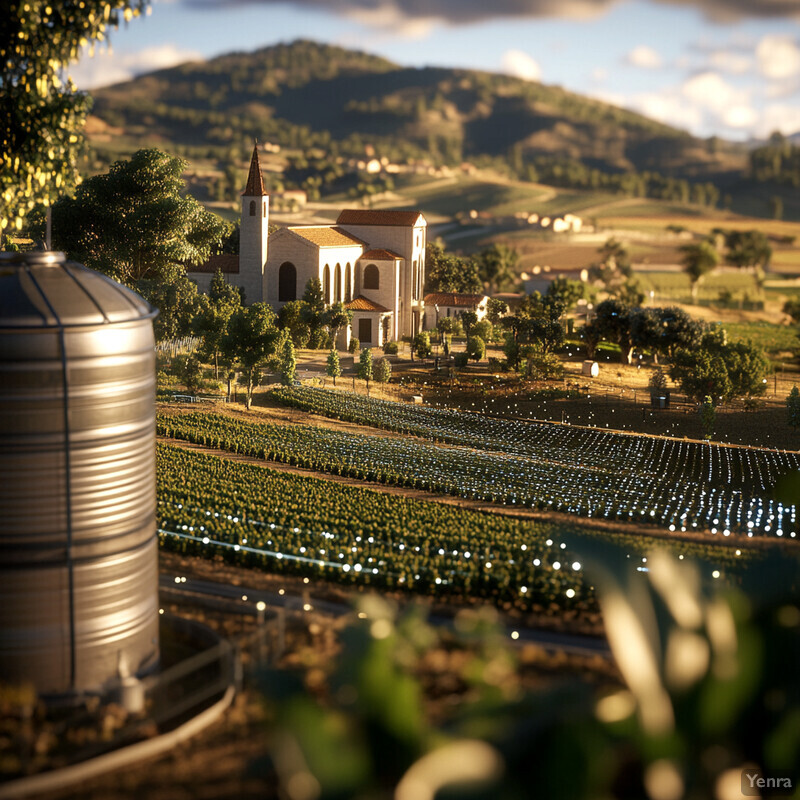
Foodborne and waterborne illnesses pose significant threats to public health, yet manual oversight of supply chains and environmental conditions is challenging. AI can monitor data from agricultural production, distribution networks, restaurant inspections, environmental sensors, and consumer complaints to identify anomalies indicating potential contamination. Early detection of issues—like a spike in bacterial load or a chemical pollutant—enables swift policy responses, such as issuing recalls, imposing stricter safety standards, or bolstering inspection protocols. This proactive approach helps prevent large-scale outbreaks, protects consumer confidence, and maintains high standards of food and water quality.
9. Rapid Policy Impact Assessment
Simulation and agent-based modeling powered by AI can quickly estimate the potential outcomes of various public health policies, allowing decision-makers to refine strategies before implementation.
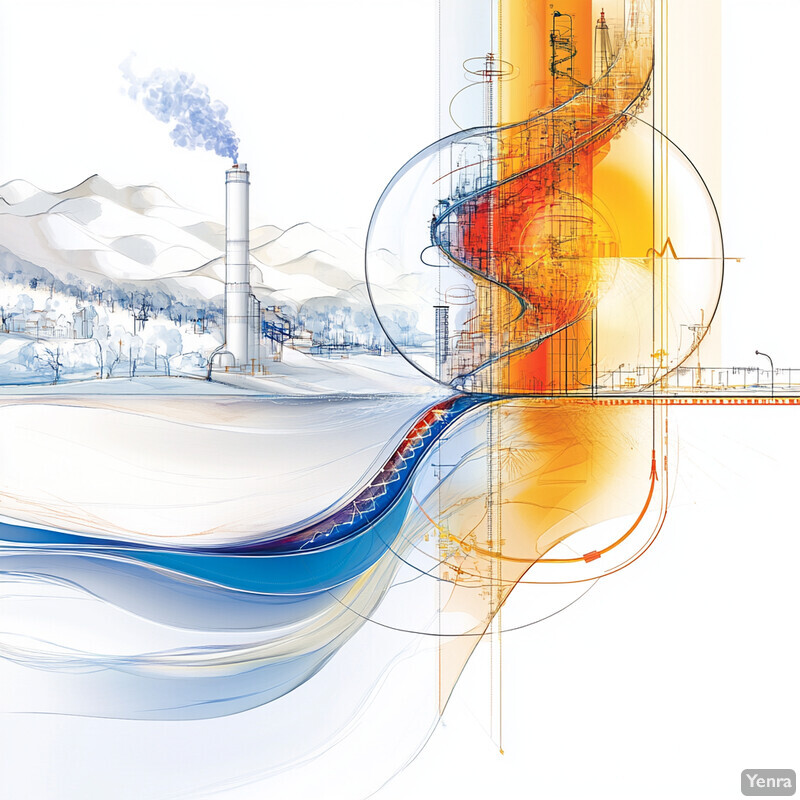
Before implementing new policies, public health authorities need to understand their potential outcomes. AI-driven simulation models and agent-based modeling can rapidly test hypothetical scenarios—such as mandating vaccines, changing quarantine durations, or altering nutritional standards in schools—to predict their impacts on population health. These virtual experiments allow policymakers to refine strategies, mitigating unintended consequences and enhancing the likelihood of a successful intervention. With AI’s speed and analytical depth, policy impact assessments become more dynamic and responsive, leading to more informed and adaptive decision-making.
10. Personalized Public Health Messaging
AI-driven segmentation of populations can inform more effective communication campaigns by tailoring messages to cultural, linguistic, and behavioral contexts, improving the uptake of preventive measures.
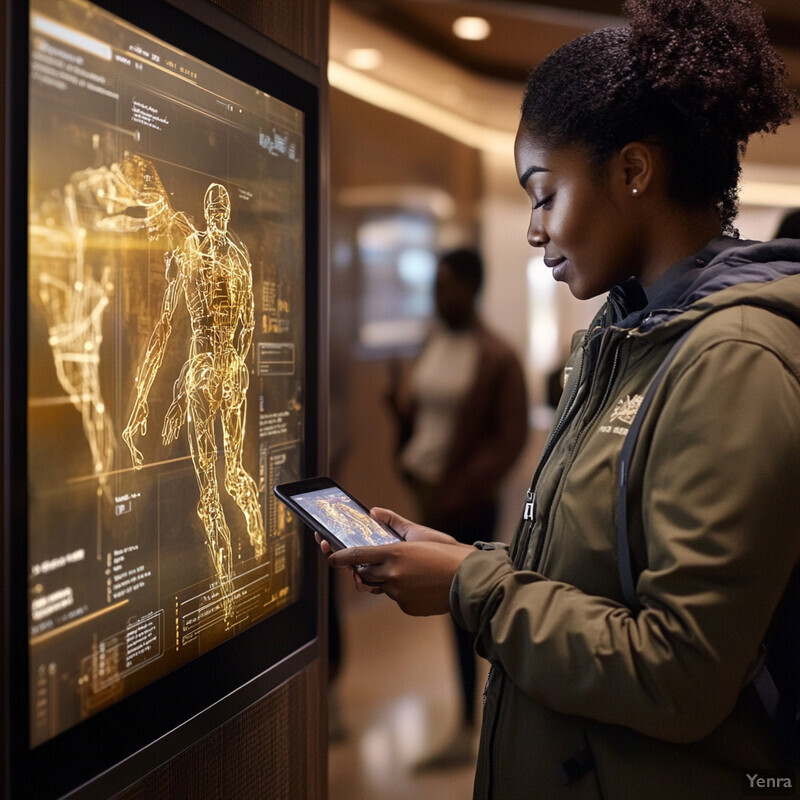
Different population segments may respond differently to the same public health message due to language, cultural norms, literacy levels, or trust in authorities. Using AI to analyze demographic data, language use, and media consumption habits, communication strategies can be tailored to effectively reach specific groups. Personalized campaigns can address cultural sensitivities, simplify medical terminology, and deliver content through the channels an audience trusts, whether it’s social media platforms, community radio, or local influencers. This tailored approach boosts community engagement, increases adherence to health guidelines, and improves overall health outcomes.
11. Drug Resistance and Pathogen Evolution Forecasting
Predictive analytics can anticipate the emergence of antibiotic-resistant bacteria or changes in viral strains, helping guide vaccination and antimicrobial stewardship policies.
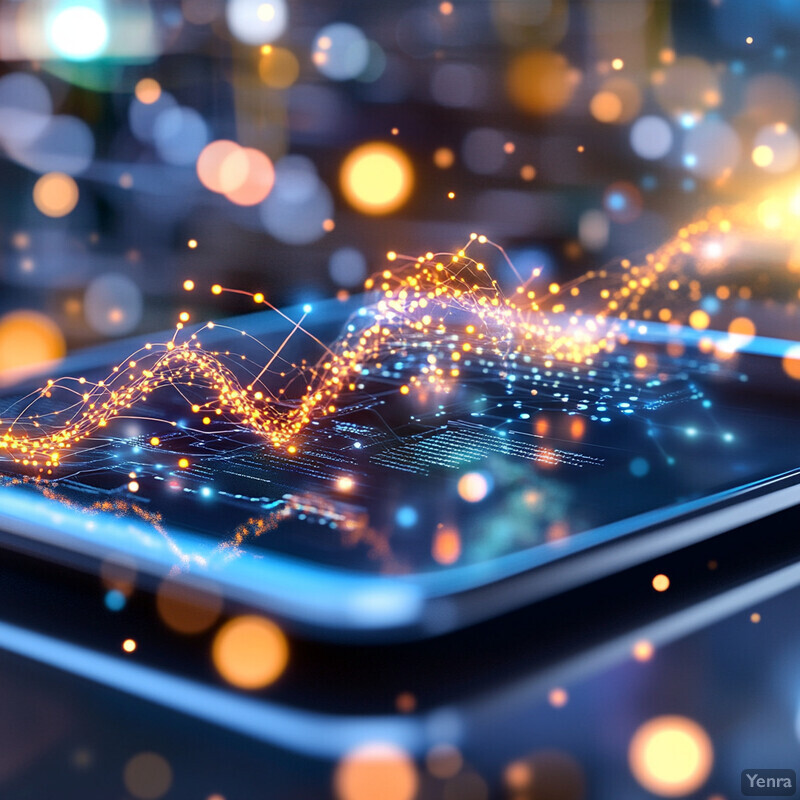
As microorganisms evolve, drug-resistant strains and vaccine-evading pathogens can emerge, posing severe challenges to existing treatments. AI can analyze genetic sequences, clinical trial data, and pathogen behavior patterns to anticipate resistance trends. By forecasting how pathogens might adapt over time, policymakers can proactively adjust antibiotic stewardship programs, fund research into new therapies, and update vaccination strategies. This preemptive stance against evolving pathogens can slow resistance, preserve the effectiveness of existing treatments, and maintain a robust medical arsenal against infectious diseases.
12. Identification of Inequities and Disparities
Machine learning can highlight patterns of healthcare access and outcomes that suggest racial, ethnic, or socioeconomic disparities, supporting policies aimed at reducing health inequalities.
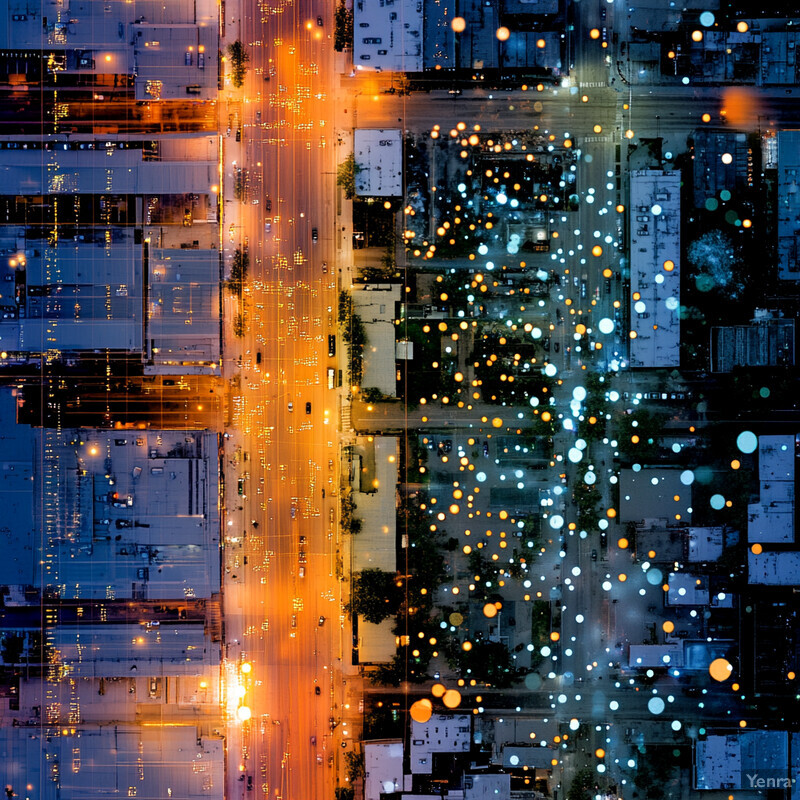
Health disparities often arise from complex intersections of race, ethnicity, gender, income, and other social factors. By applying AI to large-scale healthcare datasets, researchers can identify where inequities exist—such as certain neighborhoods experiencing higher rates of chronic diseases or certain populations having less access to preventive care. Armed with these insights, policymakers can design policies that specifically target these gaps. Measures could include improving healthcare facilities in underserved areas, increasing outreach programs, or adjusting insurance policies. Over time, this data-driven identification and correction of inequities can contribute to more equitable healthcare systems.
13. Enhanced Mental Health Policy Development
Natural Language Processing can analyze large volumes of social media and clinical notes to identify mental health trends and stressors in communities, informing policies that address preventive mental health care.
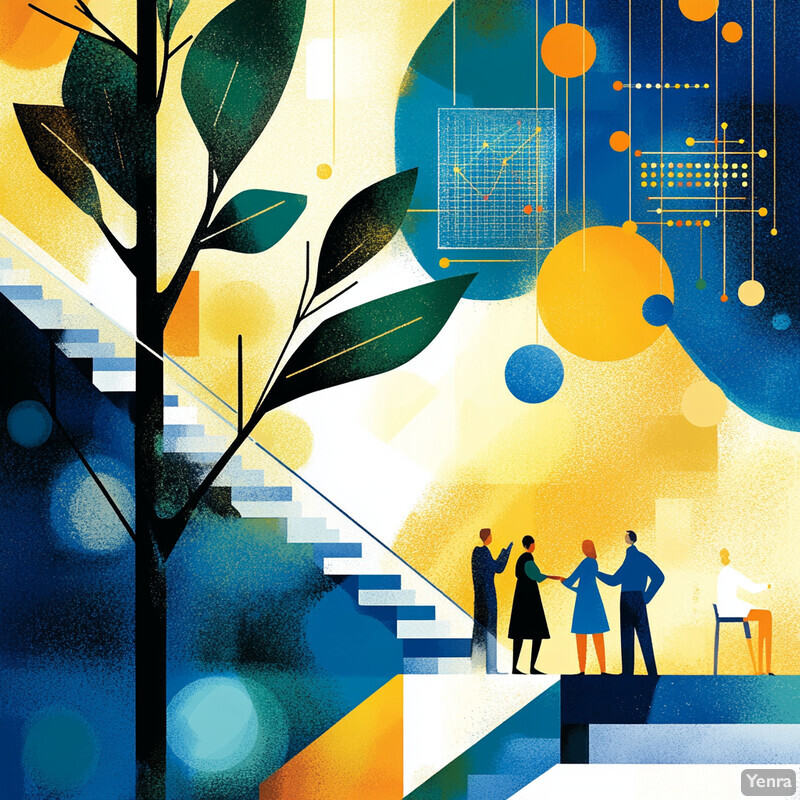
Mental health issues, including anxiety, depression, and stress-related disorders, are often underdiagnosed and misunderstood, in part because of their complex and personal nature. AI can help by analyzing large volumes of anonymized social media posts, clinical notes, and public health surveys to gauge community sentiment, detect rising stressors, and identify trends in mental health conditions. Policymakers can use these insights to tailor mental health services, allocate funding for counseling programs, and adjust education campaigns to address prevailing issues. Ultimately, AI-driven analyses can help destigmatize mental health, improve service accessibility, and enhance the quality of care.
14. Environmental Health Risk Assessment
AI models can link pollution data, weather patterns, and health records to assess exposure risks and develop policies aimed at mitigating the health impacts of environmental factors like climate change.
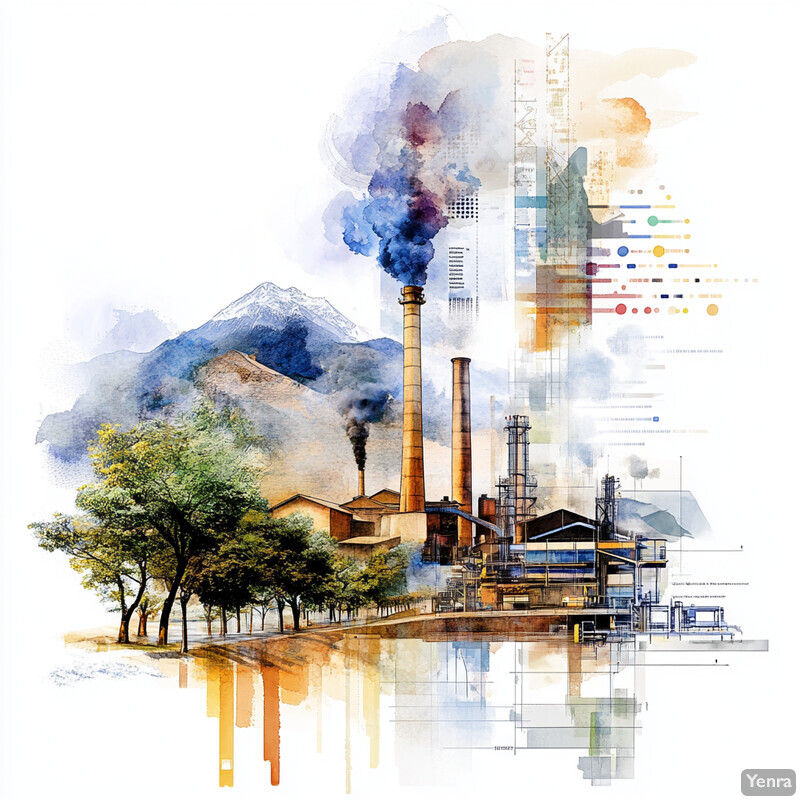
Our health is intricately tied to the quality of our environment. Factors like air pollution, climate change, and exposure to toxins in water and soil significantly influence disease patterns. AI models can correlate health records with environmental data—weather patterns, industrial emissions, urban density—to identify areas at greater risk for respiratory conditions, heat stroke, or vector-borne diseases. Policymakers can then implement targeted environmental policies—emission reductions, infrastructure improvements, or green space initiatives—to mitigate these risks. By taking a data-driven approach, public health policies can more effectively address environmental determinants of health.
15. Optimizing Vaccination Strategies
Machine learning can help determine optimal immunization schedules, distribution strategies, and communication approaches, especially crucial in resource-limited settings or during emerging epidemics.
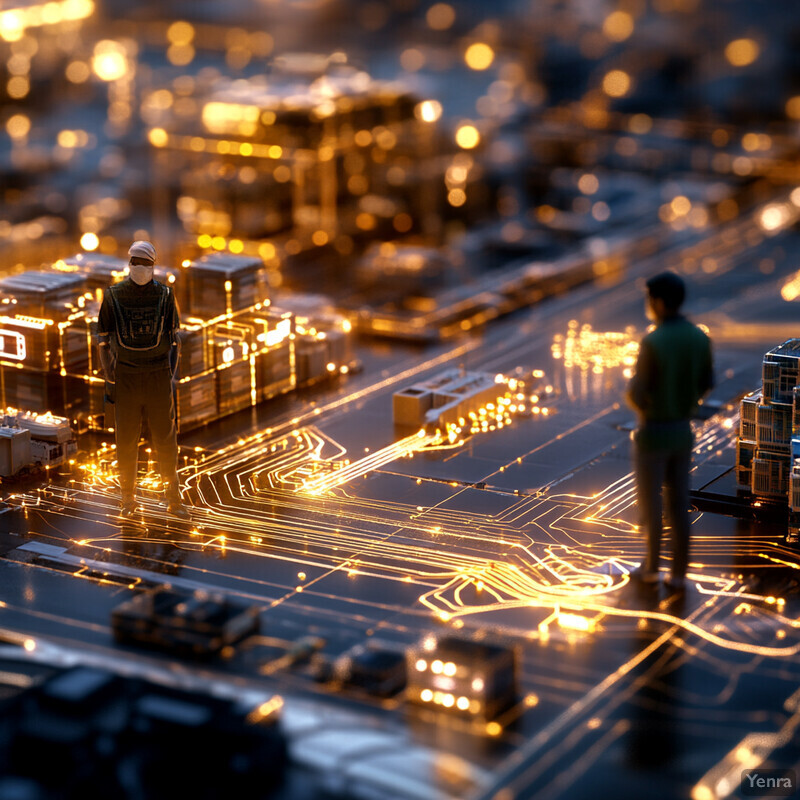
Vaccine distribution is a complex endeavor, especially when supply is limited or during a newly emerging infectious threat. AI can help by simulating various distribution strategies, identifying priority populations, and modeling the impact of different rollout timetables. By accounting for factors like geographic accessibility, population density, and at-risk groups, AI tools guide policymakers in maximizing immunization coverage and effectiveness. Additionally, AI-driven analytics can inform communication strategies to improve vaccine uptake and monitor for regions or communities with lower vaccination rates, ensuring more equitable access to this cornerstone of public health.
16. Improved Crisis Management and Response
In emergencies—such as natural disasters or pandemics—AI systems can rapidly consolidate information and guide policy interventions, from evacuation planning to targeted medical relief.
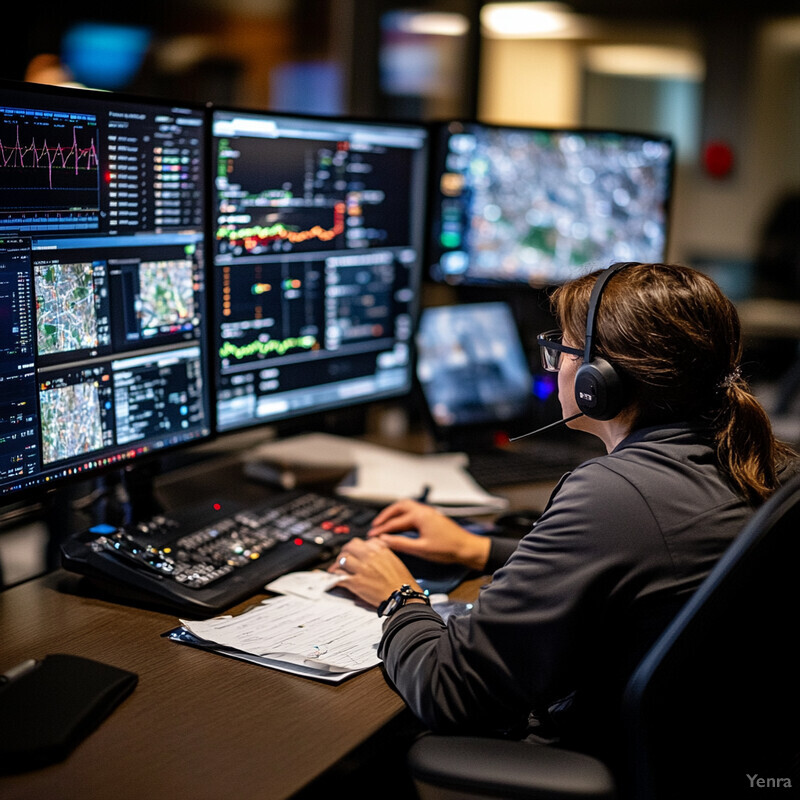
In the chaos following natural disasters, pandemics, or bioterrorist attacks, time is of the essence. AI-powered platforms can analyze multiple streams of information—weather data, emergency room admissions, news reports, and social media alerts—to provide a real-time situational picture. With these insights, policymakers can rapidly deploy mobile clinics, prioritize rescue operations, or direct relief resources to the hardest-hit areas. By enhancing the speed and accuracy of emergency responses, AI supports more resilient health systems capable of protecting populations under the most demanding conditions.
17. Enhanced Chronic Disease Management Policies
AI-driven risk prediction and patient stratification can guide policies that focus on preventing complications of chronic diseases (like diabetes or heart disease) and improving long-term health outcomes.
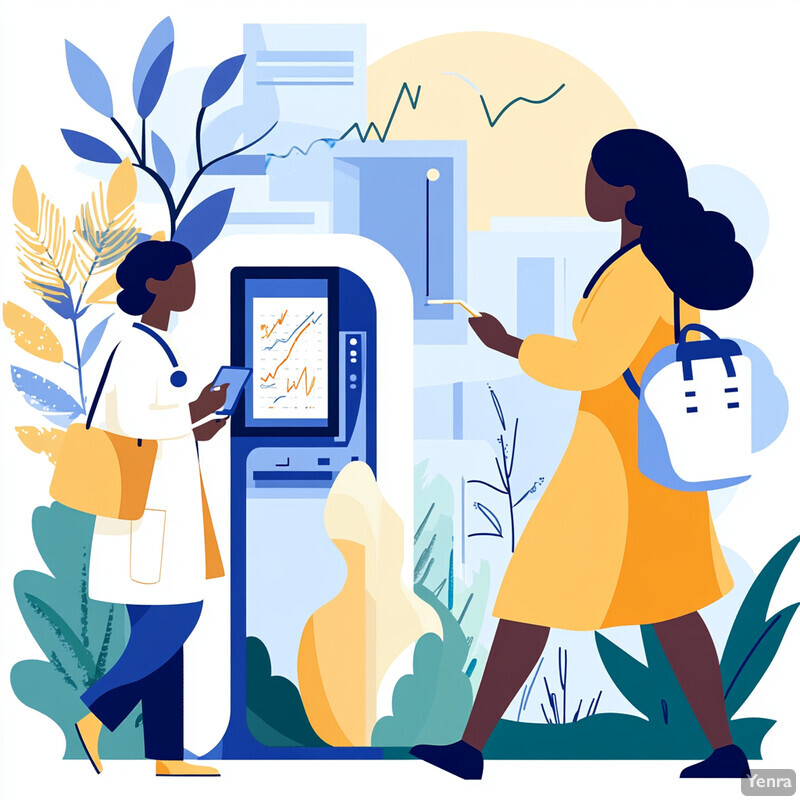
Chronic conditions like diabetes, heart disease, and asthma generate significant healthcare burdens. AI can identify patterns of high-risk patients, predict disease exacerbations, and assess the effectiveness of preventive programs. Policymakers can use these insights to devise policies that emphasize early screening, encourage healthy lifestyles, improve chronic disease education, and facilitate integrated care models. With more proactive management informed by data-driven predictions, chronic disease policies can reduce hospitalizations, lower healthcare costs, and improve patients’ quality of life.
18. Data-Driven School and Workplace Health Policies
By analyzing infection patterns or chronic health conditions, AI can inform policies around testing, hygiene practices, or ventilation standards in schools and workplaces.
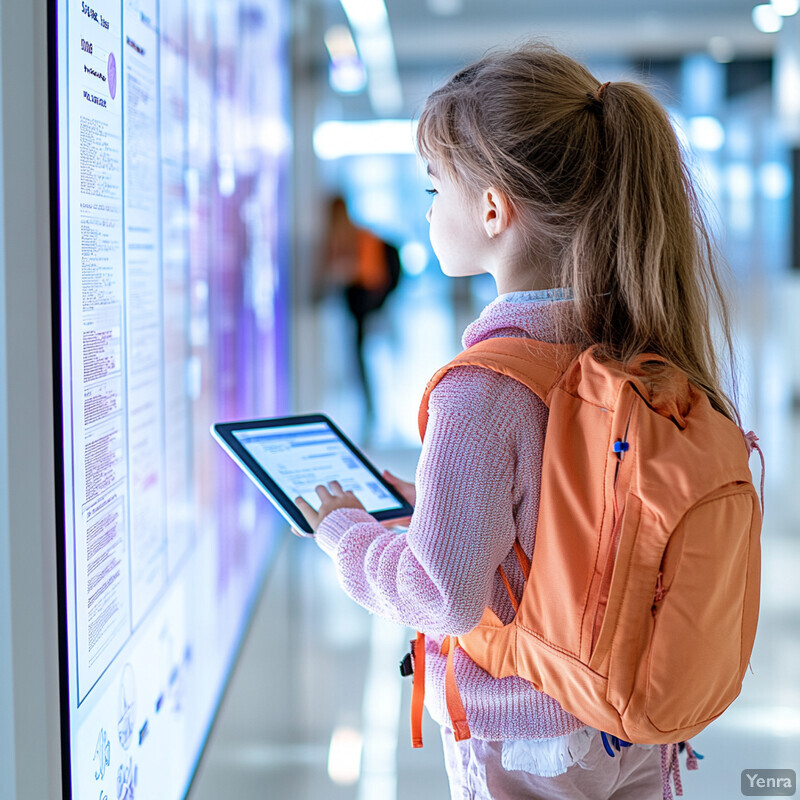
Educational institutions and workplaces are microcosms of society and often reflect broader public health trends. AI can analyze attendance records, illness reports, and environmental factors—such as ventilation quality or class size—to determine policies that reduce disease transmission and support overall well-being. Public health authorities can implement informed recommendations regarding vaccination mandates, hygiene protocols, mental health services, or healthier meal programs. By creating healthier learning and working environments, these data-driven policies contribute to a more productive and resilient population.
19. Development of Digital Public Health Infrastructures
AI supports the creation and refinement of integrated data platforms, improving policy-related workflows, and enabling more agile and informed decision-making processes.
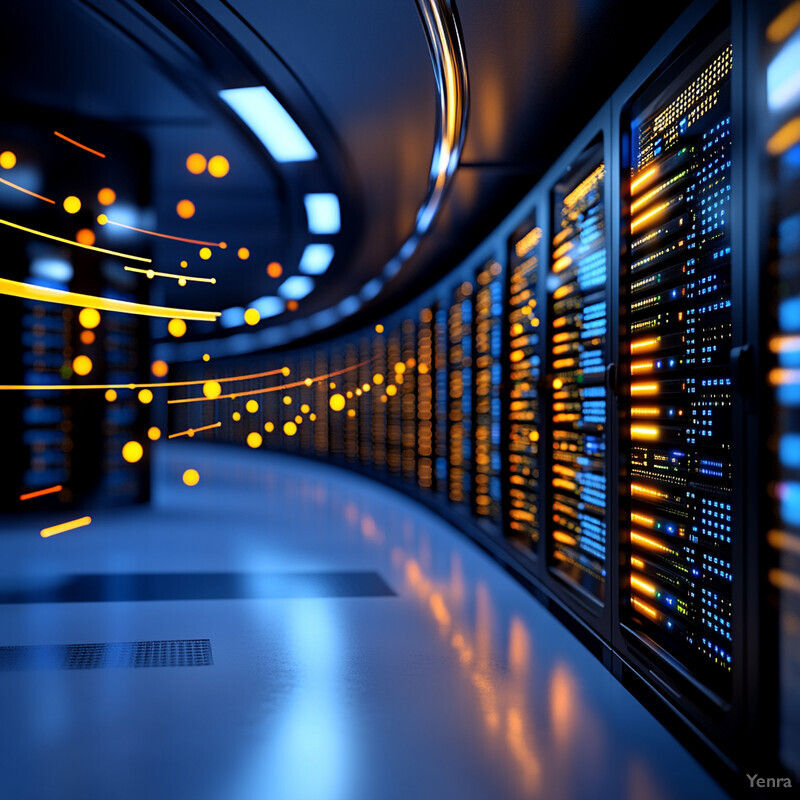
AI’s capabilities flourish in robust digital ecosystems where data is readily accessible, standardized, and secure. Investments in AI-driven infrastructure—such as interoperable electronic health record systems, secure data exchanges, and advanced analytics platforms—help policymakers create an integrated “nervous system” for public health. This digital backbone ensures that insights from AI can quickly inform decision-making processes, streamline reporting, and improve the responsiveness of the entire health sector. Over time, these infrastructural enhancements set the stage for ongoing innovation and continuous improvement in public health policy.
20. Continuous Policy Improvement through Feedback Loops
With AI’s ability to learn from new data, policymakers can establish dynamic feedback loops, continuously refining guidelines and regulations as fresh evidence emerges, ensuring policies remain current and effective.
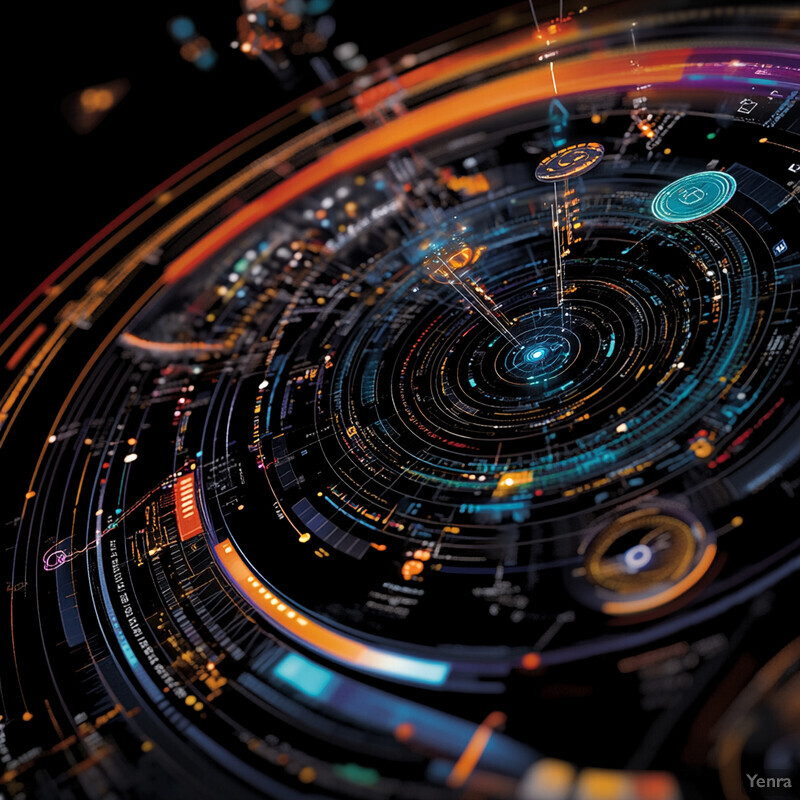
Public health policies are rarely perfect on the first attempt. AI can facilitate iterative refinement by monitoring outcomes in near real-time and providing immediate feedback to policymakers. As new data streams in, AI models update their analyses and highlight where policies are falling short or where unexpected benefits are emerging. These dynamic feedback loops enable policymakers to adjust regulations, resource allocations, or communication strategies promptly. Over the long term, this continuous learning approach ensures that public health policies remain agile, evidence-based, and aligned with evolving community needs.