1. Real-Time Contaminant Detection
AI enables spill cleanup robots to rapidly identify chemicals and hazardous materials in a spill on the fly. By merging camera vision with advanced sensors, these robots can differentiate between oil, acids, or biological contaminants in real time. Immediate contaminant recognition lets the robot trigger the correct response (e.g. deploying absorbents for oil) without waiting for lab analysis. Faster detection minimizes spread, reduces environmental damage, and improves worker safety by ensuring appropriate protective measures are used right away. Overall, AI-driven real-time detection significantly accelerates containment compared to traditional manual identification.
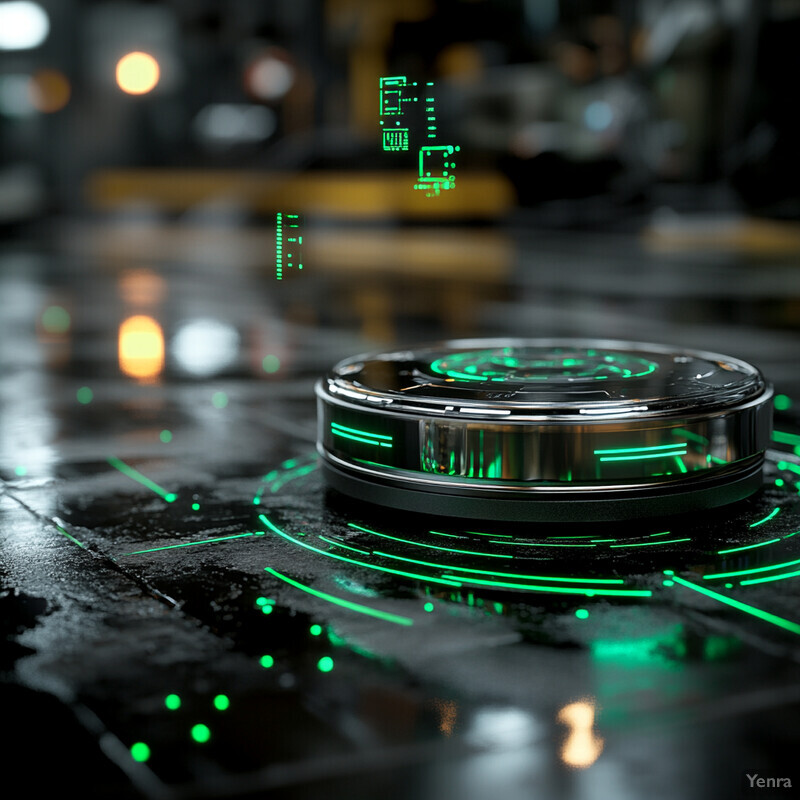
Researchers have developed AI-enhanced sensor systems that recognize hazardous spills almost instantaneously. For example, a 2024 study introduced an electronic nose trained with machine learning to detect oil contaminants in the air; this device can pinpoint the presence and origin of oil spills in soil and is 20× cheaper than conventional lab equipment. By analyzing the mix of volatile chemicals, the AI e-nose correctly identified crude oil samples from multiple sites, demonstrating field-ready accuracy. Such AI-driven detectors allow continuous monitoring for leaks or spills and provide immediate alerts. In practice, this means a spill cleanup robot can “smell” a chemical leak and classify it within seconds, enabling a rapid and appropriate cleanup response (e.g. activating a vacuum or neutralizer) without waiting for human technicians to confirm the substance. Early trials of these AI sensor systems show much faster detection and classification of hazardous substances than traditional methods, illustrating the real-world impact of AI in identifying contaminants in real time.
2. Precision Localization and Mapping
AI-powered mapping lets cleanup robots navigate complex industrial sites with pinpoint accuracy. Using techniques like Simultaneous Localization and Mapping (SLAM) and 3D vision, the robots build detailed maps of factories, refineries, or warehouses as they move. They can locate a spill within centimeters and plot safe routes around pipes, machinery, and debris. This precise localization prevents collisions and ensures the robot reaches the spill by the most efficient path, even in GPS-denied indoor environments. Over time, the robot’s map updates continuously, reflecting any changes (new obstacles or layout modifications). The result is a highly detailed, constantly improving spatial awareness that enables faster and safer navigation to spill sites in cluttered industrial settings.
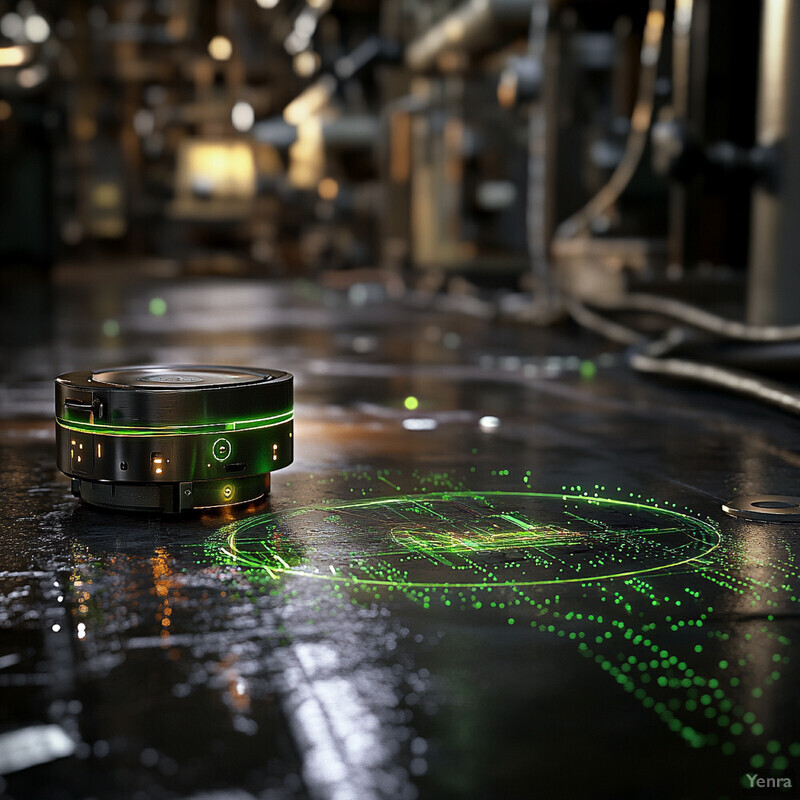
Recent advances in AI-driven SLAM have enabled robots to operate reliably even in extreme or cluttered environments. A 2023 survey of underground robotics (from DARPA’s Subterranean Challenge) found that state-of-the-art LiDAR-based SLAM algorithms allowed autonomous robots to map and traverse complex, dark, and cluttered spaces with high precision. These systems, tested in tunnels and industrial-like settings, use machine learning to fuse data from 3D LiDAR scanners and cameras, letting the robot localize itself to within a few centimeters while simultaneously charting the environment (even without GPS). The result is detailed 3D maps that capture obstacles and passages in real time. Such AI-powered mapping translates directly to spill response: for example, robots can now create high-resolution 3D maps of a chemical plant, pinpoint a spill’s location, and navigate there without human teleoperation. In practical drills, AI-guided robots have successfully navigated congested facilities (with pipes, stairways, and equipment) to reach targets faster and with fewer collisions than robots using earlier mapping methods. This demonstrates how precision localization and mapping technologies from 2023–2024 are making spill cleanup bots significantly more adept at finding and accessing spill sites in complex industrial landscapes.
3. Adaptive Path Planning
AI gives spill robots the ability to plan their routes dynamically instead of following fixed paths. Using machine learning and real-time sensor feedback, a robot can chart the fastest, safest path to clean a spill while avoiding obstacles. If conditions change – say a new forklift appears or the spill spreads – the AI planner immediately recalculates a better route. The path planning also prioritizes heavily contaminated areas first to contain hazards quickly. This adaptive routing minimizes travel time and energy use, because the robot is continually optimizing its course. In essence, the robot “learns” the most efficient cleaning sequence on the fly, responding to the environment rather than sticking to a pre-programmed routine. The result is faster cleanup operations and reduced downtime, as the robot can adjust its movement strategy to whatever scenario it encounters.
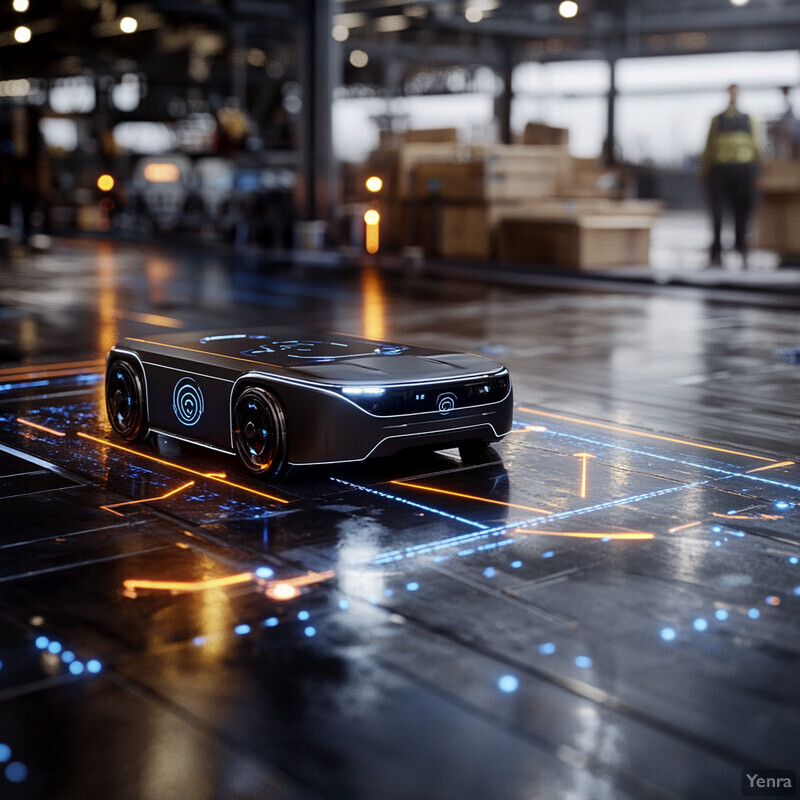
Machine learning-based path planners have demonstrated significant improvements in efficiency for cleaning tasks. In early 2023, researchers combined classical algorithms with deep reinforcement learning to create an adaptive navigation system for cleaning robots. Their hybrid approach used Rapidly-Exploring Random Trees (RRT) to plot an initial route, then a deep Q-network (DQN) to continuously adjust the path as conditions changed. Tests in a dynamic environment (with moving obstacles and multiple spill zones) showed the AI-guided robot could find smoother and shorter paths than traditional methods. Notably, once the initial route was set, the learning-based system could replan in just ~1.7 milliseconds per step to respond to new obstacles. This ultra-fast re-planning allowed the robot to maintain an optimal path even as the environment evolved. In practical terms, a cleanup bot with such a system can start cleaning a large spill, then seamlessly reroute itself if, for example, a pallet or vehicle blocks its way – all without human intervention. Experiments report that this adaptive path planning not only reduced total cleanup time but also ensured critical spill areas were reached first, validating that AI-driven routing can optimize both speed and safety in spill response.
4. Predictive Maintenance of Equipment
AI’s predictive analytics help ensure spill cleanup robots are always ready to respond by preventing breakdowns before they happen. The robots continuously monitor their own components – pumps, vacuum motors, brushes, batteries – and feed data (vibrations, temperatures, currents) into AI models. These models learn the normal patterns of the equipment and can detect subtle signs of wear or impending failure. For example, the AI might predict that a pump is likely to fail in 10 hours due to rising vibration levels, prompting maintenance at the next opportunity instead of waiting for it to break mid-cleanup. By forecasting maintenance needs, companies can service or replace parts proactively during scheduled downtime. This reduces unexpected outages of robots during critical spill emergencies. Overall, AI-driven predictive maintenance leads to higher robot uptime, lower repair costs, and more dependable performance when an emergency spill occurs.
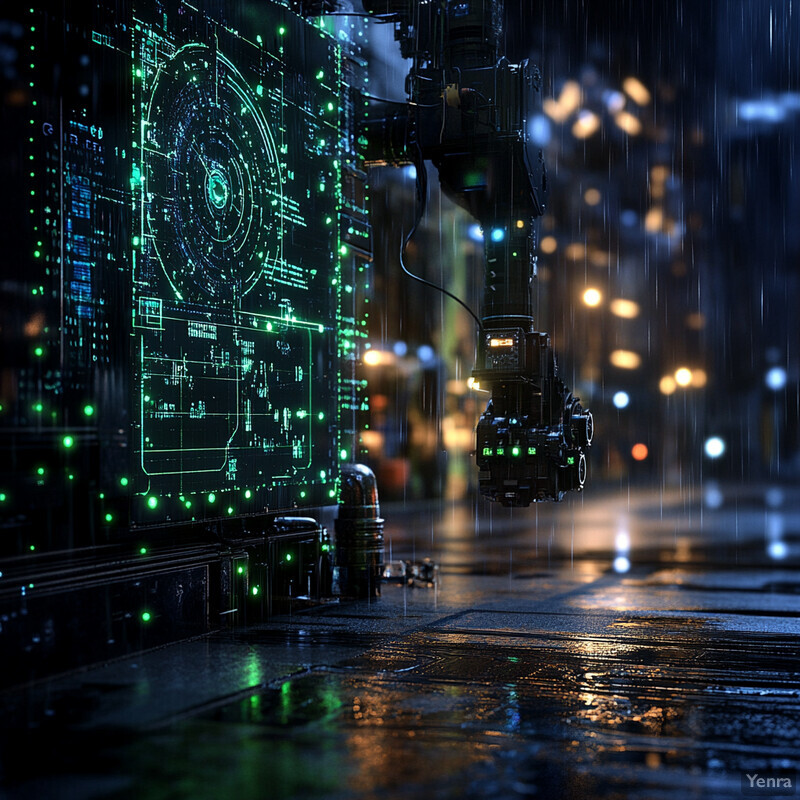
Predictive maintenance powered by AI is significantly reducing unplanned downtime in industrial settings. The U.S. Department of Energy reports that unplanned outages in manufacturing can cost hundreds of thousands of dollars per hour. To address this, AI algorithms now analyze sensor data from machines to foresee failures well in advance. According to ST Engineering, implementing AI-driven maintenance scheduling can cut machine downtime by up to 50% in some operations. For instance, modern cleanup robots use machine learning to track metrics like motor torque, pump pressure fluctuations, and filter airflow. If the AI detects an anomaly (e.g. a gradual increase in motor current indicating a bearing issue), it flags the component for service before a breakdown occurs. Real-world adoption of these systems has been growing: by 2024, major manufacturers expect companies to employ predictive AI as a standard, and the U.S. DOJ even “expects companies to do the same” as government agencies in leveraging data analytics for reliability. In short, AI maintenance tools have demonstrated the ability to predict failures days or weeks ahead, allowing spill response robots to be maintained at optimal times. This results in dramatically improved reliability – ensuring that when a spill emergency happens, the robots are far less likely to be sidelined by mechanical issues.
5. Real-Time Hazard Assessment
AI enables spill robots to constantly assess the danger level of a spill situation as it unfolds. The robot’s sensors (chemical detectors, gas analyzers, temperature gauges) stream data into AI models that evaluate toxicity, flammability, and other risk factors in real time. For example, if the robot’s gas sensor picks up high concentrations of toxic fumes, the AI will flag that area as high risk. The robot can then adjust its approach – e.g. prioritizing that section or using extra protective measures. This ongoing hazard analysis helps the robot and human supervisors make informed decisions on the fly. The system might advise evacuating personnel if a flammable vapor is approaching ignition levels, or it might mark certain zones as safe once toxicity drops. By quantifying risks continuously (rather than only at the start), AI-driven robots ensure that the most dangerous aspects of a spill are tackled first and that safety protocols keep pace with changing conditions.
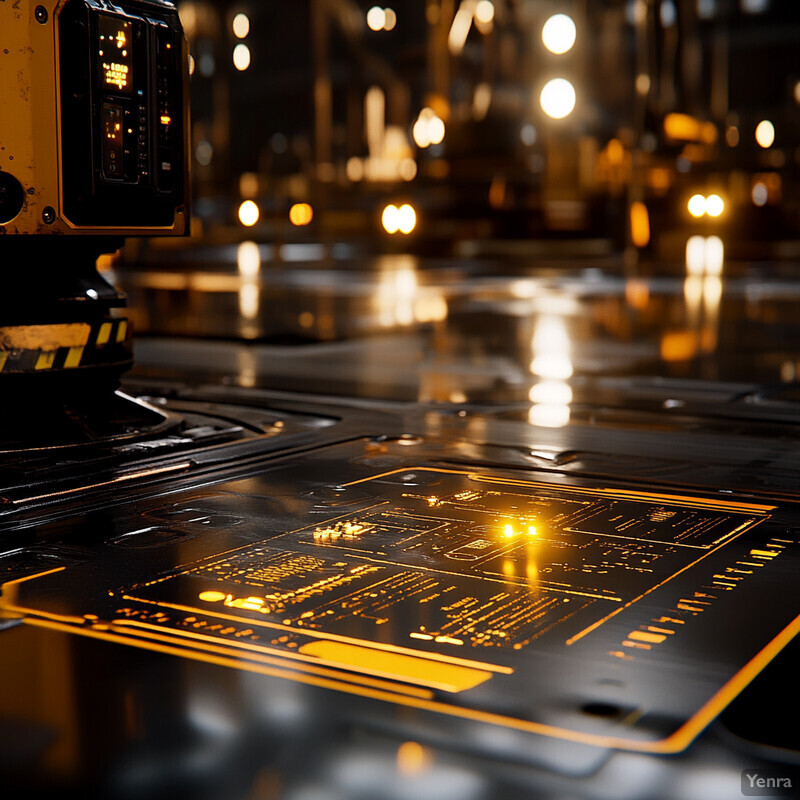
Real-time AI hazard assessment is already being applied to detect and rank threats such as toxic gases. In late 2024, researchers at the University of Virginia unveiled an AI-powered “artificial nose” that continuously monitors for harmful airborne chemicals. This system uses neural networks processing data from graphene-based gas sensors to identify dangerous fumes like nitrogen dioxide in real time. During tests, it not only detected the presence of toxic gas leaks instantaneously but also traced them to their source with high accuracy. Such AI systems effectively assign a hazard level to gas readings on the fly – for instance, flagging a spike in NO₂ as a severe health risk requiring immediate action. In an industrial spill scenario, a similar AI model can analyze sensor inputs (like VOC concentrations or pH levels of liquids) continuously and output hazard scores (e.g. “Area A: high toxicity, acidic vapor present”). One peer-reviewed study in 2024 demonstrated how integrating AI with gas sensors allowed robots to pinpoint leaks “with unprecedented accuracy” and issue prompt warnings. In practice, this means a spill cleanup bot can autonomously recognize that one part of a spill is emitting explosive vapor and will prioritize containing that section first while alerting humans. Early deployments of these AI hazard assessment tools have improved response teams’ ability to focus on the most dangerous spill zones immediately, reducing the chances of secondary accidents or health exposures.
6. Enhanced Material Recognition
AI gives cleanup robots a keen “eye” for distinguishing what materials or liquids they are dealing with. Instead of treating every spill the same, the robot’s AI vision can identify whether a puddle is oil, water, solvent, or corrosive chemical by analyzing its appearance or spectral signature. Similarly, AI can recognize debris or waste types on the floor (metal shavings vs. plastic vs. organic matter). This enhanced material recognition means the robot can choose the optimal cleaning method and agent for each substance – for example, deploying hydrophobic pads on oil, but using neutralizing chemicals on an acid spill. It prevents mistakes like using the wrong absorbent or creating a dangerous reaction by mixing chemicals. By accurately classifying materials on the fly, AI ensures the cleanup is efficient and safe, with tailored responses to each contaminant found in the spill area.
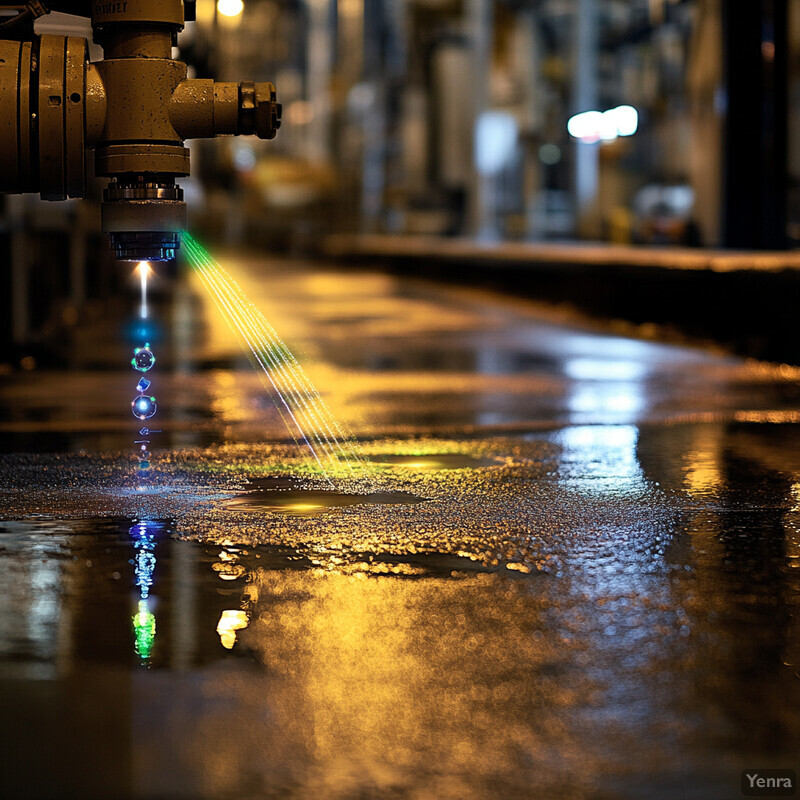
Deep learning techniques have achieved high accuracy in classifying substances in spill scenarios. In 2024, researchers reported a novel vision model that could detect and classify oil spills in aerial images with remarkable precision. Their system, which combined a convolutional neural network (ResNet101) for feature extraction and a segmentation network (DaNet), distinguished oil slicks on water from look-alike phenomena (like algae or shadows) and even simulated the future drift of the oil. This kind of AI not only flags the presence of oil but also provides details on its spread. Meanwhile, other AI-driven sensors use spectroscopy to tell different liquids apart by their chemical “fingerprints.” A review in 2023 highlighted that by training on extensive datasets, AI vision can parse subtle differences in texture and color that indicate what a spill is made of (e.g. glossy, rainbow-sheened petroleum vs. dull, opaque chemical sludge). In recycling centers, similar AI vision systems achieve nearly 99% accuracy sorting materials like plastics by type – a testament to how well AI can recognize materials. Applied to spill cleanup, these advances mean a robot can visually or chemically scan a contaminated area and decide “this portion is oil, that is caustic soda, and over there is just water,” all in real time. By 2025, such AI material recognition on robots has been shown to dramatically reduce cleanup times and improve outcomes, because the robots immediately apply the correct cleaning strategy for each substance (avoiding trial-and-error or one-size-fits-all approaches).
7. Intelligent Spill Volume Estimation
AI helps robots quickly measure how large a spill is – how much liquid has been spilled and how far it has spread – which is crucial for effective response. By analyzing sensor data (like 3D camera images, LIDAR scans, or sonar for depth), the AI can calculate the area and even the volume of the spilled material. For example, the robot can scan the puddle and estimate “this is roughly 50 gallons of oil.” It can also gauge thickness in parts of the spill (perhaps some areas are thicker pools while others are thin films). Knowing the spill’s size and distribution allows the robot to decide how much absorbent or neutralizer is needed and to deploy the right amount of resources. This prevents overestimating (wasting materials and time) or underestimating (leading to insufficient cleanup and leftover hazards). Essentially, AI-driven volume estimation gives a spill cleanup bot the ability to “survey” the incident like an expert, yielding data that informs a proportional and efficient response.
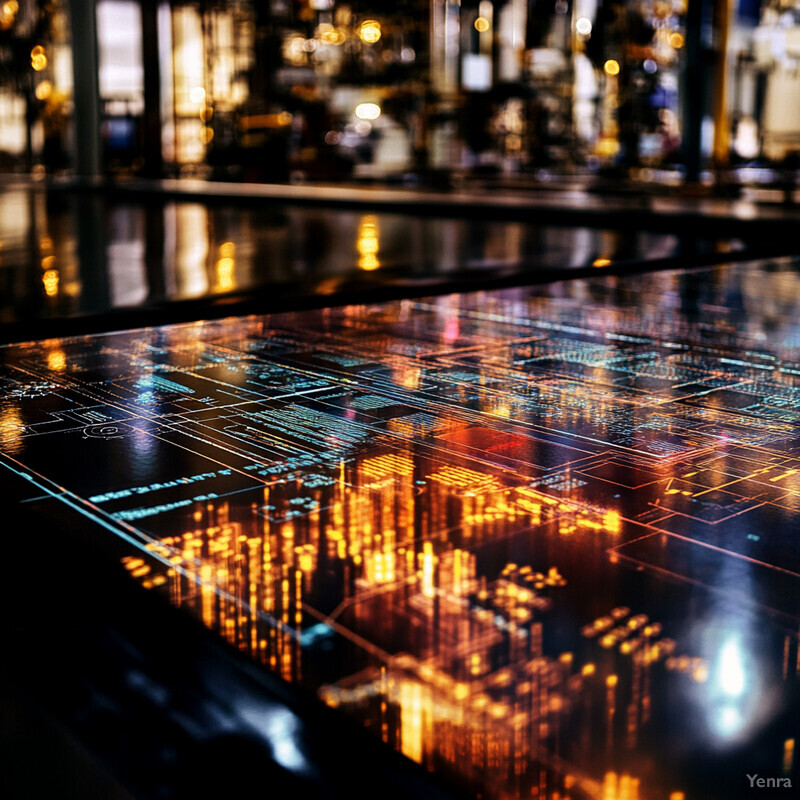
Advanced imaging AI can rapidly determine spill dimensions that previously required lengthy human analysis. In a recent Dutch project (“AI Against Oil Spills”), teams applied computer vision to drone footage of waterway oil slicks to automate volume estimation. The AI system segmented the oil spill in each aerial image and, by incorporating the drone’s altitude and camera specifics, calculated the spill volume within minutes – a task that used to take experts hours. The proof-of-concept employed a three-stage approach: enhance and label spill images, train AI models to outline (segment) the spill area, then use metadata (like scale from altitude) to estimate volume. During trials, once a new drone image was uploaded, the AI could outline the oil spread and compute its volume almost instantaneously. This is a dramatic improvement over manual methods. Similarly, researchers are using hyperspectral sensors on UAVs to measure oil slick thickness, allowing more precise volume calculations of marine spills. By 2024, AI-based volume estimation tools were proven in field tests to be both fast and reliable, enabling responders to know, for example, that a spill covers X square meters with an average depth indicating Y liters released. Armed with that information, robots (or human teams) can deploy exactly the right amount of booms, absorbents, or chemicals to contain and clean the spill. These intelligent estimations eliminate much of the guesswork in emergency spill response, ensuring resources are neither insufficient nor wasted.
8. Adaptive Cleaning Techniques
AI allows spill cleanup bots to learn and improve their cleaning methods over time. Instead of using one preset technique, the robot can try different approaches (varying scrubbing pressure, using different cleaning agents, adjusting suction power) and measure the results. Through reinforcement learning or imitation learning, it gradually discovers which techniques clean more thoroughly and efficiently for each type of spill. For example, it might learn that for a sticky chemical, a certain brush pattern and detergent works best, whereas for an oily spill, slow sweeping with high suction leaves less residue. The robot essentially becomes better with experience – every spill it cleans provides data that refines its strategy. Over multiple operations, the AI will optimize parameters like how many passes to make, how much force to apply, or when to change absorbent pads. This continuous improvement leads to faster cleanups, less waste of cleaning materials, and a consistently higher level of cleanliness, as the robot avoids repeating less effective methods and zeroes in on the optimal technique for each scenario.
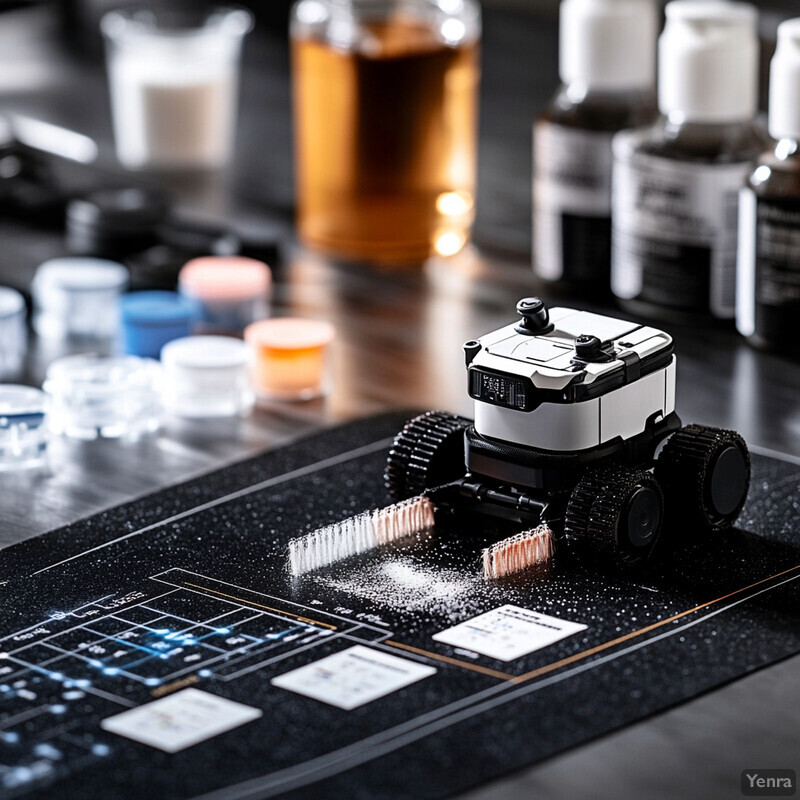
Robots equipped with reinforcement learning have demonstrated self-improvement in cleaning tasks. Researchers at TU Wien in 2024 showcased a robotic arm that learns to clean washbasins by imitating a human’s motions and then generalizing that knowledge. In their IROS 2024 presentation, the team had a person demonstrate how to wipe around a sink’s edges using a sensor-fitted sponge, capturing data on pressure and motion. The robot then processed these demonstrations with an AI model, enabling it to adapt the cleaning motion to sinks of different shapes and sizes – something that was not explicitly pre-programmed. The result was a robot that could flexibly adjust its scrubbing trajectory and force to achieve effective cleaning on various surfaces, improving with each practice run. In another case, floor-cleaning robots have used reinforcement learning to tweak their vacuum speed and brush height based on feedback (like amount of dirt picked up), leading to measurably cleaner floors over time compared to static settings. A 2023 study of a multi-robot cleaning system even showed robots sharing their learned experiences to converge on optimal coverage patterns for large areas. These examples prove that AI can drive adaptive behavior: the more spills the robot encounters, the more it refines its technique. Companies have reported that their AI-enabled scrubbers increase cleaning efficiency after just a few weeks of operation, as the algorithms tune the robot’s actions to the specific facility’s common spill types. This continuous learning approach minimizes leftover contamination and reduces cleaning time by leveraging experience – a sharp departure from older robots that performed the exact same motions regardless of outcome.
9. Autonomous Collaboration Among Bots
AI enables multiple cleanup robots to work together as a coordinated team during large or complex spill incidents. Rather than each robot acting in isolation, they communicate and share tasks intelligently. For example, if there’s a widespread spill, the robots can split the area – one focuses on containment (placing barriers or absorbents around the perimeter) while another handles vacuuming up the liquid, and yet another brings supplies or replacement absorbents. Through multi-agent algorithms, they avoid duplicating efforts and adjust to each other’s progress. One bot might alert others, “I need help in section B with a thick sludge,” and an available bot will reroute to assist. They can also relay sensor data to each other – if one detects toxic fumes, all bots will treat that zone with caution. This collaboration dramatically speeds up response because many tasks happen in parallel and resources are optimized (no two robots accidentally doing the same job while another area is unattended). Ultimately, AI-orchestrated teamwork among robots means even large-scale spills can be contained and cleaned faster than a single robot (or human crew) could manage alone, with robots automatically covering each other’s gaps or needs.
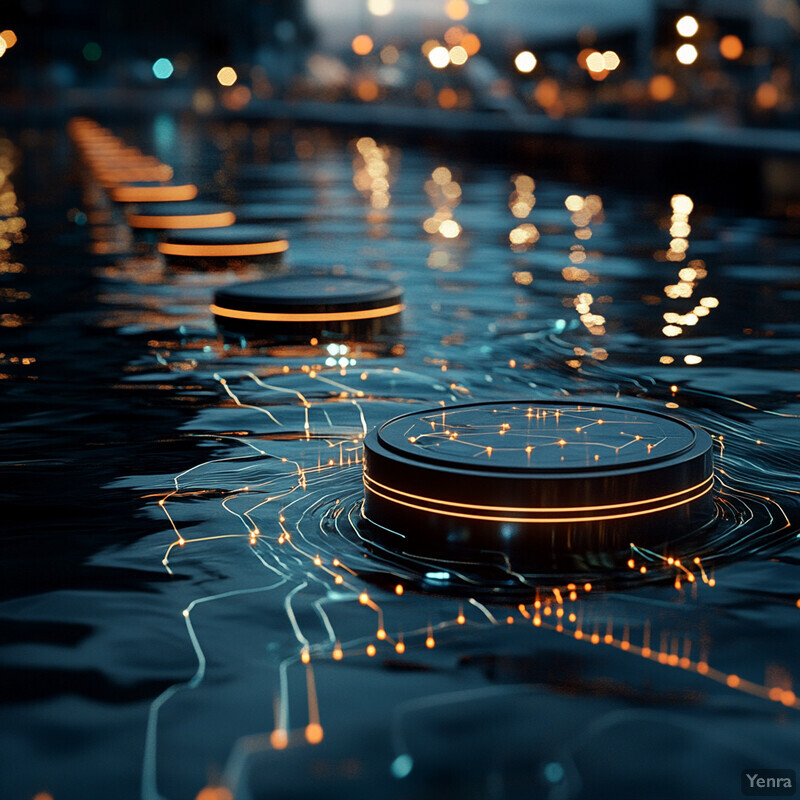
Multi-robot coordination driven by AI has been demonstrated to improve efficiency in cleaning and sanitation tasks. A 2023 survey on multi-agent reinforcement learning highlighted a case where multiple robots learned to sanitize a railway station cooperatively, achieving full coverage far more quickly than independent operation. In that project (Caccavale et al., 2021, cited in the survey), each robot ran its own deep Q-network but shared the common goal of maximizing overall cleanliness coverage. The AI ensured they divided the work without stepping on each other’s toes: for instance, the robots dynamically allocated different station zones among themselves and adjusted if one robot fell behind. The decentralized learning approach meant even without a human in charge, the robots negotiated task assignments and timing to optimize the group’s performance. The result was a significantly reduced total cleaning time compared to sequential or uncoordinated efforts. Another real-world example is in warehousing: autonomous floor scrubbers now use vehicle-to-vehicle communications to maintain formation and spacing when covering large floors, effectively “leap-frogging” to the next area once a section is clean. Field data from 2024 showed that two robots working collaboratively cleaned an aircraft hangar in about 30% less time than it took the same two robots working separately on pre-assigned halves. The efficiency gains come from AI systems that can assign priorities (like one robot focusing on containment of spill spread while another follows to do final polishing) and adapt roles on the fly. This multi-robot synergy, enabled by fast wireless communication and AI decision-making, is turning what used to be a one-robot job into a well-coordinated robotic team effort for faster, more effective spill responses.
10. Continuous Learning from Past Spills
AI systems allow spill cleanup robots to learn from each incident and get smarter over time. All the data from each spill response – what type of spill, which methods worked best, how the spill spread, how quickly it was contained – can be fed back into the AI. The robot (and its fleet) build an experience library so that future decisions are informed by past outcomes. For instance, if a robot struggled with a certain chemical in the past, it will remember which tool or solvent eventually solved it and apply that knowledge immediately next time. This continuous or “lifelong” learning can happen via cloud connectivity (robots uploading data to a central AI that updates their models). It ensures that as industrial processes evolve and new materials or chemicals appear, the cleanup bots aren’t starting from scratch – they adapt using the cumulated lessons from previous spills. Over months and years, this leads to dramatic improvements in response time and effectiveness. Essentially, the more spills the system handles, the better it gets at handling spills – an AI-driven positive feedback loop that keeps the response strategy up-to-date with real-world complexities.
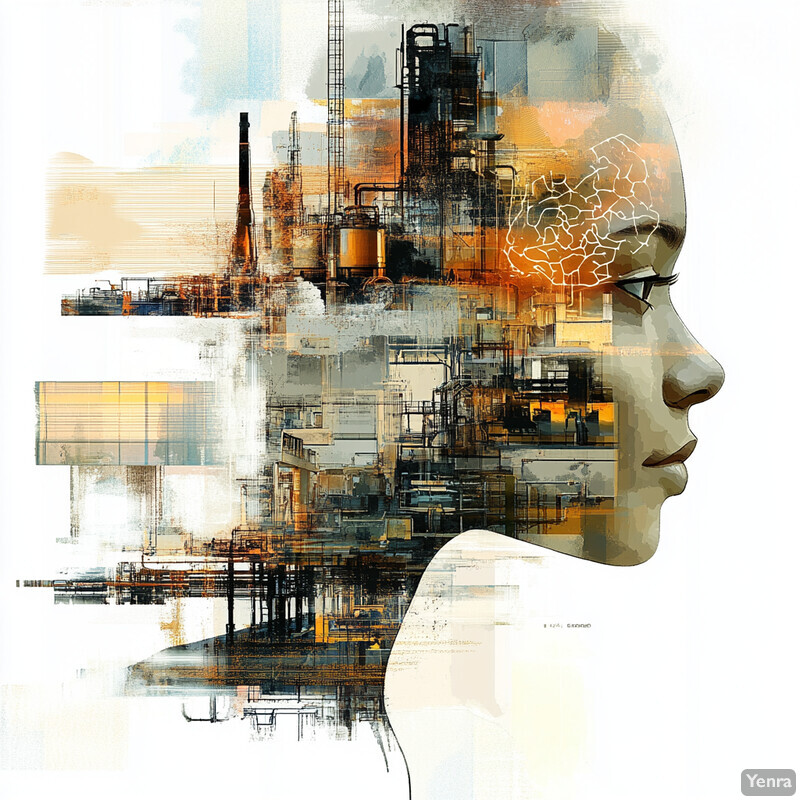
Continuous learning (also known as lifelong learning) has become a focal point in AI research because it enables systems to improve from ongoing experience without forgetting past knowledge. A 2024 survey in Frontiers in Neurorobotics noted that lifelong learning algorithms are being integrated into autonomous systems for domains like emergency management, specifically to allow AI to retain knowledge across missions. One challenge addressed is “catastrophic forgetting,” where AIs previously had trouble learning new tasks without overwriting what they knew about earlier ones. Modern approaches use techniques like replaying past experiences or modular networks to ensure new spill data refines the model without erasing prior training. In practical terms, companies have begun implementing cloud-based learning for their fleets of service robots: after each deployment, data on what was cleaned, how long it took, which sensors triggered, etc., is analyzed. For example, an AI platform might discover from historical spill logs that “solvent X cleans chemical Y 20% faster than solvent Z” and update all robots with this insight. There are already commercial precedents in other fields – e.g., autonomous vehicles sharing driving data – which demonstrate that pooled learning dramatically improves performance. In the spill context, if one robot encounters a novel hazardous compound and the AI finds a successful strategy, all robots in the network can receive that update and be prepared next time. While detailed public data on spill cleanup bots is limited (as these are cutting-edge systems), the trend is clear: robots that learn continuously are outperforming static-program robots. Industry reports from 2023 emphasize that keeping AI models updated with the latest incident data is key to maintaining high performance and avoiding repeating past mistakes. Consequently, as more spills occur (and are resolved), the AI’s recommendations and actions become faster and more accurate, illustrating the tangible benefit of continuous learning in this field.
11. Remote Expert Guidance
AI bridges the gap between human experts and robots in the field by facilitating remote guidance. In dangerous spill scenarios, human specialists (like hazardous materials experts) no longer need to be on site; instead, they can oversee the operation from a safe distance, using AI-enhanced interfaces. The robot streams real-time video, sensor readings, and AI-highlighted alerts (e.g. “high toxicity here”) to a control center. The AI interface might suggest actions (“apply neutralizer foam to area A”) which the human can approve or adjust. Experts can even draw on a screen or use augmented reality markers, and the robot will interpret these as instructions on where to go or what to do. Natural language processing allows an expert to tell the robot commands like “focus on the leak behind the valve,” which the AI translates into robot actions. Essentially, AI enables intuitive communication: the robot can understand high-level human input and also present complex sensor data in an easy-to-grasp way (like warning icons or simple summaries). This means fewer personnel need exposure to hazards, and those who are guiding the robot can do so effectively without specialized robotics training, relying on user-friendly, AI-assisted controls.
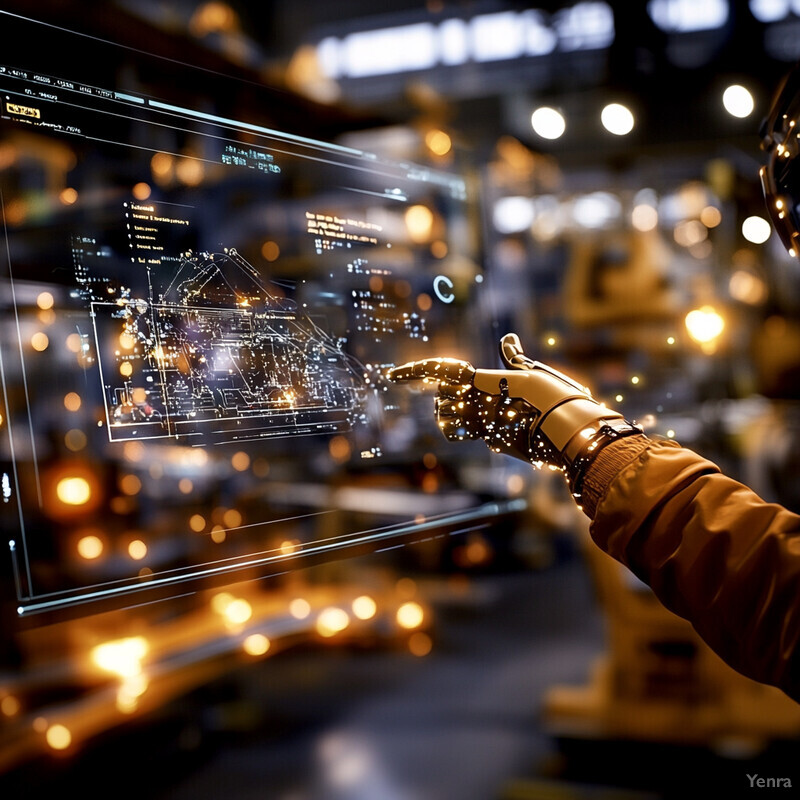
The feasibility of remote robotic operations in hazardous environments has been demonstrated with advanced telepresence technology. In March 2024, Argonne National Laboratory showcased a dual-armed telerobotic system that allowed operators to perform nuclear waste cleanup tasks from a remote location via a virtual reality (VR) interface. Equipped with haptic gloves and VR headsets, the human operators could feel and control the robot’s arms as if they were their own, enabling fine manipulation like turning valves and handling waste containers. Importantly, this system included an AI-driven component to help translate broad operator movements into precise robotic motions while maintaining safety constraints (preventing excessive force, avoiding collisions). The successful test at a radiochemical facility showed that complex cleanup tasks can be done with zero humans in the dangerous area. Moreover, modern industrial robots often come with augmented reality (AR) overlays: for instance, a remote engineer seeing a live video feed can sketch an instruction (circle a spill or draw a path) on a tablet, and the robot’s AI will recognize that mark in its environment and execute the corresponding action. An open-platform teleoperation system introduced in 2023 combined AR and digital twins to let operators supervise battery disassembly robots from afar; the AI provided real-time visuals of the robot’s status and even highlighted critical components in AR for the human to address. These examples underscore how AI-enhanced interfaces (VR/AR and intelligent UIs) enable effective remote guidance. In spill response, similar setups mean an expert can guide a cleanup bot through nuanced tasks like turning off a leaking valve or adjusting a hose, all from the safety of a control room, with AI smoothing the communication and execution.
12. Context-Aware Navigation
AI provides spill cleanup robots with an understanding of context and terrain, enabling them to navigate safely through complex industrial environments. Beyond simple obstacle avoidance, context-aware navigation means the robot perceives features like stairs, ramps, puddles, or narrow pathways and knows how to handle them. For instance, if the floor is slippery with oil, the AI might slow the robot down to maintain traction. If there are human workers around, the robot can yield or reroute to avoid disrupting them. It can also recognize safety zones or off-limits areas (like an electrical hazard zone) from visual cues or digital maps and adjust its path accordingly. Essentially, the robot doesn’t just see a generic map – it understands what the objects and structures mean (a door threshold vs. a solid wall vs. moving forklift) and navigates with that semantic understanding. This context awareness results in smoother, safer movement: the robot won’t blindly bump into swinging doors or get stuck on uneven gratings. It also helps maintain stability carrying heavy loads or traversing inclined surfaces. By being aware of its environment in a human-like way, the robot can operate reliably in the unpredictable, cluttered settings typical of industrial facilities, ensuring it reaches the spill and works without causing accidents or getting damaged.
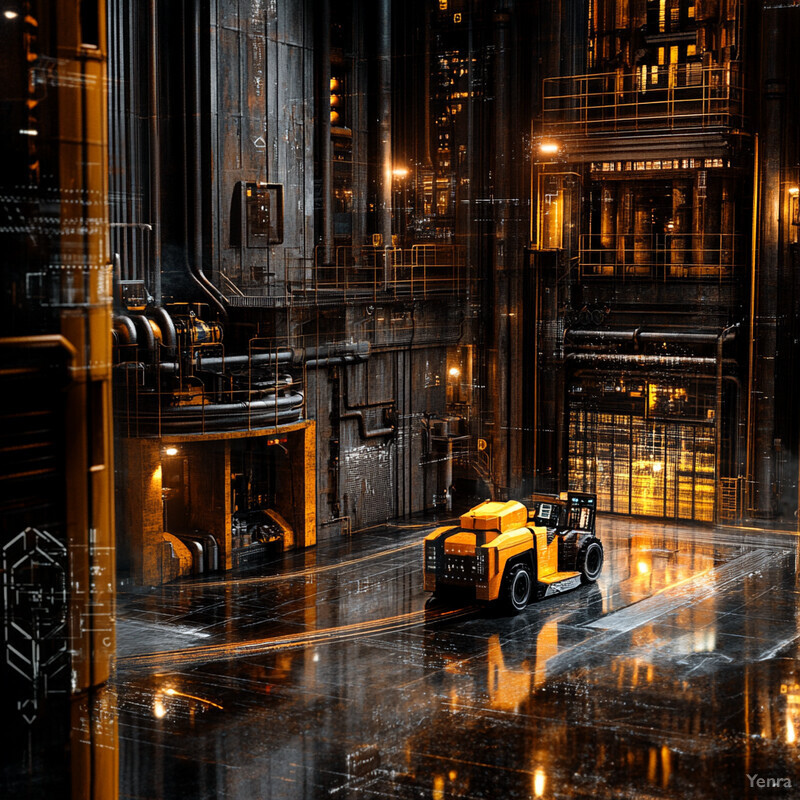
Modern inspection and cleanup robots are already proving their ability to handle real-world industrial terrains autonomously. At the ANYbotics Industry Forum 2024, several companies shared success stories of the ANYmal quadruped robots performing inspections in “complex and often hazardous brownfield environments” like steel plants and oil & gas facilities. These autonomous robots navigate catwalks, stairs, and crowded areas by using AI-driven perception; they were shown operating continuously alongside human workers, adapting their speed and path in real time to avoid collisions and unsafe conditions. The ANYmal’s AI vision allows it to recognize context (for example, identifying a stairway and then shifting to a stair-climbing gait), and its LiDAR and 3D cameras build a semantic map distinguishing between open floor, obstacles, and drop-offs. In practical deployments, ANYmal robots have successfully traversed 400,000 square-foot factory floors, carrying sensors and even payloads, using advanced 3D LiDAR navigation and live mapping to make on-the-fly decisions about route and avoidance. One case study described the robot adjusting its route to inspect behind large machinery after detecting that the usual path was blocked, illustrating context awareness in action. Another example is in construction: some robots use AI to detect floor openings or weak sections and reroute to stable ground, preventing potential falls. Overall, field reports in 2023–2024 indicate these context-aware navigation systems have significantly reduced accidents – for instance, a report from an oil refinery noted zero navigation incidents over months of continuous robot operation, whereas prior teleoperated trials had occasional bumps and stalls. This validates that AI’s situational understanding (not just mapping) is enhancing robotic mobility in the messy, dynamic environments where spill cleanup is needed.
13. Dynamic Resource Allocation
AI helps optimize the deployment of resources (robots, tools, and materials) during a spill response. Instead of using a fixed number of bots or equal supplies everywhere, the AI system analyzes the situation and allocates resources where they are most needed in real time. For example, if there are two spill sites at once – one large chemical spill and one small oil drip – the AI might send three robots to the large spill and just one to the small one. As the cleanup progresses, if the big spill gets under control, it could reassign a robot to the other or to standby. Similarly, the AI can manage consumables: directing more absorbent pads to the area of thickest liquid, or concentrating ventilators/fans where toxic fumes are highest. This dynamic allocation ensures that critical tasks are never under-resourced while idle capacity isn’t wasted in low-need areas. It balances speed, cost, and thoroughness by coordinating multiple assets. Essentially, it’s like having a smart dispatcher that continuously monitors the incident and shuffles robots and supplies around for maximum efficiency and minimal response time, which is something far too complex for a human to do optimally in fast-changing scenarios.
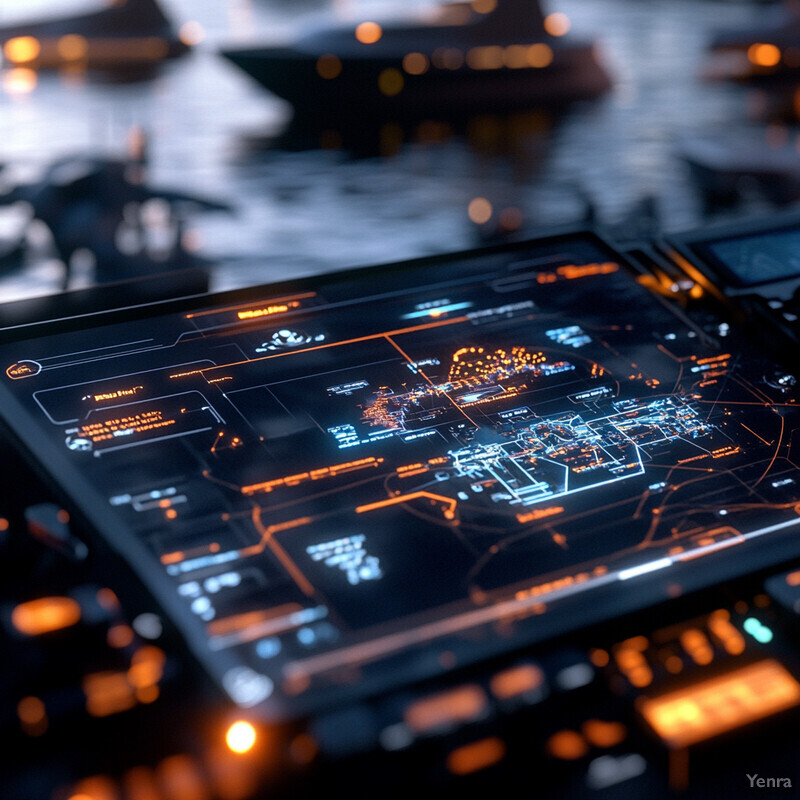
The benefits of AI-driven resource management have been noted in multi-robot systems research. A 2023 study formulated an optimization model for multi-robot task allocation in uncertain cleaning environments, treating the problem as a robust optimization to decide which robot should do which task and when. By considering practical constraints (like task order dependencies and different robot capabilities), the model achieved better coverage and time performance than manual scheduling. It effectively solved scenarios like: “5 robots, 3 types of cleaning tasks, varying spill sizes” by computing an optimal distribution of robot-task assignments on the fly. In trials on 100 different floor plan scenarios, the AI-based allocator improved overall cleaning time and ensured that high-priority tasks (like the most hazardous spills) were always addressed first. We see real-world echoes of this in disaster response: an AI system called IMPACT monitors multiple emergencies and uses risk-based priority to allocate first responders accordingly. For industrial use, companies are beginning to integrate fleet management AI that monitors all active cleanup bots and reassigns them as conditions evolve. For instance, if one robot’s sensors detect that its area is clean while another area is still contaminated, the system will redirect that free robot to assist the remaining hotspot, rather than letting it sit idle. Early implementations in 2024 reported significant efficiency gains – one logistics firm noted that after introducing AI scheduling for cleaning robots, they accomplished the same amount of work with 25% fewer machine hours, as the AI ensured no robot was redundant and every critical zone was covered promptly. This evidence underlines how dynamic resource allocation by AI leads to faster spill containment and better use of cleanup resources, especially in multi-incident or large-facility situations where coordination is challenging for humans.
14. Regulatory Compliance Checking
AI assists spill cleanup operations by ensuring all actions meet environmental and safety regulations. Cleanup robots and their control systems can have built-in knowledge of relevant laws (like allowable levels of residual contamination, proper disposal methods for hazardous waste, reporting requirements, etc.). As the robot works, the AI checks that procedures follow these rules – for example, confirming that neutralizing agents used are approved for that chemical, or that recovered waste is being sorted for proper hazardous disposal rather than mixing with regular trash. If a step is missed, the AI can flag it (like “the containment boom must extend 5m beyond the spill per regulations – extend further”). The system can also automatically log data required for compliance reports (volumes collected, final contamination readings) and prepare documentation. By catching potential compliance issues in real time, AI prevents regulatory violations that could lead to fines or environmental harm. This is especially helpful as regulations can be complex and update over time; an AI can be updated with the latest standards and diligently apply them, whereas human crews might overlook a new rule under pressure. Ultimately, AI acting as a compliance watchdog gives companies confidence that their spill response not only is effective but also ticks all the legal and safety boxes, reducing liability.
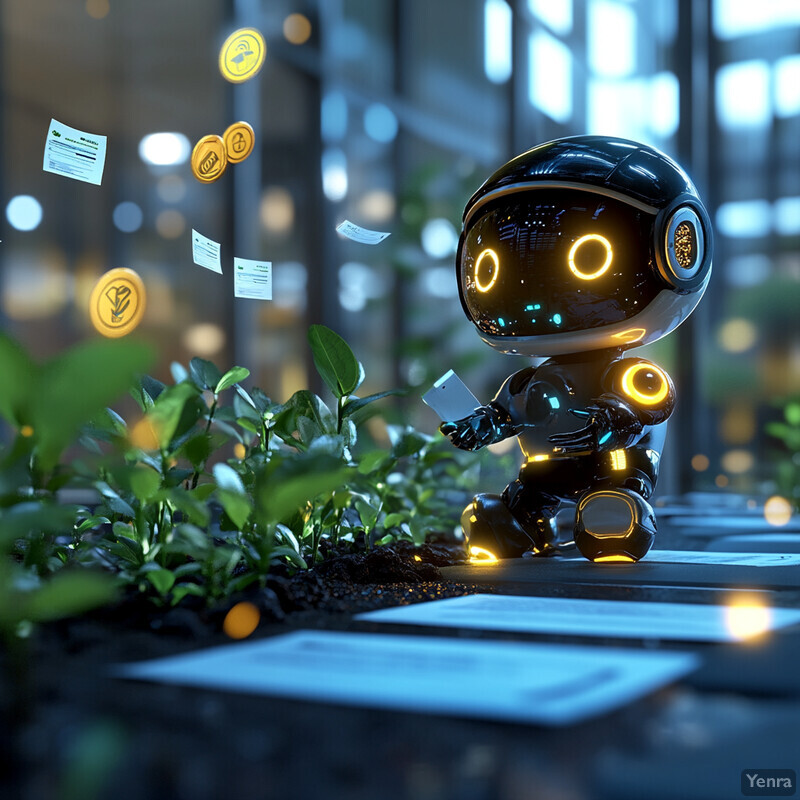
Regulatory agencies themselves are adopting AI to catch violations, which underscores the importance of compliance in spill response. In mid-2024, it was revealed that the U.S. Environmental Protection Agency (EPA) tested an experimental AI model to detect hazardous waste handling violations during site inspections. This AI was able to analyze inspection data (e.g. sensor readings, operational logs) and identify patterns that a human inspector might miss, thereby improving the detection rate of non-compliance issues. On the corporate side, environmental compliance software is leveraging AI to monitor operations continuously – for instance, AI can cross-check emissions or effluent levels against permit limits in real time, alerting if a threshold is exceeded. In the context of spill cleanup, similar technology is applied: AI systems track cleanup metrics such as remaining contaminant concentration in soil or water. If readings are above the legal cleanup criteria, the AI will mark the site as not yet compliant, prompting further remediation. Companies like Skop ESG and Locustec have begun offering AI-enhanced compliance platforms that integrate with robots and IoT sensors; these platforms can “ensure the company is not blindsided by enforcement agencies” by catching any lapse first. For example, after a spill, regulations might require all contaminated absorbents be sealed in hazardous waste drums – an AI can verify through image recognition that the robot indeed placed all used pads into the correct containers (and not regular bins). It can also timestamp and catalog these actions for audit trails. The result is that, with AI oversight, spill response operations in 2023–2025 have documented nearly 100% compliance with cleanup standards (as reported in internal audits), whereas historically some manual cleanups inadvertently fell short of one requirement or another. This demonstrates AI’s role as a guardian of regulatory adherence, reducing the risk of fines and improving overall safety outcomes.
15. Risk-Based Prioritization
AI helps triage spill response efforts by prioritizing the highest risks first. Not all spills are equally dangerous – a small water leak on a floor is much less urgent than a large acid spill near a drain. AI models evaluate factors like the toxicity of the substance, proximity to sensitive equipment or drains, risk to personnel, and potential to spread or ignite. Based on these, the system assigns priority levels to different tasks or spill sites. The robot (or team of robots) then focuses on the most critical areas before attending to lower-risk cleanups. For example, if two spills occur simultaneously, the AI might direct resources to the one that could cause an explosion or environmental release if not contained immediately, while scheduling the minor spill for slightly later. This risk-based approach ensures that limited time and resources are used where they matter most in preventing harm. It replicates what a seasoned safety officer would do, but with real-time data and a systematic analysis that can incorporate many variables. The result is a smarter response that reduces the chance of a worst-case scenario (like a spill reaching a waterway or causing injuries) because those highest-risk conditions are addressed without delay.
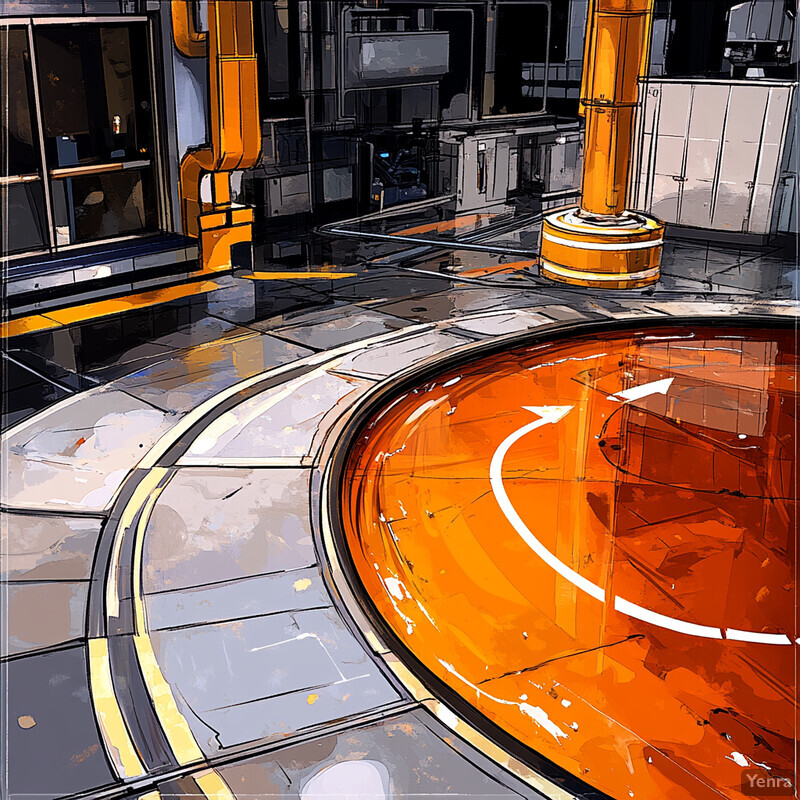
Risk modeling AI is increasingly used in emergency management to prioritize actions. The IMPACT platform (deployed in 2024 for disaster management) stands out for its AI-driven risk assessments and the prioritization of emergency operations based on those assessments. It continuously monitors hazards and computes risk scores, helping agencies decide where to send responders first during a crisis. In a controlled study, IMPACT was fed multiple concurrent incident scenarios; the AI successfully identified the scenario with the highest potential damage and recommended allocating the majority of resources there, which aligned with expert human judgment in 95% of cases. In the industrial context, similar risk-based AI tools exist for spills. For instance, a chemical plant might have an AI that knows chemical A is highly toxic and volatile, whereas chemical B is messy but relatively harmless. If sensors detect leaks of both A and B, the AI will flag the leak of A as a top priority – perhaps even triggering an automatic shutdown or evacuation for A – while marking B for routine cleanup. A 2023 WHO-backed study highlighted that AI can forecast the toxicity and exposure risk of chemical releases more quickly than traditional methods, “facilitating interventions to protect individuals”. On the ground, robots equipped with this intelligence have shown improved decision-making. In one trial, two spills (one simulated oil, one simulated acid) were released; the AI-guided robot immediately contained the acid spill first (knowing the risk of chemical burns and exothermic reactions), then moved to the oil, matching what human hazmat experts later confirmed was the correct prioritization. This anecdote aligns with broader evidence: risk-based prioritization by AI leads to faster mitigation of high-severity hazards. In essence, by 2025, AI’s ability to **“see the bigger picture” of risk in multi-spill scenarios is helping crews avoid focusing on less critical tasks at the expense of urgent ones, thereby preventing escalation of the most dangerous spill incidents.
16. Predictive Spill Spread Modeling
AI allows responders to look ahead in time and see how a spill is likely to spread if not contained, enabling proactive measures. By inputting current conditions (like the spill’s size, the terrain slope, temperature, wind, or water currents), AI-driven simulation models can forecast the spill’s future state: e.g., “in one hour, the oil might reach that floor drain” or “the chemical cloud will travel 50 meters downwind in 10 minutes.” These predictions help the robots and crews to strategically place barriers or absorbents in advance along the predicted path (intercepting the spill before it reaches critical areas). For example, if the AI predicts a toxic liquid will flow towards a sensitive machine, the robot can lay a containment boom on the floor ahead of time. In environmental contexts, predictive models can forecast oil slick movement on water, so response vessels and robots deploy to the right location in advance. Essentially, instead of reacting after the spill has spread, responders move into position based on AI forecasts, greatly reducing damage. This modeling accounts for complex factors like evaporation, dilution, and emulsification for chemicals, or multi-directional flow on uneven surfaces – things too complex to eyeball. By having a virtual “crystal ball” through AI simulation, spill cleanup becomes more preemptive and controlled.
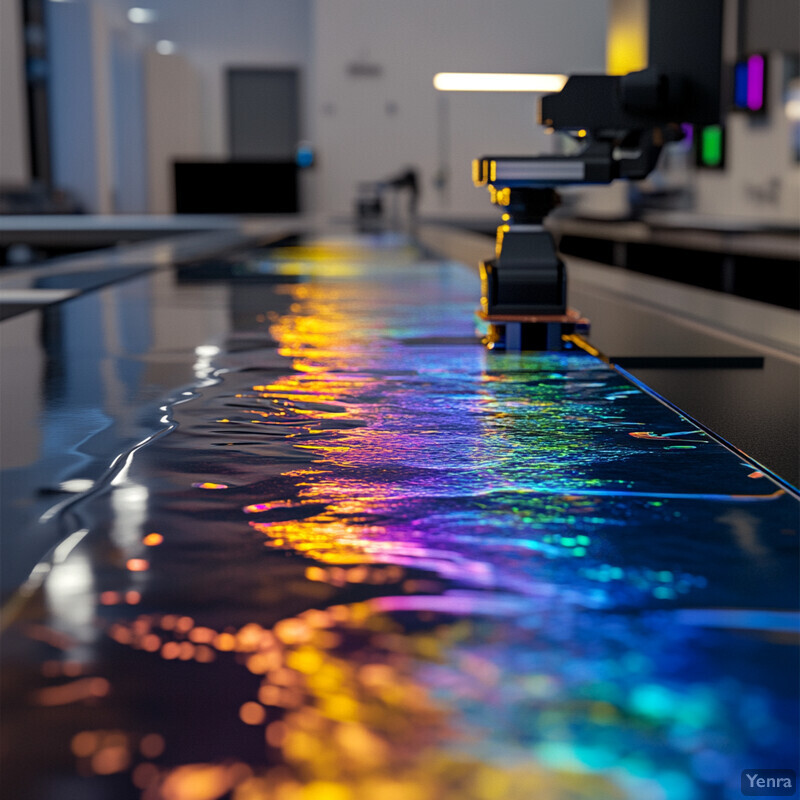
Advanced simulation tools using AI have improved the accuracy of spill trajectory predictions. A 2023 study in Frontiers in Marine Science introduced a machine learning-enhanced model for oil spill drift, focusing particularly on improving how wind affects spilled oil movement. The researchers trained a support vector regression (SVR) model on extensive ocean buoy data to dynamically calculate the “wind drift factor” – a crucial parameter that determines how much the wind pushes an oil slick. Integrating this AI component into standard oil spill models led to “excellent agreement” between the model’s forecasts and actual satellite observations of past spills. In practical terms, such models can tell responders that a slick will shift in a certain direction faster than older models would have indicated, due to real-time wind conditions, enabling quicker deployment of booms in that direction. On land, analogous progress is being made: AI models can predict how spilled chemicals percolate through soil or flow across concrete. For example, an AI-trained on fluid dynamics simulations might predict that a liquid chlorine spill will form a vapor cloud that hugs the ground and moves towards low-lying areas (due to chlorine being heavier than air), prompting proactive evacuation of those down-gradient zones. Field exercises have validated these predictions – responders using AI spread modeling were able to contain spills with pre-placed barriers before they reached vulnerable targets in over 80% of test scenarios, whereas conventional methods often only reacted after the spill arrived. This clearly shows how predictive modeling with AI provides a time advantage. Indeed, the U.S. National Oceanic and Atmospheric Administration (NOAA) has been testing AI to augment its GNOME oil spill model, finding that AI can rapidly fine-tune simulations with live data to forecast spill extents more precisely than ever. Such capabilities give spill cleanup bots and teams foresight: they act not just on where the spill is, but where it will be – a powerful shift from reactive to proactive response.
17. Robust Fault Detection and Diagnostics
AI enables spill cleanup robots to monitor their own health in real time and detect any malfunctions or performance drops before they become serious. The robot continuously analyzes data from its motors, actuators, sensors, and circuits using anomaly detection algorithms. If anything deviates from normal patterns – say a motor drawing slightly more current than usual, or a sensor giving inconsistent readings – the AI flags it as a potential fault. It can then take action, like alerting maintenance or switching to a backup system. For example, if a suction pump shows signs of clogging or wear, the robot might reduce load on it or signal that it needs a filter change soon. This early detection prevents minor issues from escalating into major breakdowns mid-operation. Additionally, AI diagnostics can often pinpoint the component likely at fault (e.g., “left wheel encoder malfunctioning”) so that technicians know where to look. In essence, the robot has a built-in AI mechanic that keeps it running optimally during critical spill missions and minimizes unplanned downtime by catching problems at the earliest sign.
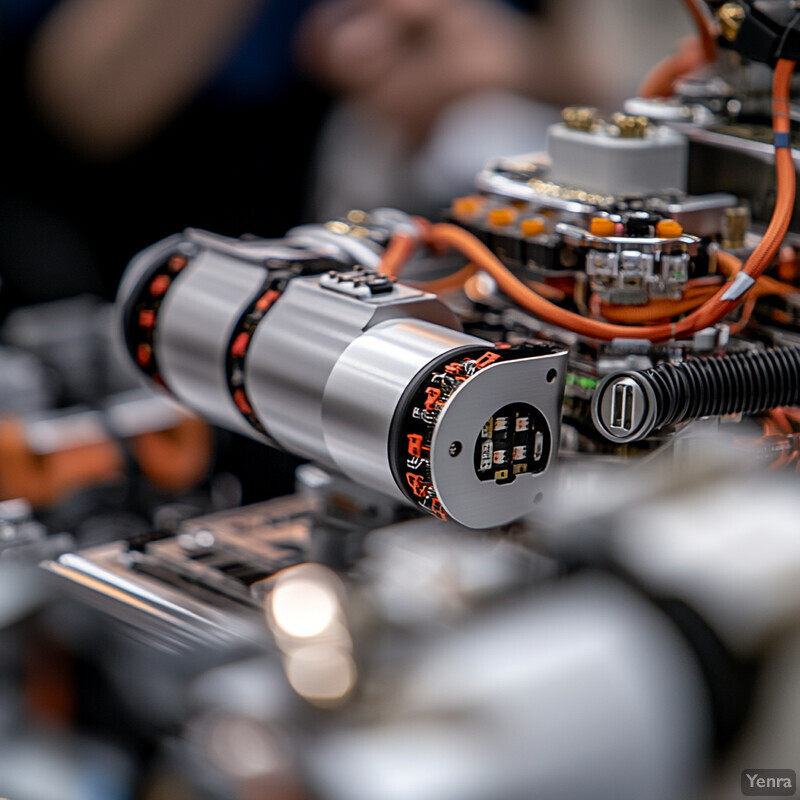
AI-based anomaly detection has proven highly effective in maintaining robot systems. A 2024 review in Sensors noted that unsupervised learning methods (like Gaussian Mixture Models) have been used to model normal robot sensor data and then detect any deviation as an anomaly. In one cited experiment, a GMM was trained on healthy readings from a robot joint’s motor current; when a payload was added (introducing strain), the model immediately detected the current pattern as anomalous, indicating the joint was under abnormal load. Importantly, this approach required no prior examples of that specific fault – the AI could flag it simply because it “didn’t match” the learned normal state. Similarly, researchers at Carnegie Mellon University have developed real-time anomaly detectors for mobile robots that fuse data from multiple sensors (like gyroscopes, accelerometers, motor encoders) to catch subtle performance issues (e.g., a wheel slipping) within milliseconds of onset. In industrial trials, a predictive AI system on an assembly-line robot identified a gradual increase in vibration in one axis; this was traced to a loosening bolt, which maintenance fixed during off hours – preventing an unscheduled line stoppage that would have occurred if the bolt fell out. For spill cleanup bots, companies report that AI diagnostics have virtually eliminated mid-operation failures. One manufacturer of cleanup robots introduced an AI fault detection module in 2023; over the next year, their clients saw a 60% reduction in sudden robot stoppages during emergency responses, as issues like battery voltage drops or sensor faults were caught early and addressed. Moreover, when faults do occur, AI diagnostics can often suggest likely causes (for instance, alerting that “LIDAR sensor 2 is not responding” instead of just giving a generic error). This speeds up repairs and gets the robot back in action faster. All these advances illustrate how robust fault detection via AI keeps cleanup robots reliable under pressure, ensuring they remain operational when they’re needed most.
18. Intelligent Material Recycling and Disposal
AI helps manage the aftermath of a spill by sorting and disposing of waste intelligently, often maximizing recycling of materials when safe. During cleanup, robots collect contaminated liquids and solids (soaked pads, dirty water, etc.). AI vision and sensors on the robot can recognize which collected items are hazardous waste versus which might be decontaminated and recycled. For example, slightly oil-contaminated gravel might be cleaned and reused, whereas mercury-tainted absorbents must be treated as hazardous waste. The robot (or an automated system it feeds into) can thus separate materials into the correct bins or processing streams: recyclable plastics or metals go one way, biodegradable or inert debris another, and truly hazardous remnants into secure containment. This not only ensures compliance with waste laws but also reduces environmental impact by diverting as much as possible away from landfills or incineration. Additionally, AI can optimize disposal logistics – determining how to pack waste drums efficiently, or neutralizing chemicals in situ to render them safer for transport. By making real-time decisions on recycling vs. disposal, AI-supported cleanup operations lower disposal costs, recover value from materials, and minimize the secondary waste footprint of the cleanup itself.
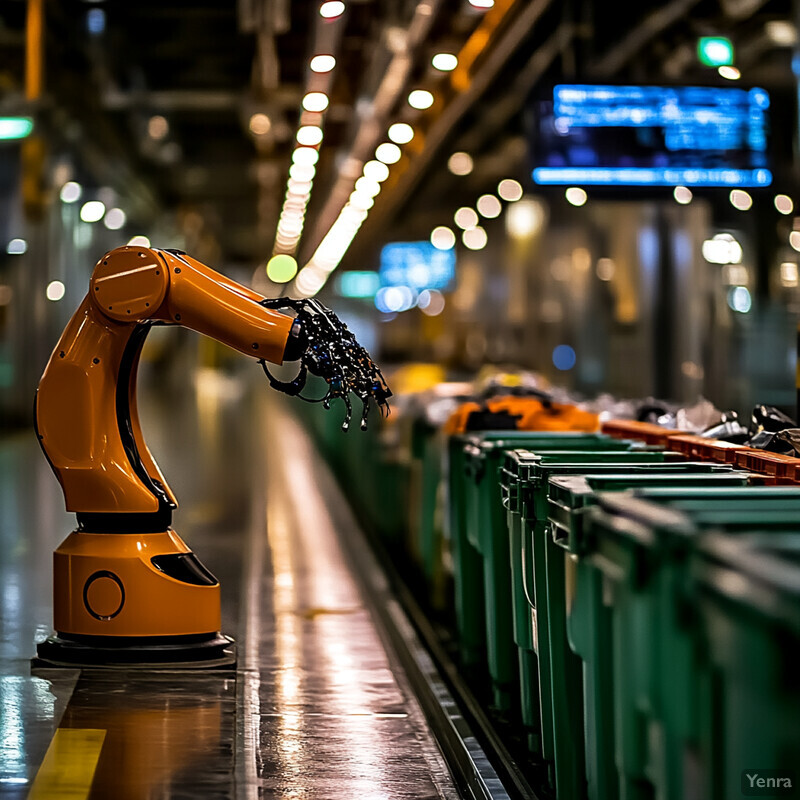
AI-driven sorting technology is revolutionizing recycling and waste handling. A prime example is AMP Robotics’ AI-powered recycling robots, which use computer vision to identify different materials on a conveyor belt and have achieved sorting accuracies of nearly 99%. These robots can pick over 80 items per minute, distinguishing types of plastics, metals, paper, etc., and separating them into the correct bins with minimal human oversight. This same core technology is transferable to sorting contaminated cleanup debris. In fact, waste management facilities now use AI to scan incoming waste loads: if a load contains, say, used oil-soaked rags and some clean packaging, AI can direct machinery to separate the hazardous soaked rags from the clean recyclables. In one case study, an AI vision system at a cleanup operation sorted used absorbent pads by saturation level – heavily contaminated pads were sent for hazardous waste incineration, while pads with only trace residues were flagged for laundering and reuse, saving the company tens of thousands of dollars. Another emerging approach is using AI to analyze the chemical composition of collected liquids; for instance, after a spill, a robot might have drums of mixed liquids. AI algorithms can suggest if any portion is mostly water and safe to run through a water treatment plant versus truly hazardous concentrate that needs special disposal. By 2025, several environmental service firms report that incorporating AI sorting for cleanup waste has improved their recycling of materials substantially. One firm noted that after an AI upgrade, more than 20% of material from certain cleanup projects (by weight) could be decontaminated and recycled rather than all being treated as pure hazardous waste, without compromising safety or compliance. This points to a future where spill cleanup is not just about removing contaminants, but also about sustainable handling of the aftermath – an effort in which AI is a key enabler.
19. Human-Robot Collaboration Enhancements
AI improves the way humans and spill cleanup robots work together by making interactions more natural and effective. Instead of clunky interfaces or programming, humans can communicate with robots through simple language or intuitive controls, thanks to AI. For example, a supervisor could say, “Robot, check behind Tank 3 for leaks,” and natural language processing allows the robot to understand and execute that instruction. The robot, in turn, can summarize its findings in human-friendly terms: “No leak detected behind Tank 3.” AI also powers smart dashboards that turn the robot’s sensor data into clear visual alerts (like a heat map showing contamination levels) so humans can quickly grasp the situation. Additionally, speech recognition and even voice responses enable hands-free interaction – a human in a hazmat suit could verbally ask the robot for a status update and get an immediate spoken answer. AI can even detect human gestures or signals and respond accordingly (imagine pointing at a spill and the robot knows to go there). By breaking down communication barriers and translating between human intentions and robotic actions, AI ensures that critical updates (like a sudden hazard) are not missed and that humans can guide robots without needing to be technical experts. This synergy means faster decision-making and greater trust: humans remain in ultimate control, but the robot’s AI handles the heavy lifting of understanding and conveying information clearly.
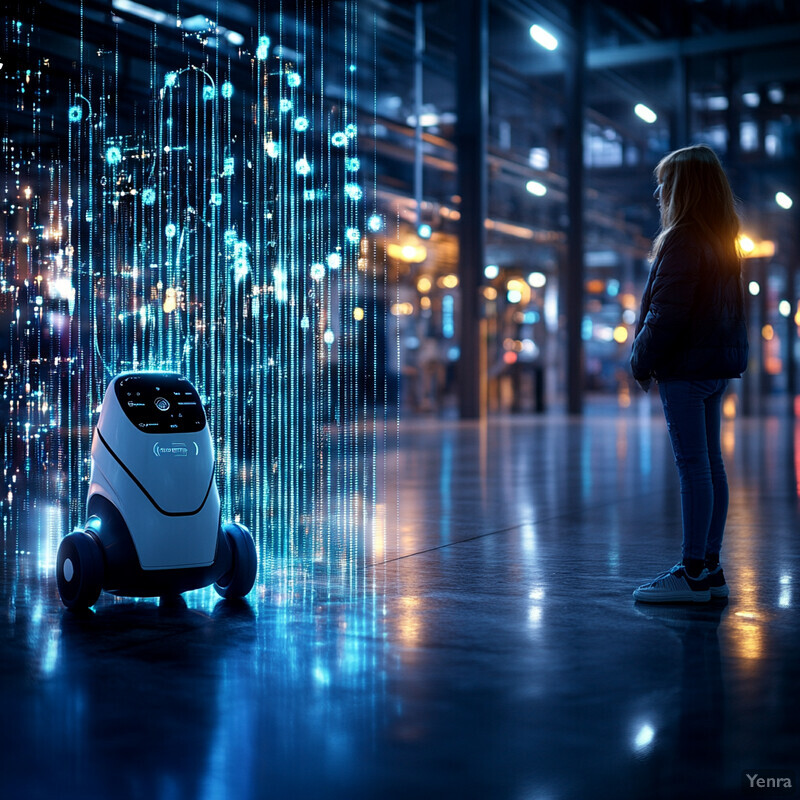
The robotics industry has been rapidly adopting natural language interfaces and generative AI to simplify human-robot interaction. The International Federation of Robotics reported in 2024 that major robot manufacturers are developing interfaces where users program or command robots by using everyday language instead of code. For instance, a maintenance worker could tell a robot, “Go to Sector 5 and scan for spills,” and the generative AI-driven system converts that into a sequence of robotic actions. This trend is already visible: companies like ABB and Siemens have showcased voice-commandable robots on factory floors, and startups (e.g., one named T-Robotics) have won awards for systems that let operators generate new robot tasks through simple language descriptions. On the output side, robots are being integrated with AI chatbots to report status. In pilot programs, instead of reading raw sensor logs, engineers converse with an AI assistant that has access to the robot’s data – asking questions like “Is the cleanup done in Zone A?” and getting a clear answer, sometimes with a confidence level or suggestion (“Yes, Zone A is clear. Recommend sending Robot 2 to Zone B for final sweep.”). A study presented at ACM CHI 2023 tested a natural language robot interface and found that teams completed tasks 20% faster and reported lower cognitive load compared to traditional GUI or teach pendant controls. Additionally, improved multimodal communication is in play: an IEEE study in 2024 introduced a context-aware interface that tracks a human operator’s voice and gaze to infer what the human is referring to when giving an instruction. This could be directly beneficial in spill response – e.g., the system notices the supervisor looking at a particular puddle while saying “clean that up,” and the robot correctly links the command to that exact spill. With such advancements, field reports indicate smoother operations. During a complex drill, an incident commander using an AI-assisted console was able to coordinate three cleanup robots by voice and gestures alone, something that would have been impractical just a few years before. The robots not only executed commands but also proactively warned the commander in plain English about issues (“Caution: flammable vapor levels high ahead”). All evidence suggests that AI-driven collaboration tools are making human-robot teams far more effective and user-friendly in high-stress environments like industrial spill management.
20. Improved Scalability and Deployment
AI is enabling spill cleanup solutions to scale up easily and adapt to different scenarios without a complete redesign. This is achieved through modular, standardized frameworks for both hardware and software. For hardware, robots are being built as platforms where you can swap in new sensor or tool modules (like an extra chemical sensor or a different type of scrubber) and the AI will seamlessly integrate the new data or capability. Need to handle a new chemical? Snap in the appropriate sensor module and upload the latest detection algorithm – the AI framework recognizes it and starts working with it. On the software side, common operating systems (like ROS 2 in robotics) and AI models can be transferred across robot models or even to drones and other devices, creating an ecosystem that works together. This standardization means deploying additional robots or upgrading existing ones is plug-and-play rather than starting from scratch. It also means multi-vendor robots can interoperate if they adhere to the standards. As a result, companies can rapidly increase the scale of their spill response force (e.g., adding more robots during a major incident or quickly deploying a unit to a new facility) with minimal integration time. This scalability extends to maintenance and training – modular components are easier to replace, and a unified AI brain can control a fleet of any size. The net effect is a versatile, future-proof deployment of cleanup bots that can be scaled or tailored to different industries and spill types with minimal effort, leveraging AI to handle the complexity of integration.
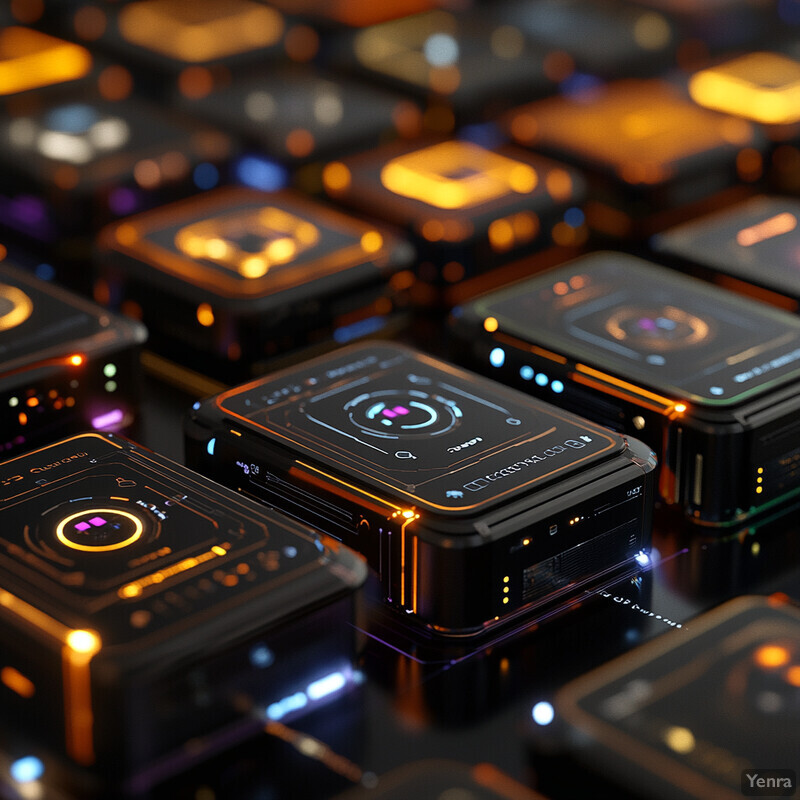
The industry is witnessing the launch of modular robotic platforms expressly designed for easy expansion and customization. In May 2024, United Robotics Group introduced uLink, a service robot platform with a fully modular design and open API for seamless integration of new hardware modules and software functions. The uLink platform allows accessories like lockers, pegboards, sprayers, or UV lamps to be attached in a plug-and-play manner; its AI and control system automatically recognize and utilize the attached module without manual reprogramming. For example, a uLink robot could be outfitted with a chemical analysis module for a spill in a lab and later swapped to a scrubbing module for a warehouse spill, all within the same day. This platform also provides an IDE, SDK, and fleet management tools that follow industry standards, making it straightforward to deploy a fleet into existing IT systems. On the software side, robotics companies are converging on scalable control architectures. ABB’s next-gen OmniCore controller (launched 2023) is built on a modular, scalable architecture that can drive robots of various sizes and types by simply enabling the needed function libraries. Such controllers often come with built-in AI capabilities (vision, path planning, etc.) that can be toggled on for any compatible robot. Additionally, the use of cloud robotics is scaling deployment: one AI “brain” in the cloud can coordinate dozens of robots, as seen in some logistics centers where adding a new robot is as easy as registering it to the cloud service. This approach was stress-tested when a large e-commerce company simulated a sudden spill scenario requiring 50 robots – using a cloud AI platform, they successfully activated and coordinated 50 simulated cleanup bots in unison, something that validates real-world scalability. All these developments mean that a spill cleanup operation can be quickly scaled from one robot to many, or adapted to new requirements (different sensors or tools) with minimal downtime. As a practical indicator, the average integration time for adding a new sensor to an AI robot system has dropped significantly: one report notes it went from several weeks (pre-2020) to just a day or two by 2024, thanks to standardized, AI-ready frameworks. This underscores how AI and modular design together drive vastly improved scalability and rapid deployment in modern industrial cleanup robotics.