1. Semantic Search and Understanding
AI-driven legal research tools leverage natural language processing to interpret queries semantically, rather than relying solely on keyword matching. This allows attorneys to find relevant cases or statutes even when their search terms don’t exactly match the legal language of the documents.
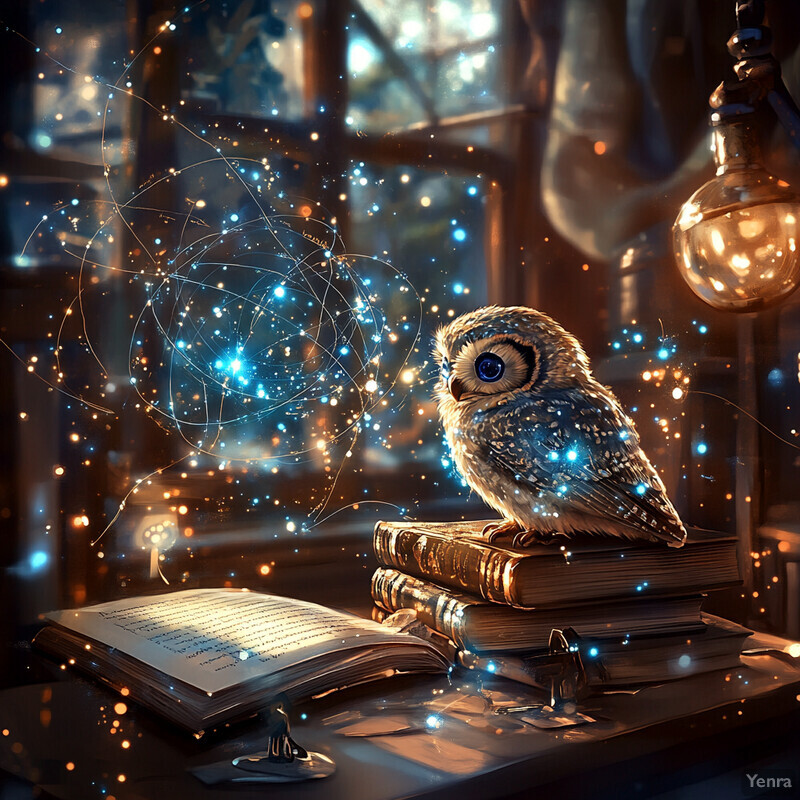
Traditional legal research tools often rely on exact keyword matching, which can overlook documents that use different terminology to describe similar legal concepts. AI-powered semantic search tools harness natural language processing and deep learning to understand the underlying meaning of queries. Instead of merely scanning for keyword occurrences, these systems analyze the context of words, their synonyms, and how they relate to one another. For example, a query about “employee dismissal” might retrieve cases related to “termination,” “firing,” or “unlawful discharge,” even if those exact terms weren’t used in the search. By interpreting the intent behind the query, semantic search significantly increases precision and recall, helping legal professionals find the most relevant authority in a fraction of the time.
2. Contextual Document Ranking
AI models apply context-sensitive ranking algorithms that prioritize documents by their relevance within a legal domain. They consider subtle relationships between legal concepts, cases, and statutes to deliver the most pertinent results first.
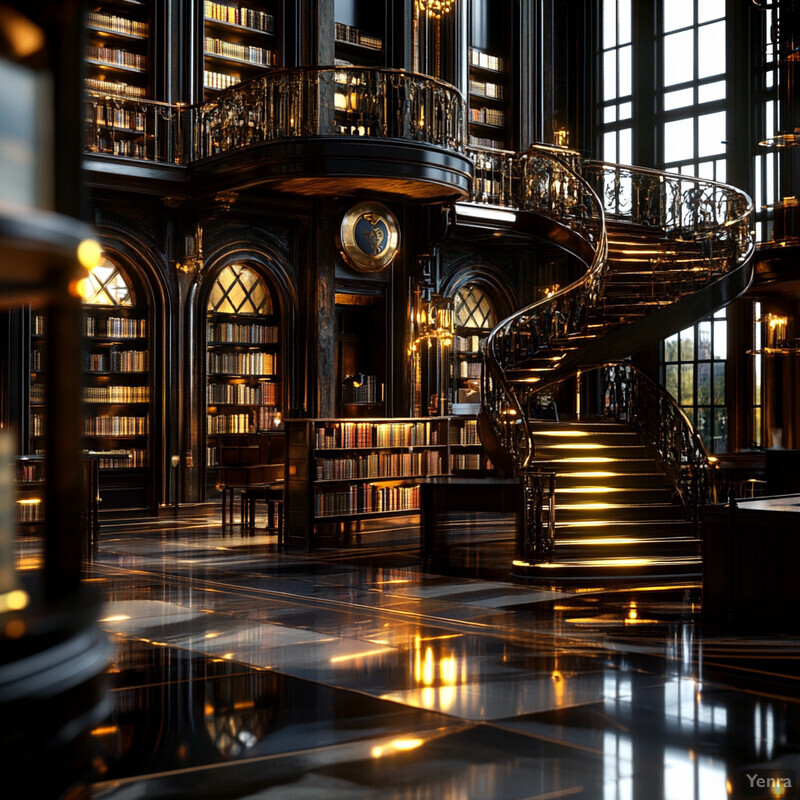
Legal research often results in numerous hits, and not all results are equally useful. AI-driven retrieval tools go beyond simple keyword frequency rankings by employing sophisticated algorithms that consider legal context, relationships between cited authorities, and the importance of certain passages. For instance, an AI system will consider not just how many times a case is cited, but whether it’s frequently cited for the same issue under review. By integrating legal domain knowledge into ranking models—such as recognizing which cases are considered landmark precedents—these systems prioritize the documents most likely to answer a researcher’s questions. The result is a more intuitive, context-informed hierarchy of search results that mirrors the way a seasoned attorney would sift through authorities.
3. Automated Topic Classification
Advanced machine learning classifiers can automatically categorize legal documents by topic, jurisdiction, and practice area. This streamlined categorization lets researchers quickly navigate to the relevant sets of cases or regulations.
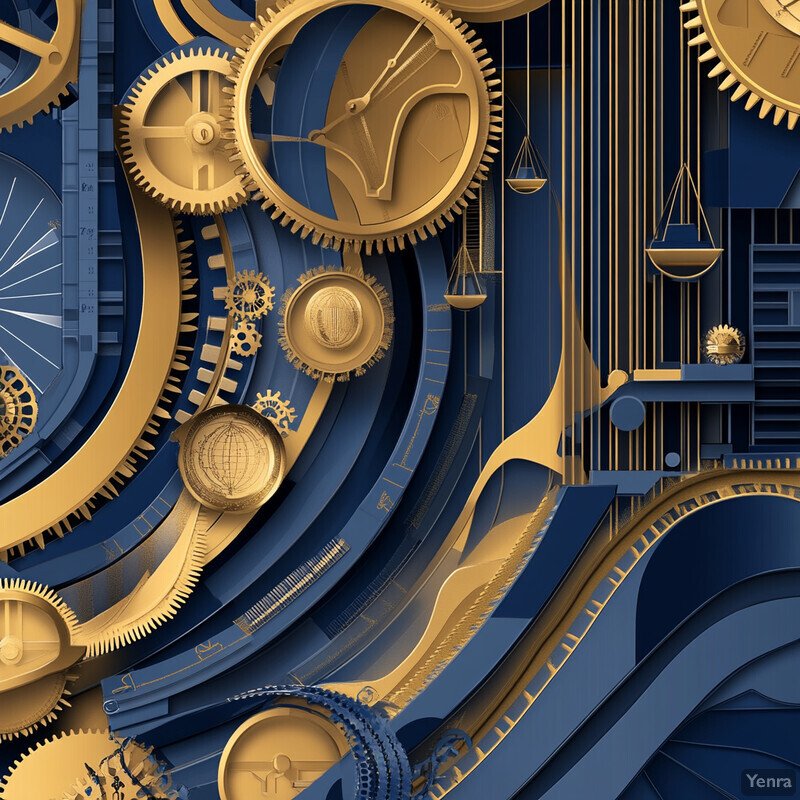
With vast amounts of legal content available, organizing documents by topic, jurisdiction, or practice area can be tedious. AI uses machine learning classifiers to automatically label and categorize documents, clustering them into coherent topical groups. This is particularly useful when dealing with multi-issue cases, voluminous discovery documents, or large regulatory archives. Instead of manually sorting through hundreds of documents to find those dealing with intellectual property or antitrust issues, legal researchers can rely on AI-driven topic classification to guide their searches directly to the relevant subsets. By reducing the labor-intensive aspects of classification, lawyers can focus on higher-level analysis and strategy.
4. Advanced Citation Analysis
AI systems can identify and weigh citations in judicial opinions to show how influential a case might be. By mapping citation networks, legal researchers can easily see which cases are considered central authorities and how legal arguments have evolved.
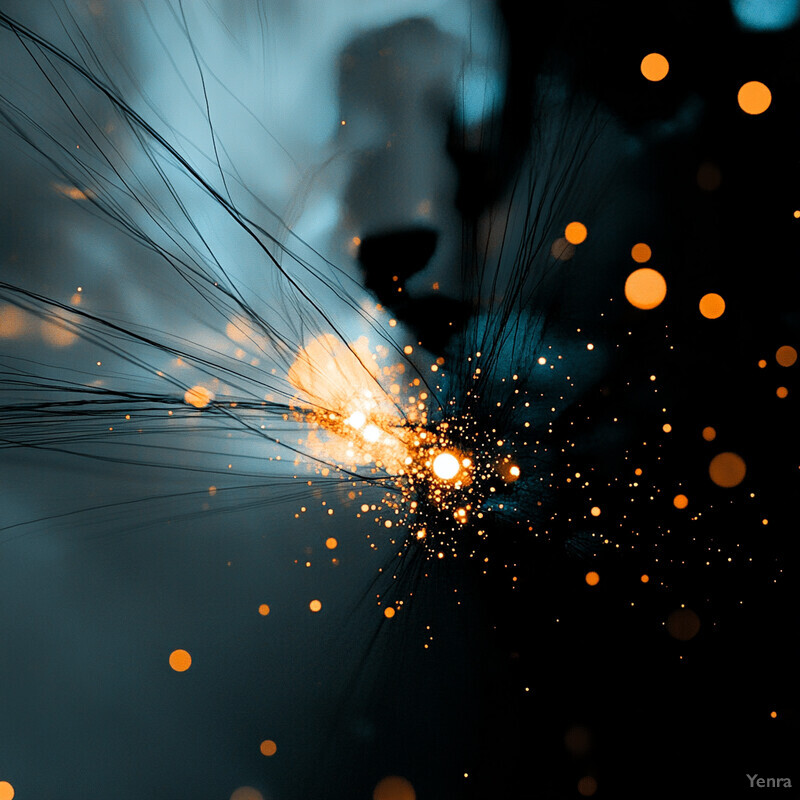
Citations form the backbone of legal research, illustrating how cases build upon or distinguish themselves from one another. AI tools can model legal citation networks to reveal patterns of authority and influence within a body of jurisprudence. They can track how often a case is cited, in what contexts, and whether it has been positively or negatively treated. This goes beyond a mere citation count and identifies key legal opinions that shape the interpretation of statutes or common law doctrines. Consequently, attorneys can quickly determine which cases carry the most weight, ascertain trends in judicial reasoning, and better anticipate how a court might view their arguments, ensuring that no influential precedent is missed.
5. Extracting Key Passages and Summaries
Using techniques such as summarization and passage retrieval, AI tools can pinpoint the most important sections of a long legal opinion or statute. Lawyers can quickly understand the crux of a case without reading every page.
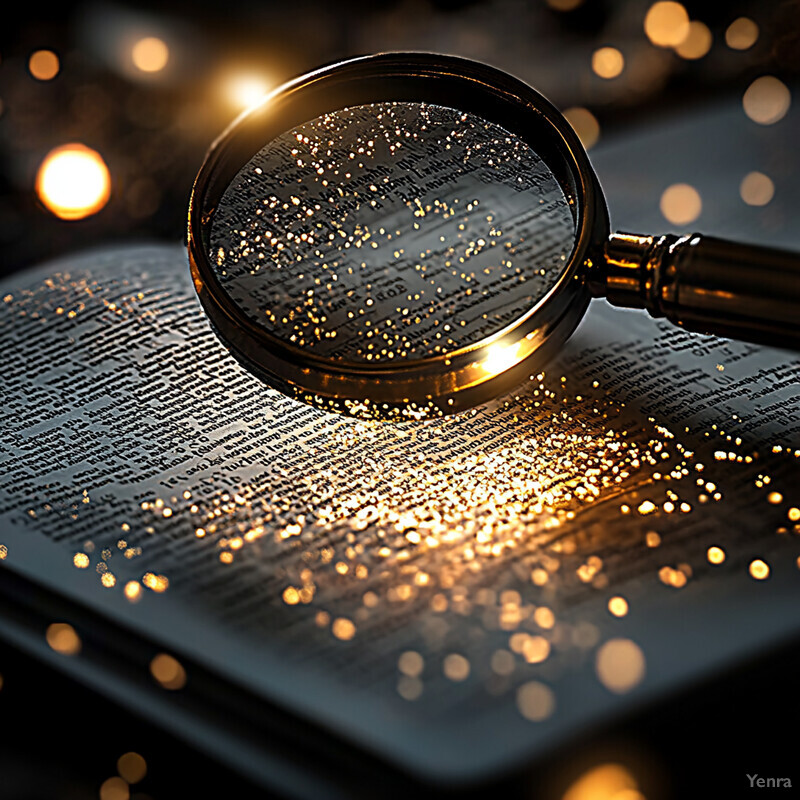
Long legal opinions, regulations, and statutes can run to hundreds of pages, making it difficult and time-consuming to identify the most relevant sections. AI-driven summarization algorithms and passage retrieval techniques allow researchers to pinpoint the essential parts of a document quickly. These tools highlight key holdings, legal standards, and reasoning paragraphs that matter most for a particular query. By reducing the time spent sifting through irrelevant text, legal professionals can focus on the substance of the authority, formulating arguments and strategies more efficiently. Summaries also help new practitioners or clients grasp complex legal issues without needing to become experts in the underlying intricacies.
6. Knowledge Graph Construction
AI can build rich knowledge graphs connecting various legal concepts, precedent relationships, and entities. This structured representation helps researchers discover indirect connections, related concepts, and comprehensive overviews of complex areas of law.
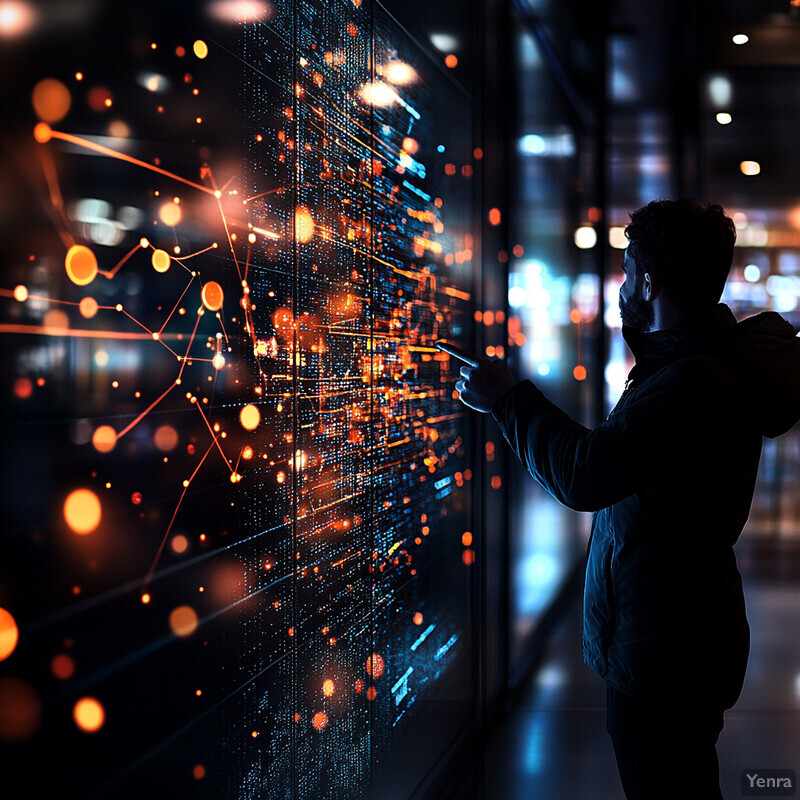
AI systems can represent legal concepts, entities, and relationships as knowledge graphs—complex data structures that map how statutes, regulations, and cases interrelate. By connecting people, laws, judicial decisions, and topics, these graphs enable researchers to navigate a rich web of information. For instance, a knowledge graph might show how a particular federal regulation interacts with multiple state statutes, related administrative rules, and leading cases that interpret its terms. This structured representation helps identify indirect links, discover previously unknown authorities, and gain a holistic understanding of complex legal frameworks. Knowledge graphs serve as a powerful tool for visualizing and exploring the interconnected nature of legal knowledge.
7. Question-Answering on Legal Material
With advances in large language models, AI can answer specific legal queries by navigating large corpora of case law and statutory text. This includes not just finding documents but providing direct, contextually accurate responses.
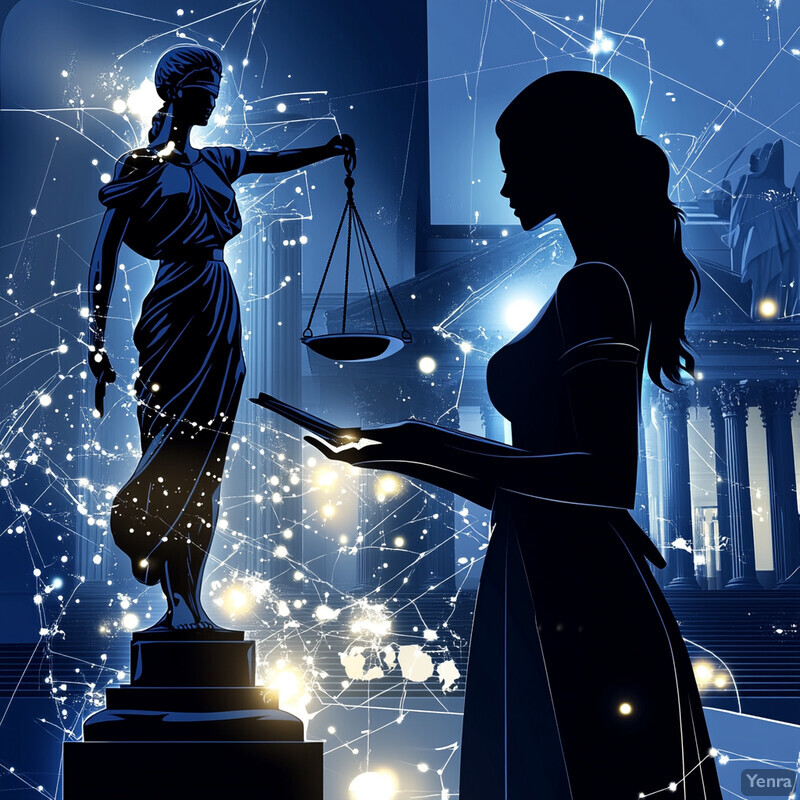
Advanced AI models now excel at answering direct queries posed in natural language. Instead of requiring a user to guess the right terms or filters, a legal researcher could ask, “What are the standard defenses to a breach of contract claim in California?” and receive a synthesized, contextually accurate response. Behind the scenes, the AI quickly navigates through relevant case law, statutes, and commentaries to present an integrated answer. These question-answering tools transform static legal databases into dynamic advisors, allowing even less-experienced practitioners to access targeted legal insights rapidly. By streamlining the question-to-answer workflow, attorneys save time and can focus on refining strategy rather than on mechanical information retrieval.
8. Context-Aware Referrals to Secondary Sources
AI systems can automatically detect when a researcher may benefit from secondary sources like treatises, annotations, or legal commentary. By understanding the context of a query, these tools suggest complementary reference materials.
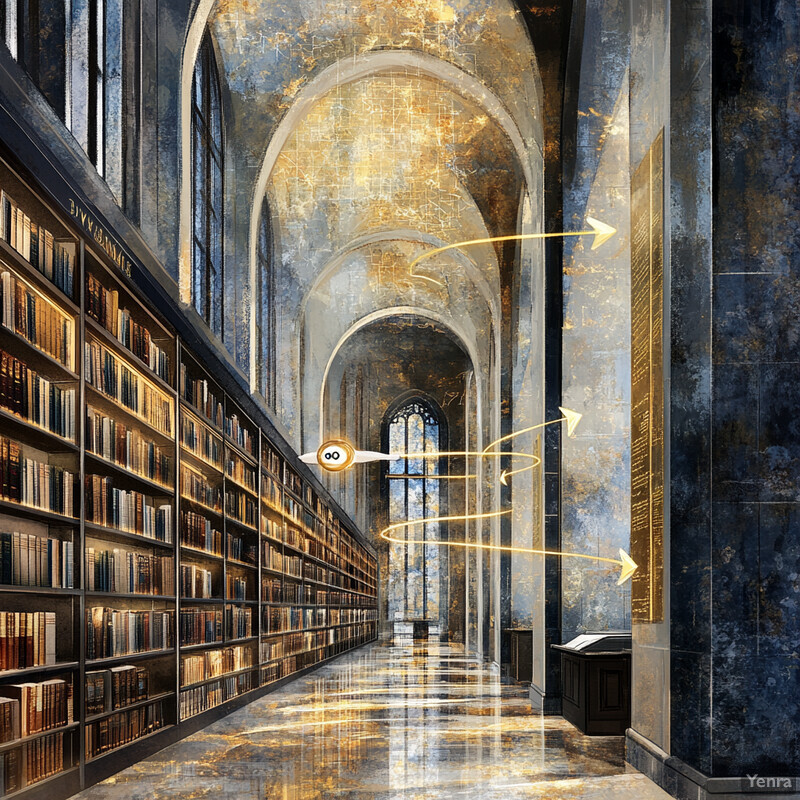
When a researcher examines a particular statute or case, AI tools can recognize if supplemental materials such as treatises, law review articles, or practice guides would provide additional insight. Context-aware recommendation engines tap into learned patterns of which secondary sources commonly help clarify certain legal ambiguities or provide deeper historical context. For example, while reviewing a labor law case, an AI system might suggest authoritative commentaries or leading treatises that analyze the evolution of that area of law. These curated referrals help legal professionals gain a richer, more informed perspective on the issues at hand, aiding both novices and experts in building stronger legal arguments.
9. Named Entity Recognition and Entity Linking
NLP models identify and standardize references to parties, judges, statutes, and regulations within documents. This allows researchers to filter by specific entities, track litigation histories, or uncover relationships between parties and outcomes.
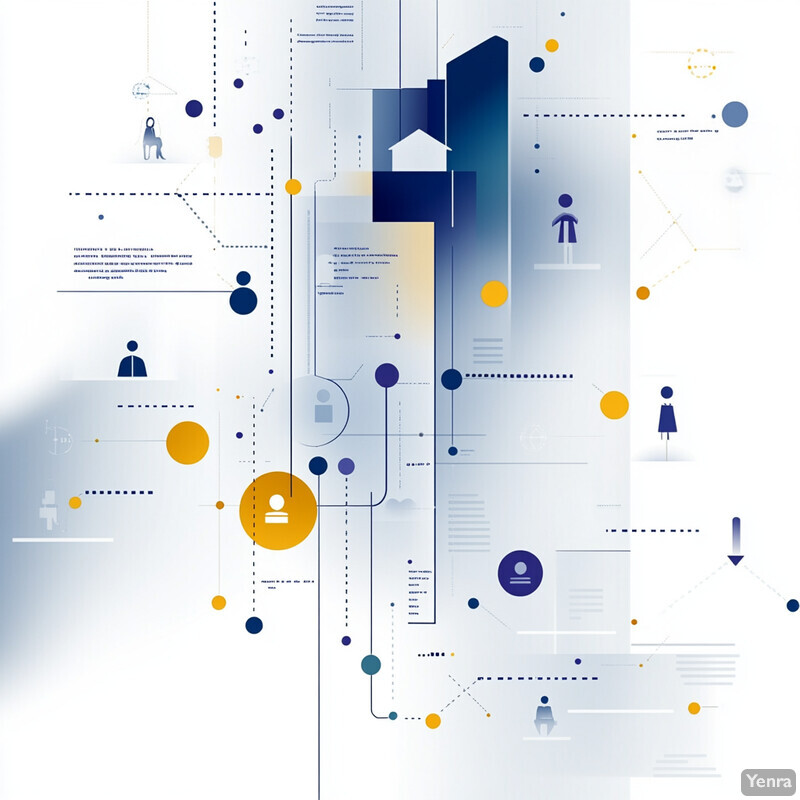
Legal documents frequently reference a myriad of entities: parties to a lawsuit, judges, administrative bodies, statutes, regulations, and geographical locations. AI-powered named entity recognition identifies these entities automatically, extracting structured data from unstructured texts. By linking these entities to standardized identifiers (such as the unique ID of a statute or the biography of a judge), researchers can seamlessly filter documents by the involved parties, find all cases mentioning a specific entity, or uncover patterns in how certain judges rule on similar issues. This enhanced entity-level granularity speeds up the research process and enriches the user’s overall understanding of the legal ecosystem.
10. Predictive Discovery of Relevant Cases
By analyzing patterns in prior searches and user behavior, AI can predict what type of cases or statutes a researcher might find most useful. This personalization saves time and ensures a more focused search experience.
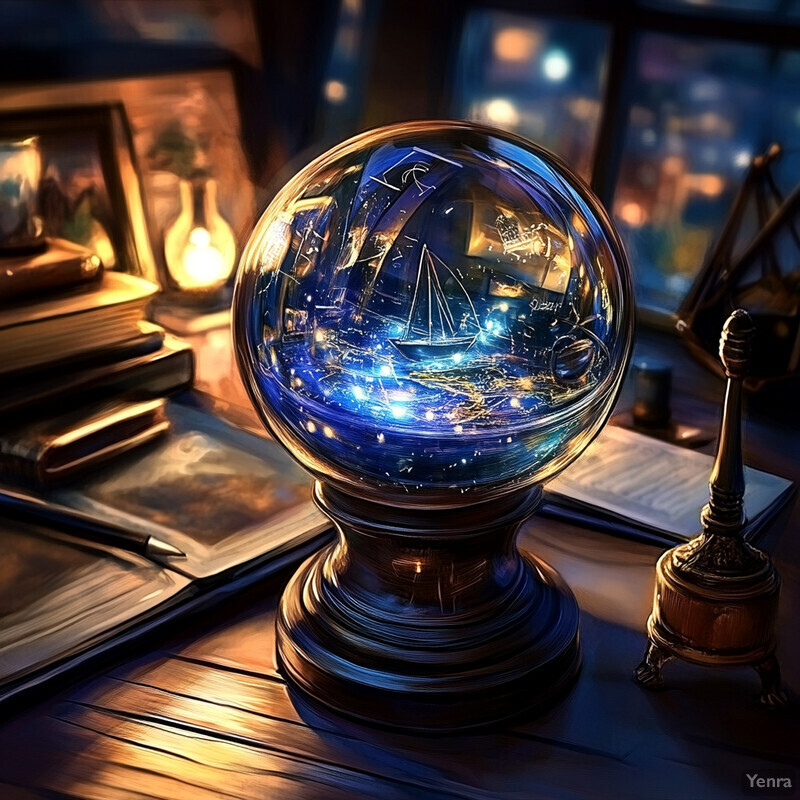
As an attorney navigates through documents, AI systems learn from their search patterns, noted cases, and favored jurisdictions. Over time, the system refines its predictions about what new cases or statutes the attorney might find most pertinent. By tailoring recommendations to user behavior, these predictive tools function like personalized research assistants that anticipate a researcher’s needs. They might suggest a lesser-known but factually similar case from another circuit or highlight an obscure statutory provision that supports an argument. In doing so, predictive discovery not only accelerates research but also ensures that valuable, underutilized sources are not overlooked.
11. Multilingual and Cross-Jurisdictional Retrieval
AI supports understanding and indexing of legal documents in multiple languages and from different jurisdictions. This capability broadens the search horizon for cross-border litigation or comparative legal research.
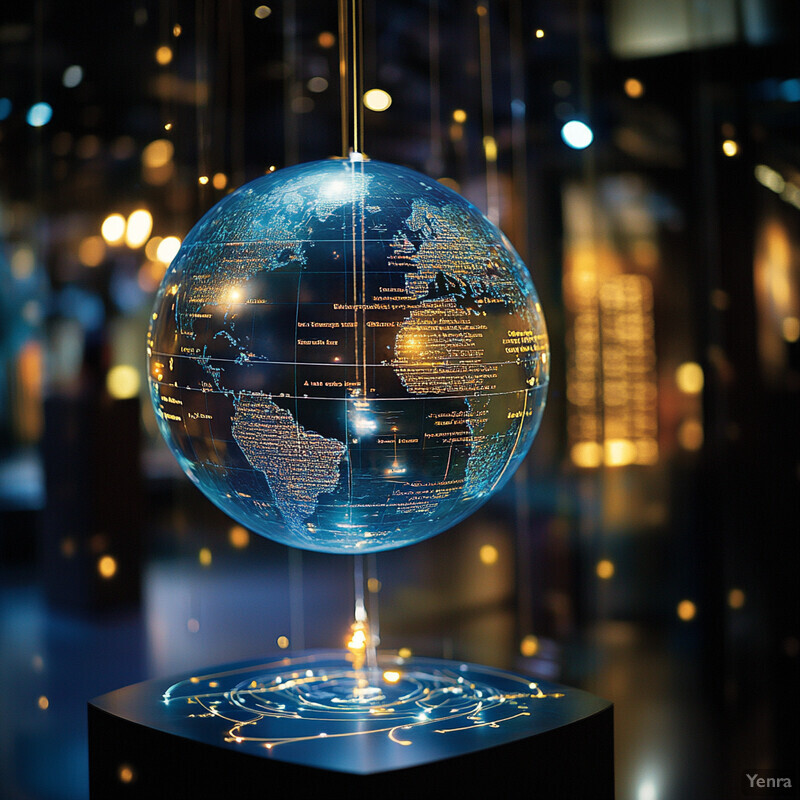
In an increasingly globalized legal environment, attorneys must sometimes work across multiple languages and legal systems. AI-driven translation and cross-lingual retrieval capabilities allow legal researchers to access foreign authorities or comparative law analyses. By harmonizing search queries across languages and legal traditions, AI tools make it possible to compare how different jurisdictions address a particular issue. This is indispensable in fields like international arbitration, cross-border M&A transactions, and human rights litigation. Efficiently retrieving and understanding foreign legal materials empowers attorneys to construct more robust, comparative legal arguments and broaden the scope of their practice.
12. Contextual Suggestion of Related Documents
Intelligent recommenders suggest related cases, commentary, or updated regulations as the user reviews a particular document, preventing critical omissions and supporting comprehensive research.
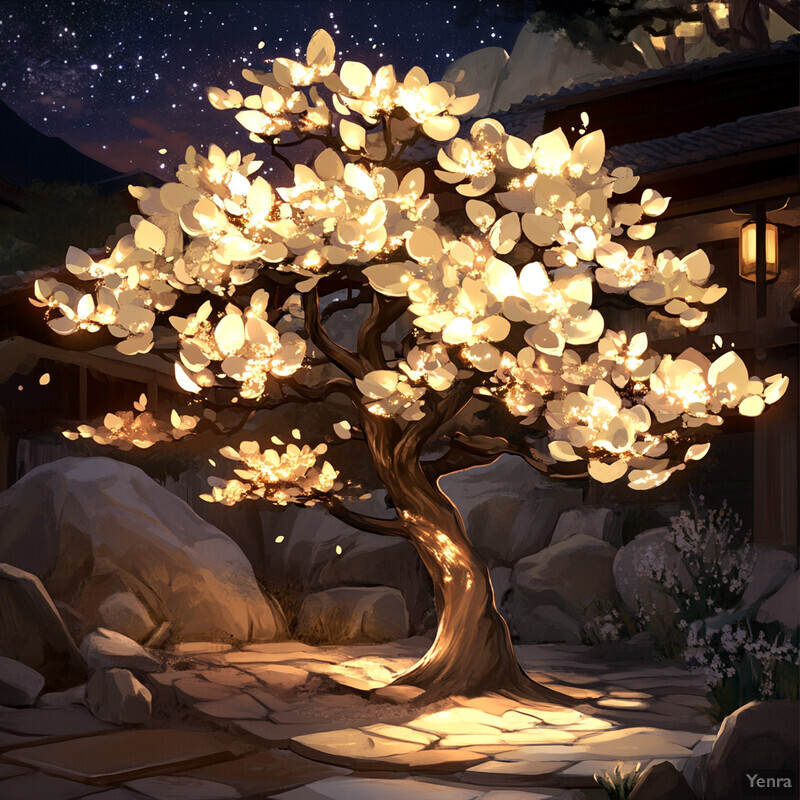
While researching one case or regulation, a lawyer might not realize that another, seemingly unrelated, precedent is relevant. AI-based recommendation engines, similar to those used by e-commerce platforms, continuously analyze what the user is reading, what issues are being explored, and which jurisdictions apply. They then suggest related cases, statutes, commentaries, or practice notes that could prove useful. This proactive guidance ensures that attorneys explore all angles of a legal problem and remain current with evolving authorities and interpretations. By surfacing these related materials at the right moment, contextual suggestions streamline the research process and lead to more comprehensive analysis.
13. Extractive Policy and Regulation Understanding
Machine learning models can parse through complex regulatory documents, extracting relevant clauses and highlighting areas of compliance or risk. This allows quick access to the core obligations without manual parsing.
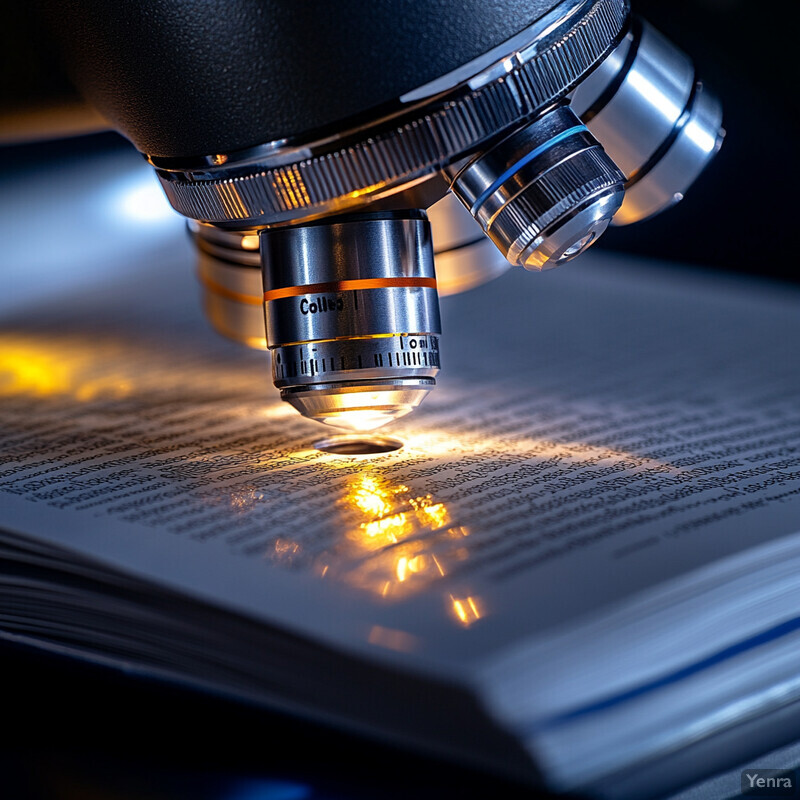
Regulatory frameworks can be dense and complex, making it challenging to extract specific obligations or compliance requirements. AI models trained on regulatory texts can scan through lengthy policy documents, identify key clauses, highlight critical definitions, and summarize requirements in plain language. This is particularly valuable in heavily regulated industries like healthcare, finance, or environmental law, where missing a subtle regulatory nuance can lead to non-compliance. With AI’s help, lawyers can quickly access the relevant portions of a rule, measure compliance risks, and advise clients effectively without spending hours parsing arcane legal prose.
14. Timeline and Historical Analysis
AI can assist in creating time-sensitive narratives of how a particular legal concept has evolved. Researchers can view the chronological development of case law, amendments, or doctrinal shifts, enabling a deeper historical understanding.
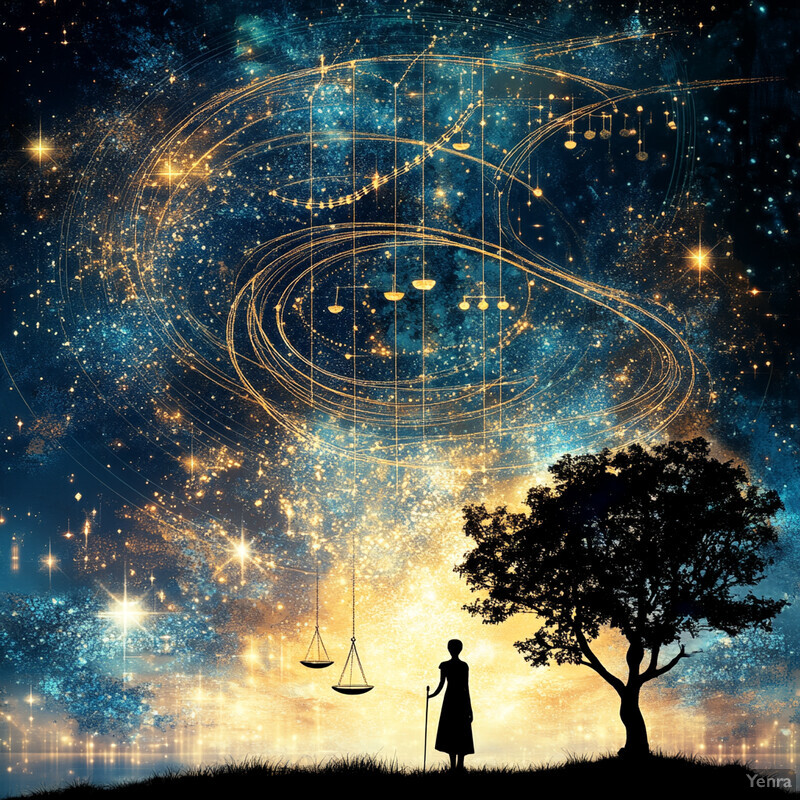
Understanding how legal doctrines evolve over time is crucial for both scholarly work and litigation strategy. AI tools can help create timelines that trace the development of a legal principle or statutory interpretation. By analyzing versions of statutes, legislative history documents, and the chronological order of relevant cases, attorneys can see how certain interpretations gained traction or lost influence. This perspective is invaluable when arguing that a doctrine should be extended, narrowed, or applied differently given historical trends. AI-generated timelines highlight shifts in judicial opinion and legislative intent, enabling lawyers to present nuanced arguments grounded in a deep historical context.
15. Enhanced Search for Legislative History
By applying NLP to legislative documents, drafts, committee reports, and debates, AI-powered tools help lawyers uncover legislative intent and the evolution of statutes, aiding in interpretation and argumentation.
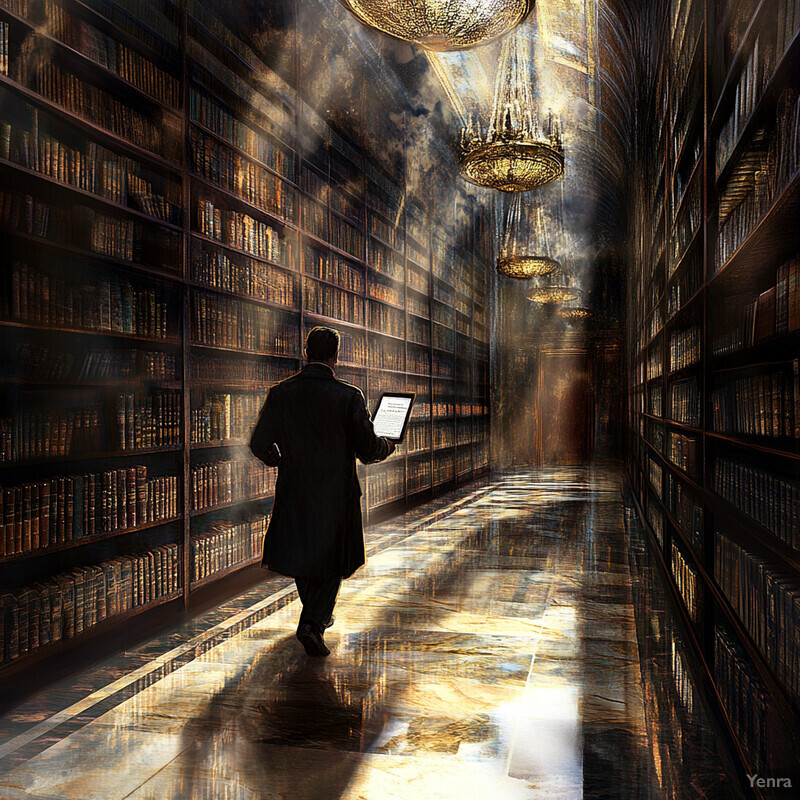
Determining legislative intent often requires examining not just the final statute but also committee reports, hearing transcripts, proposed amendments, and floor debates. AI systems can aggregate these scattered materials and make them searchable using natural language queries. Beyond mere keyword hits, advanced NLP tools can identify themes and patterns in the legislative record. For instance, a query about why certain language was added or removed from a bill can yield relevant excerpts from committee discussions. By simplifying this previously cumbersome process, AI empowers attorneys and judges to ground their interpretations in the actual aims and policy considerations that shaped the law’s enactment.
16. Adaptive Query Refinement
Intelligent search systems can suggest refinements or expansions to a query in real-time, guiding users toward better search strategies. This may involve recommending synonyms, related concepts, or more specific legal terms.
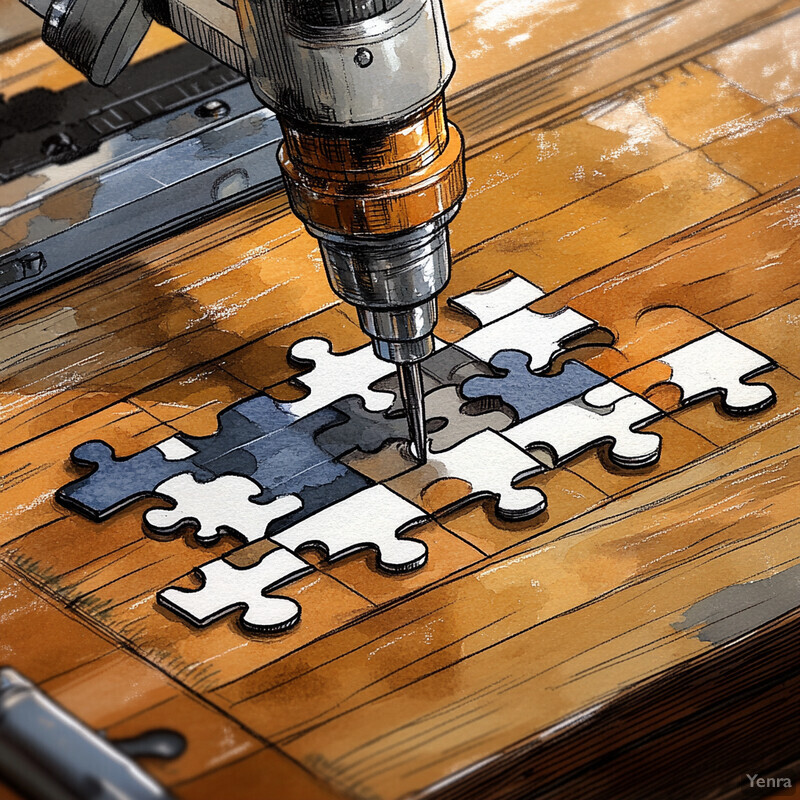
Many legal research queries begin vaguely, as lawyers might only have a rough idea of what they need. Adaptive query refinement tools use AI to provide dynamic suggestions—such as synonyms, legal terms of art, or related concepts—that can clarify or expand the search. If a user’s initial query returns too few relevant results, the system might propose more specific keywords or narrow down the jurisdiction. If the query is too narrow, it might suggest broader concepts or related issues. This iterative, intelligent feedback loop ensures that researchers rapidly converge on the most effective search strategy, reducing the risk of missing critical information.
17. Discovery of Unusual or Overlooked Precedents
Using pattern recognition, AI can highlight less-cited or older decisions that might still hold relevance. By surfacing these 'hidden gems,' researchers gain a more complete perspective.
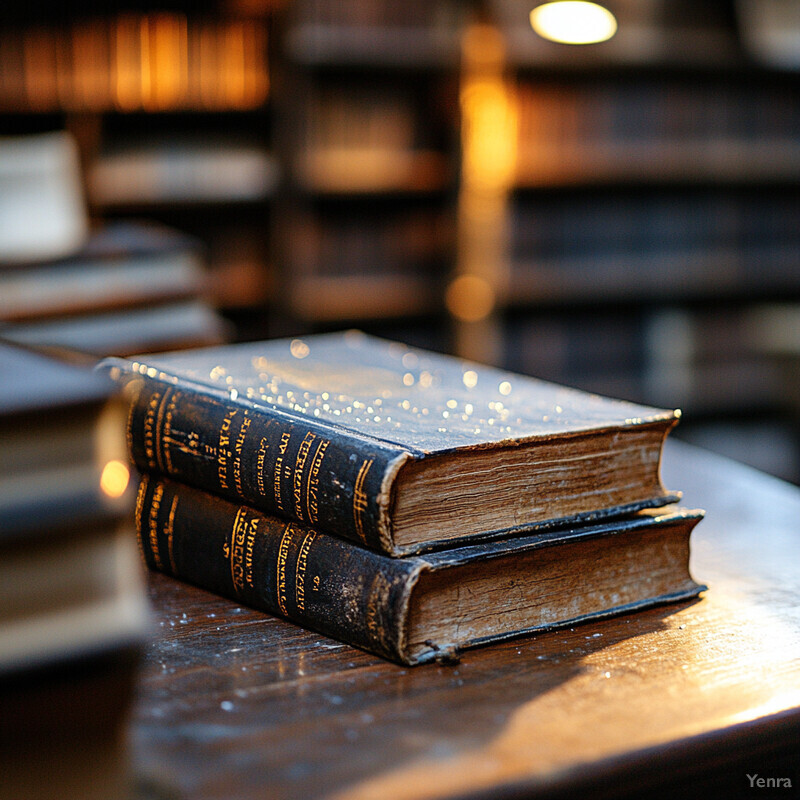
Some important cases may be cited infrequently or buried in older volumes, making them easy to miss during manual research. AI-powered retrieval systems, by virtue of their comprehensive analysis, can spotlight these “hidden gems” when they match the factual pattern or legal issue at hand. These cases might offer unique arguments, novel interpretations, or precedents from unusual jurisdictions that could strengthen a legal position. By surfacing older or less prominent authorities that still hold legal weight, AI ensures a more thorough and inventive approach to legal reasoning and advocacy.
18. Real-time Updates and Alerts
AI continuously indexes and analyzes newly published decisions, regulations, and commentary. Users can set tailored alerts to be immediately notified of relevant new developments, ensuring up-to-date research.
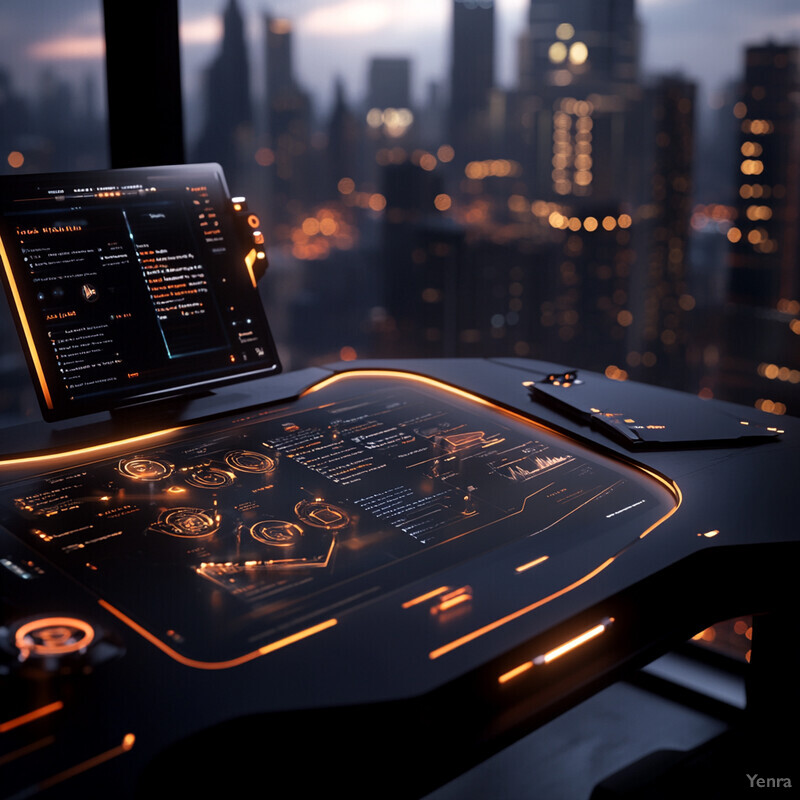
The law is constantly evolving, and staying abreast of new rulings, regulations, and legislative developments is a challenge. AI tools can monitor legal databases in real-time, automatically sending alerts when new materials relevant to the user’s interests or ongoing cases are published. These updates might include fresh appellate decisions on a key issue, newly passed legislation in a target jurisdiction, or the release of a critical regulatory guidance document. Such proactive notification systems enable lawyers to remain current, adapt arguments swiftly, and advise clients with the most up-to-date legal intelligence at their fingertips.
19. Integration with Litigation Analytics
By combining retrieval capabilities with predictive analytics, AI can not only find relevant documents but also inform strategies. For example, it might highlight how certain judges have ruled on similar motions or how particular legal arguments fare.
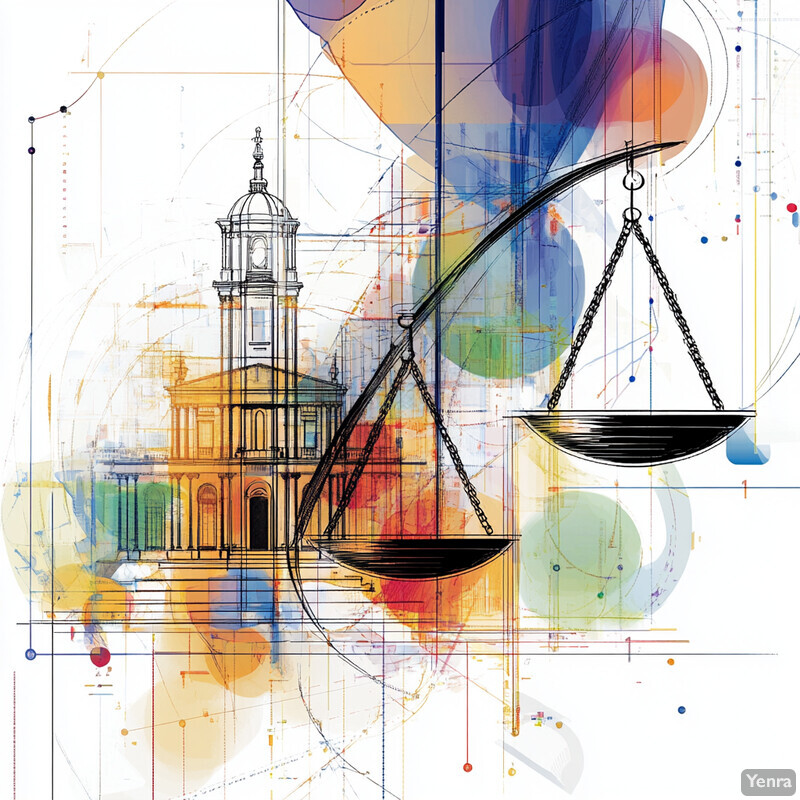
Beyond retrieving documents, AI-enhanced research platforms can integrate with litigation analytics systems that predict outcomes, analyze judicial decision patterns, and assess opposing counsel strategies. By linking retrieval and analytics, a lawyer researching a particular judge’s rulings on summary judgment motions could not only find relevant precedents but also understand how often that judge grants such motions. This synergy helps attorneys gauge the strength of their case, tailor their arguments, and allocate resources efficiently. The result is a more strategic and data-driven legal practice that leverages both authoritative documents and empirical insights.
20. Document Normalization and Standardization
AI techniques can clean and standardize varying formats of legal documents, making them easier to search and compare. This harmonization process reduces the impact of inconsistent formatting or archaic language.
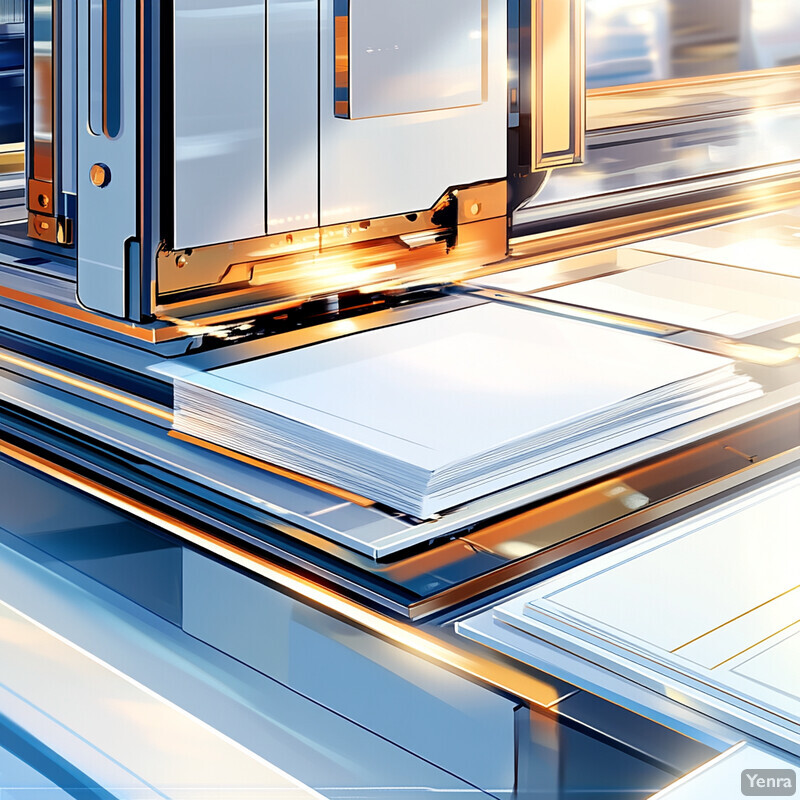
Legal documents come from myriad sources and may vary in format, style, and structure. AI can normalize these documents—harmonizing formatting, numbering systems, citation styles, and metadata—so that comparisons and cross-referencing become seamless. For example, if older cases use archaic terms or citation formats, the AI system can standardize them to modern equivalents, making it easier to conduct parallel analysis. This uniformity streamlines data management, simplifies advanced searches, and ensures that differences in document presentation do not hinder researchers. Ultimately, standardized document corpora increase efficiency, consistency, and clarity in the legal research process.