1. Precision Habitat Mapping
AI-powered satellite image analysis and aerial drone imagery allow for precise identification and mapping of critical habitats and degraded areas in need of restoration. Advanced computer vision algorithms can distinguish subtle vegetation types, enabling planners to focus interventions exactly where they’re most needed.
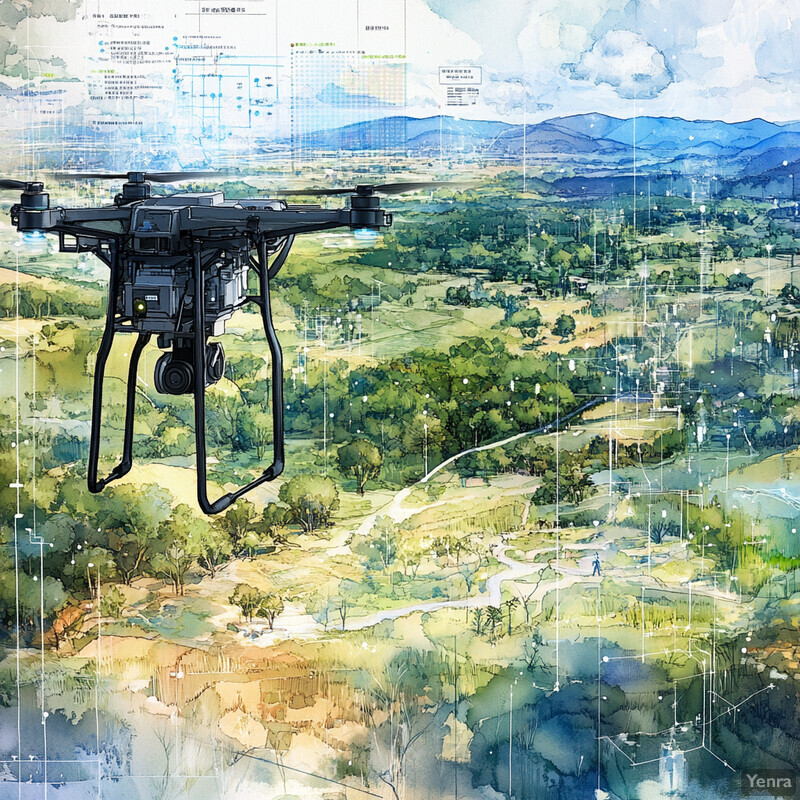
AI-powered geographic information systems (GIS) and high-resolution remote sensing technologies enable conservationists to build incredibly detailed maps of landscapes, pinpointing exact vegetation types, soil conditions, and water availability. Using satellite imagery, LiDAR scans, and drone-captured video, advanced computer vision algorithms can distinguish subtle habitat differences, identify areas with invasive species, and highlight regions suffering from nutrient deficiencies or fragmentation. This precision guides restoration teams by directing them to the most critical sites and allowing them to tailor their interventions—such as selective planting, controlled burns, or erosion control measures—to the unique characteristics of each location. As a result, restoration efforts become more targeted, resource-efficient, and ultimately more successful.
2. Predictive Modeling of Ecosystem Change
Machine learning models can predict how landscapes will evolve under different climate scenarios, guiding long-term restoration strategies. By simulating future outcomes, planners can choose interventions that are more resilient to changing conditions such as temperature shifts, altered rainfall patterns, and pest outbreaks.
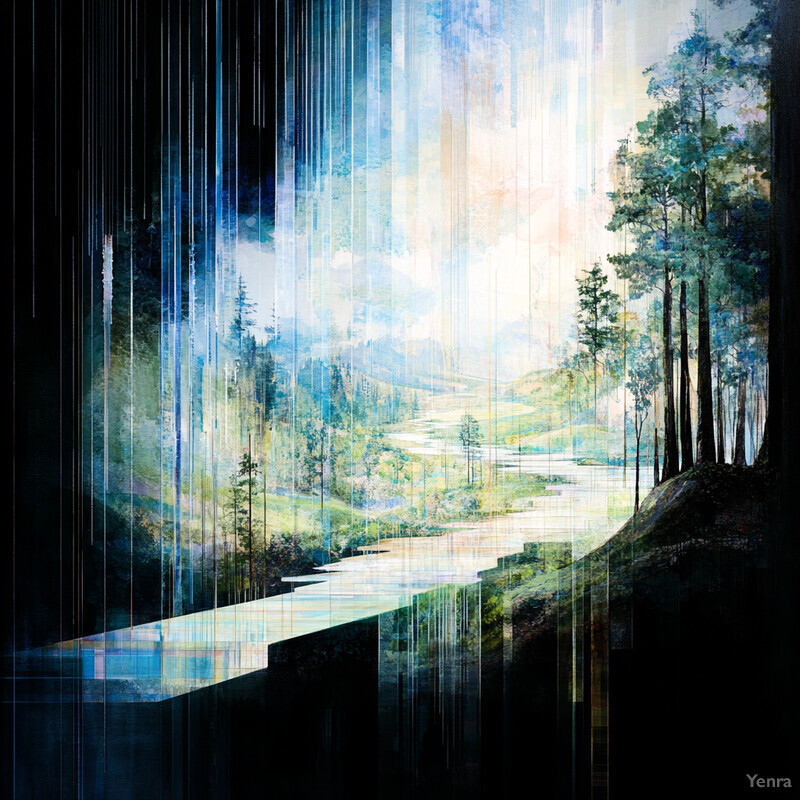
Machine learning models trained on historical and environmental data can forecast how natural habitats will evolve under various climate and land-use scenarios. These predictions help researchers and policymakers anticipate the impacts of rising temperatures, shifting precipitation patterns, and extreme weather events on biodiversity, vegetation growth, and soil stability. By modeling long-term outcomes, teams can identify restoration strategies that will remain viable decades into the future, choosing native species that are more drought-tolerant or planning for corridors that maintain connectivity despite changing landscapes. Such insight leads to adaptive, forward-looking plans that ensure restored habitats can withstand and thrive amid ongoing environmental changes.
3. Optimizing Seed Selection
AI-driven genomic analysis helps identify native plant species and seed genotypes best suited to particular soil and climate conditions. This ensures that reintroductions have a higher survival rate and better long-term ecosystem impact.
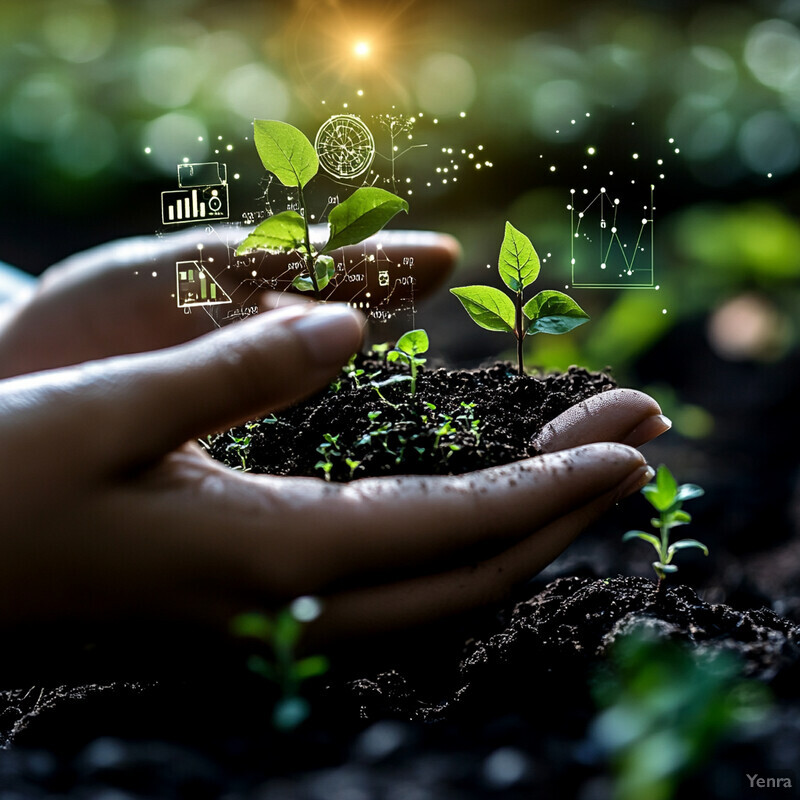
AI tools analyze large genomic and phenotypic datasets of native plant species to identify which seeds are most suitable for a given restoration site. By considering factors such as soil acidity, water retention capacity, local pollinators, and historical climate patterns, these systems can recommend hardy plant varieties that have a higher survival rate. In addition, AI-based models can predict how plants may respond to stressors like disease or drought. This approach ensures that restored habitats are composed of resilient plants genetically adapted to local conditions, reducing the need for repeated interventions and accelerating the establishment of stable, self-sustaining ecosystems.
4. Drone-Assisted Reforestation
Unmanned aerial vehicles equipped with AI-based navigation and species-recognition software can autonomously plant seeds in deforested areas. Smart systems determine the best planting locations, seed density, and species mix to accelerate forest recovery.
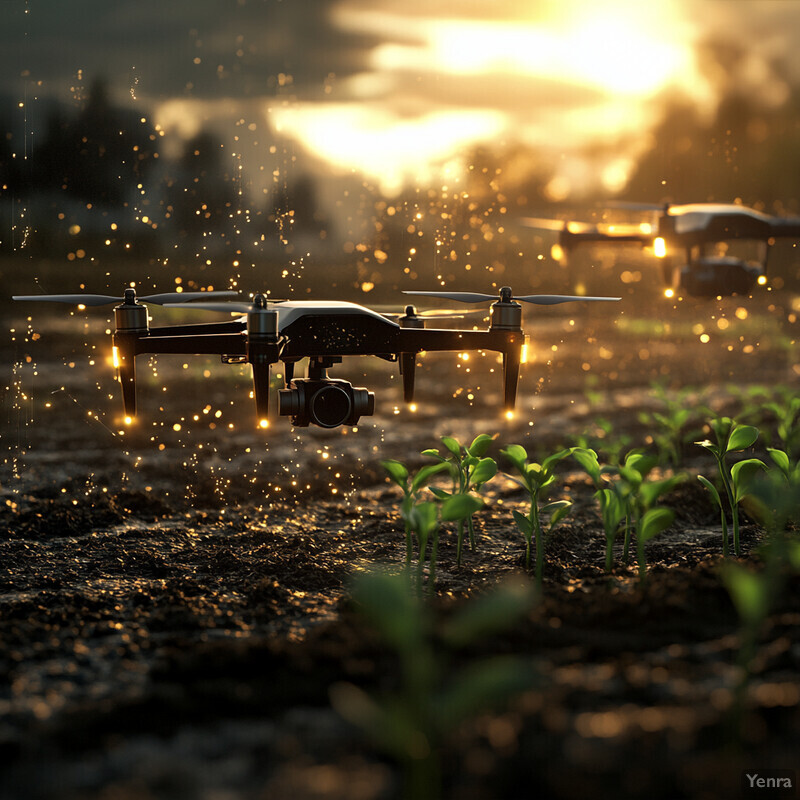
Drones equipped with AI-based navigation and terrain analysis software can autonomously plant seeds or seedlings in targeted patterns and densities. These aerial devices can quickly cover large tracts of land, even those that are difficult for human crews to reach, and drop seeds precisely where they’re needed. By analyzing topography, soil moisture, and temperature gradients in real-time, AI ensures that each seed is placed in conditions favorable for germination and growth. Drone-assisted reforestation not only expedites restoration activities but also reduces human labor costs, enabling conservationists to revegetate large areas more rapidly and at lower expense.
5. Invasive Species Detection and Management
Computer vision models can recognize invasive species from remote-sensing data or camera traps. Once identified, these models can prescribe targeted removal or treatment measures, helping restore native habitats more efficiently.
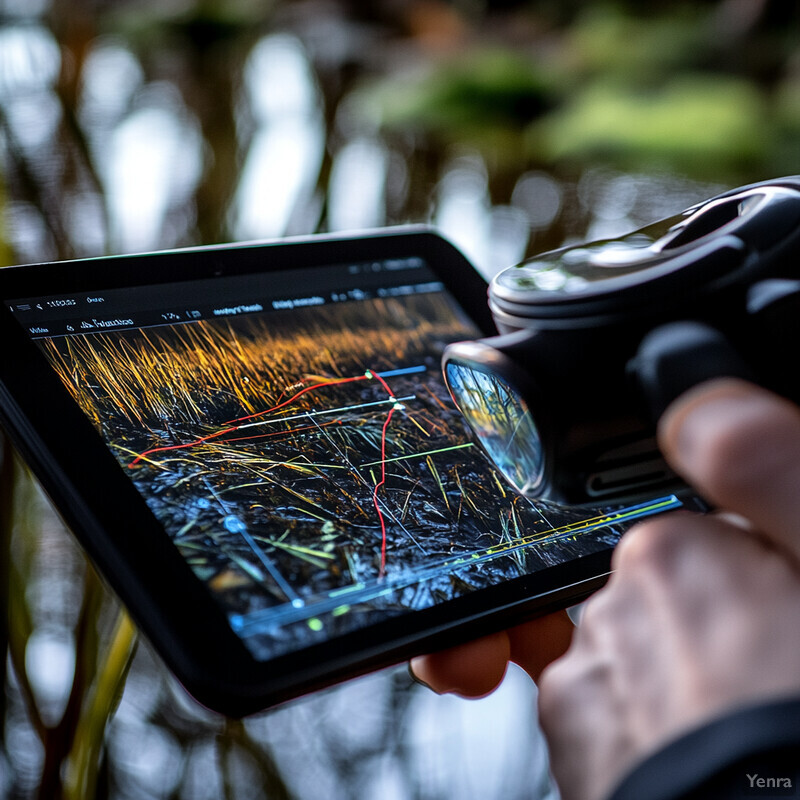
Invasive plants, insects, and pathogens can undermine restoration efforts by outcompeting or preying on native species. AI-driven image recognition systems can sift through satellite data, aerial footage, or trail camera feeds to identify the presence and spread of non-native organisms. By correlating these observations with environmental variables, the AI can predict where invasives might appear next or which habitats are most vulnerable. Armed with this knowledge, land managers can strategically remove or treat invasives, apply biological controls, or change restoration tactics. This targeted approach minimizes ecological disruption, preserves biodiversity, and helps ensure that native species can reestablish themselves successfully.
6. Soil Health Monitoring
Advanced AI algorithms analyze soil composition data, moisture levels, and nutrient availability, allowing restoration ecologists to identify soil deficiencies and recommend amendments. This data-driven approach ensures more effective and sustainable habitat revitalization.
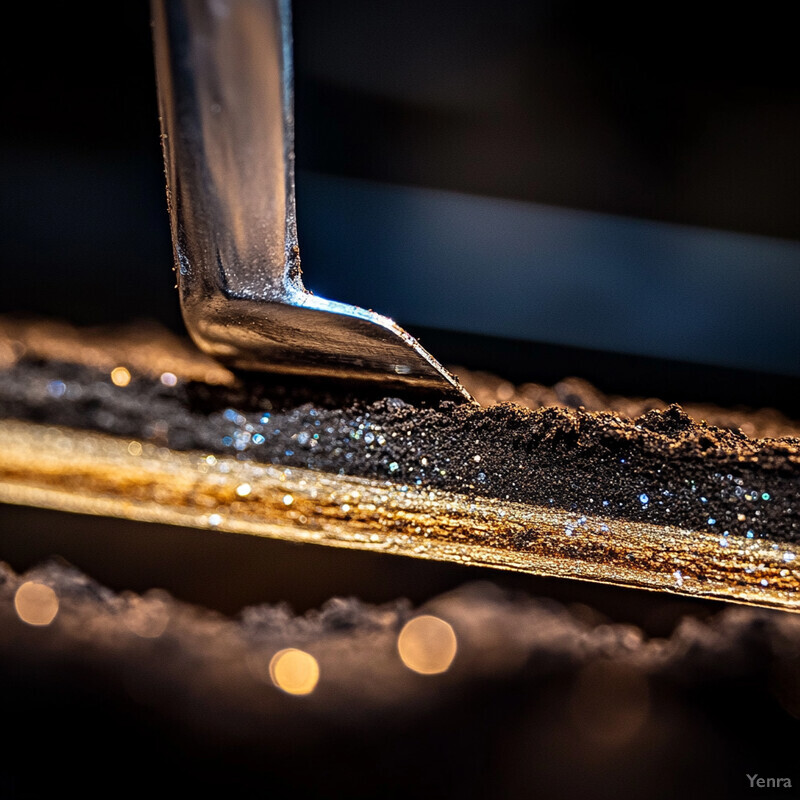
Healthy soil is the foundation of any thriving habitat. AI-powered tools integrate data from soil sensors, chemical assays, and environmental models to assess nutrient levels, microbial communities, compaction, and water infiltration rates. Machine learning algorithms can then recommend specific soil amendments—such as biochar additions, compost treatments, or mycorrhizal inoculations—tailored to restore soil fertility and structure. By understanding soil health at a granular level, restoration practitioners can choose the right mix of interventions to improve plant establishment and accelerate the development of a balanced ecosystem from the ground up.
7. Wildlife Population Tracking
AI-analyzed camera trap imagery, acoustic recordings, and even social media data can monitor wildlife presence and movement. By better understanding species distributions and behaviors, conservationists can adapt restoration efforts to support breeding success and connectivity.
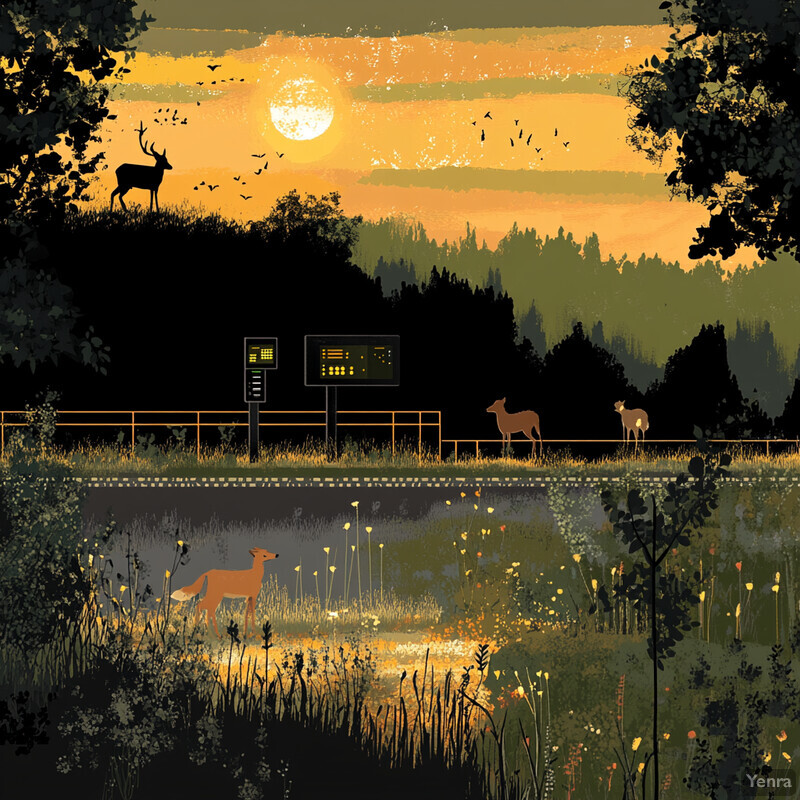
The success of habitat restoration often hinges on whether it supports stable, diverse wildlife populations. AI-driven analytics applied to camera trap images, acoustic recordings, and even social media sightings can quickly reveal which species are present, how their numbers are changing, and where they congregate. By understanding population dynamics—where animals breed, feed, and migrate—conservationists can adjust management techniques to maintain key resources, reduce human disturbances, and improve habitat connectivity. Over time, this feedback loop helps ensure that restored habitats truly function as healthy ecosystems, providing shelter and sustenance to a wide range of native fauna.
8. Intelligent Corridor Planning
Machine learning algorithms can integrate topography, vegetation, water sources, and species movement data to identify the best corridors connecting fragmented habitats. These corridors promote gene flow, reduce human-wildlife conflicts, and ensure long-term ecosystem health.
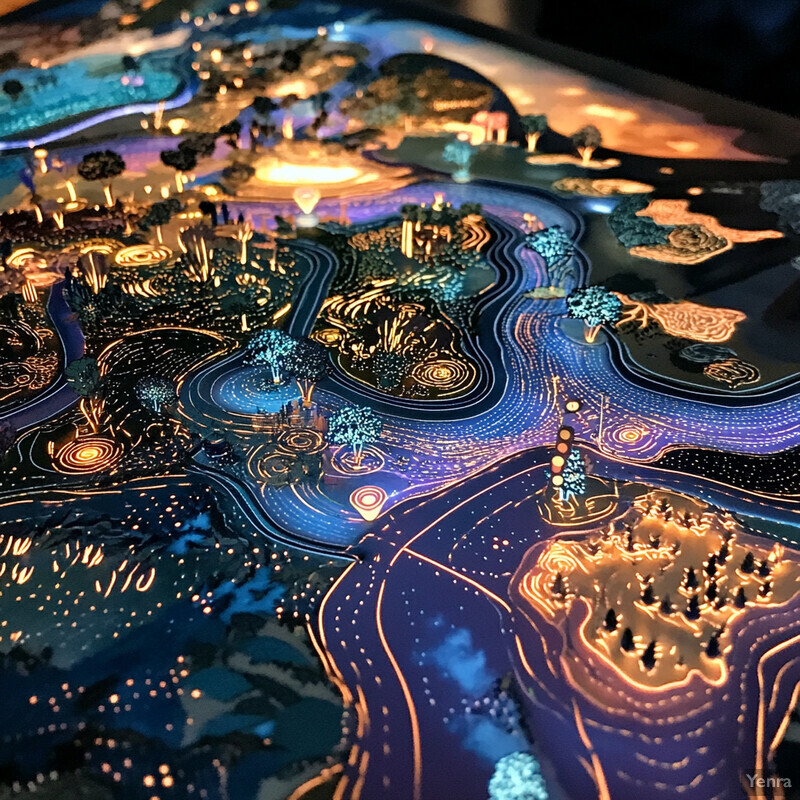
Fragmentation isolates populations and prevents the natural movement of species, weakening genetic diversity and ecosystem resilience. AI-based planning tools integrate data layers like terrain, vegetation, hydrology, and species distribution to recommend corridors that reconnect pockets of suitable habitat. These corridors facilitate gene flow, enhance resilience against environmental changes, and allow species to move between protected areas to find resources or adapt to shifting climates. By using AI to optimize corridor placement, restoration practitioners can ensure that restored habitats form part of a larger, integrated landscape network supporting long-term biodiversity.
9. Adaptive Management Feedback Loops
AI-driven platforms integrate monitoring results in near-real-time, feeding insights back into restoration plans. As conditions evolve, managers can use these insights to adjust approaches, apply new treatments, or refocus restoration goals promptly.
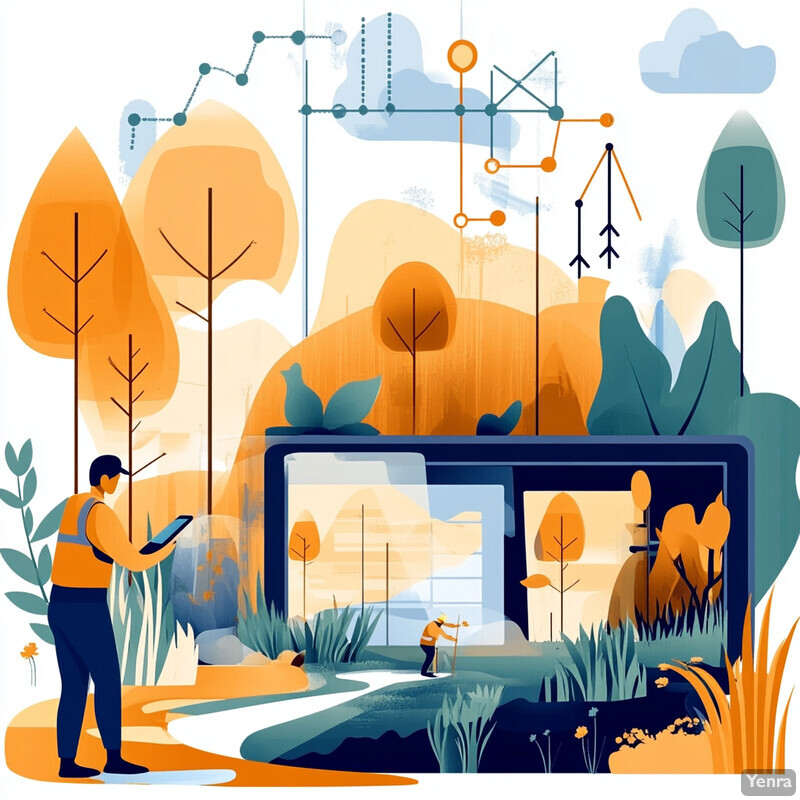
Restoration is not a one-and-done process; it requires iterative assessment and adjustment. AI-based platforms can continuously integrate new data from field surveys, remote sensors, and ongoing experiments, and then apply machine learning to update management recommendations. This dynamic feedback loop enables conservationists to quickly respond to unforeseen changes—such as a sudden pest outbreak or a drought—and implement course corrections. Over time, this adaptability leads to more robust restoration strategies, prevents wasted resources, and ensures that habitats are managed intelligently as they mature and evolve.
10. Climate-Resilience Modeling
Restoration efforts must consider not only current conditions but also future climates. AI tools can factor in temperature changes, increased drought frequencies, or extreme weather events, helping design habitats resistant to climate stressors.
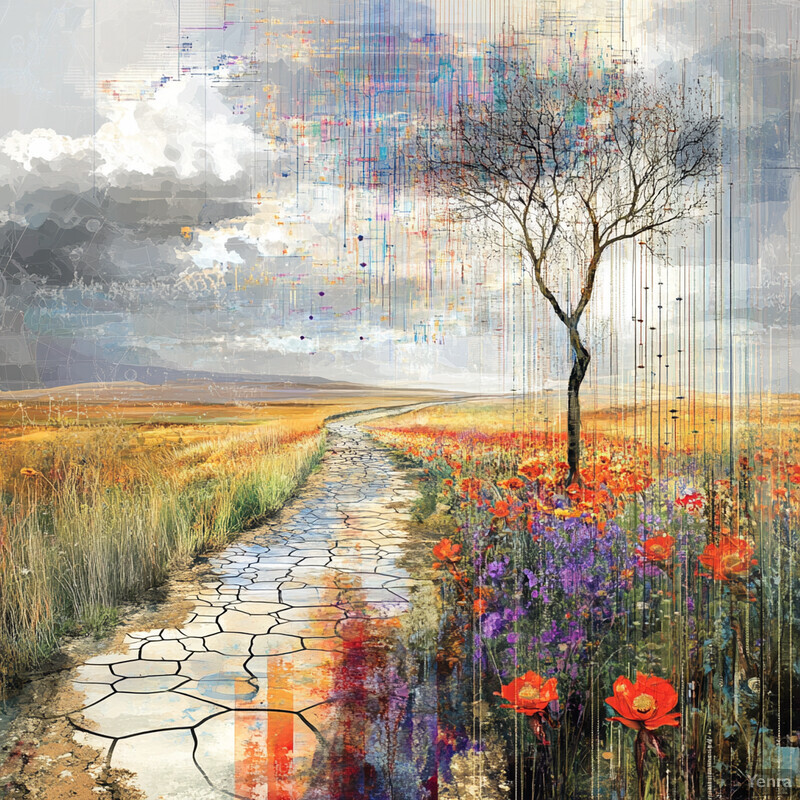
As climate change intensifies, restoration efforts must consider how habitats will respond to warming temperatures, changing precipitation patterns, and extreme weather events. AI-driven climate models can reveal which species and ecosystem configurations are likely to remain stable in the decades ahead. Restoration practitioners can use these insights to choose species assemblages that will thrive in future conditions, design landscapes that buffer against floods or fires, and prioritize interventions that foster long-term resilience. By thinking ahead, restoration plans become proactive rather than reactive, helping habitats endure an uncertain environmental future.
11. Ecosystem Service Valuation
AI can analyze large datasets—from pollinator activity to water filtration rates—and help quantify the ecosystem services provided by restored habitats. This valuation informs policymakers and stakeholders about the tangible benefits of restoration, guiding resource allocation.
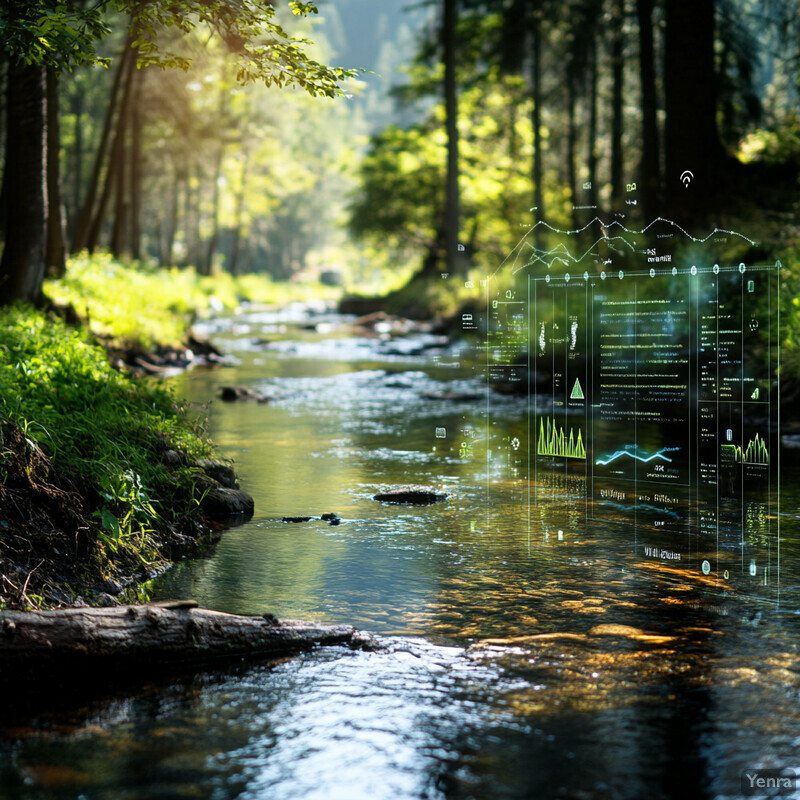
Natural habitats provide valuable services—pollination, carbon storage, water filtration, and recreational opportunities—that benefit both wildlife and human communities. AI can analyze complex data sets, from pollinator activity logs and carbon flux measurements to tourism and community health metrics, to quantify these benefits. Such valuations help policymakers and stakeholders understand the economic and social returns on restoration investments, guiding resource allocation and shaping conservation policies. By making the benefits of restoration more tangible, AI-driven ecosystem service valuation strengthens public support and incentivizes long-term habitat stewardship.
12. Species-Specific Restoration Strategies
Machine learning models can process data on species’ life cycles, microhabitat requirements, and responses to environmental stressors. This leads to tailored restoration actions that better ensure individual species recover and thrive.
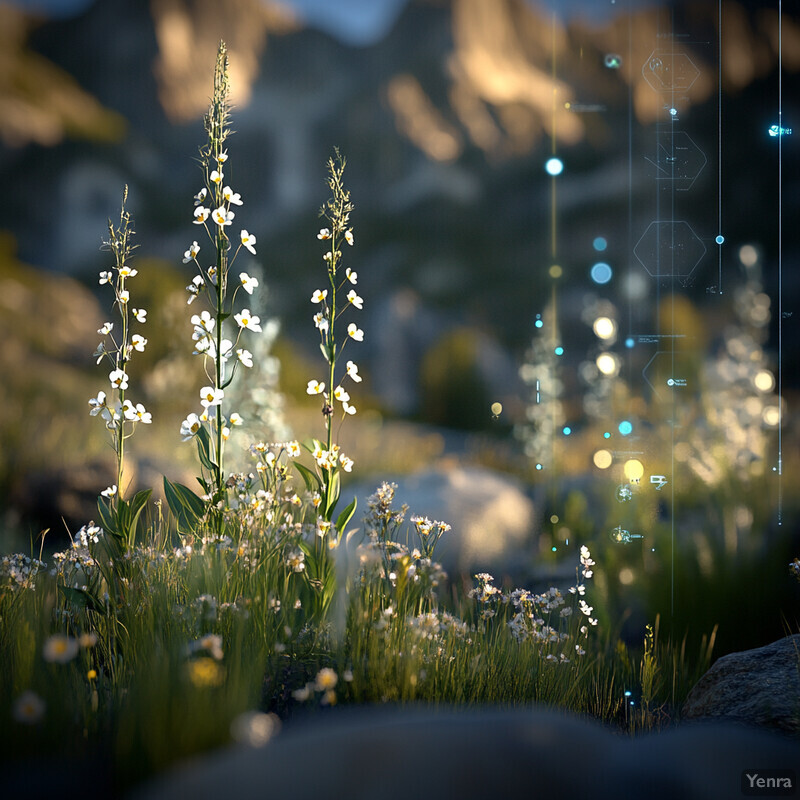
Different species have different ecological roles, habitat preferences, and tolerance thresholds. AI models, trained on species-level ecological data, can highlight the unique conditions each organism needs to survive and reproduce. By incorporating factors such as breeding cycles, food availability, and microclimate conditions, these models help practitioners design targeted interventions to support keystone species or restore critical pollinators. With such customized strategies, restoration efforts can rebuild intricate ecological networks more effectively, ensuring that each species that returns to a restored area fits seamlessly into the broader ecosystem.
13. Early Detection of Stressors
By analyzing remote sensing data and environmental sensors, AI can detect early signs of ecosystem stress—such as drought, disease outbreak in plants, or pollution. Early detection enables preventive measures before irreversible damage occurs.
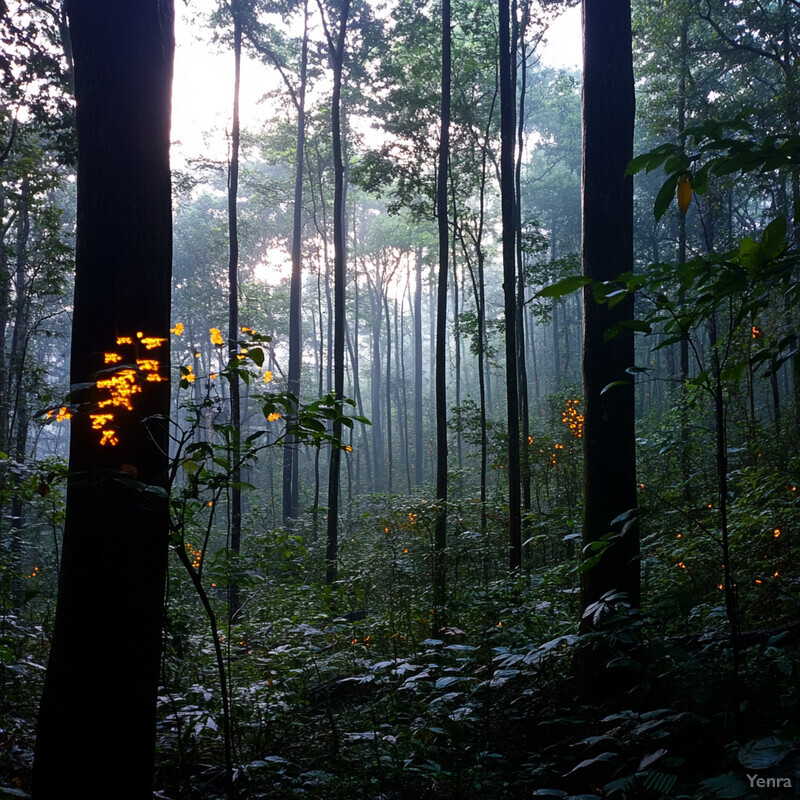
Detecting ecosystem stress—such as nutrient imbalances, pollution events, disease outbreaks, or early drought conditions—can prevent minor issues from evolving into major crises. AI algorithms analyze continuous data streams from sensors, remote imagery, and field reports, identifying anomalies that human observers might miss. By catching these warning signs early, managers can apply timely countermeasures, such as adjusting water inputs, applying natural biocontrol agents, or modifying planting plans. Early intervention reduces restoration setbacks and increases the chances of maintaining stable, thriving habitats in the long run.
14. Behavioral Insights from Bioacoustics
Advanced AI models decode complex animal calls and bird songs, detecting shifts in behavior or community composition. Such insights help restoration ecologists assess the effectiveness of habitat improvements and identify new conservation targets.
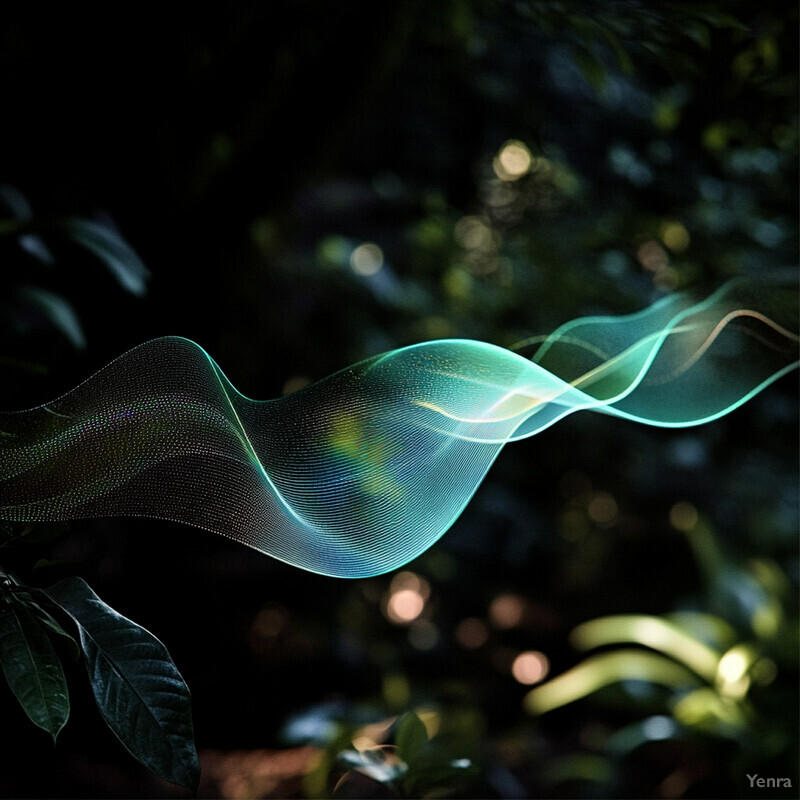
Sound is a rich source of ecological information. AI-based audio analysis can decode complex animal calls, bird songs, and even insect chirps, revealing changes in species abundance or altered community structures. By comparing acoustic data before and after restoration interventions, scientists can track whether the habitat is attracting and retaining the target fauna. Moreover, changes in call frequency, pitch, or timing may indicate stress or shifts in resource availability. Armed with these acoustic insights, managers can refine restoration strategies to better meet the needs of wildlife, thereby improving habitat quality and biodiversity outcomes.
15. Precision Pest and Disease Management
AI can forecast pest outbreaks and disease spread patterns. Armed with this information, managers can use targeted, minimal interventions such as localized pesticide applications or biological controls, thus reducing environmental harm and helping native species recover.
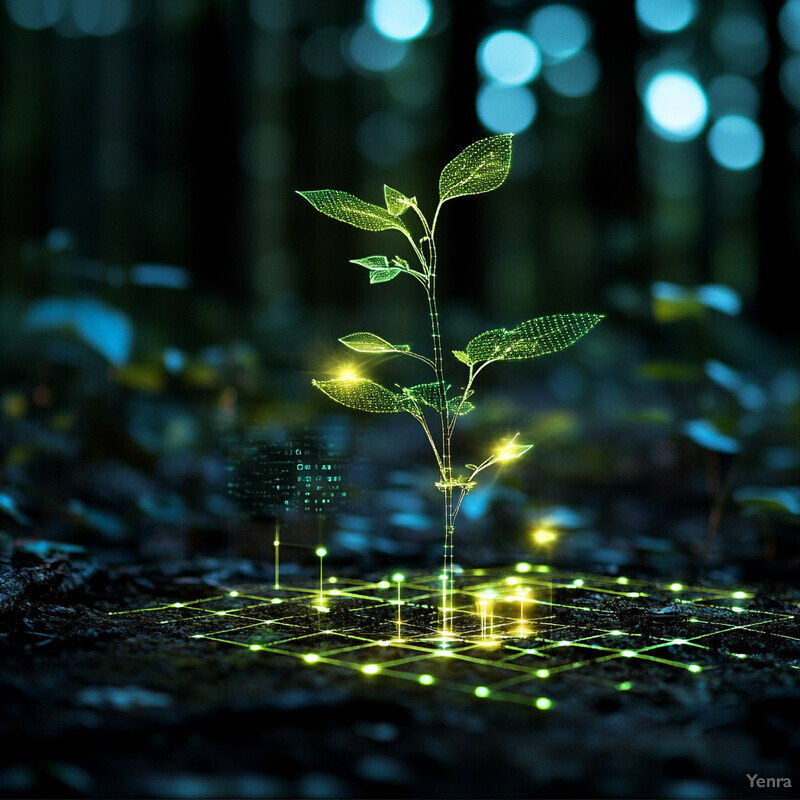
Pests and pathogens can quickly ravage newly planted seedlings or stressed ecosystems. AI-driven models that consider historical outbreaks, climate conditions, host plant distributions, and vector behaviors can forecast where and when pests or diseases are likely to strike. Knowing this, restoration teams can deploy targeted interventions—such as pheromone traps, beneficial predators, or spot applications of eco-friendly pesticides—precisely where they’ll have the greatest impact. By minimizing widespread chemical use and focusing on areas of highest risk, these methods help protect restored habitats from major setbacks and maintain ecological balance.
16. Automated Restoration Equipment
AI-guided machinery can handle complex tasks like selective brush removal, topsoil preparation, or controlled burns. This ensures restoration work is done swiftly, safely, and in a manner that optimally supports ecosystem regrowth.
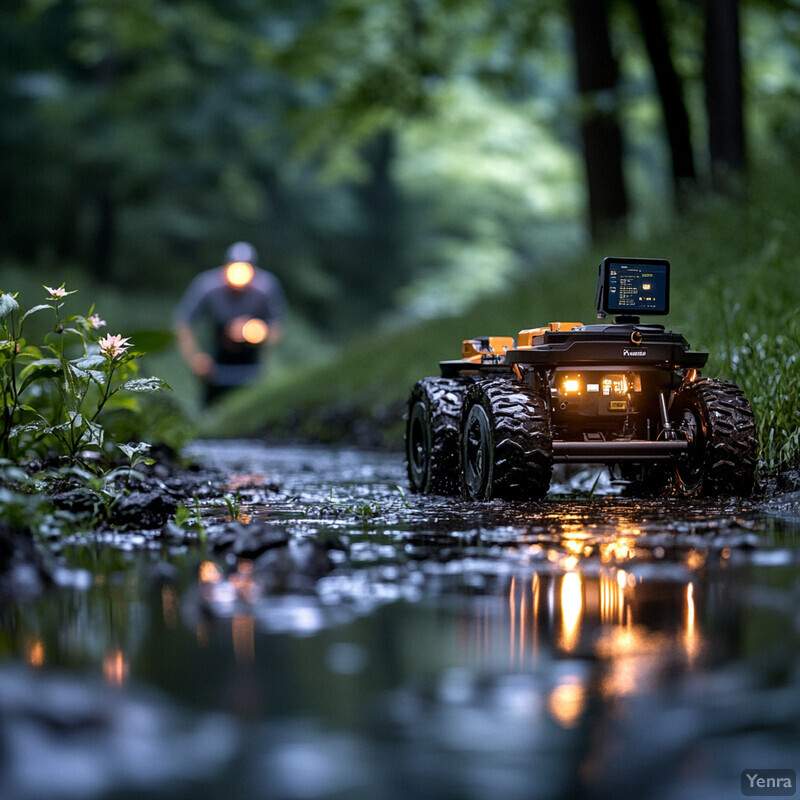
Advances in robotics and AI allow for the development of smart, automated machinery tailored for restoration tasks. For example, autonomous vehicles can navigate rough terrain to perform selective brush clearing, apply mulch layers, or sculpt land contours without human supervision. Machine vision helps these robots distinguish between native and invasive plants, ensuring that only undesirable vegetation is removed. By increasing efficiency, reducing labor costs, and improving safety for human workers, automated equipment speeds up habitat restoration processes and achieves more consistent, high-quality results.
17. Multi-Criteria Decision Support Systems
By integrating diverse datasets (soil health, species presence, climate projections), AI-based decision support tools help stakeholders evaluate trade-offs and select restoration actions that maximize benefits for both biodiversity and human communities.
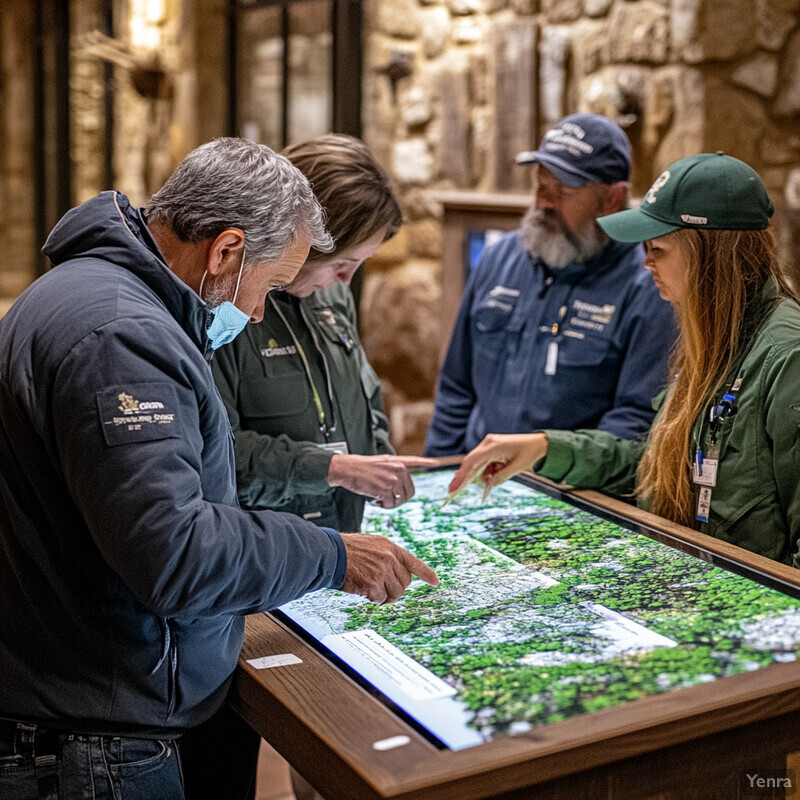
Effective habitat restoration often involves balancing multiple, sometimes conflicting objectives—such as maximizing biodiversity, improving water quality, and supporting local livelihoods. AI-driven decision support systems can integrate large, complex datasets and apply optimization algorithms to evaluate different restoration scenarios. These tools help stakeholders weigh trade-offs, identify win-win situations, and select the most effective strategies based on robust evidence. The result is more informed, transparent, and participatory decision-making that aligns with both ecological goals and human needs.
18. Nutrient Cycle Optimization
AI models can track the flow of nutrients and energy through ecosystems. Restoration practitioners can then choose interventions—like adding certain decomposer organisms or optimizing plant mixtures—to strengthen nutrient cycles and accelerate habitat recovery.
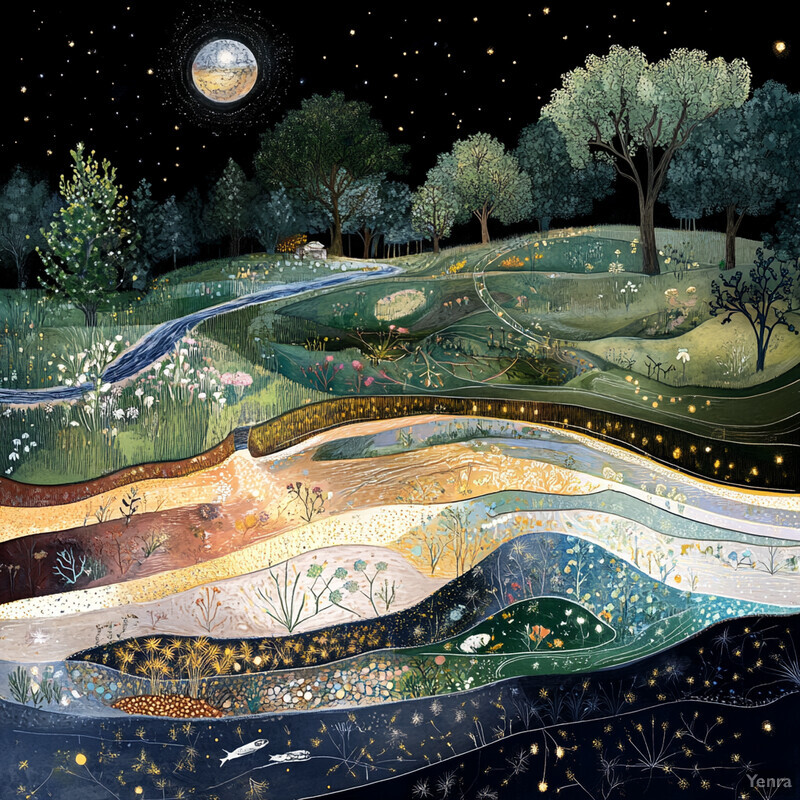
A functioning ecosystem relies on efficient cycling of nutrients—like nitrogen, phosphorus, and carbon—through soil, vegetation, and wildlife. AI can model these biogeochemical cycles, highlighting bottlenecks or imbalances that hinder restoration. By pinpointing where key nutrients are lacking or locked up in unavailable forms, managers can apply targeted interventions, such as introducing soil microbes or planting specific species that fix nitrogen. Fine-tuning nutrient cycles not only accelerates vegetation establishment but also supports a broader range of species and fosters long-term ecological stability.
19. Learning from Past Interventions
By employing machine learning to study historical restoration project outcomes, AI can highlight what works best under particular conditions. Over time, this knowledge base evolves, increasing the success rate of future projects and reducing costs and guesswork.
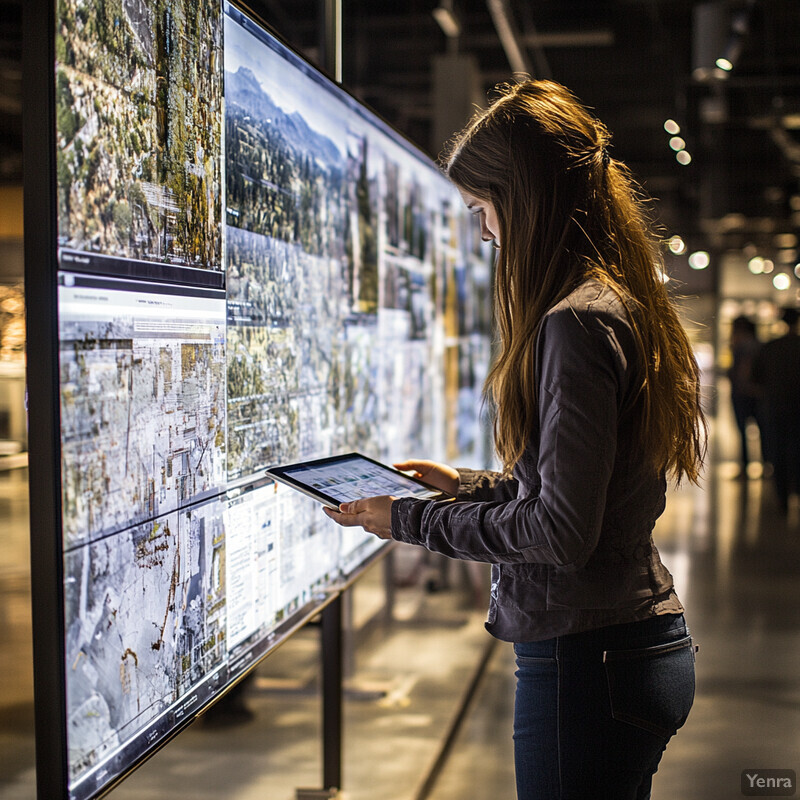
Each restoration project generates valuable insights. AI can analyze historical data—spanning decades of habitat interventions—to reveal patterns in what works and what doesn’t under different environmental conditions. Machine learning models identify successful planting mixtures, effective erosion control techniques, or optimal timing for management actions. As these lessons accumulate, restoration practitioners gain a refined toolkit, reducing trial-and-error approaches and increasing the likelihood of achieving rapid, robust recovery in future projects. This continual learning process fosters adaptive management and elevates the science and practice of restoration ecology.
20. Engaging Local Communities with Predictive Apps
AI-powered mobile tools can help local communities understand how their habitat restoration efforts translate into long-term gains. Predictive applications showcase future landscapes under different restoration scenarios, increasing community support, involvement, and stewardship.
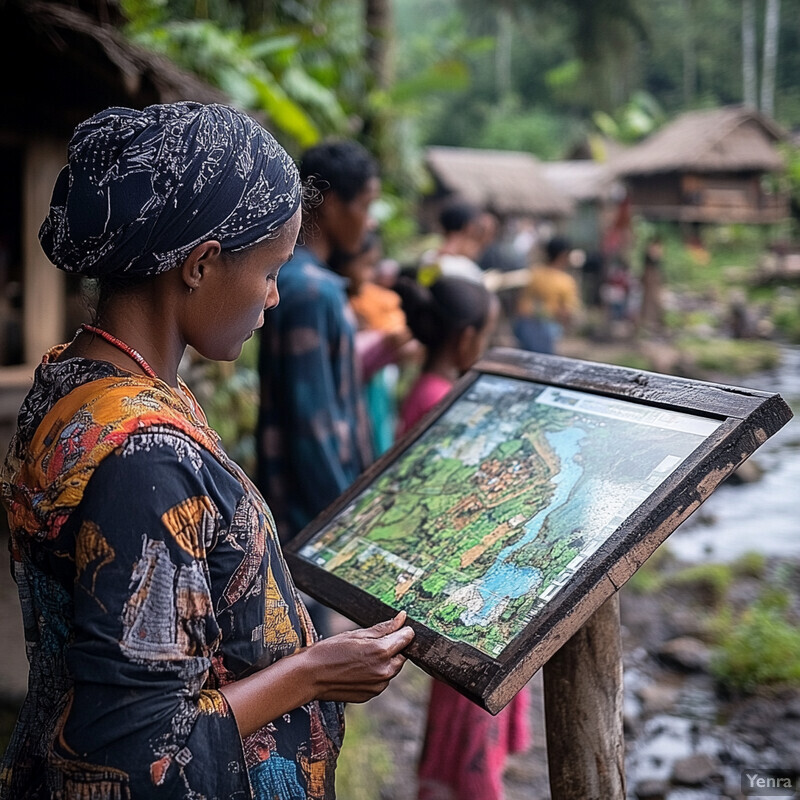
AI-powered mobile applications can visualize future habitat scenarios under different restoration plans, helping local communities understand how their efforts today will shape tomorrow’s landscapes. These user-friendly tools can show projected tree cover, wildlife sightings, or improvements in water quality, fostering a sense of ownership and motivation. By involving local stakeholders in data collection and decision-making, communities become active participants rather than passive observers. This empowerment encourages long-term stewardship, improves compliance with conservation measures, and enhances the overall success and sustainability of restoration initiatives.