1. Predictive Soil Moisture Modeling
AI-driven models can predict future soil moisture levels based on variables like current moisture readings, crop types, soil composition, and upcoming weather forecasts. This helps farmers apply water precisely when plants need it.
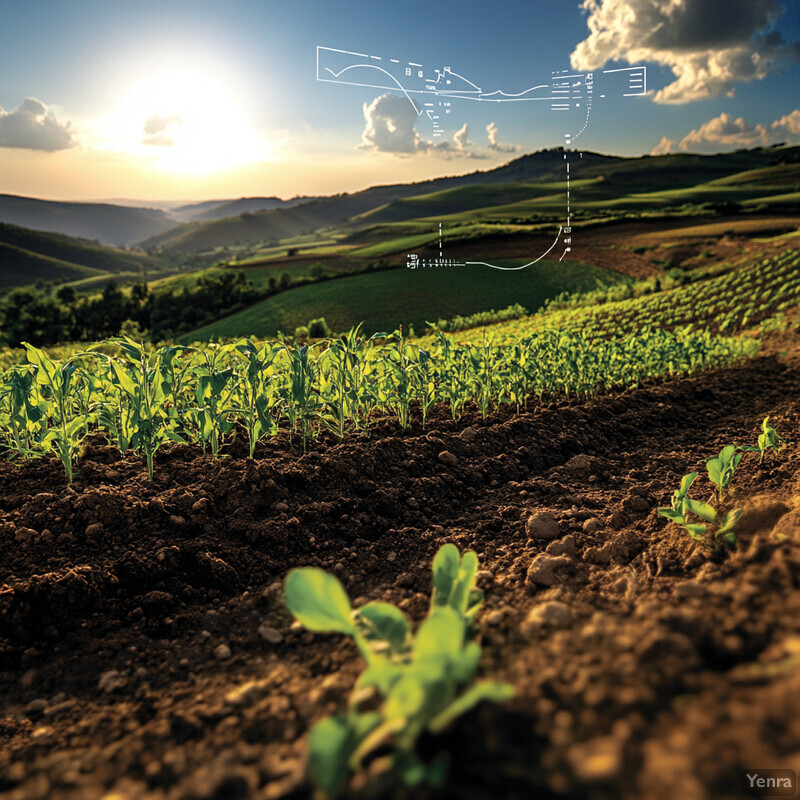
AI-based predictive soil moisture modeling employs advanced algorithms that take into account current soil moisture readings from sensors, along with soil texture, porosity, and organic content. By analyzing these parameters in combination with future weather forecasts—such as rainfall predictions, temperature fluctuations, and humidity levels—AI models can estimate future water availability in the soil. This predictive capability allows farmers to schedule irrigations more precisely, providing just enough water at the right time rather than adhering to fixed intervals. The result is more efficient water use, healthier plant growth, and ultimately higher yields due to consistently optimized soil moisture conditions.
2. Machine Learning-Based Weather Forecast Integration
Advanced machine learning algorithms can integrate high-resolution weather forecasts and climate models to estimate evapotranspiration rates, rainfall probabilities, and temperature changes, allowing for proactive adjustments in irrigation schedules.
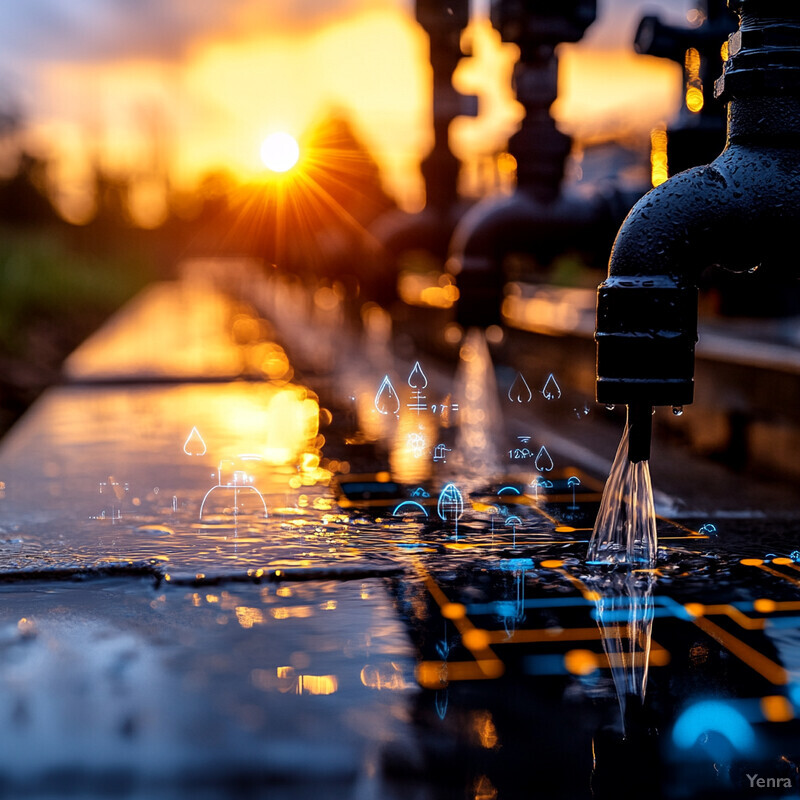
Machine learning systems excel at processing large volumes of data, including high-resolution meteorological information like radar images, satellite data, and localized climate patterns. By integrating these detailed forecasts into irrigation models, AI can predict evapotranspiration rates—the combination of water loss from soil and plant surfaces—and even anticipate unusual weather events well in advance. This integration ensures that irrigation schedules are adjusted dynamically to either capitalize on upcoming rainfall or compensate for dry, hot spells. The improved accuracy leads to water savings, reduced runoff, and an enhanced ability to maintain consistent plant growth conditions.
3. Crop-Specific Irrigation Prescriptions
AI can account for the unique water needs and growth stages of different crops, learning from past yield data and agronomic recommendations, thereby creating tailor-made irrigation timetables that optimize plant health and maximize yield.
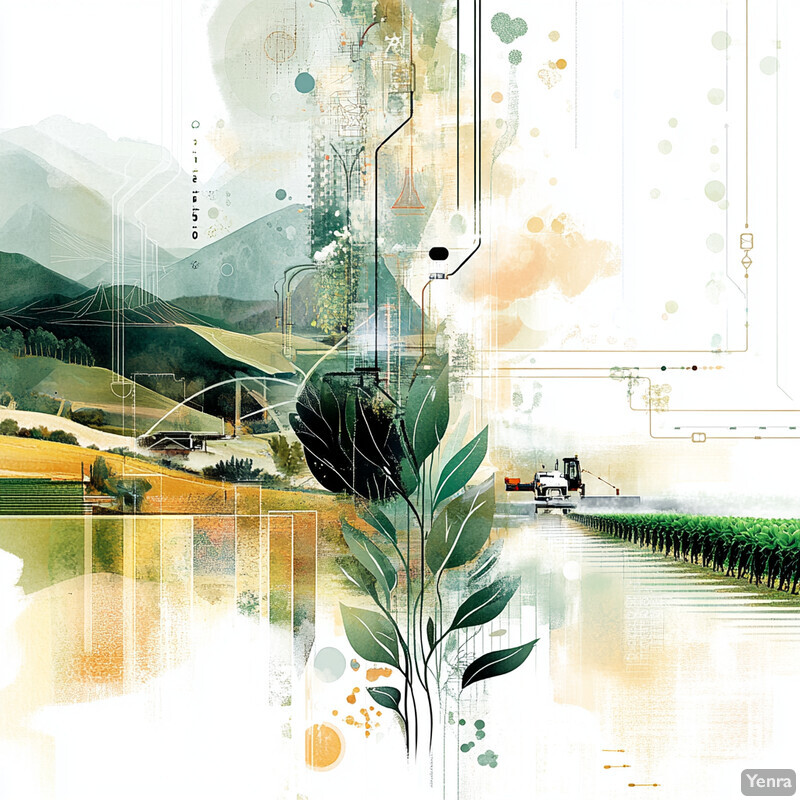
Different crops have distinct water demands throughout their growth cycles. By training on historical yield data, soil characteristics, and the physiologic water needs of specific plant species, AI can produce highly customized irrigation schedules. These schedules take into account the crop’s developmental stage—such as germination, vegetative growth, flowering, and fruiting—ensuring that water is supplied in the ideal amounts at each critical stage. Crop-specific prescriptions help maintain optimal plant health, reduce water waste, and can even enhance flavor, size, and nutritional quality of produce by matching water supply to the crop’s exact requirements.
4. Adaptive Scheduling Through Reinforcement Learning
Reinforcement learning techniques enable the system to “learn” from real-world feedback, continually refining its irrigation decisions to balance water savings with crop performance.
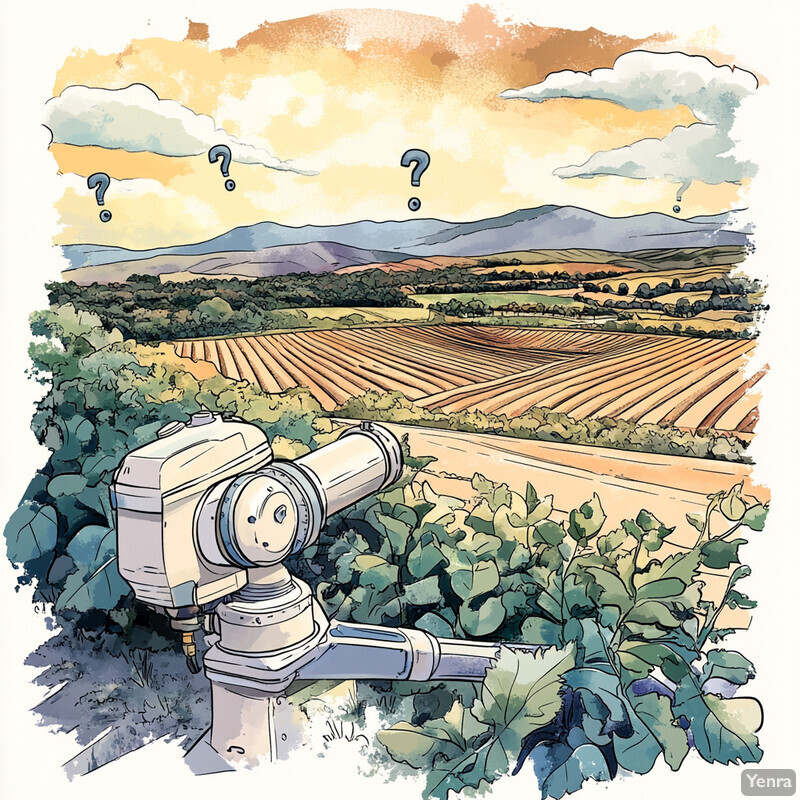
Reinforcement learning (RL) algorithms learn by doing, adjusting their strategies based on feedback from the environment. In the context of irrigation scheduling, the RL agent starts with a baseline strategy and then fine-tunes its approach by interacting with real-world conditions—evaluating outcomes such as plant growth, water use efficiency, and yield. Over multiple growing seasons, the RL system refines its policies to reduce water consumption while maintaining or improving yield. This adaptive, trial-and-error learning process creates a cycle of continual improvement, ensuring that the irrigation regimen evolves along with changing environmental conditions and farm objectives.
5. Precision Integration With Soil and Plant Sensors
AI can fuse data from soil moisture probes, root-zone sensors, and leaf wetness sensors, enabling the irrigation system to fine-tune water application at the plant and subfield level.
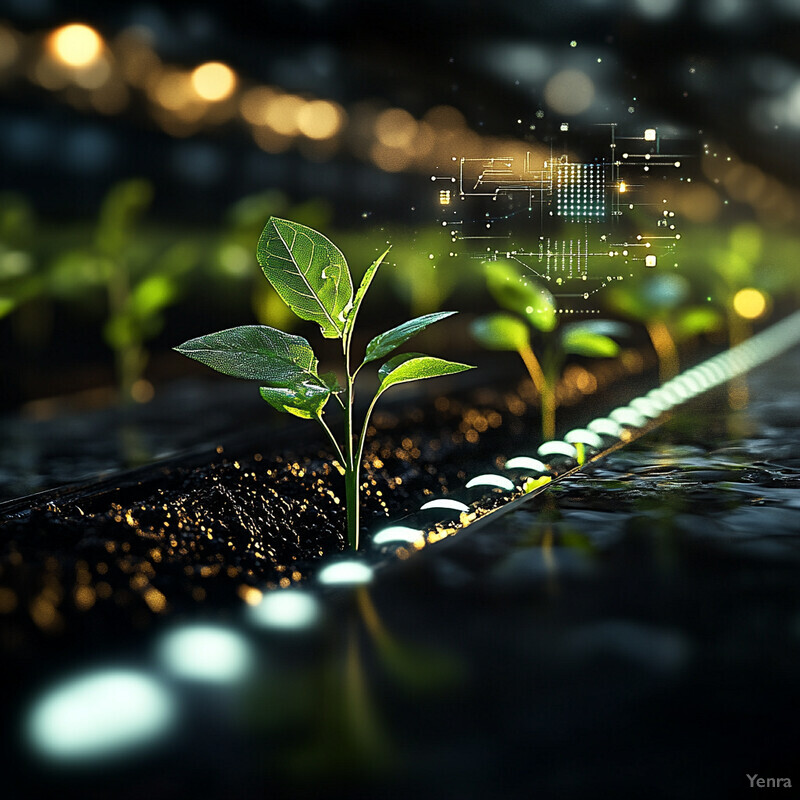
AI-driven irrigation leverages networks of sensors placed both in the soil and within the plant canopy. Soil moisture probes provide granular data on water content at varying depths, while leaf and stem sensors detect subtle signs of plant stress. Combining this data, AI algorithms can pinpoint exactly when and where water is needed, delivering irrigation not only at the field level but down to the individual plant zone. Such precision ensures that no area is over-watered or under-watered, leading to uniform plant growth, minimized nutrient leaching, and reduced risks of root diseases or waterlogging.
6. Remote Sensing and Satellite Imagery Analysis
By using computer vision and deep learning techniques to analyze high-resolution satellite imagery, drones, or hyperspectral images, AI can detect crop stress levels and vegetation indices, informing more precise irrigation timing.
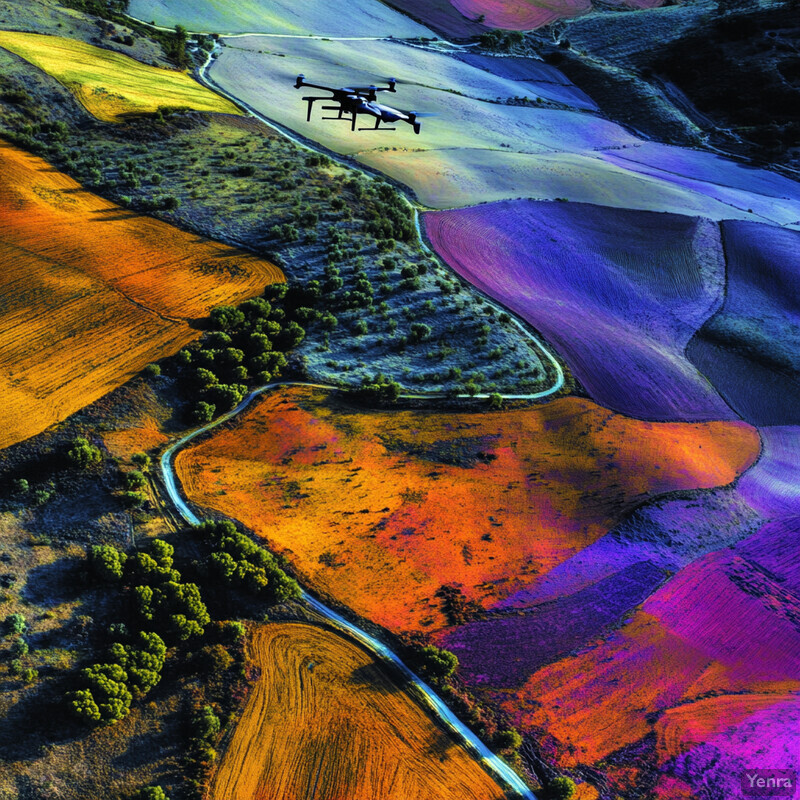
High-resolution imagery from satellites, drones, or hyperspectral cameras offers valuable insights into crop health. AI-based image processing and computer vision techniques can interpret color patterns, vegetation indices (like NDVI), canopy temperature differences, and even subtle spectral signatures indicating water stress. By correlating these visual indicators with on-the-ground sensor data, AI can predict where irrigation is needed and by how much. The ability to cover large areas from above makes remote sensing analysis highly scalable, delivering insights to large farms or even entire agricultural regions.
7. Real-Time Optimization With IoT Connectivity
Combining AI-driven models with IoT-enabled irrigation hardware allows dynamic, on-the-fly adjustments, ensuring irrigation is never fixed but constantly responsive to changing conditions.
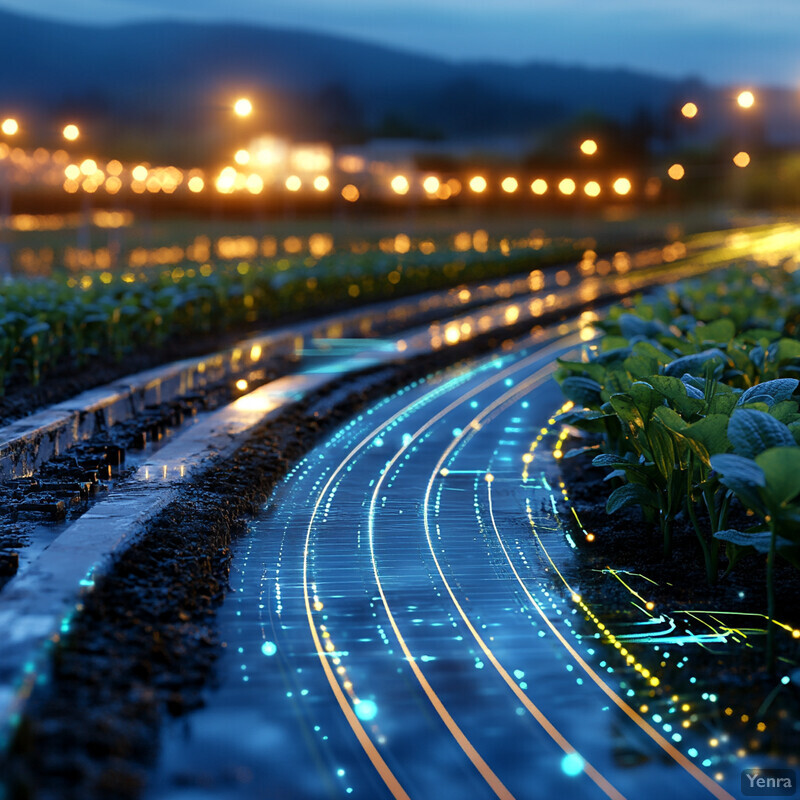
Irrigation systems connected via the Internet of Things (IoT) allow pumps, valves, and drip lines to be controlled remotely and adjusted in real-time. AI algorithms, continuously fed with sensor data and forecasts, can send instant commands to hardware components, opening or closing valves at just the right moment. This dynamic responsiveness ensures that irrigation schedules are never static; instead, they are fluid and adaptable. The result is a system that can reduce water waste during unexpected rain events, rapidly respond to signs of plant stress, and optimize resource usage without waiting for human intervention.
8. Seasonal and Long-Term Trend Analysis
Advanced time-series analysis techniques can identify seasonal and year-to-year shifts in water requirements, informing strategic irrigation planning.
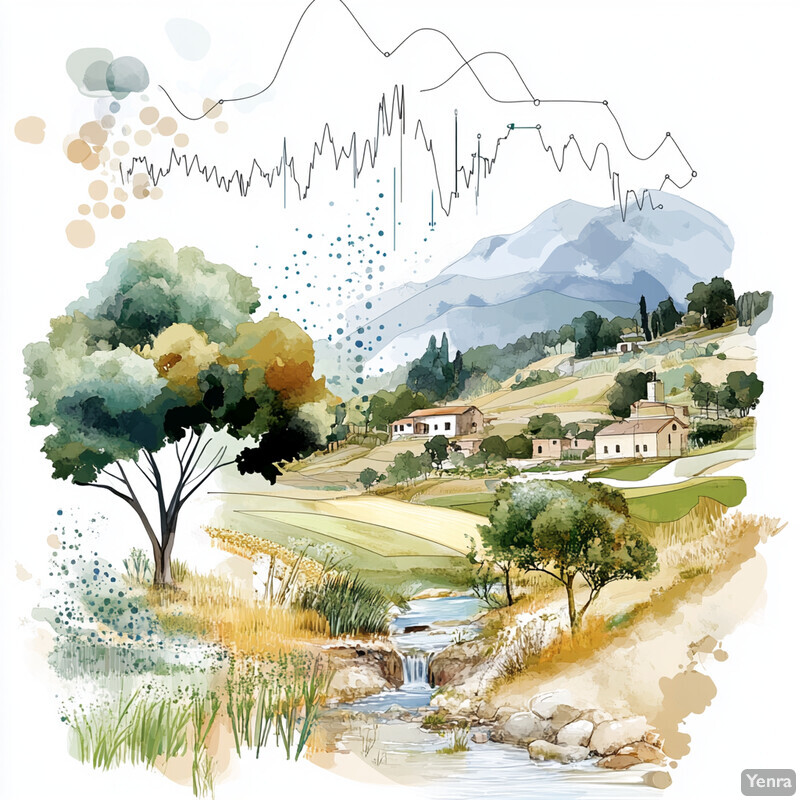
Over multiple growing seasons, farms accumulate extensive datasets on weather patterns, water usage, yields, and soil health. AI excels at analyzing these temporal data streams to identify trends and patterns that might be invisible to human observers. By examining long-term changes—such as gradual shifts in climate, soil fertility, or groundwater availability—AI can inform strategic decisions like choosing drought-tolerant crop varieties or adjusting irrigation infrastructure. Long-term insights guide more sustainable farming practices, ensuring that irrigation strategies remain effective and resource-efficient as environmental conditions evolve.
9. Integration of Soil Physics and Hydraulic Models
AI can utilize complex soil hydrology models to better understand water movement, ensuring that applied water reaches the root zone efficiently.
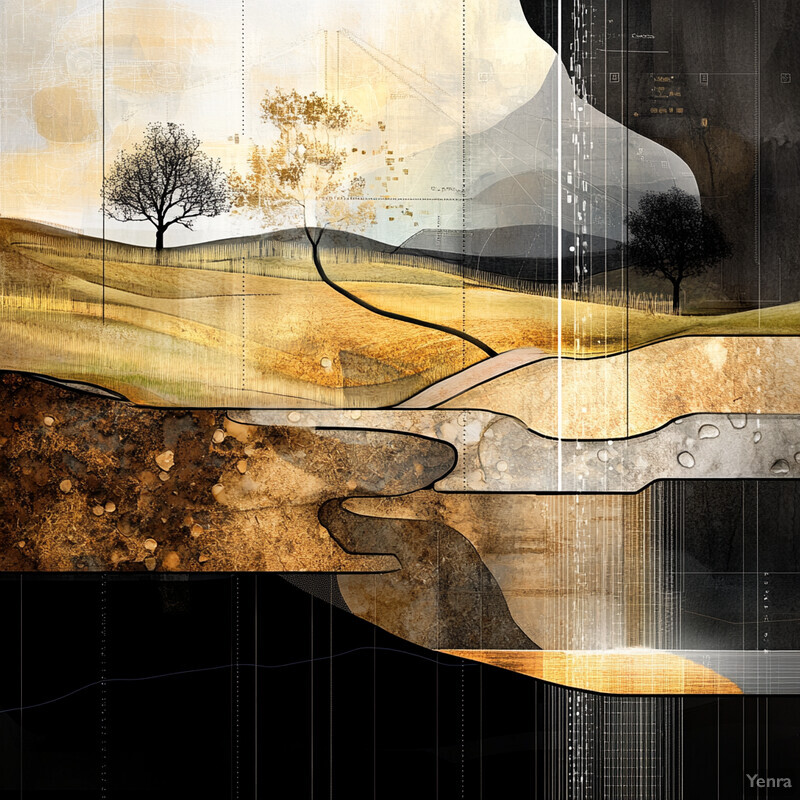
Soil hydrology is complex, influenced by infiltration rates, soil layering, and capillary action. AI systems can incorporate advanced soil physics and hydraulic simulations into their decision-making processes. By modeling how water moves through different soil profiles, the AI can predict the distribution of moisture beneath the surface and ensure irrigation precisely replenishes the root zone without excessive deep percolation or runoff. This combination of theoretical modeling and empirical data leads to a more accurate understanding of soil-water-plant interactions, translating into irrigation strategies that maximize water uptake by the roots.
10. Decision Support Tools With User-Friendly Interfaces
AI-based decision-support systems can present straightforward recommendations, allowing farmers to apply the right amount of water without delving into the underlying complexities.
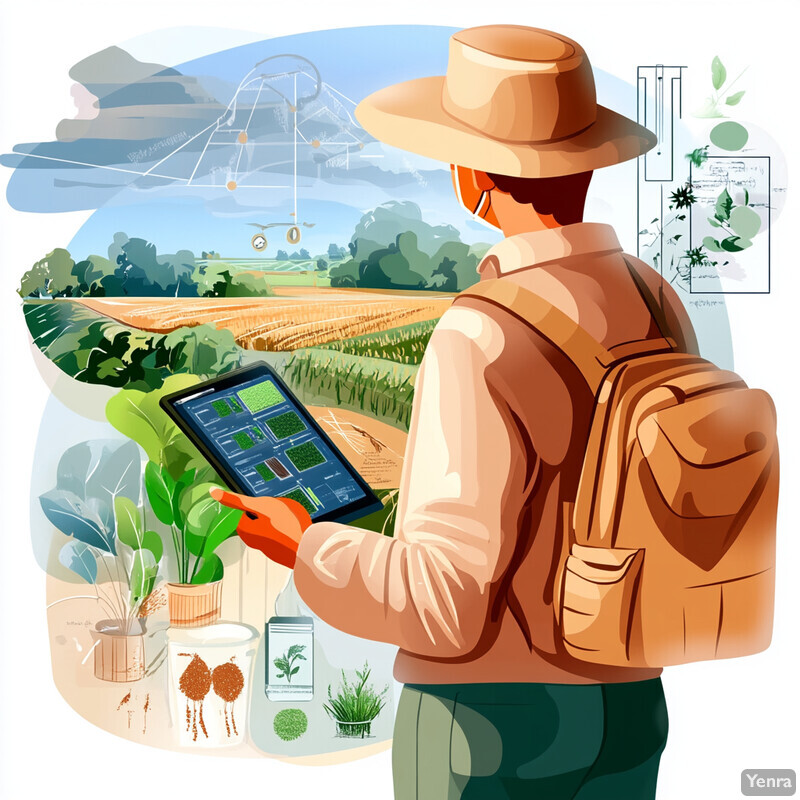
Even the most advanced AI models won’t help farmers if the information they produce is too complex. That’s why modern AI-driven irrigation solutions often feature intuitive dashboards, mobile apps, and simple data visualizations. Behind the scenes, complex machine learning algorithms crunch numbers, but the farmer only sees straightforward recommendations—like “Irrigate Field A with 10 mm of water tomorrow afternoon.” By bridging the gap between complexity and usability, these decision support tools enable farmers to confidently adopt data-driven irrigation practices, leading to more consistent yields and better water management.
11. Predicting Droughts and Water Scarcity Scenarios
AI can help forecast extended dry spells or water shortages, enabling proactive water-saving measures and strategic crop choices.
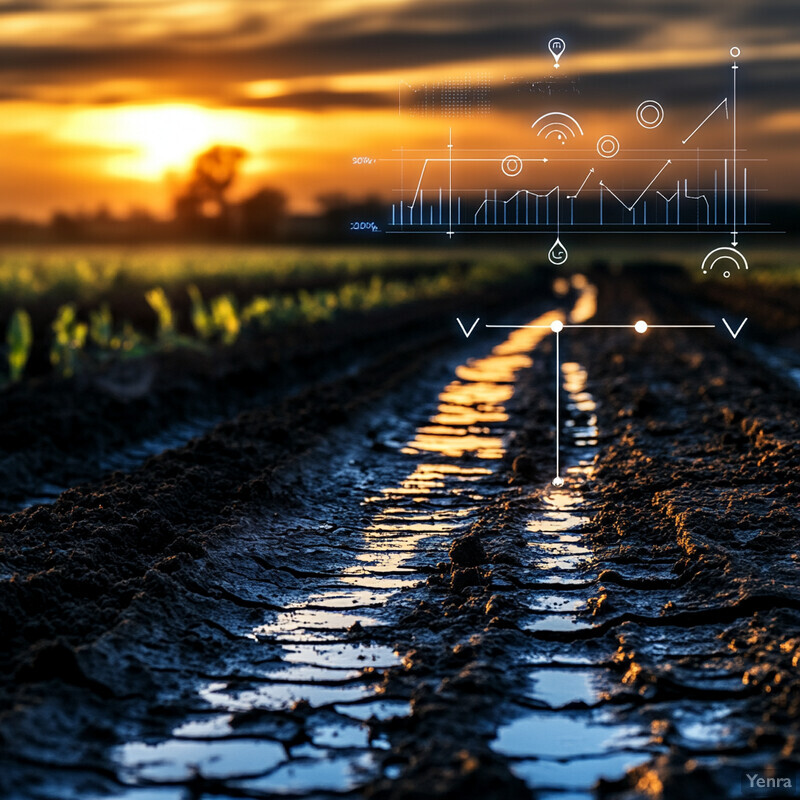
Water availability can be unpredictable, and anticipating extended droughts or water restrictions is crucial for long-term planning. AI can analyze historical climate data, large-scale weather patterns (such as El Niño and La Niña), and seasonal forecasts to predict periods of water scarcity. With advanced warning, farmers can switch to crops better suited to drought conditions, implement water-saving measures early, or adjust their irrigation schedules to prioritize critical growth stages. This predictive insight reduces vulnerability to climate-related risks and supports resilient, sustainable farming operations.
12. Resource Allocation and Cost Savings
AI can minimize pumping costs, reduce energy consumption, and manage water use efficiently, improving farm profitability.
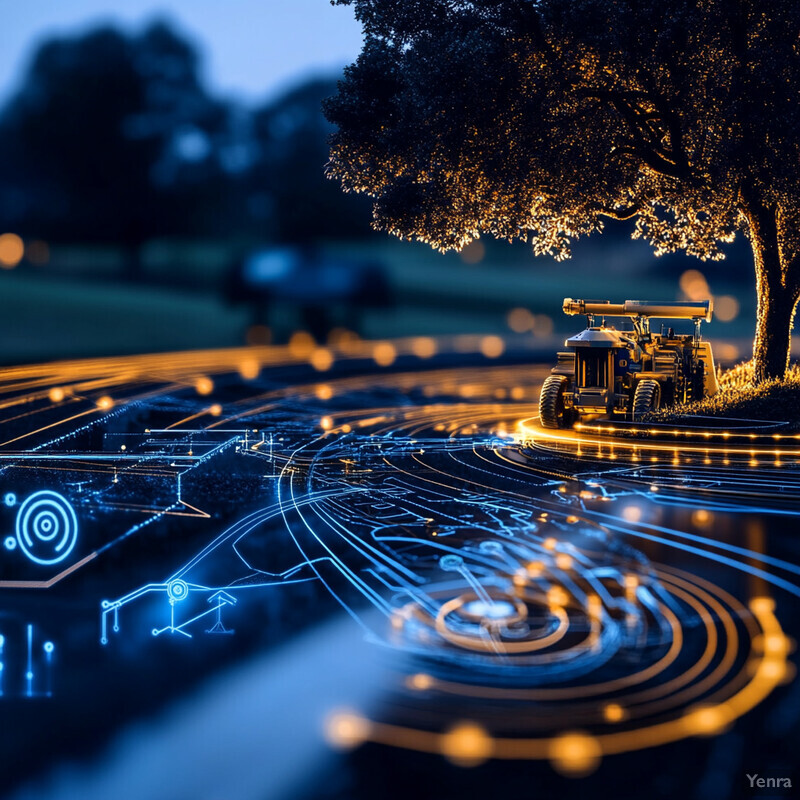
Water is not just an environmental resource; it’s also an economic input that impacts farm profitability. AI can optimize irrigation scheduling to minimize operational costs, factoring in energy prices, pump efficiencies, and water fees. For example, the system might choose to irrigate at night when electricity rates are lower or coordinate irrigation across multiple fields to reduce labor costs. Over time, these incremental savings add up, improving the farm’s bottom line and making it easier for small and medium-sized operations to remain competitive in an increasingly resource-constrained world.
13. Dynamic Threshold Adjustments for Trigger Points
Instead of fixed soil moisture thresholds or timers, AI systems continuously adapt start and stop points for irrigation based on real-time conditions.
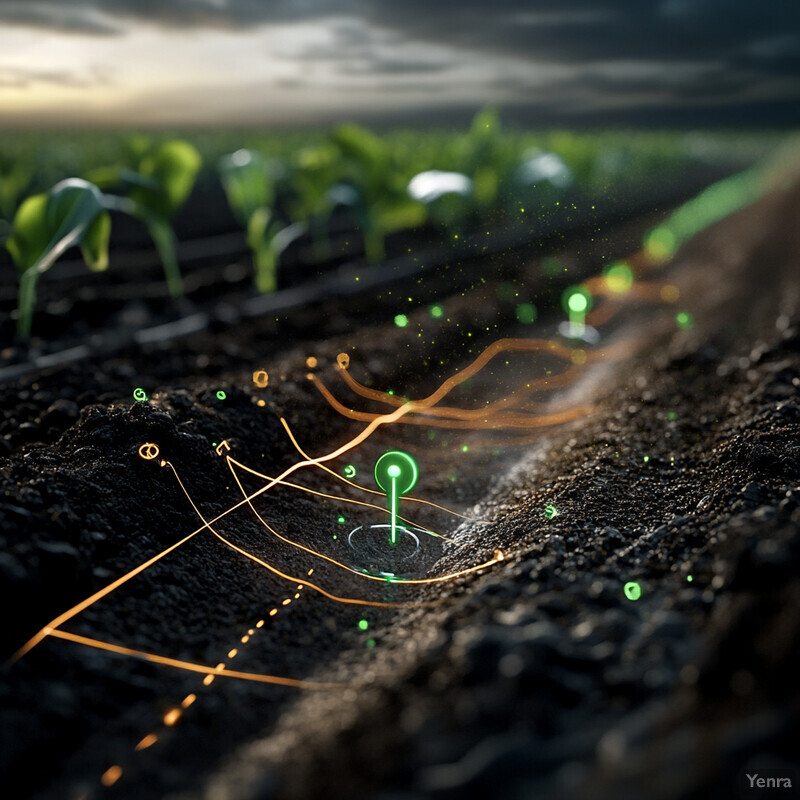
Traditional irrigation scheduling might rely on a fixed soil moisture threshold or timer-based approaches. AI breaks this rigidity by continuously adjusting the thresholds that trigger irrigation events. As weather patterns, crop growth stages, and soil conditions change, the AI system recalibrates its “start” and “stop” points, ensuring that plants receive water exactly when needed. This dynamic approach reduces instances of over-irrigation—leading to wasted water and nutrient leaching—or under-irrigation, where plant health and yield could suffer. It’s a fine-tuned balancing act that ensures water is always allocated efficiently.
14. Integration With Nutrient Management Systems
By linking irrigation schedules with fertigation plans, AI ensures that water and nutrients are delivered together for optimal plant growth and minimal runoff.
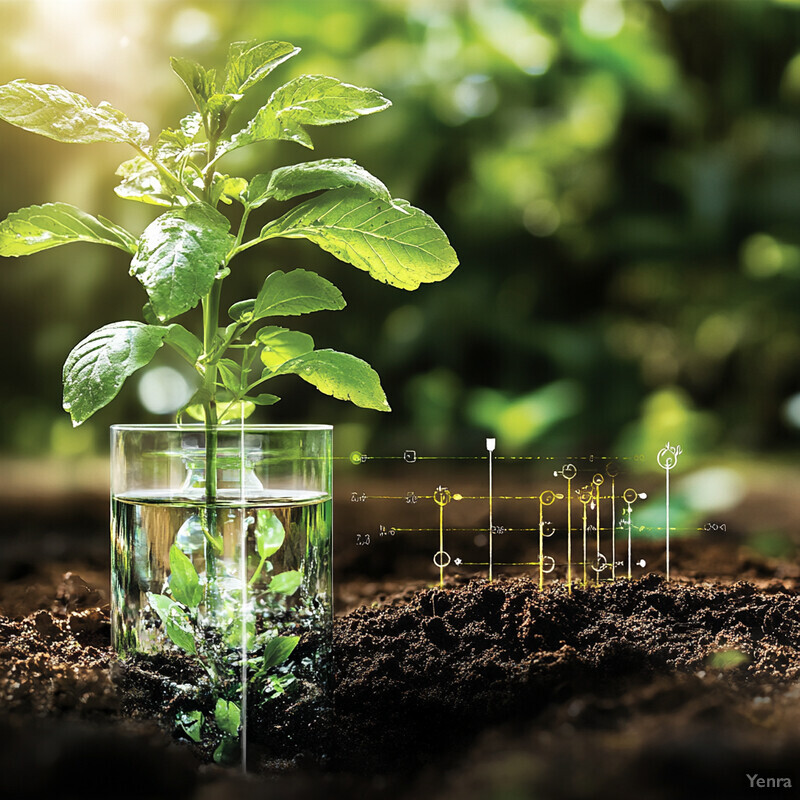
The timing and amount of water applied have a direct impact on nutrient uptake and leaching. By connecting irrigation scheduling with fertigation plans—where fertilizers are applied through irrigation lines—AI ensures that water and nutrients arrive in the root zone simultaneously. This synergy improves nutrient use efficiency, reduces fertilizer runoff, and can enhance overall plant health. Over the long term, integrated water and nutrient management guided by AI results in stronger plants, fewer environmental impacts, and cost savings due to more effective use of inputs.
15. Stress Detection via Plant Physiological Models
AI can interpret subtle plant stress signals from imaging and sensor data, adjusting irrigation schedules before visible damage occurs.
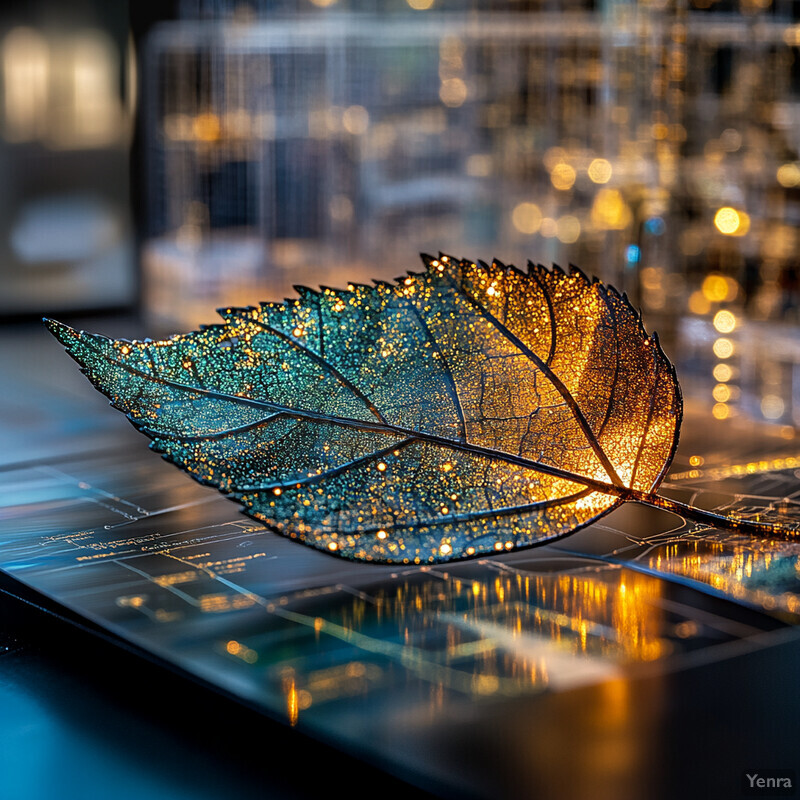
Healthy plants have certain physiological signatures, including how their leaves reflect light, how their canopy temperature fluctuates, and how their chlorophyll content changes over time. AI-based systems can integrate imaging and sensor data to detect subtle signs of plant stress—such as mild water deficiency—long before these symptoms are visible to the naked eye. By responding proactively, irrigation schedules can be adjusted to prevent damage, maintain steady growth conditions, and protect yields. Early intervention saves resources, reduces crop losses, and leads to more resilient production systems.
16. Uncertainty Quantification and Risk Management
AI models can factor in probabilistic weather forecasts and sensor noise, making irrigation decisions more robust against uncertainties.
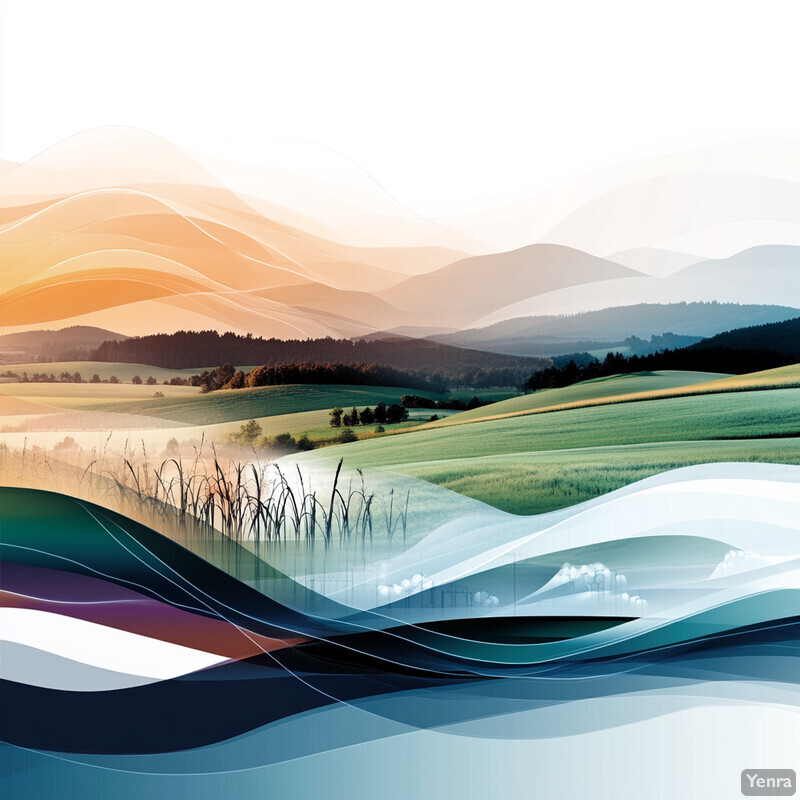
Forecasts, whether for weather, soil moisture, or plant growth, inherently contain uncertainties. AI-driven irrigation models can incorporate probabilistic reasoning and uncertainty quantification, enabling them to provide risk-informed recommendations. If the probability of rain is low but not zero, the system can weigh that information against the risks of underwatering. This ability to handle uncertainty makes irrigation decisions more robust and resilient, ensuring that even if some data or forecasts are off-target, the resulting irrigation plan still remains close to optimal.
17. Self-Learning From Historical Performance Data
By analyzing past irrigation events, yields, and resource use, AI refines its approach over time, continually improving water-use efficiency.
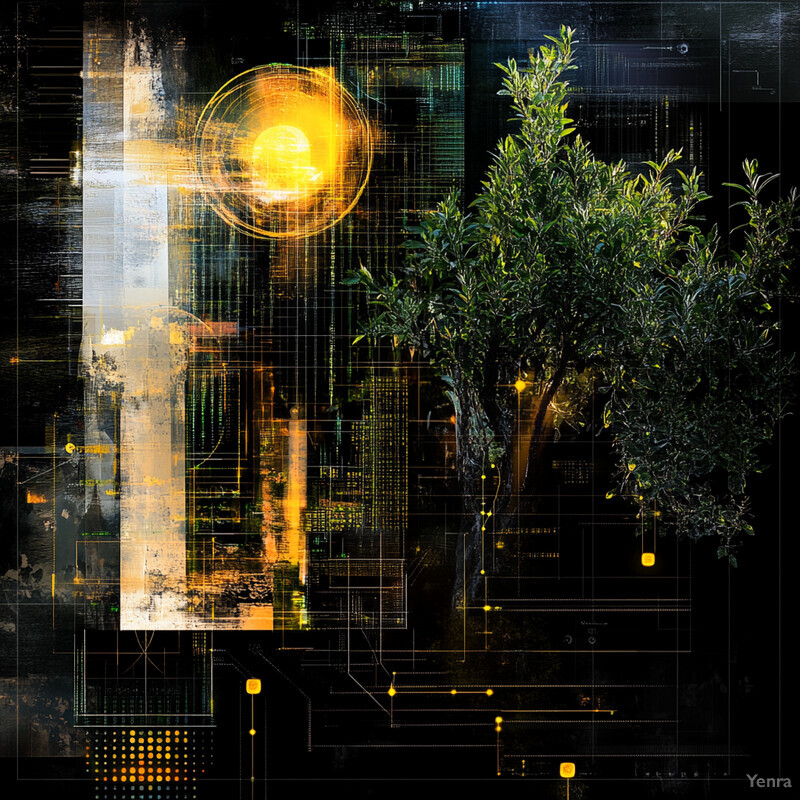
AI thrives on data, and every irrigation event provides valuable feedback. By looking at past irrigation decisions, yield outcomes, and resource usage, the system can learn what strategies worked best and under what conditions. Over multiple growing seasons, the AI refines its models, becoming better at predicting when to irrigate and how much water to apply. This continuous improvement process helps ensure that the system stays relevant and efficient as climate conditions, soil properties, and cropping systems evolve over time.
18. Integrating Grower Preferences and Constraints
AI respects farmer-defined water quotas, regulatory limits, and crop quality goals, tailoring its irrigation recommendations accordingly.
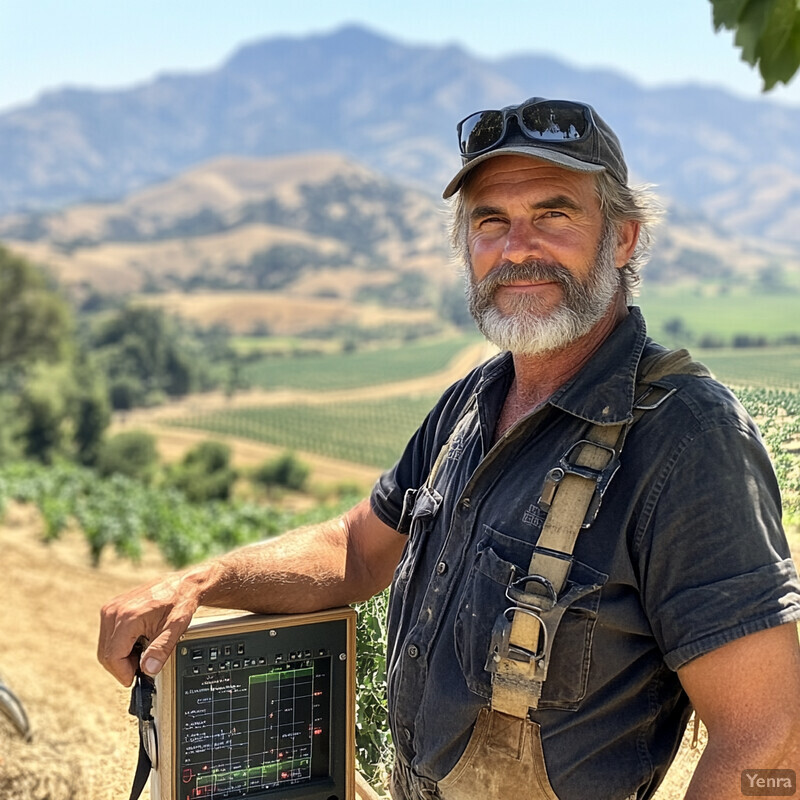
Farms vary widely in terms of available water resources, energy costs, infrastructure, and farmer preferences. AI-based irrigation solutions can incorporate human-defined constraints such as a maximum allowable water withdrawal, desired crop quality levels, or compliance with local water regulations. By respecting these parameters, the system provides recommendations that are not only technically optimal but also feasible and acceptable to the farm’s unique situation. This human-in-the-loop approach ensures that technology enhances, rather than overrides, the expertise and priorities of the farmer.
19. Scaling From Small Farms to Large Operations
AI-based irrigation scheduling can be applied to operations of any size, adapting from a single field to vast agricultural enterprises.
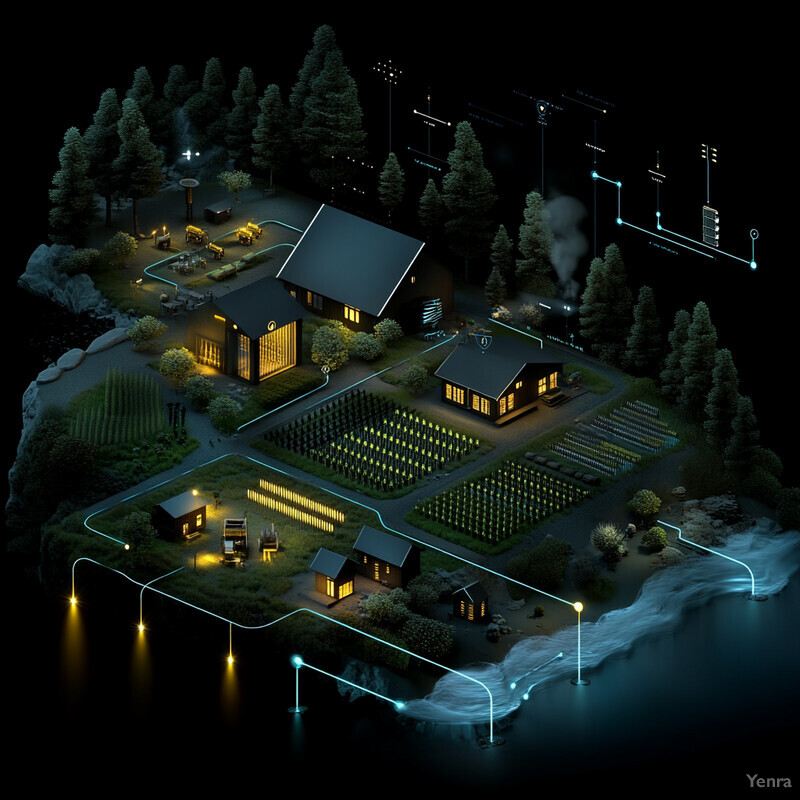
The principles behind AI-based irrigation scheduling apply at any scale. Smallholders can benefit from simple smartphone-based apps that provide timely advice, while large commercial operations with extensive sensor networks can deploy highly complex, automated systems. As farms grow or shrink, AI models can scale accordingly, handling more sensors, more irrigation zones, and more sophisticated optimization targets without losing accuracy or efficiency. This scalability makes AI-driven solutions attractive to the entire agricultural sector, from family farms to large agribusinesses.
20. Continuous Improvement Through Open Data and Collaboration
As AI models access more regional trials, global climate data, and research inputs, irrigation recommendations become increasingly accurate and universal.
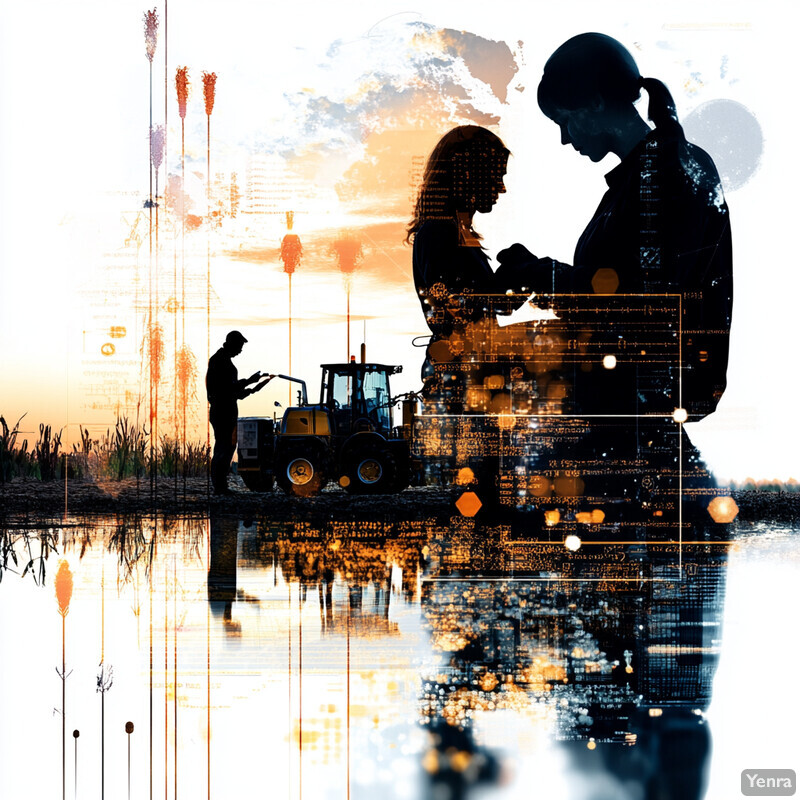
AI-based irrigation systems benefit from the collective wisdom of the global farming community, research institutions, and environmental agencies. As more data—on weather patterns, soil types, new crop varieties, and water-saving techniques—is shared and standardized, AI models become more robust and widely applicable. Collaborative platforms and open data initiatives allow for rapid improvements in the algorithms, ultimately leading to more universally accurate recommendations. This ecosystem of shared knowledge drives innovation, ensuring that farmers everywhere gain access to cutting-edge irrigation scheduling tools that enhance sustainability, productivity, and profitability.