1. Real-time Adaptive Process Control
AI-driven controllers can adjust parameters such as temperature, pressure, or flow rates on-the-fly, ensuring each wafer experiences optimal conditions and improving overall process stability.
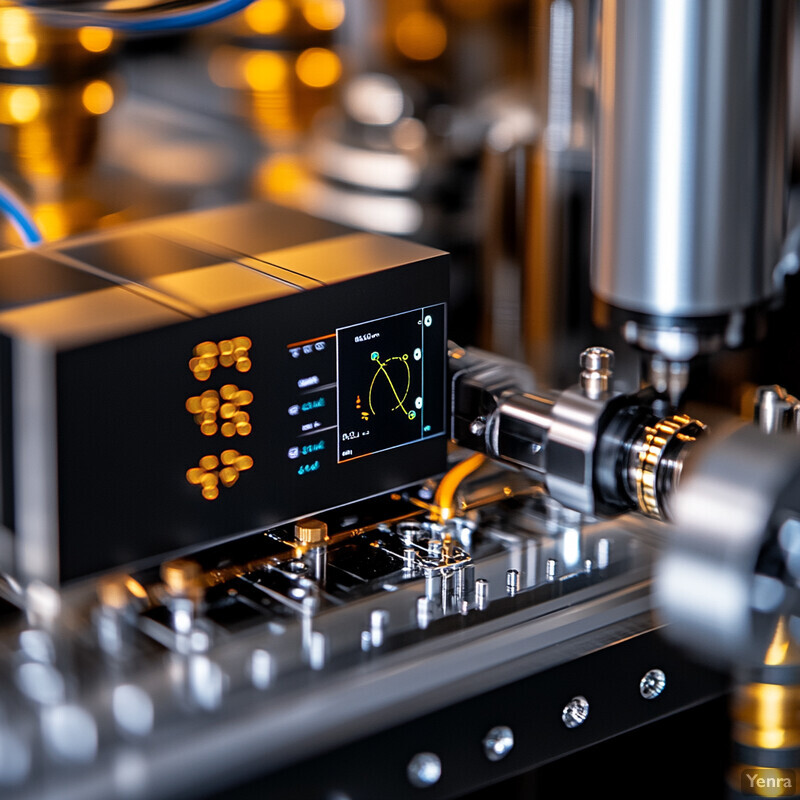
Traditionally, micro-fabrication processes relied on fixed process conditions determined before production began, leaving minimal room for on-the-fly adjustments. With AI-driven real-time adaptive control, sensor data from process chambers is continuously evaluated, enabling instant parameter recalibrations—such as adjusting gas flow, pressure, or temperature—to maintain optimal conditions for each wafer. This dynamic approach helps counteract environmental disturbances, equipment aging, or unexpected material variations. The result is increased consistency in device dimensions, better layer uniformity, and improved overall process stability.
2. Predictive Yield Optimization
Machine learning models can analyze historical and current process data to predict yield outcomes. By proactively adjusting inputs, they help maintain high yields despite subtle environmental variations.
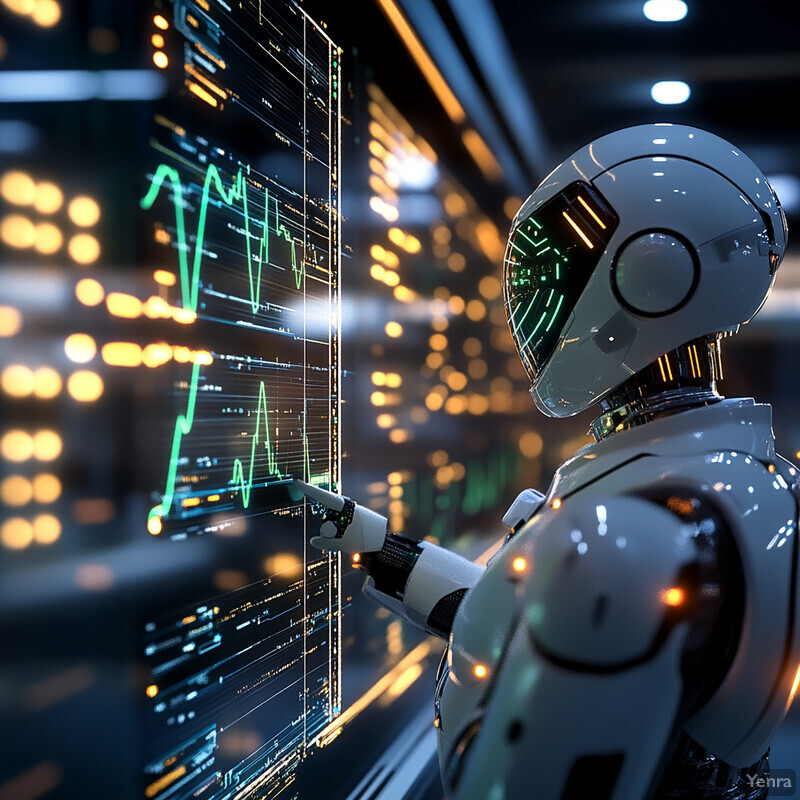
By analyzing historical production data, process recipes, metrology results, and environmental variables, machine learning models can forecast the final yield of ongoing production runs. Using these predictions, adjustments can be made to equipment setpoints, recipe times, or chemical concentrations before defects proliferate. This proactive approach ensures that even minor fluctuations in process conditions are corrected early, helping to maintain consistently high yields. Ultimately, predictive yield optimization reduces waste, improves cost efficiency, and supports faster time-to-market for advanced devices.
3. Defect Detection and Classification
Advanced image recognition algorithms can identify microscopic defects and anomalies in patterning, etching, and deposition steps, enabling quicker interventions and preventing defective batches.
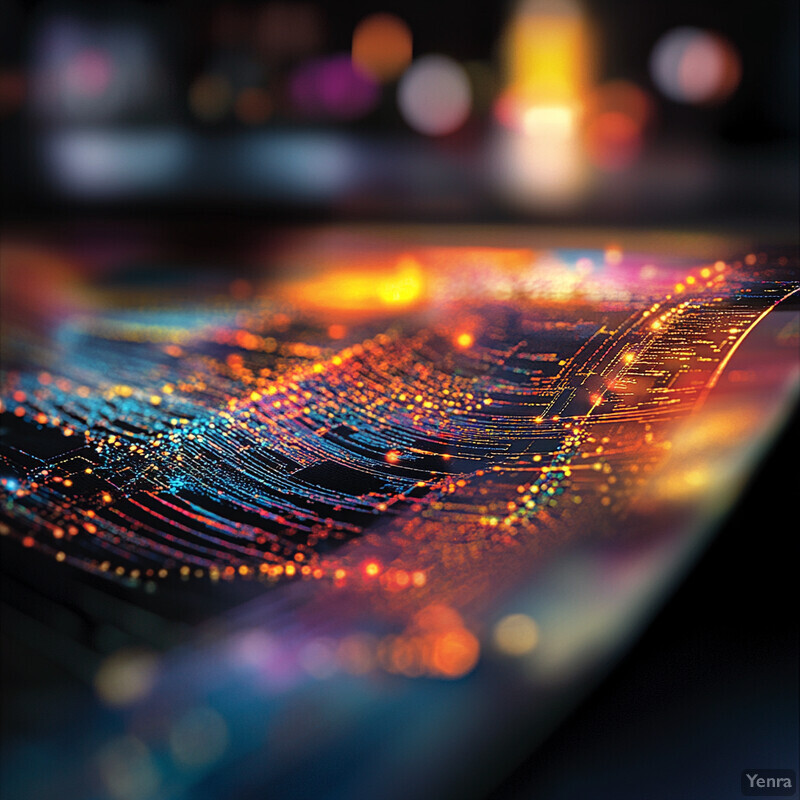
Advanced deep learning algorithms can scrutinize wafer images at nanometer-scale resolutions to identify subtle pattern irregularities, edge roughness, or micro-voids that traditional methods might overlook. Instead of relying solely on basic thresholding, these AI-driven systems learn from large libraries of known defects and can classify them into categories—such as lithography misalignment, etch irregularities, or contamination events. This classification guides process engineers in pinpointing the exact cause of defects and implementing timely countermeasures. As a result, defect densities are minimized, and overall device reliability and performance are enhanced.
4. Virtual Metrology and Reduced Measurement Overhead
AI can infer critical metrics (like line widths or layer thicknesses) without direct measurement, using process and equipment data to estimate metrology results, reducing both costs and cycle time.
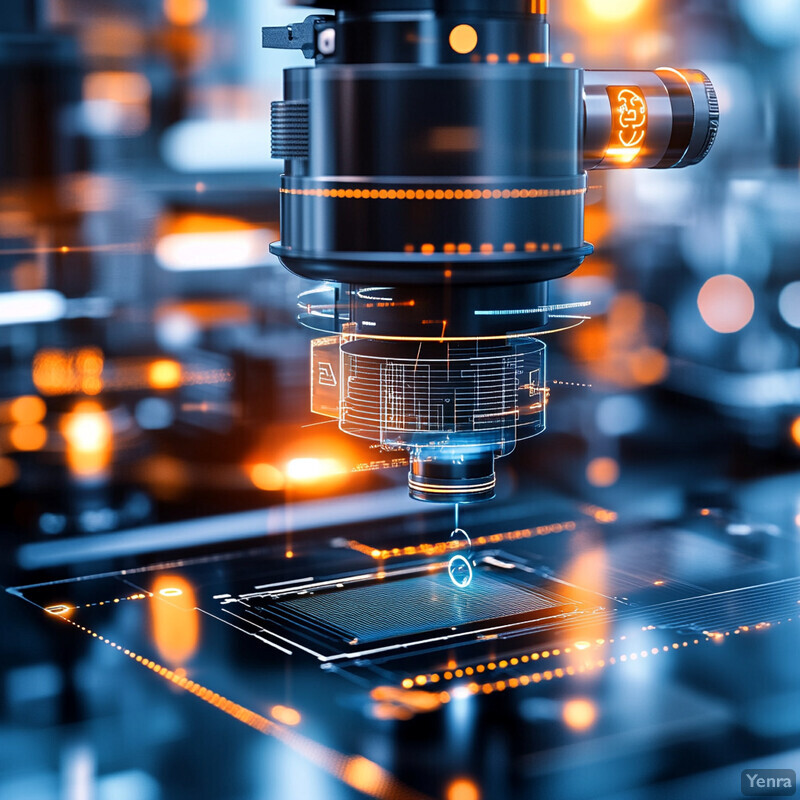
Metrology steps, while critical, add time and cost to production, as every measurement requires removing wafers from the line and using expensive instruments. AI-driven virtual metrology uses process and equipment data to infer critical dimensions, layer thicknesses, and other key parameters without direct physical measurement. Through sophisticated modeling, the system correlates known metrology outcomes with tool parameters, enabling real-time, non-destructive estimation of wafer quality. This reduces the frequency of off-line measurement steps, speeds up the fabrication process, and lowers operating costs while maintaining high product quality.
5. Automated Parameter Optimization
Reinforcement learning and optimization algorithms can explore vast parameter spaces, pinpointing the ideal process recipes for maximum throughput, uniformity, and yield.
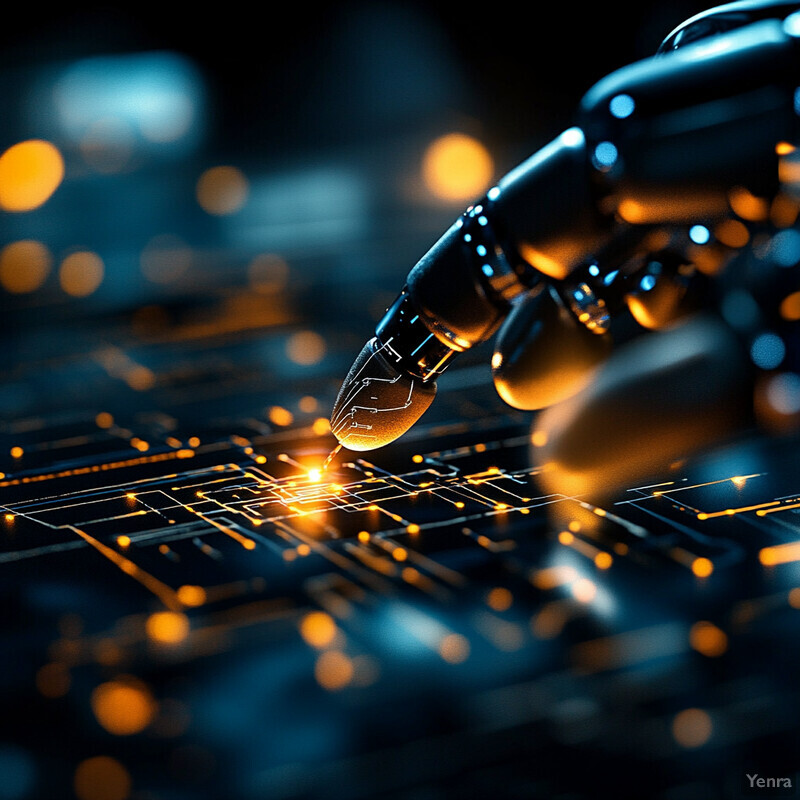
Finding the right process recipe often involves experimenting with a large parameter space—temperature, gas composition, chamber pressure, and more. AI optimization algorithms, such as those using reinforcement learning or genetic algorithms, systematically navigate these variables to converge on the best possible combination. By rapidly testing and refining recipes in simulation or through minimal wafer trials, the system arrives at stable, high-yield conditions more efficiently than human trial-and-error methods. As a result, development cycles shorten, and manufacturing ramps up faster with fewer wasted materials and higher device performance.
6. Predictive Maintenance of Equipment
Analyzing sensor data and tool logs, AI systems detect patterns indicative of imminent equipment failures. Preventative maintenance can then be scheduled to minimize downtime and defect rates.
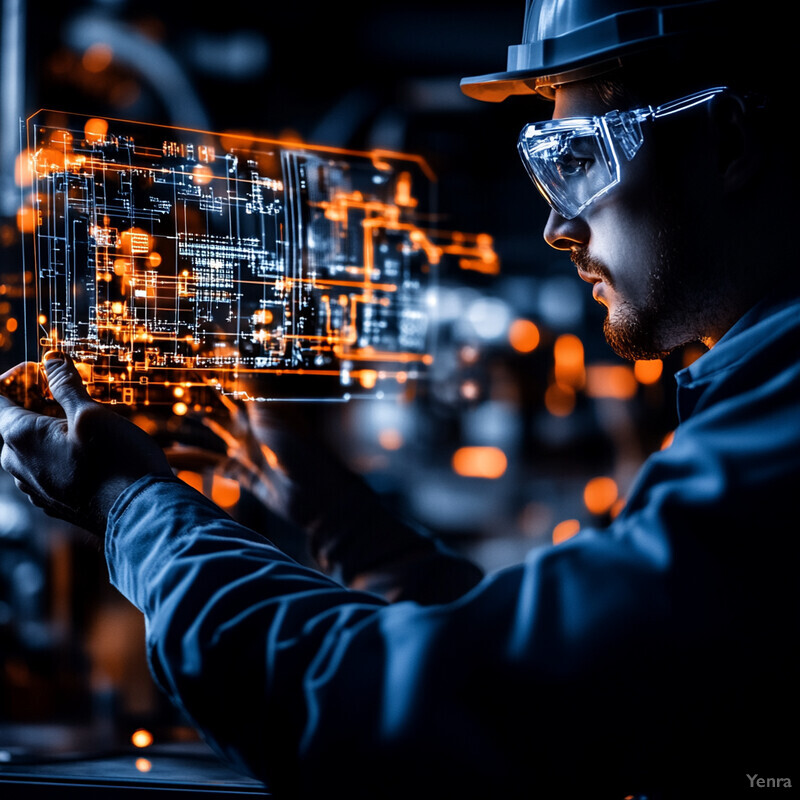
Equipment downtime and unpredictable failures can lead to significant yield losses and wasted production capacity. AI-driven predictive maintenance models monitor large volumes of tool operational data—like vibration signatures, temperature profiles, and sensor signals—to identify precursors to failure. This enables maintenance to be scheduled preemptively, preventing catastrophic breakdowns on critical process tools. The outcome is better equipment utilization, minimized downtime, consistent process quality, and more predictable production cycles.
7. Intelligent Job Scheduling and Resource Allocation
AI can streamline fab operations by optimizing the sequencing of wafer lots, tool allocation, and maintenance activities, thereby reducing cycle time and operational costs.
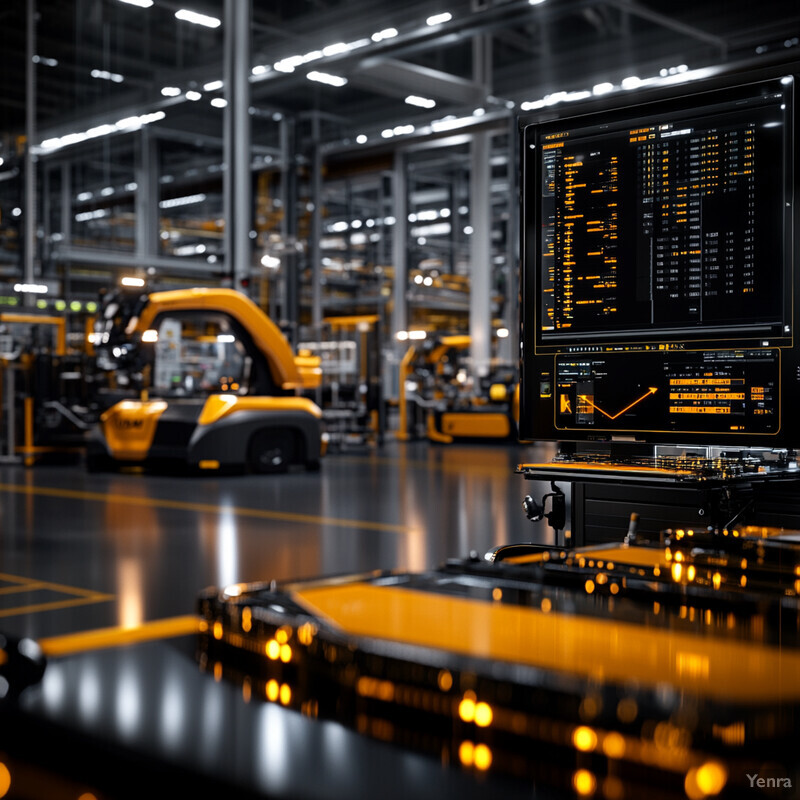
A semiconductor fab is a highly complex environment with hundreds of tools and thousands of wafer lots moving through the production line. AI-based scheduling systems analyze real-time fab conditions, tool availability, and production priorities to optimize the routing of wafers. By minimizing idle times, balancing workloads, and reducing bottlenecks, these systems ensure that the right wafers get processed at the right time. This intelligent orchestration shortens cycle times, boosts throughput, and enhances overall factory efficiency.
8. Early Process Drift Detection
Machine learning models can sense gradual drifts in etch rate, deposition uniformity, or exposure conditions before they lead to significant yield loss, prompting timely corrective measures.
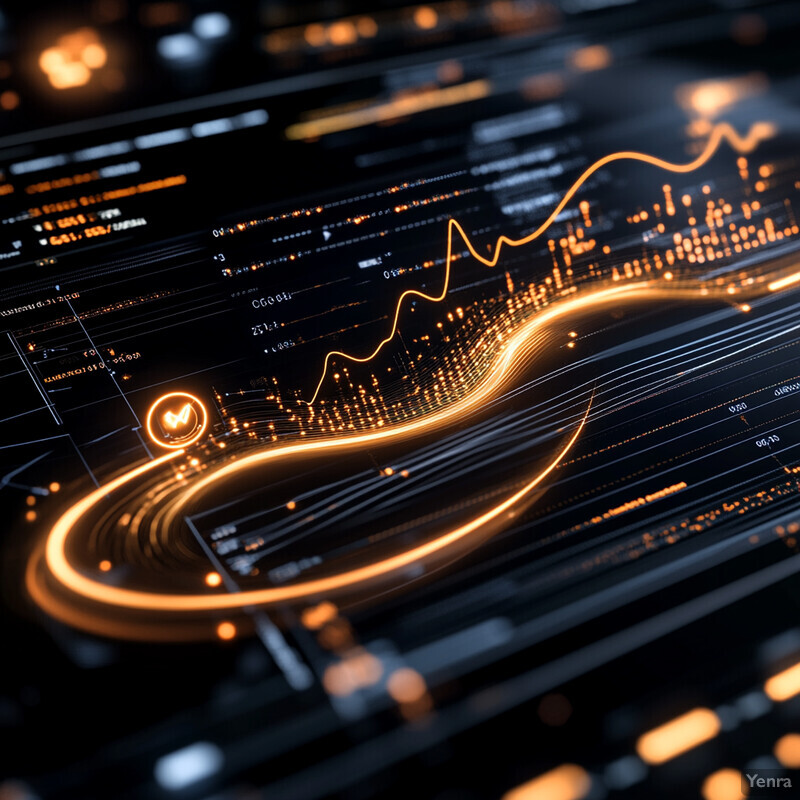
Over time, process conditions can shift slowly due to tool wear, environmental changes, or subtle material inconsistencies. AI-driven drift detection models continuously compare real-time process data against a baseline of optimal operation. When early signs of drift appear—such as slight deviations in etch rate or film thickness—process engineers can step in before yields suffer. This proactive response maintains the desired specifications, keeps production stable, and ensures consistent device performance and quality.
9. Rapid Root Cause Analysis
AI-powered analytics swiftly correlate process anomalies or yield drops to their underlying cause—be it a specific tool, recipe step, or material lot—enabling immediate corrective action.
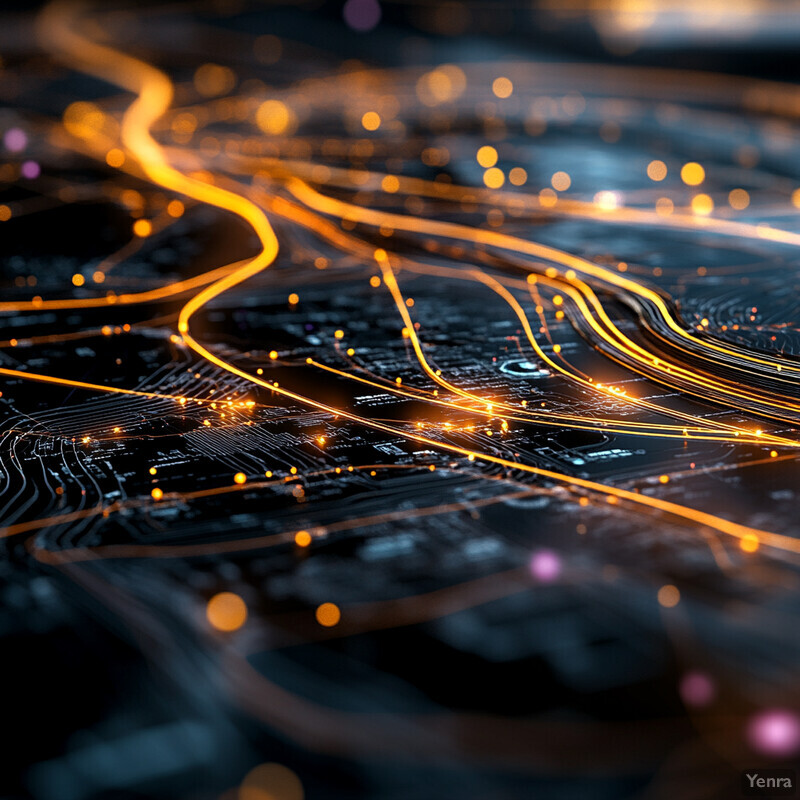
When yield dips or defect patterns emerge, determining the exact cause can be challenging, given the myriad of factors influencing micro-fabrication. AI-driven analytics tools correlate wafer maps, process logs, environmental readings, and equipment performance data to quickly isolate the root cause of anomalies. Instead of spending days or weeks dissecting data manually, engineers gain immediate insights into which tools, steps, or materials triggered the issue. Rapid root cause identification prevents recurring problems, cuts down on production delays, and improves fab-wide learning.
10. Equipment Health Monitoring
Continuous sensor data analysis by AI helps maintain equipment in optimal operating conditions, identifying minor degradations that can impact process uniformity or equipment availability.
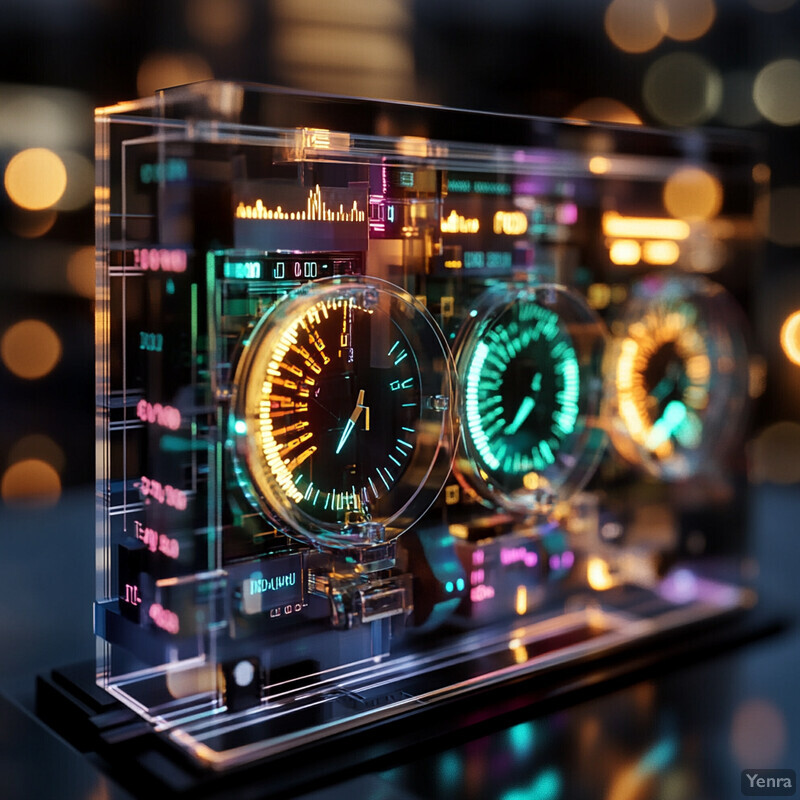
Just as the human body shows subtle signs before full-blown illness, equipment exhibits small anomalies before serious malfunctions occur. AI-based health monitoring systems analyze a wide array of signals—pressure stability, motor current patterns, gas flow uniformity—to spot these early warning signs. By focusing on subtle trends rather than waiting for overt breakdowns, maintenance teams can perform targeted interventions. This ensures process tools remain at peak performance, maintaining consistent quality and reducing costly, unplanned stoppages.
11. Cleanroom Environmental Control
AI-driven environmental models can detect subtle shifts in humidity, temperature, or particulate levels that influence process outcomes, guiding climate systems to maintain stable conditions.
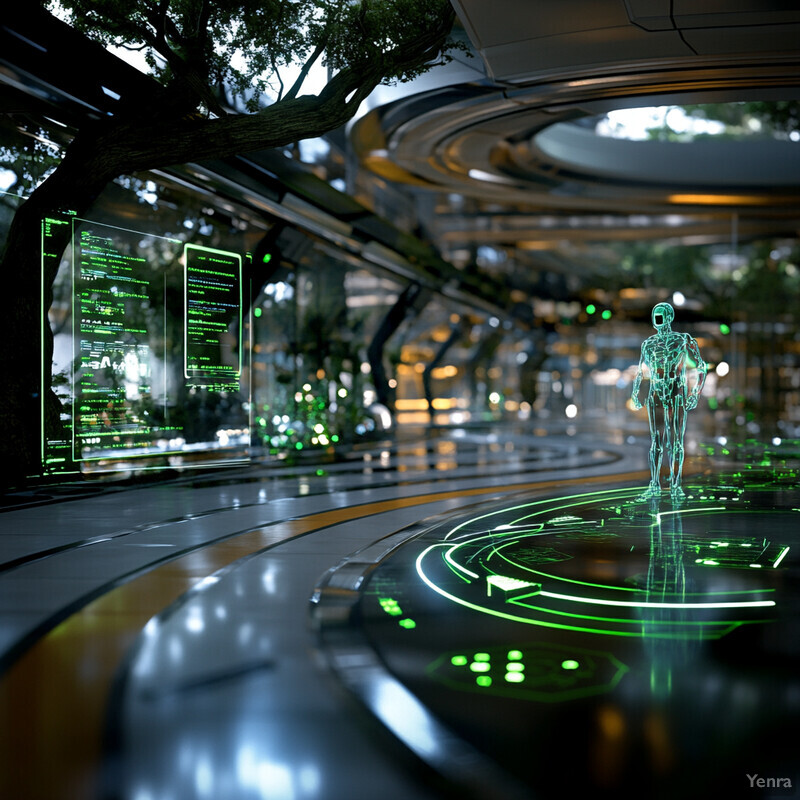
The micro-fabrication environment is controlled to extraordinary precision, with stringent requirements on temperature, humidity, and contamination levels. AI-driven environmental models integrate sensor inputs, weather predictions, and operational schedules to predict the impact of slight fluctuations on yield. Adjusting HVAC settings, airflow patterns, or filter replacement schedules ensures stable conditions that prevent particle contamination or device variability. Ultimately, maintaining a pristine environment improves yield consistency and device reliability.
12. Overlay and Alignment Enhancement in Lithography
Deep learning techniques improve pattern alignment and overlay accuracy by instantly adjusting exposures, lens focus, and wafer positioning, crucial for nanoscale pattern fidelity.
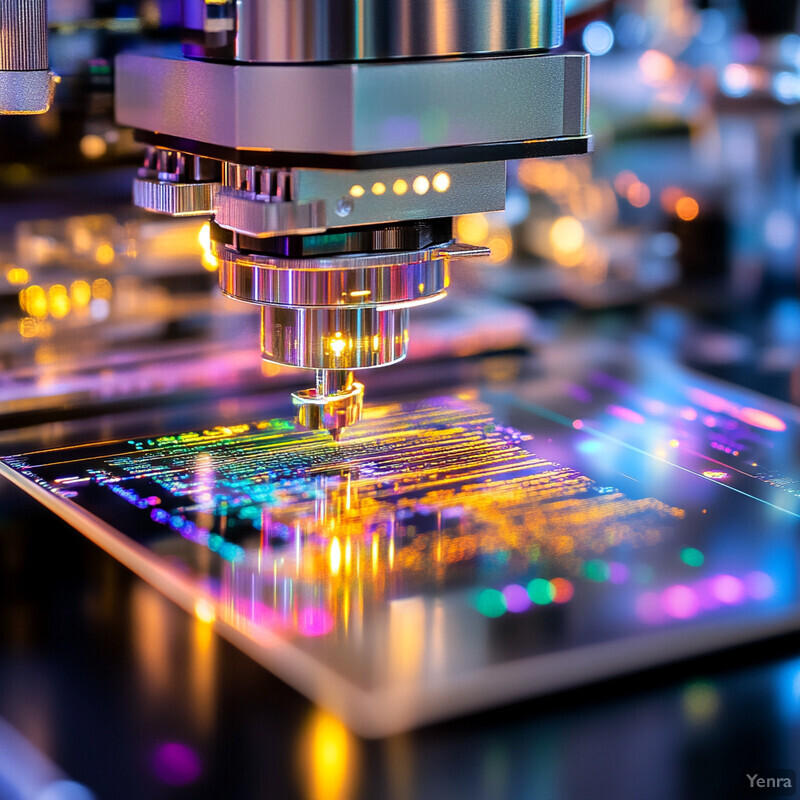
As device features shrink to the nanometer scale, even minuscule misalignments between layers can compromise yield. AI-assisted lithography control uses deep learning to analyze alignment marks, tool-specific signatures, and real-time image data to refine positioning and focus adjustments. By dynamically compensating for tool drifts, lens distortions, and wafer warpage, these systems ensure near-perfect overlay of successive layers. High overlay accuracy leads to better device performance, reduced rework, and overall improved process capability.
13. Advanced Wafer Map Pattern Recognition
Machine learning can detect complex defect distribution patterns across wafers, pointing to systemic process issues or tool malfunctions that would be hard to identify by manual inspection.
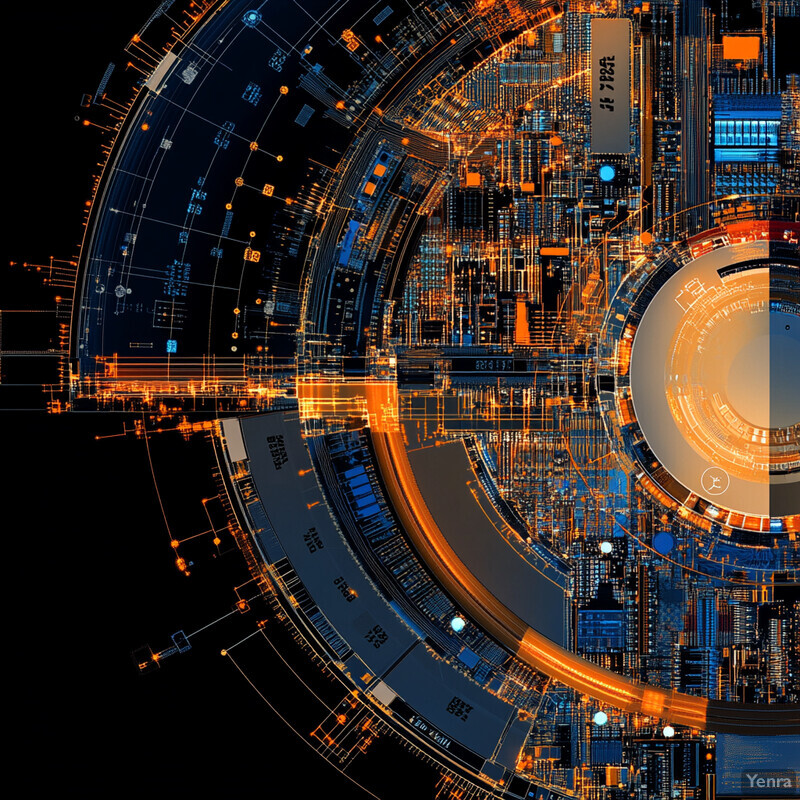
Defects often appear in patterns across wafers—rings, clusters, gradients—indicating specific sources of variation. AI pattern recognition algorithms identify these complex spatial arrangements to correlate defects with root causes. For instance, a ring-like pattern might suggest a gas distribution issue in an etch chamber, while cluster defects may point to lithography focus errors. Understanding these patterns improves troubleshooting efficiency and drives targeted process improvements that enhance yield and reduce defectivity.
14. Neural Network-Based APC (Advanced Process Control)
Deep neural networks model nonlinear process relationships, allowing more precise setpoint adjustments and tighter control loops for plasma etching, chemical vapor deposition, and more.
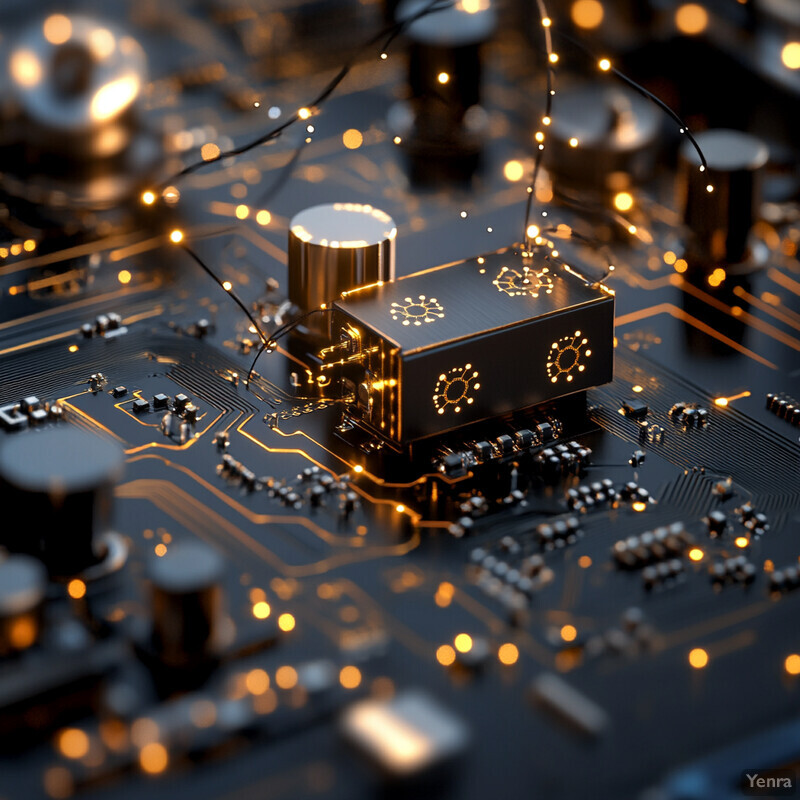
Classical process control models may not fully capture the nonlinear, interdependent relationships among process parameters. Neural networks excel at modeling these complex interactions, enabling more precise and nuanced APC strategies. By better predicting how changes in one parameter affect others, the system continuously refines control loops for deposition, etching, or plating steps. This advanced approach ensures tighter control, higher yield, and the ability to push device performance to cutting-edge limits.
15. Accelerated Process Simulation
AI-augmented simulations enable faster, more accurate modeling of multi-physics phenomena in deposition and etch processes, guiding engineers to optimal conditions without exhaustive trial-and-error.
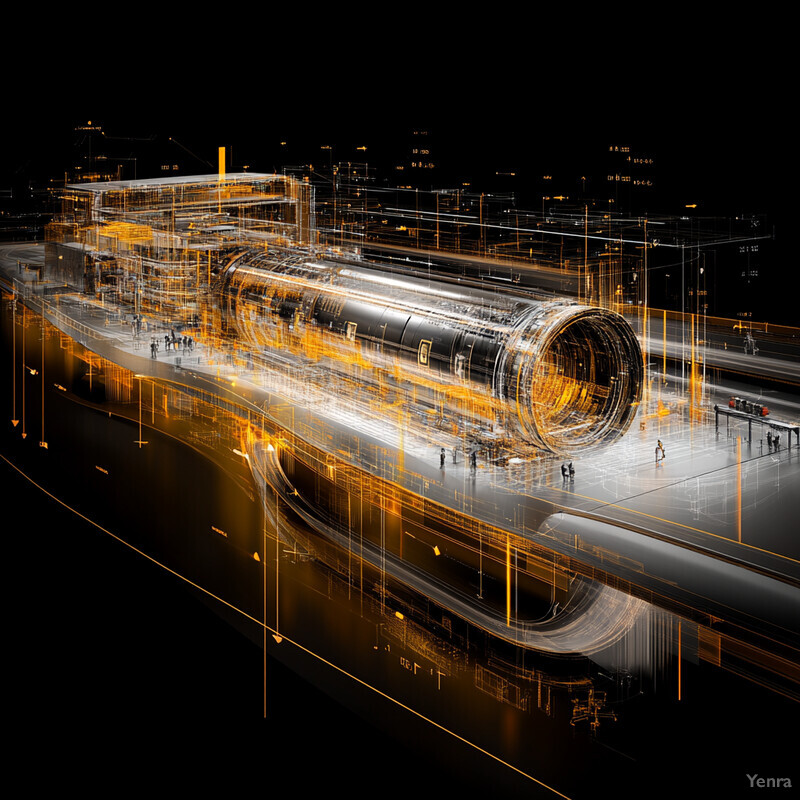
Process simulation tools help engineers understand the effects of recipe variations without expensive and time-consuming experiments. AI-enhanced simulation models rapidly process huge datasets and incorporate learning from previous runs to predict outcomes more accurately. By reducing the need for extensive trial-and-error wafer runs, engineers can quickly validate new materials, chemistries, or process conditions. This cuts down on development costs, accelerates innovation, and helps bring new technologies to market faster.
16. Cross-Parameter Correlation Discovery
Unsupervised learning can reveal hidden correlations between parameters (e.g., gas flow, chamber pressure, substrate temperature) that affect end results, informing better recipe design.
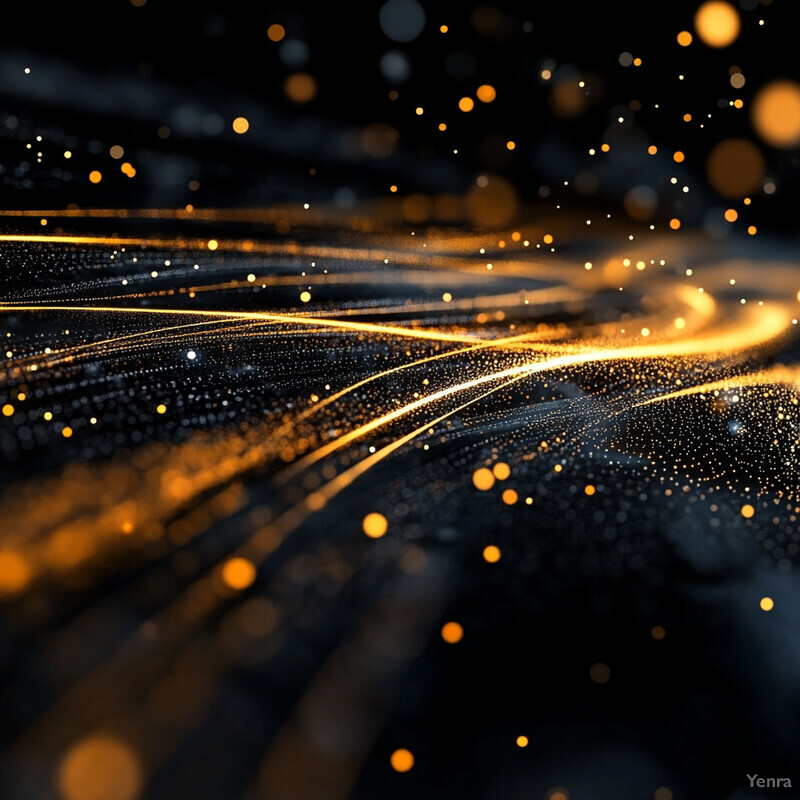
Micro-fabrication involves hundreds of variables—some obvious, others hidden—that collectively determine the quality of the final device. AI algorithms, especially unsupervised learning methods, sift through massive datasets to uncover correlations not initially considered. For example, a slight variation in chamber humidity might interact with gas chemistry to affect film density. By identifying these hidden relationships, engineers can refine recipes and control strategies, leading to more robust and repeatable processes.
17. Self-Calibration of Metrology Tools
AI-guided calibration routines reduce downtime and improve measurement accuracy by learning from historical instrument data and adjusting tools accordingly.
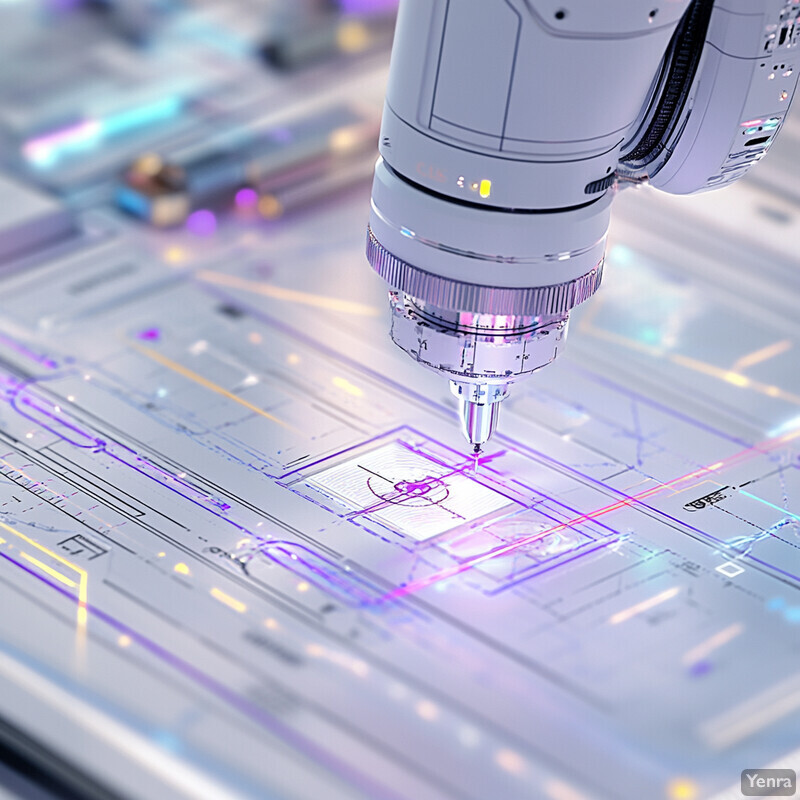
Metrology equipment must be rigorously calibrated to deliver accurate measurements, but manual calibration is time-consuming and susceptible to human error. AI-enabled self-calibration monitors the drift in measurement tools, comparing their readings against known references and historical performance. It then suggests or automatically applies calibration adjustments in real-time. This continual fine-tuning ensures measurement accuracy, reduces downtime, and improves confidence in process control decisions.
18. Enhanced Fault Detection and Classification (FDC)
Advanced classification algorithms reduce false alarms, ensuring that line stops or recipe changes happen only when genuinely needed and not due to spurious sensor noise.
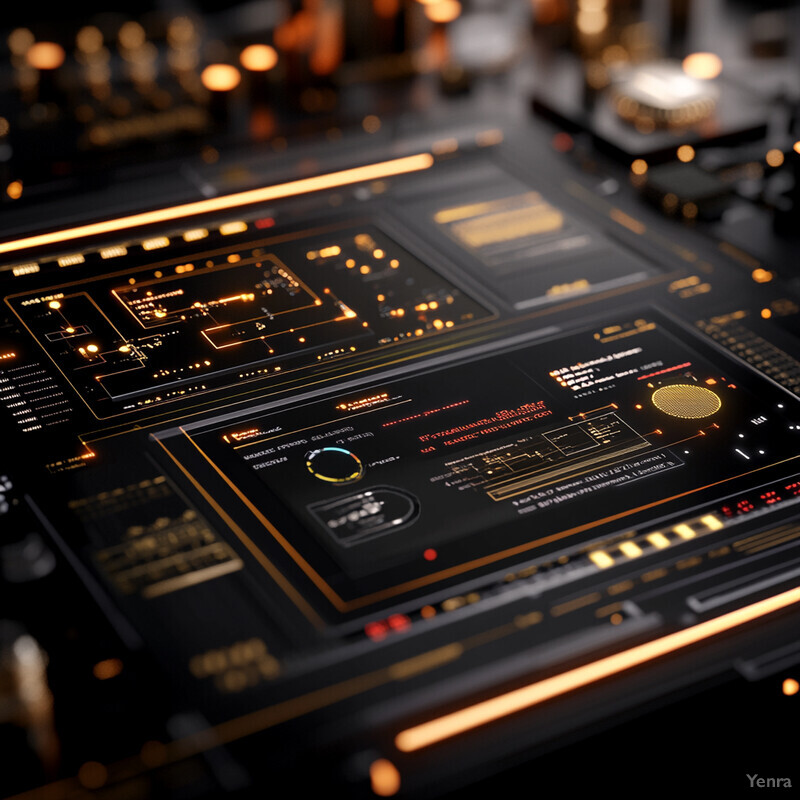
Tools in a fab generate continuous streams of sensor and operational data. AI-powered FDC systems analyze this data to identify anomalies that may indicate process faults. More sophisticated than simple threshold-based alarms, these models learn normal operating conditions and detect subtle deviations. By reducing both missed faults and false alarms, FDC driven by AI improves fab efficiency, minimizes unnecessary tool stoppages, and ensures reliable and stable operations.
19. Dynamic Recipe Tuning
AI agents continuously refine process recipes to accommodate wafer-to-wafer variations, material quality shifts, and evolving equipment performance, maintaining consistently high yield.
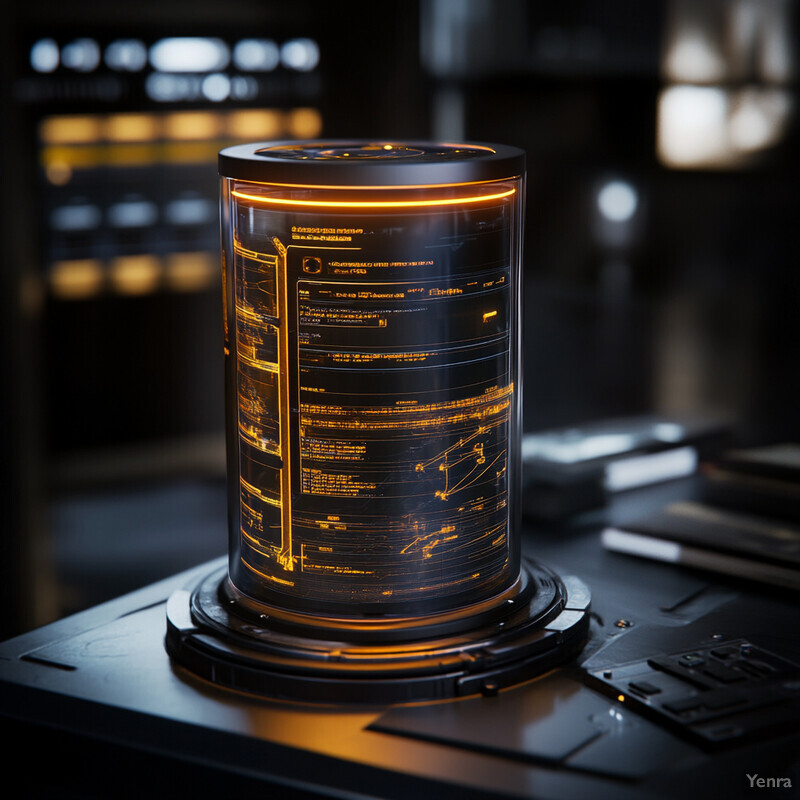
As raw material batches or environmental conditions change, sticking to a static process recipe can degrade yield over time. AI systems dynamically adjust recipes to compensate for these variations—fine-tuning temperatures, flow rates, or exposure times in response to wafer-level feedback. This ensures that each wafer receives the ideal process conditions, improving within-lot and lot-to-lot uniformity. The net effect is consistent high yield, better device performance, and reduced operator intervention.
20. Integration with Digital Twins
AI works in tandem with digital twins of fabrication lines, running virtual experiments to predict outcomes, test improvements, and implement changes in the actual production environment with minimal risk.
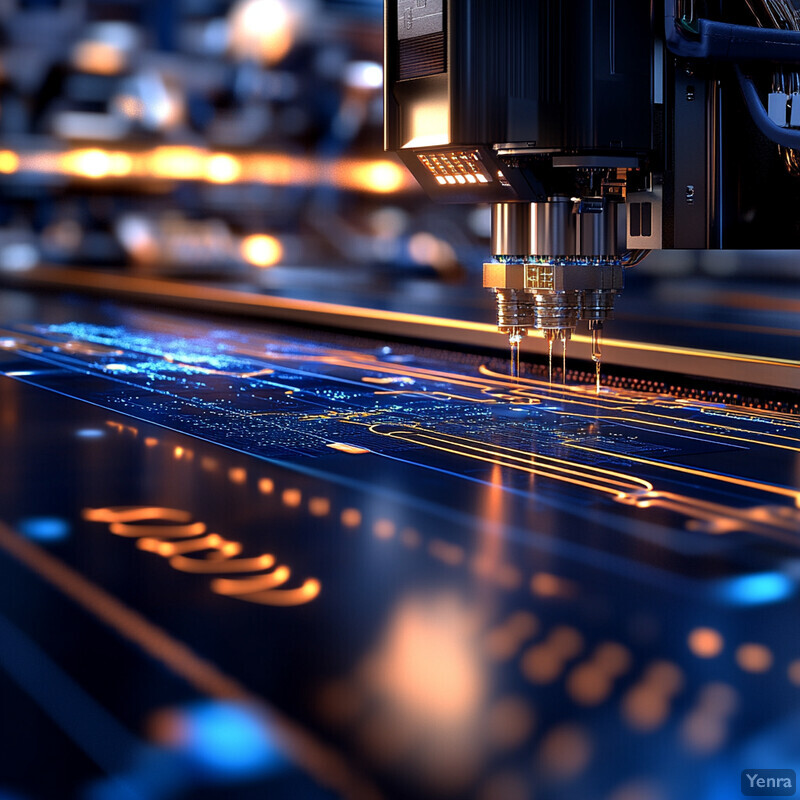
A digital twin is a virtual replica of the physical fab environment, including tools, recipes, and workflows. AI-driven digital twins simulate production scenarios in real-time, evaluating the impact of changes in process conditions, equipment configurations, or scheduling strategies before implementing them on the actual line. Engineers can run “what-if” analyses, test process improvements, and validate new recipes digitally with minimal risk. This synergy of AI and digital twins leads to more informed decisions, faster problem-solving, and continuous improvement in the complex world of micro-fabrication.