1. Leak Detection and Localization
AI-driven anomaly detection models can identify unusual drops in pressure or flow patterns, quickly pinpointing leaks so that maintenance crews can address issues before they cause major water loss.
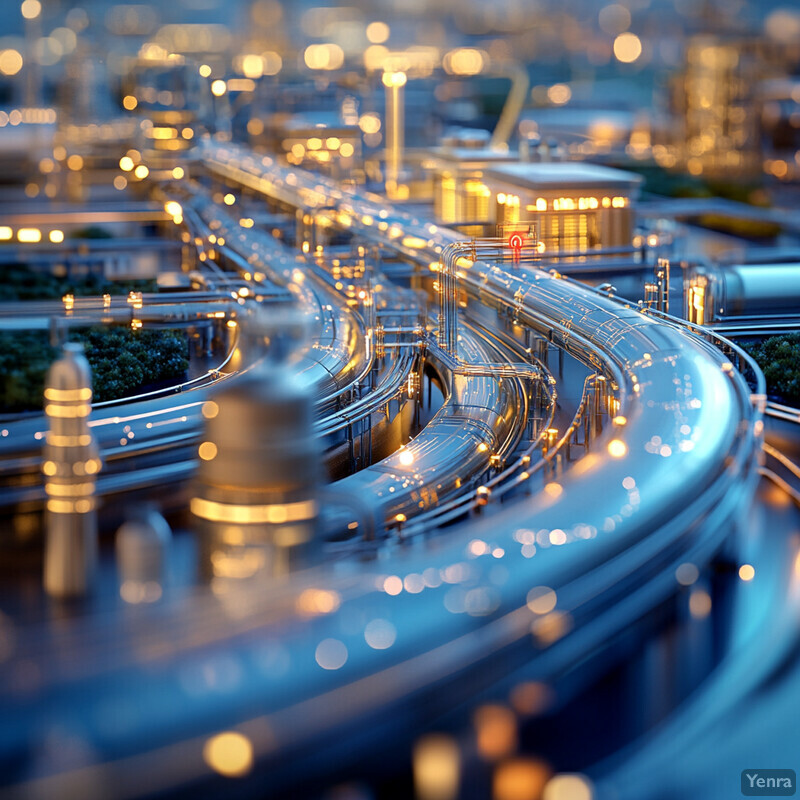
AI-driven leakage detection relies on advanced pattern recognition and anomaly detection algorithms that continuously assess sensor data, such as flow rates, pressure readings, and acoustics within pipes. By comparing current sensor outputs against historical baselines and modeling expected behaviors, these systems can quickly identify subtle deviations that indicate hidden leaks. Once a leak is suspected, AI-enhanced localization techniques use distributed sensor inputs and hydraulic modeling to pinpoint the precise section of pipe that requires inspection. Early and accurate detection enables utilities to address leaks promptly, reducing both water loss and the potential for infrastructure damage while lowering operational costs.
2. Demand Forecasting
Machine learning algorithms can accurately predict future water consumption patterns based on historical usage, weather data, and demographic trends, enabling utilities to optimize supply and reduce wastage.
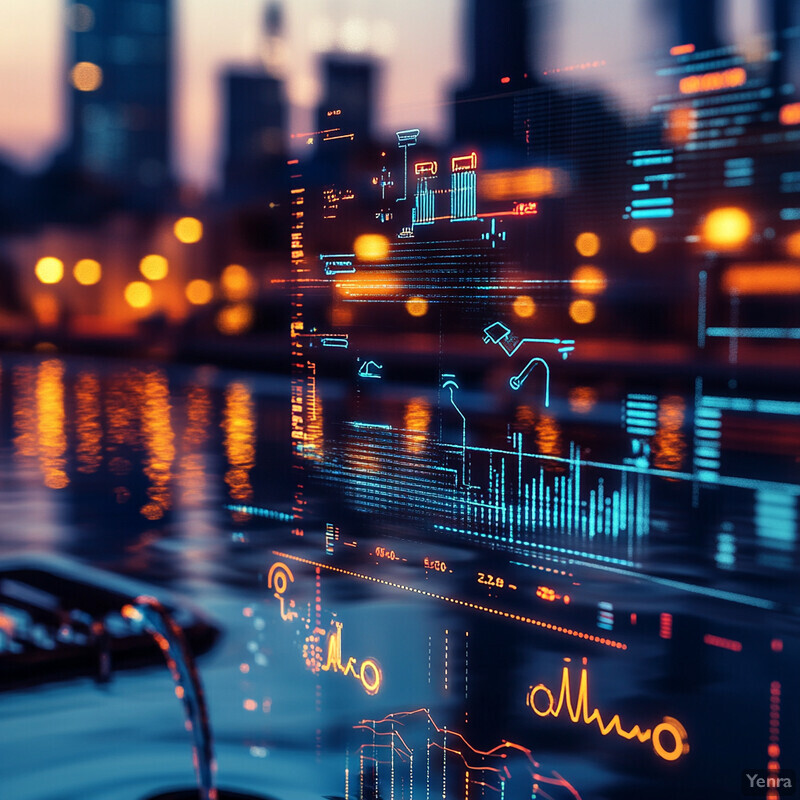
Machine learning models leverage historical consumption data, demographic patterns, local weather forecasts, and even special events (like holidays or public festivals) to predict future water demand. By training on large, diverse datasets, these models can accurately anticipate short-term fluctuations and long-term trends. As conditions change—such as seasonal variations, population growth, or industrial shifts—AI continually updates its forecasts. Reliable demand forecasting guides water utilities in optimizing supply, storage, and pressure management, ensuring that sufficient volume is always available without excessive pumping, thereby cutting energy costs and minimizing water waste.
3. Pressure Optimization
AI-based optimization models can determine the ideal pump speeds and valve settings to maintain consistent pressure across the network, reducing stress on infrastructure and energy costs.
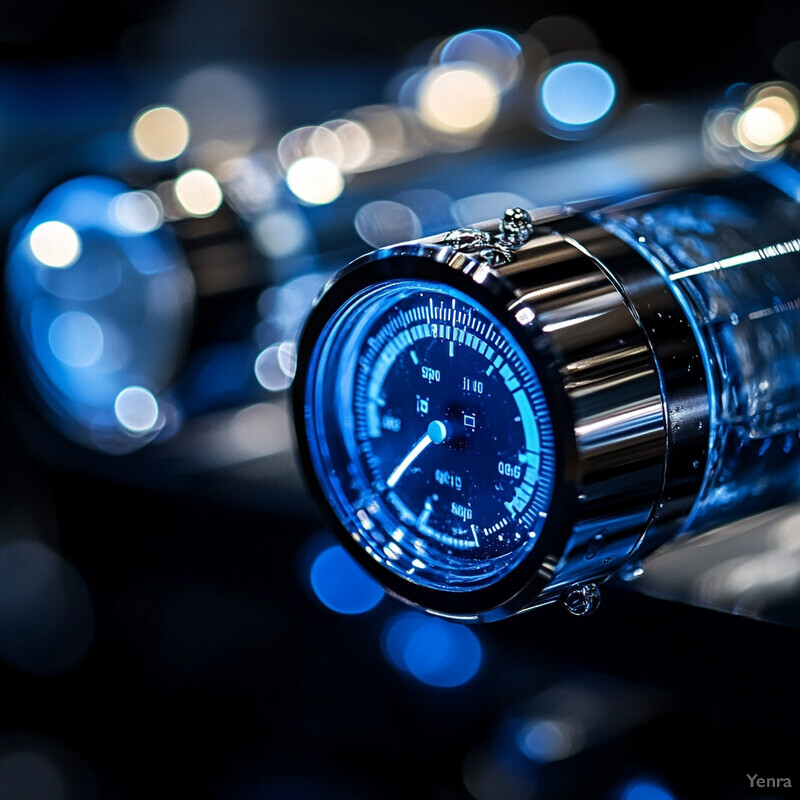
Intelligent pressure management employs AI-based optimization to maintain stable, efficient pressure throughout the distribution network. Algorithms analyze real-time sensor inputs, system topology, and consumer usage patterns to adjust pump speeds, valve openings, and pressure set points. By ensuring that pressure levels remain within optimal ranges, these solutions help prevent pipeline fatigue, reduce the likelihood of bursts, and lower energy consumption. Over time, the system learns to anticipate pressure drops or spikes, continually refining its control strategy to improve overall network reliability and extend infrastructure life.
4. Real-Time Network Control
Intelligent control systems use AI to respond dynamically to changing conditions (e.g., sudden spikes in demand, pipe bursts, or pump failures), adjusting operations autonomously for optimal network performance.
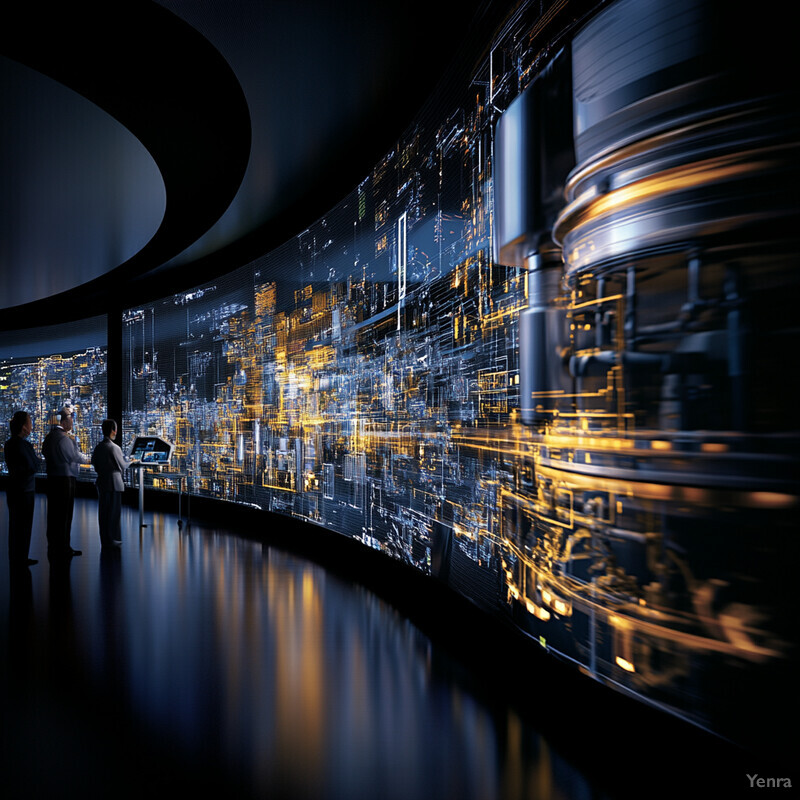
Advanced control algorithms, powered by AI, dynamically regulate system operations in response to rapid changes such as sudden demand surges, pipe bursts, or pump failures. By continuously monitoring key performance indicators, the AI can autonomously adjust pump schedules, valve positions, and reservoir levels to restore equilibrium. In doing so, it ensures uninterrupted service to consumers and safeguards water quality. Operators benefit from having a self-regulating system that can respond to emergencies quicker than human intervention alone, ultimately increasing the resilience and robustness of water supply networks.
5. Predictive Maintenance of Assets
Advanced analytics and machine learning models can track equipment condition data, such as vibration, pressure, and temperature readings, to anticipate equipment failures and schedule maintenance before breakdowns occur.
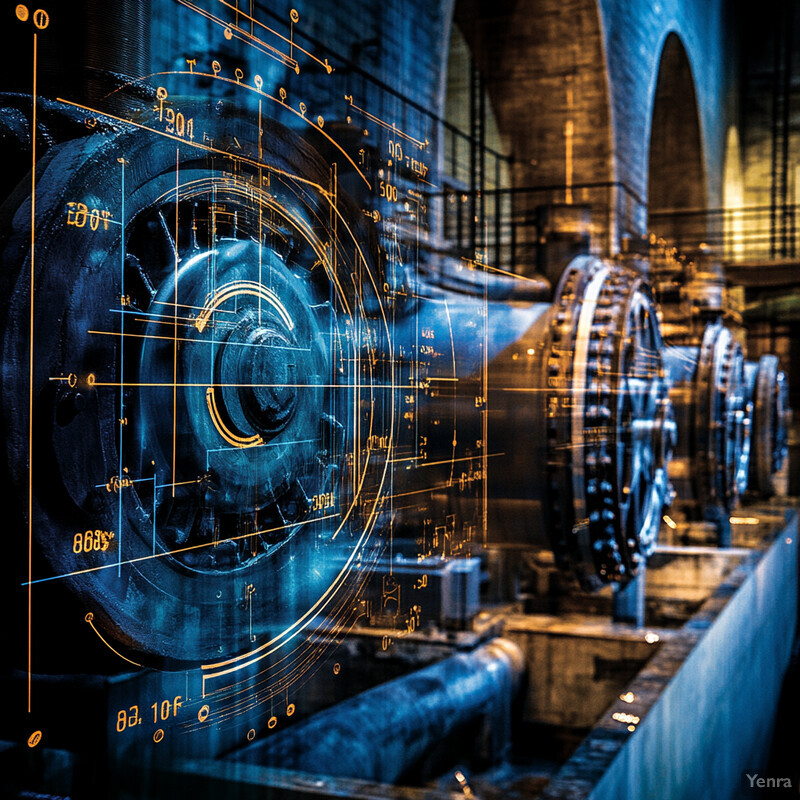
Predictive maintenance strategies use AI to interpret signals from pumps, valves, meters, and other critical assets, detecting subtle signs of wear or impending failure. Machine learning models examine parameters such as vibration frequencies, acoustic signatures, temperature fluctuations, and energy consumption to identify abnormal patterns. By forecasting when equipment is likely to fail, utilities can plan proactive maintenance schedules, procure spare parts in advance, and avoid costly emergency repairs. Over time, predictive maintenance reduces service interruptions, prolongs asset lifespan, and lowers the total cost of ownership.
6. Water Quality Monitoring
AI can process and analyze sensor data related to turbidity, pH, chlorine residuals, and other quality indicators to alert operators to potential contamination events and prompt timely interventions.
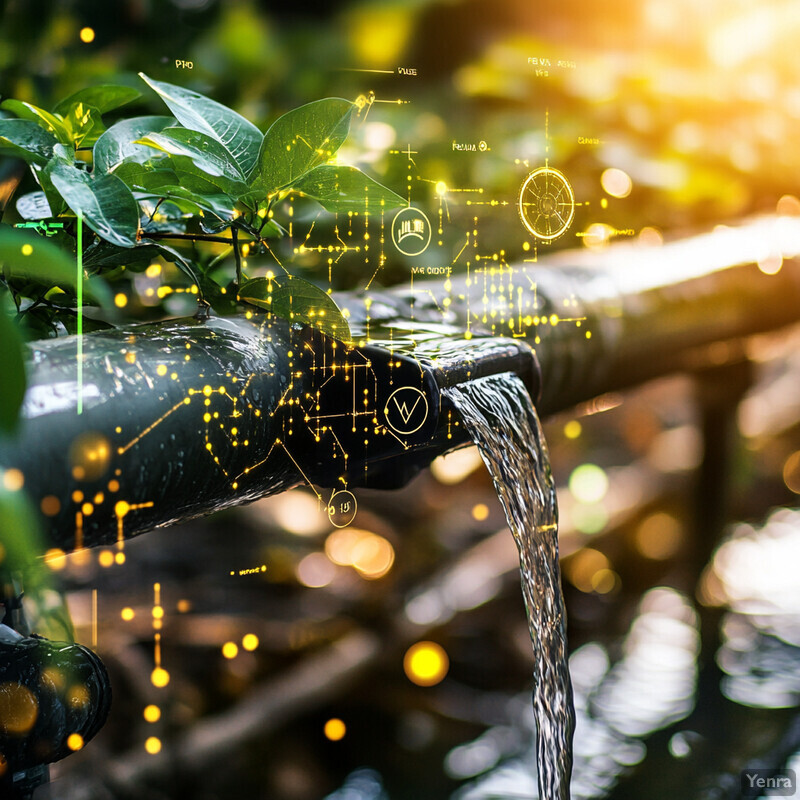
AI-enhanced systems continuously evaluate sensor readings for key water quality indicators like turbidity, pH, chlorine residuals, and bacterial counts. Sophisticated algorithms integrate these inputs with operational data and environmental conditions to detect emerging quality issues. By identifying deviations from established benchmarks, AI can raise early alerts and prompt intervention, whether by adjusting chemical dosing in treatment plants or isolating sections of the network. This targeted, timely response ensures safer, cleaner water for consumers and helps utilities maintain compliance with health and safety regulations.
7. Fault Classification and Diagnosis
By training on historical data, AI systems can classify the nature of faults (e.g., mechanical failures, sensor malfunctions, or hydraulic anomalies) and guide operators to the quickest and most cost-effective remedies.
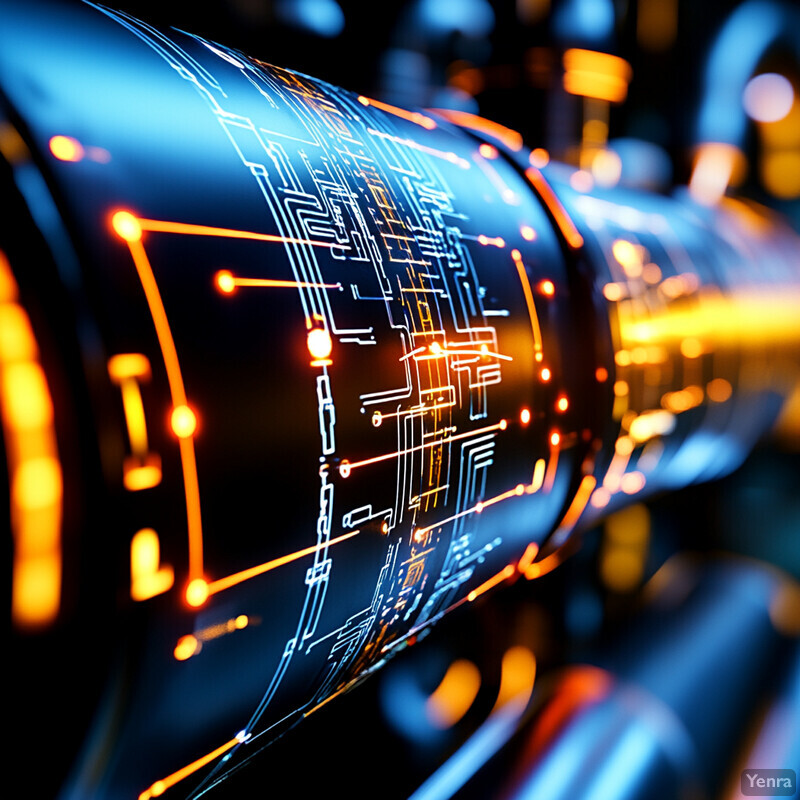
When anomalies arise—such as unexpected pressure drops or strange flow variations—AI-driven classification systems use historical data and predefined categories of common malfunctions to rapidly diagnose problems. Through pattern recognition and statistical inference, these tools can differentiate between mechanical breakdowns, instrumentation errors, and hydraulic disturbances. Presenting operators with a ranked list of probable causes and recommended actions, AI reduces diagnostic time, streamlines troubleshooting, and ensures that maintenance efforts are promptly directed toward the most effective solution.
8. Pump and Valve Scheduling
Optimization algorithms can compute the most energy-efficient operational schedules for pumps and valves, minimizing energy consumption and lowering operational expenses while meeting demand constraints.
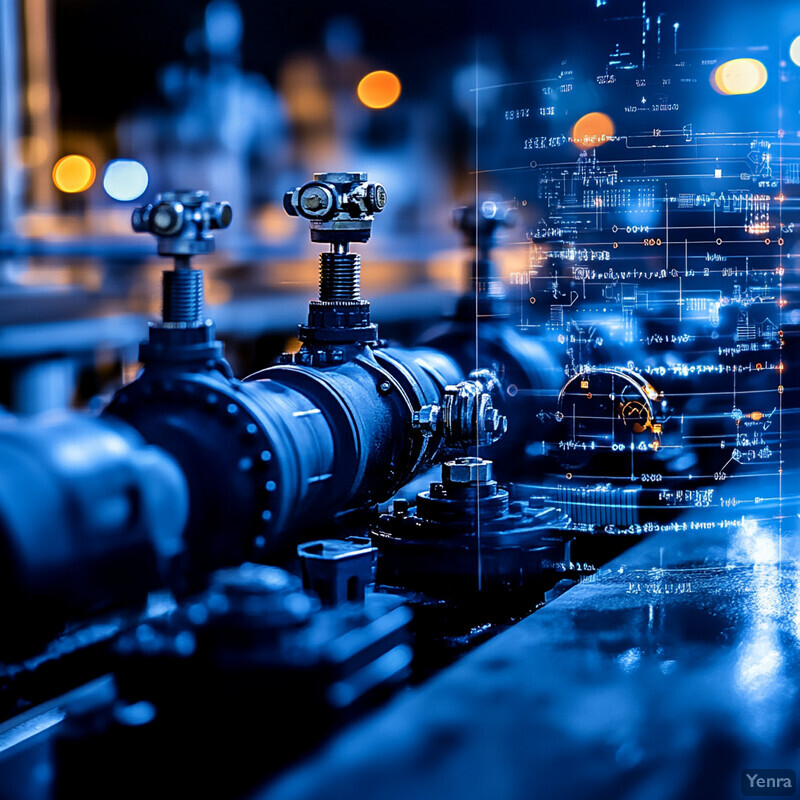
Optimizing pump and valve operations is a balancing act between energy efficiency, service reliability, and infrastructure longevity. AI-based optimization algorithms combine hydraulic modeling with real-time demand data, electricity tariff information, and asset conditions to create cost-effective schedules. By automatically adjusting pump runtimes and valve configurations, these intelligent schedulers minimize energy consumption, reduce peak load charges, and maintain adequate pressures and flows. In the long run, such proactive management significantly cuts operational costs and environmental impact.
9. Sensor Network Optimization
AI tools can identify the best locations and configurations for new sensors within the distribution network, ensuring comprehensive data coverage at a minimal cost.
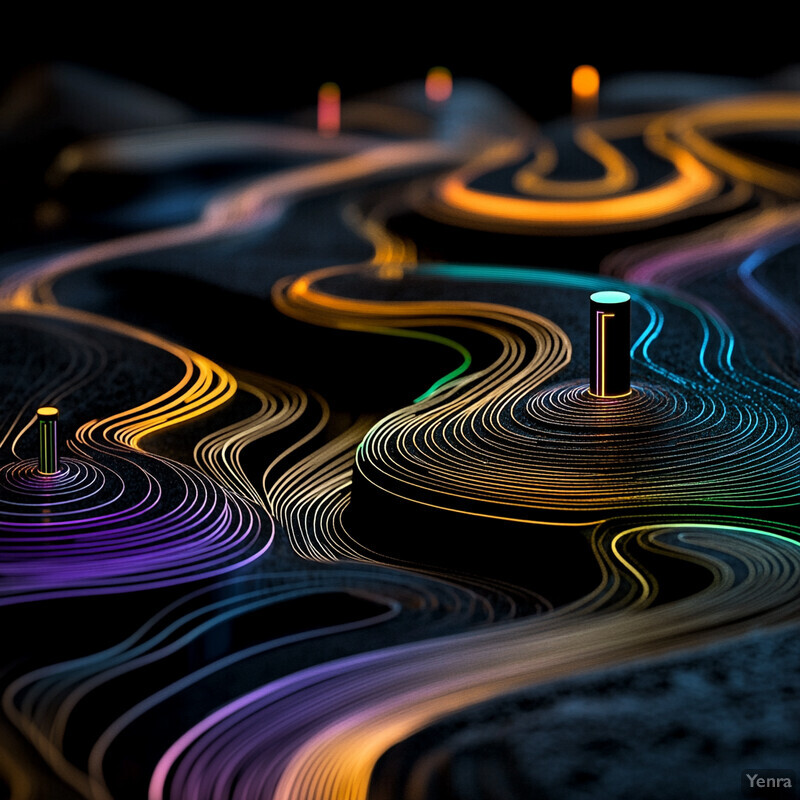
Determining where to place sensors is crucial for achieving comprehensive network visibility without incurring excessive costs. AI-driven sensor placement optimization uses algorithms that simulate various sensor configurations to determine which arrangement yields the best coverage and diagnostic potential. Factors considered include the network’s topology, vulnerability points, and hydraulic behavior. This approach ensures robust monitoring capabilities, allowing utilities to capture critical data that informs everything from leak detection to quality assurance and operational strategy.
10. Water Loss Reduction
By detecting patterns indicative of leaks, theft, or meter inaccuracy, machine learning models can help utilities reduce non-revenue water and improve overall system efficiency.
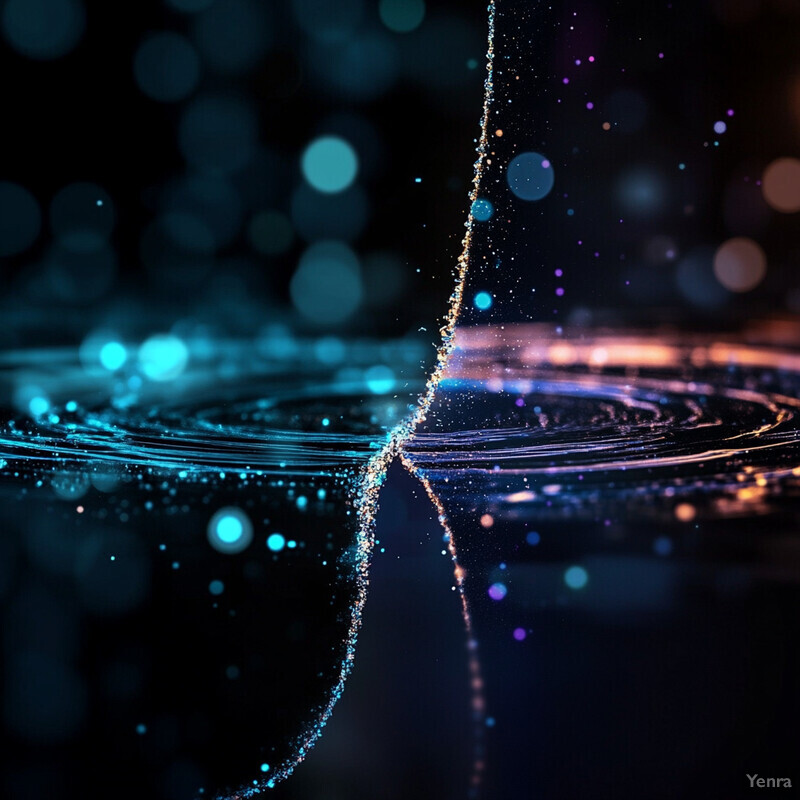
Non-revenue water (NRW)—the portion lost through leaks, theft, or inaccuracies—is a costly challenge for utilities. AI-based analytics identify consumption outliers, suspicious meter readings, and other patterns suggestive of unauthorized connections or malfunctioning equipment. Coupled with proactive leak detection and well-informed asset management, these insights help utilities reduce NRW by pinpointing its sources and prioritizing countermeasures. Ultimately, AI-driven strategies minimize water waste, protect revenue streams, and ensure a more equitable and efficient use of this vital resource.
11. Climate-Responsive Planning
AI can integrate climate forecasts (rainfall, temperature fluctuations, drought likelihood) into water distribution strategies, ensuring the network is resilient to long-term environmental changes.
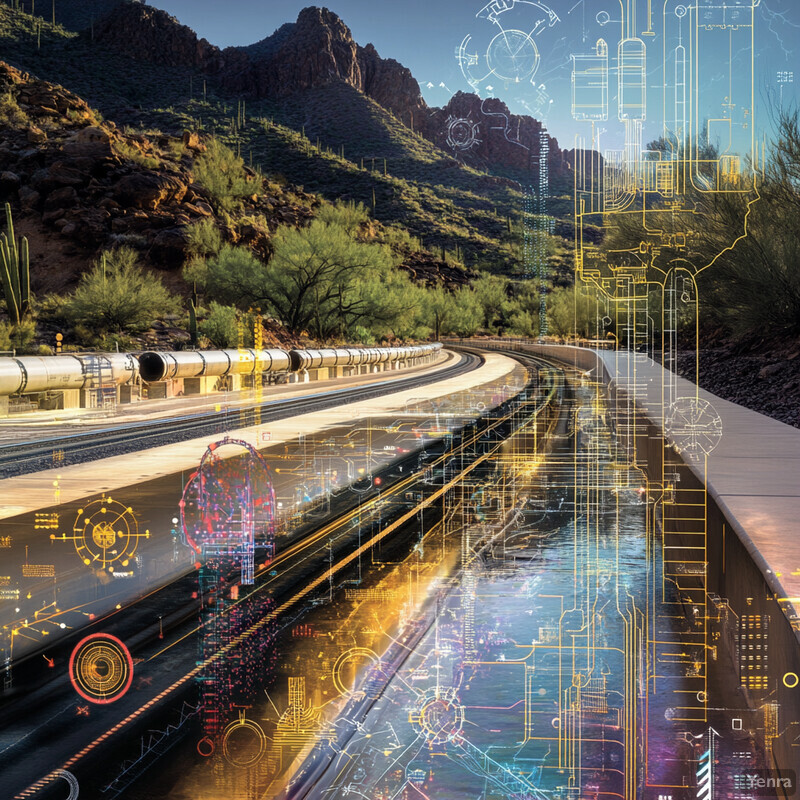
As climate change alters weather patterns and water availability, AI tools integrate historical climate data, current conditions, and long-term projections into planning models. They consider factors like anticipated droughts, rainfall variability, temperature shifts, and population dynamics. In doing so, AI helps utilities design more resilient distribution systems that can handle extremes, whether that means adjusting storage capacities, modifying treatment protocols, or rerouting supplies. By preparing for uncertainty, water networks can maintain reliable service in the face of environmental change.
12. Hydraulic Simulation and Calibration
AI can assist in calibrating hydraulic models, using large sets of operational data to improve model accuracy, making simulations more robust and reliable.
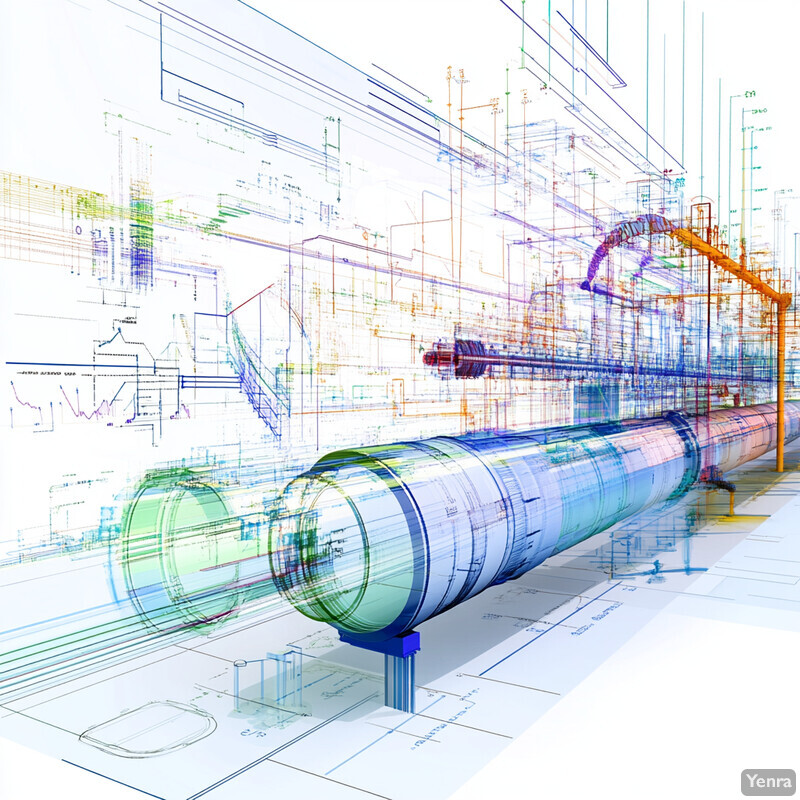
Traditional hydraulic modeling can be cumbersome and imprecise if not well calibrated. AI simplifies the calibration process by systematically comparing model outputs against real sensor measurements, adjusting parameters to achieve a better fit. These improvements result in simulations that closely mirror actual conditions. With high-fidelity models, engineers can make more informed decisions about system expansions, upgrades, and maintenance. Automated calibration processes ensure that these models remain accurate over time, adapting to changes in both the network and consumer demand.
13. Integration with Smart Cities
As part of broader smart city frameworks, AI can help synchronize water distribution with other utility services (electricity, gas, transportation), ensuring balanced resource allocation and optimal urban planning.
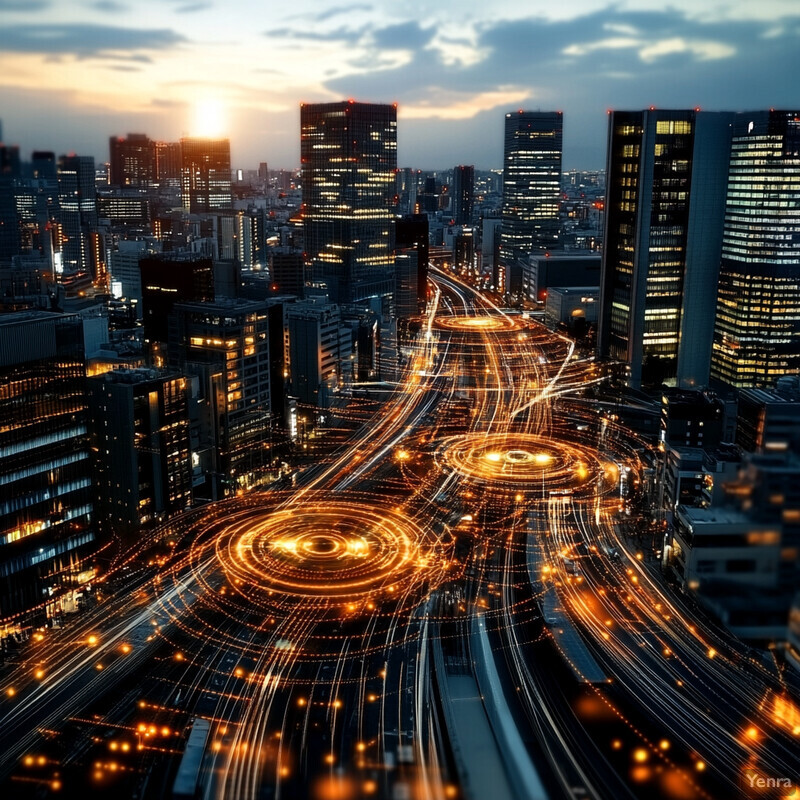
Modern smart city initiatives aim to unify infrastructure systems for greater efficiency, security, and resilience. AI enables water distribution networks to coordinate seamlessly with other urban services—like energy grids, transportation hubs, and telecommunications—by sharing data, responding to joint demands, and managing resources holistically. This integrated approach allows water utilities to fine-tune supply in sync with broader city dynamics, ensuring sustainable development, improved quality of life, and lower environmental footprints.
14. Automated Alarm and Event Management
Intelligent filtering of sensor alarms reduces false positives. AI algorithms can recognize genuine emergencies and rank their severity, helping operators prioritize corrective actions.
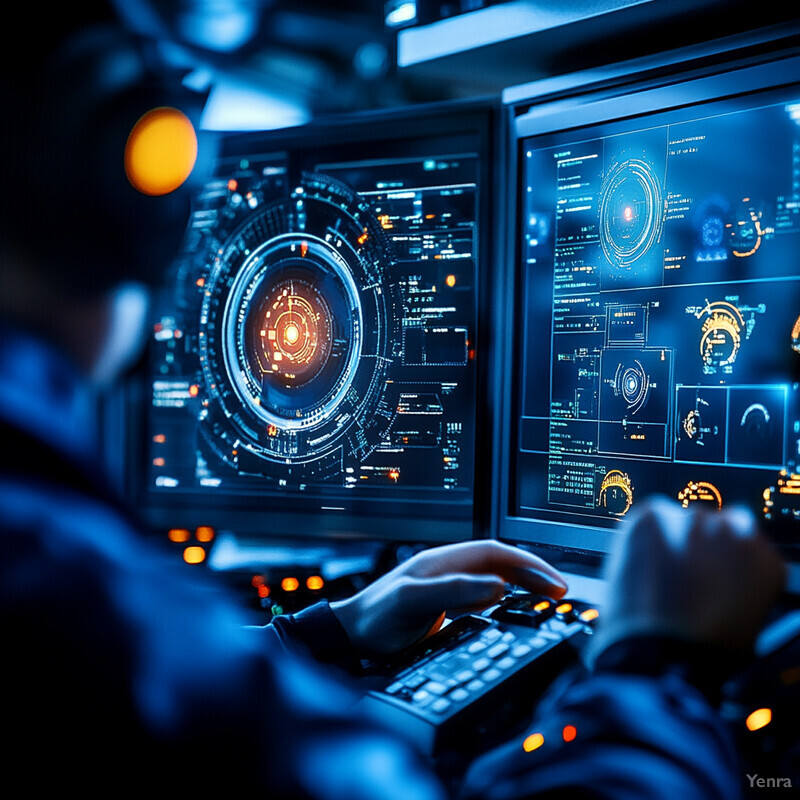
Complex sensor grids often trigger frequent alarms—many of which are false or non-urgent. AI-powered filtering and event categorization distinguish between genuine emergencies and trivial anomalies. By prioritizing the severity and likelihood of alarms, operators receive a manageable, context-rich set of alerts. This reduces alarm fatigue, enabling human supervisors to focus on critical events. As a result, response times improve, resolution actions become more targeted, and overall operational efficiency increases.
15. Anomaly and Event Prediction
Beyond simple faults, AI can foresee unusual flow patterns related to major events—such as industrial accidents or infrastructure damage—enabling proactive mitigation steps.
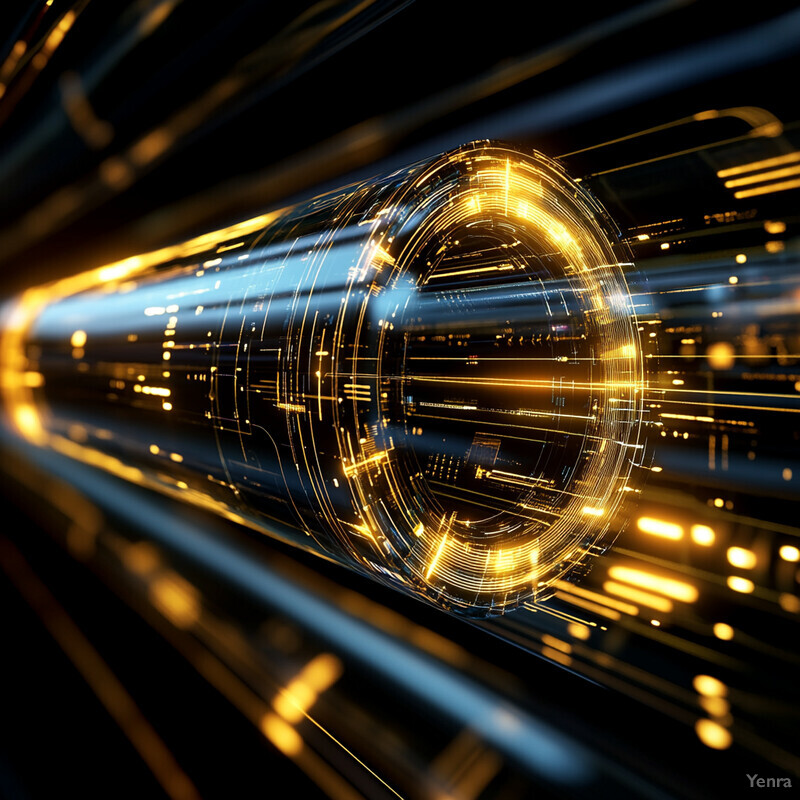
Beyond detecting immediate faults, AI anticipates unusual system states before they fully emerge. By analyzing historical patterns, network geometry, and environmental variables, AI-based tools can predict events like sudden pump failures, pressure surges caused by external interferences, or unexpected shifts in usage triggered by industrial accidents. Early warnings enable utilities to take preventative actions—such as adjusting flows, dispatching maintenance crews, or notifying customers—thereby mitigating or even averting negative outcomes.
16. Customer Behavior Analytics
By analyzing user consumption patterns, AI can help utilities design targeted water-saving initiatives, personalize tariffs, and improve customer service through proactive communication.
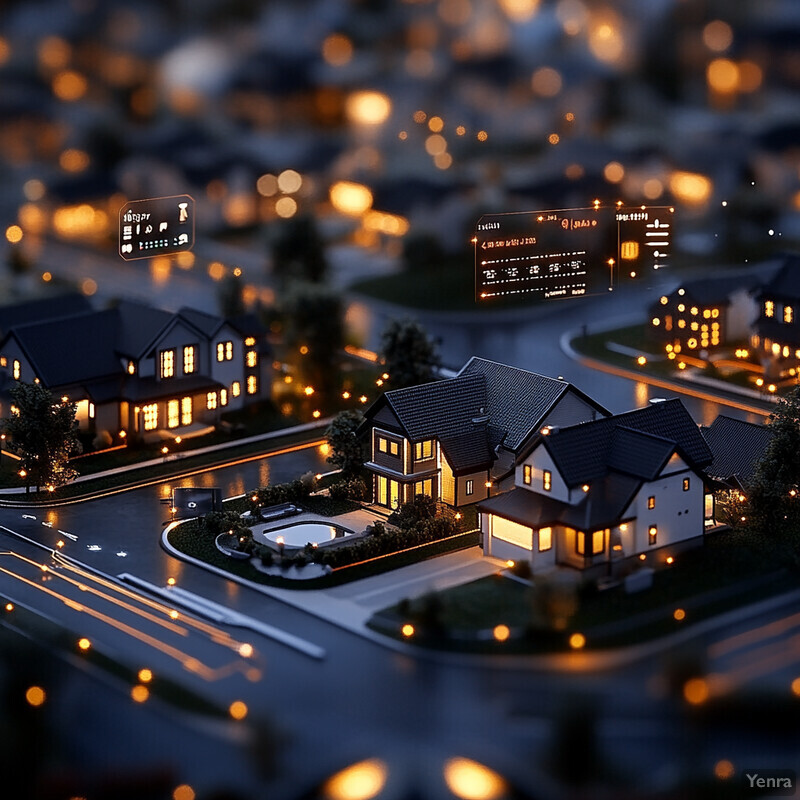
AI-driven analytics delve into user consumption data to identify segments, patterns, and preferences. By understanding how different communities use water—peak usage times, response to pricing strategies, or sensitivity to conservation campaigns—utilities can tailor demand management efforts. Personalized tariffs, targeted communication campaigns, and efficient resource allocation all stem from this deeper insight into customer behavior. The end result is a more customer-centric approach that enhances satisfaction, promotes water-saving habits, and ensures a fair and effective pricing structure.
17. Cybersecurity Threat Detection
With AI-driven analytics, systems can identify suspicious network activity or cyberattacks on SCADA systems, improving the security and reliability of automated water distribution operations.
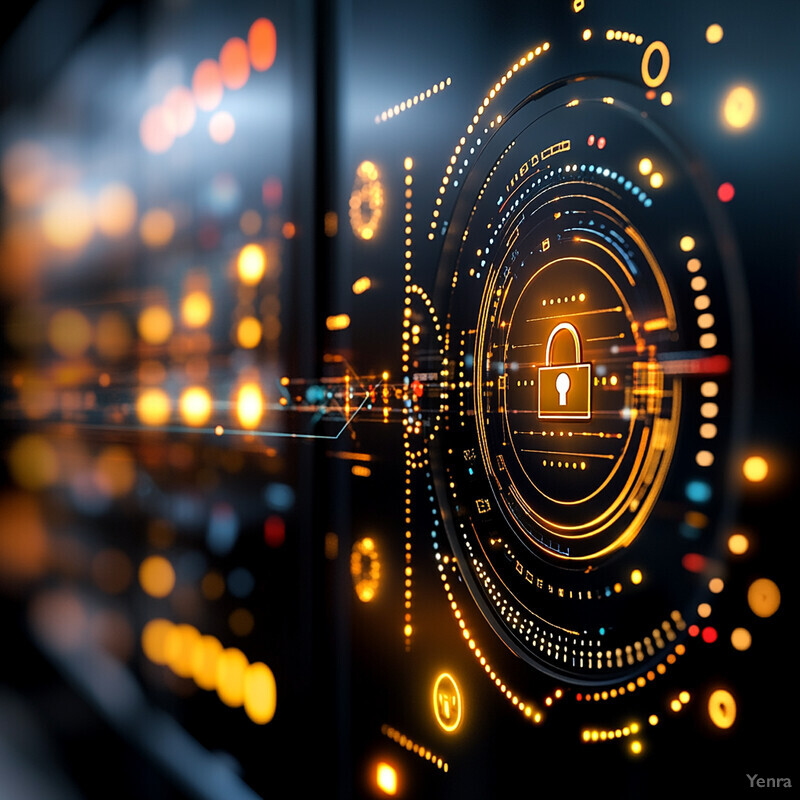
As water networks become more digitized, they face heightened cybersecurity risks. AI-based anomaly detection and intrusion detection systems continuously scan network traffic, SCADA signals, and control commands for suspicious patterns. Rapid identification of unusual activity—like unauthorized access attempts or suspicious data flows—enables quick isolation and remediation of threats. This proactive stance helps safeguard critical infrastructure, ensuring that water distribution remains stable, secure, and trustworthy even as cyber threats become more sophisticated.
18. Digital Twins of Water Networks
AI-enabled digital twins can mirror real-time conditions in a virtual environment, allowing operators to run simulations, test scenarios, and optimize strategies without affecting the physical infrastructure.
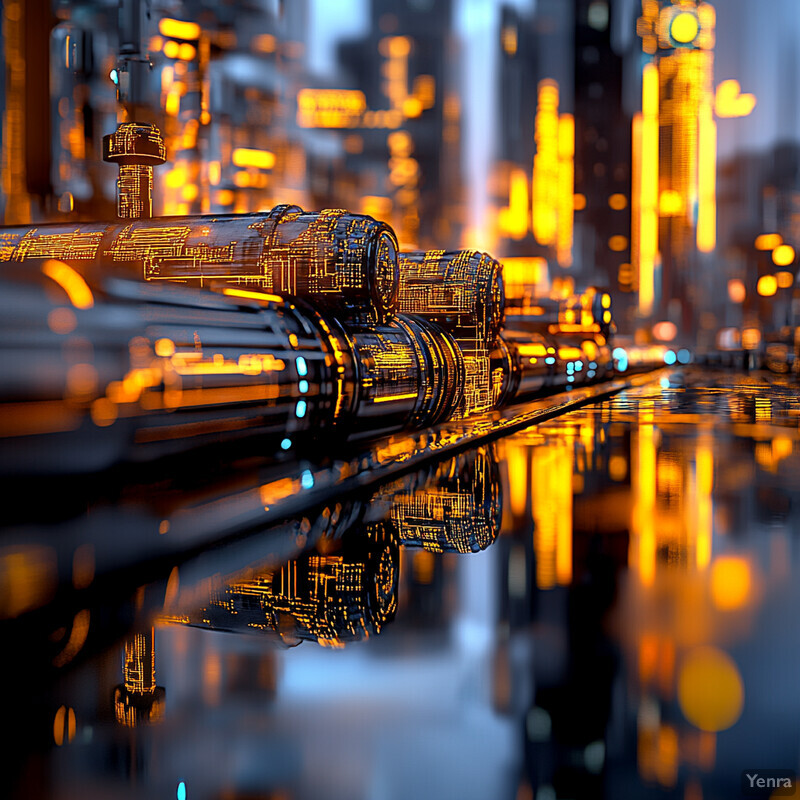
A digital twin is a virtual, AI-enhanced replica of the physical water network. It continuously integrates real-time sensor data, operational conditions, and environmental variables to mirror the state of the system. Engineers and operators can then simulate various scenarios—like equipment failures, demand spikes, or quality incidents—without risking real-world disruptions. By experimenting within this safe digital environment, utilities discover optimal strategies, refine operational rules, and verify the effects of upgrades before implementing them in the physical network.
19. Optimized Capital Planning
Data-driven insights help utilities allocate funds for infrastructure upgrades and replacements more strategically, prioritizing high-risk zones and ensuring the longevity of assets.
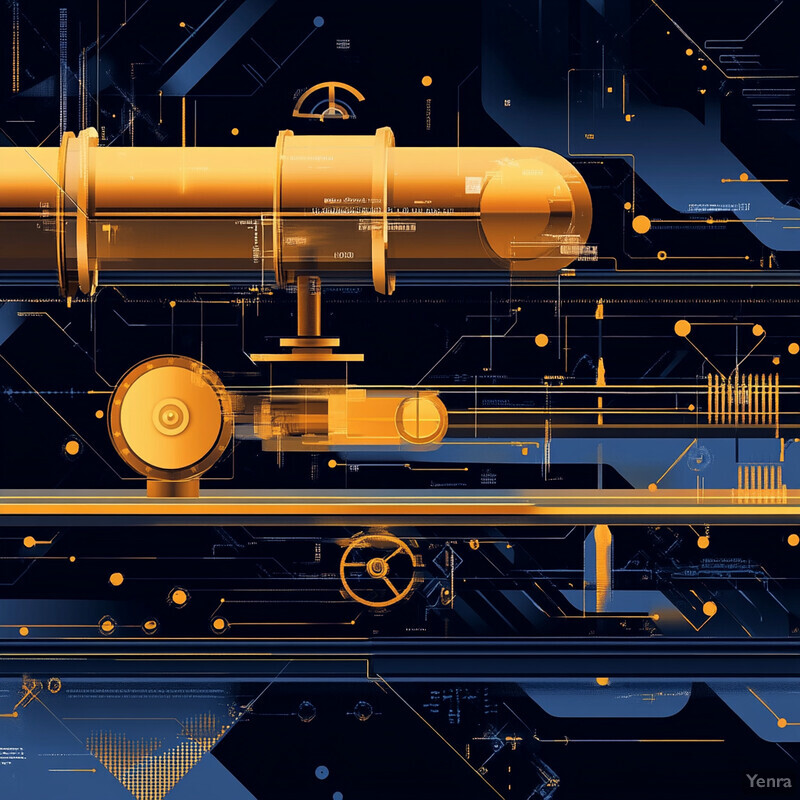
Capital investments in water infrastructure—such as replacing aging pipes or upgrading treatment facilities—carry significant financial stakes. AI-driven analytics help utilities prioritize these investments by evaluating asset risk profiles, historical failure rates, maintenance costs, and future demand projections. By focusing on high-risk areas or infrastructure components nearing the end of their useful life, utilities can invest wisely, reduce long-term expenses, and ensure that limited capital leads to tangible improvements in reliability, efficiency, and safety.
20. Continuous Improvement through Machine Learning
As AI models learn from ongoing operations, they continually refine their predictions, control strategies, and maintenance schedules, driving incremental improvements in efficiency, reliability, and sustainability.
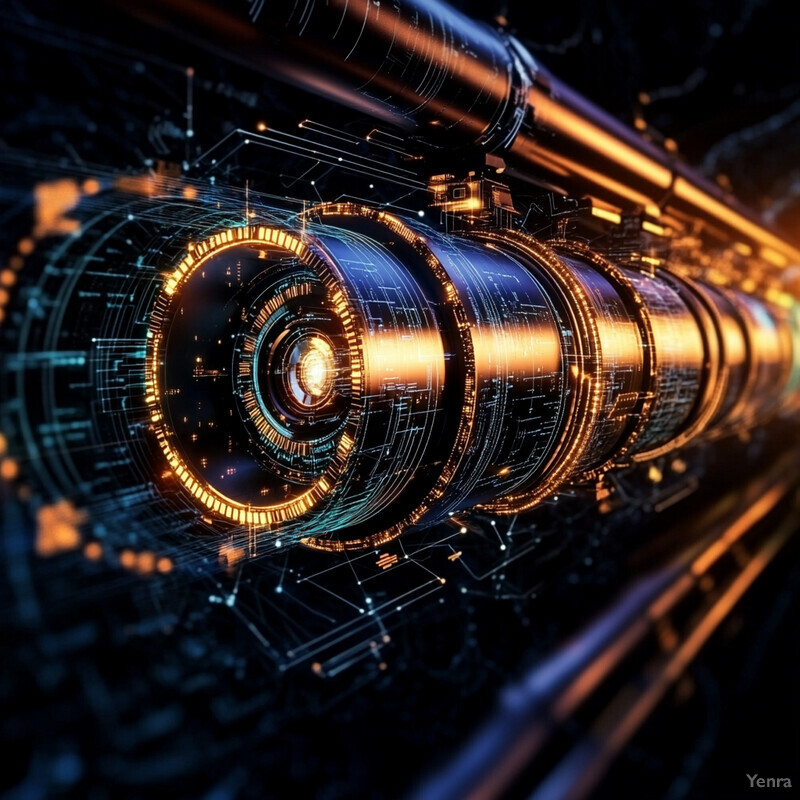
Unlike static, rules-based systems, AI-driven water management solutions evolve and improve over time. Continuous learning processes ingest new data, incorporate user feedback, and update models based on changing conditions. As the AI refines its predictions and control strategies, the water distribution network becomes ever more efficient, reliable, and sustainable. This iterative process ensures that the system adapts to new challenges—such as emerging regulations, technological advancements, or unprecedented demand patterns—and remains at the forefront of intelligent water management.