1. Adaptive Difficulty Modulation
AI can dynamically adjust the complexity and intensity of simulation scenarios based on a learner’s performance and progress, ensuring that each trainee is consistently challenged at the appropriate level.
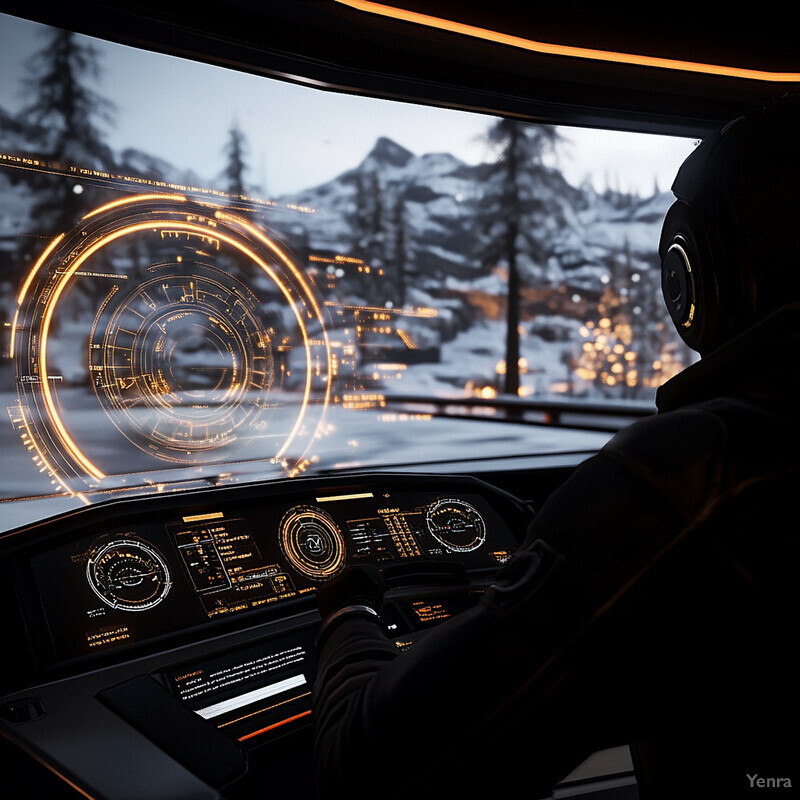
AI-driven simulations continually assess a trainee’s skill level, response time, and decision-making patterns to adjust the complexity of scenarios in real-time. For instance, if a learner is consistently excelling, the system might introduce more variables, higher stakes, or faster-paced challenges. Conversely, if the trainee struggles, the simulation can lower certain thresholds or simplify specific tasks to maintain a zone of optimal difficulty. This approach ensures that learners remain engaged, neither overwhelmed by tasks that are too difficult nor disengaged from tasks that are too easy. Over time, adaptive difficulty modulation fosters steady improvement, making training more efficient and enjoyable.
2. Personalized Learning Paths
Intelligent algorithms can tailor training modules to individual needs, focusing on areas where the trainee struggles and streamlining content for areas they have already mastered.
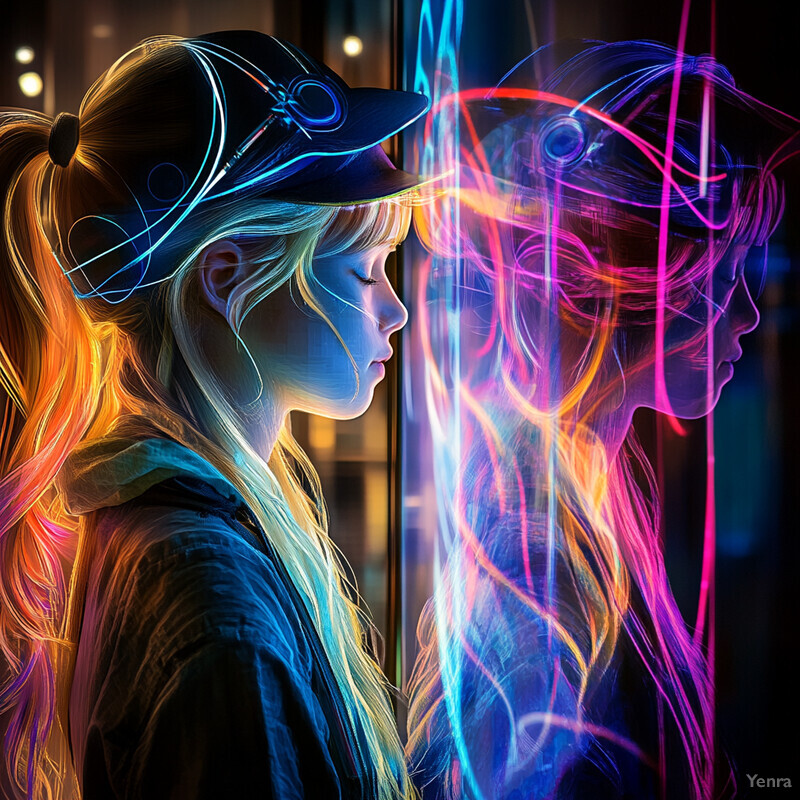
AI can analyze a trainee’s previous performance and skill gaps to curate a unique training trajectory. Rather than following a one-size-fits-all curriculum, learners receive tasks, exercises, and challenges that specifically address their weaknesses and reinforce their strengths. For example, if a medical trainee demonstrates a strong grasp of diagnostic procedures but struggles with patient communication, the simulation can prioritize scenarios emphasizing bedside manner. This focused approach saves time and resources by skipping content the learner has mastered and diving deeper into areas that need attention, leading to higher-quality skill acquisition.
3. Contextualized Feedback in Real-Time
Rather than generic end-of-session critiques, AI-powered simulations provide immediate, situation-specific guidance, helping trainees correct mistakes or reinforce good practices as they train.
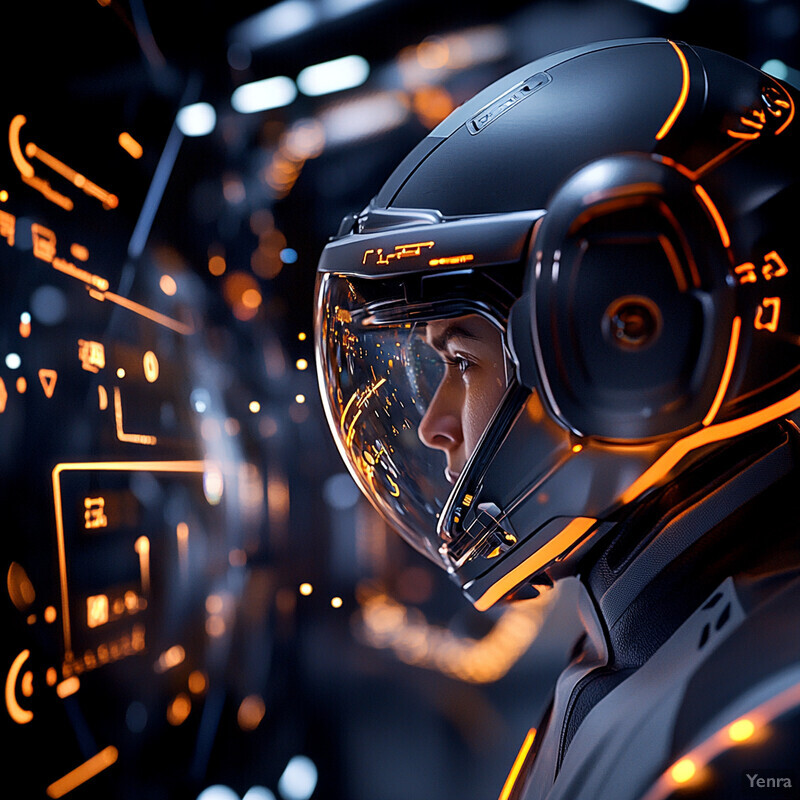
Traditional instruction often relies on post-session reviews, which means mistakes or misunderstandings may go uncorrected for extended periods. AI alters this dynamic by providing instant, situation-specific input right as the trainee performs each action. If a pilot trainee applies the wrong landing technique, the AI can immediately highlight the error and suggest corrective measures before bad habits form. This timely guidance accelerates learning, helping trainees internalize correct methods more quickly and reducing the time spent on repetitive practice of incorrect approaches.
4. Natural Language Understanding and Interaction
AI-driven speech and language models allow trainees to interact naturally with virtual instructors, teammates, or simulated entities, enabling more intuitive and authentic communication training.
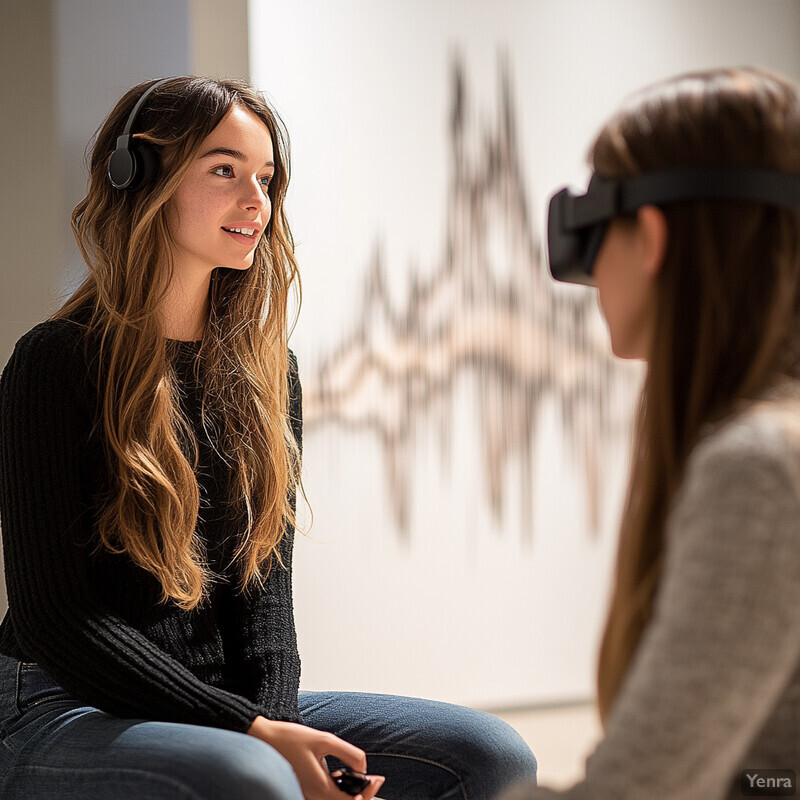
By incorporating advanced natural language processing, AI-based simulations let learners interact with virtual mentors, role-players, or team members using everyday speech. For example, a sales trainee can practice pitching a product to an AI client that understands nuanced queries, follow-up questions, and emotional cues. The system can respond with realistic dialogue, providing a dynamic environment that builds trainees’ communication skills. This creates a more authentic, human-like learning experience, allowing learners to hone their interpersonal abilities in a safe, controlled setting.
5. Predictive Performance Analytics
Advanced analytics can identify patterns and predict where trainees are likely to make errors in the future, enabling proactive adjustments or supplementary exercises before issues become ingrained.
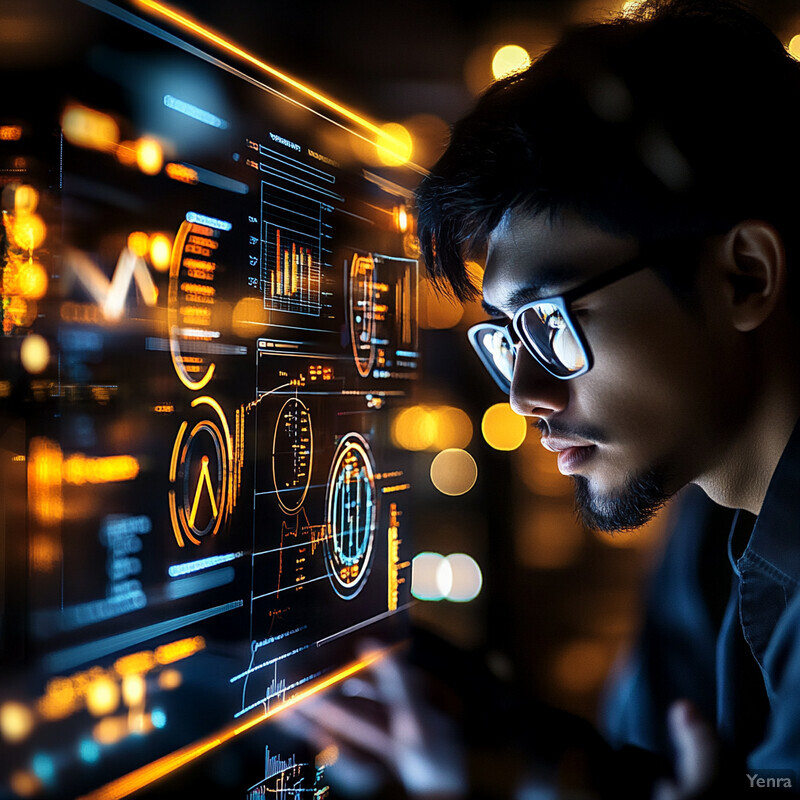
AI analytics can delve into a wealth of performance data to identify subtle patterns and forecast future outcomes. Suppose a firefighter-in-training repeatedly struggles with navigating smoke-filled rooms. Through data analysis, the AI might predict that the trainee will also struggle in complex rescue scenarios that involve poor visibility and high stress. Armed with these insights, instructors and the system itself can proactively introduce targeted challenges and supportive learning materials, ensuring that trainees confront and overcome potential weaknesses before they arise in real-world conditions.
6. Scenario and Environment Randomization
AI can generate varied, unpredictable scenarios to prevent trainees from simply memorizing solutions, ensuring they develop true problem-solving skills adaptable to changing conditions.
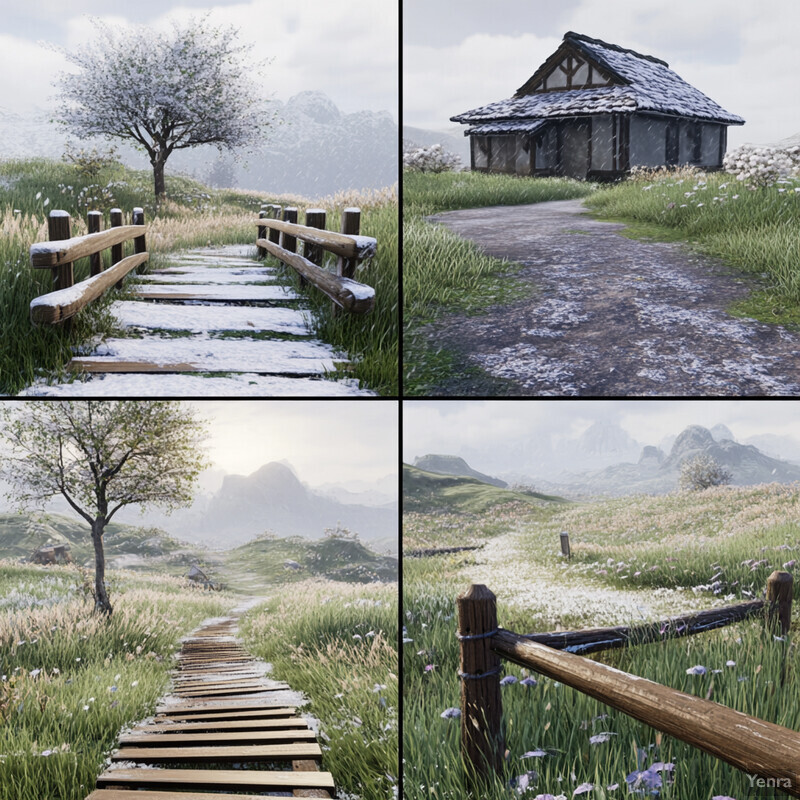
Fixed, repetitive scenarios can lead learners to memorize sequences rather than building adaptable skills. AI mitigates this risk by programmatically randomizing variables, such as weather conditions, time of day, equipment failures, or human behavior. A trainee pilot may encounter sudden engine troubles during one run, then face unexpected air traffic issues in another, without knowing what to expect beforehand. This variety fosters true problem-solving abilities and resilience, preparing trainees to adapt to a wide range of real-life situations and conditions.
7. Enhanced Virtual Role-Players and NPC Behavior
AI-enabled non-player characters can demonstrate more realistic decision-making, emotional responses, and behaviors, improving the authenticity of team-based or interpersonal training scenarios.
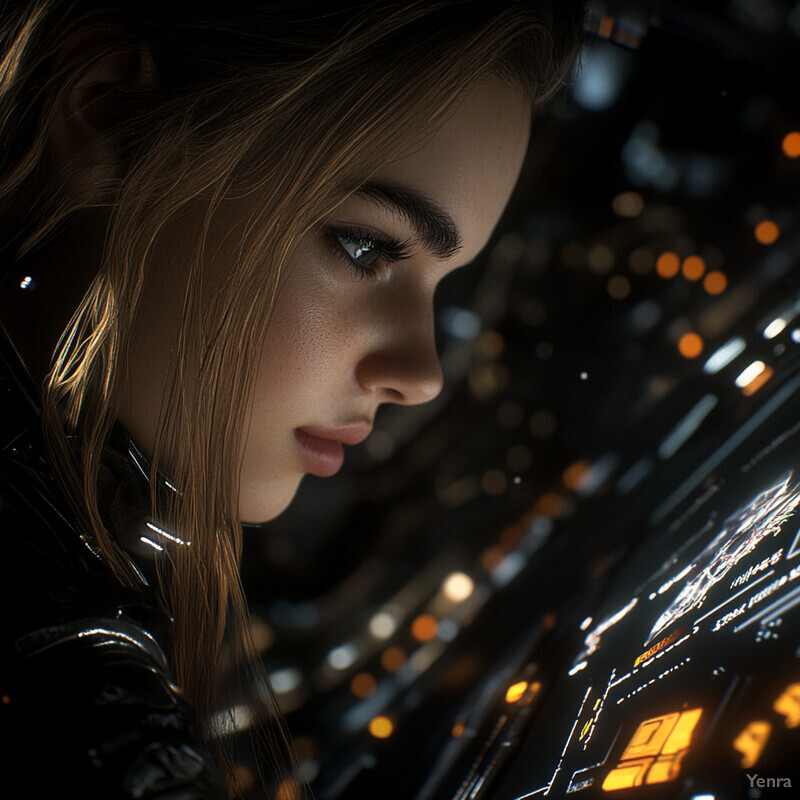
AI models can imbue non-player characters with realistic personalities, adaptive responses, and emotional intelligence. For instance, a medical trainee practicing patient interviews can engage with a virtual patient who expresses anxiety or frustration in response to certain questions. The character’s reactions evolve based on the trainee’s choices, pushing learners to develop empathy, communication techniques, and interpersonal skills. These highly believable interactions help trainees prepare for the complexity and unpredictability of real-world human encounters.
8. Continuous Skill Assessment
Instead of static quizzes or checkpoints, AI algorithms can provide continuous evaluation, using performance metrics and behavioral markers to refine understanding of a trainee’s skill level at any moment.
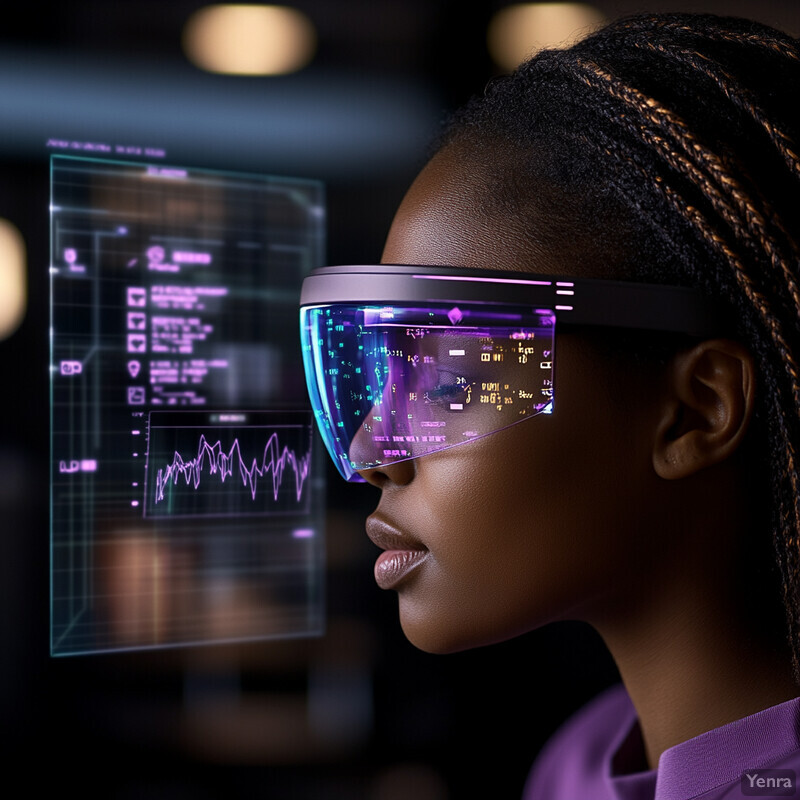
Instead of relying solely on exams or structured evaluations at the end of training sessions, AI enables continuous assessment. By analyzing every decision, movement, or piece of dialogue a trainee produces, the system maintains a running profile of their evolving competencies. For a military trainee, each tactical choice made in a simulation updates a skill map, indicating whether they need more practice in strategy, resource management, or teamwork. This ongoing feedback loop ensures that training never becomes stagnant and that progress is constantly tracked and refined.
9. Expert Knowledge Encoding
AI can assimilate insights from subject matter experts and replicate their decision-making processes, making highly specialized expertise accessible to trainees anywhere, anytime.
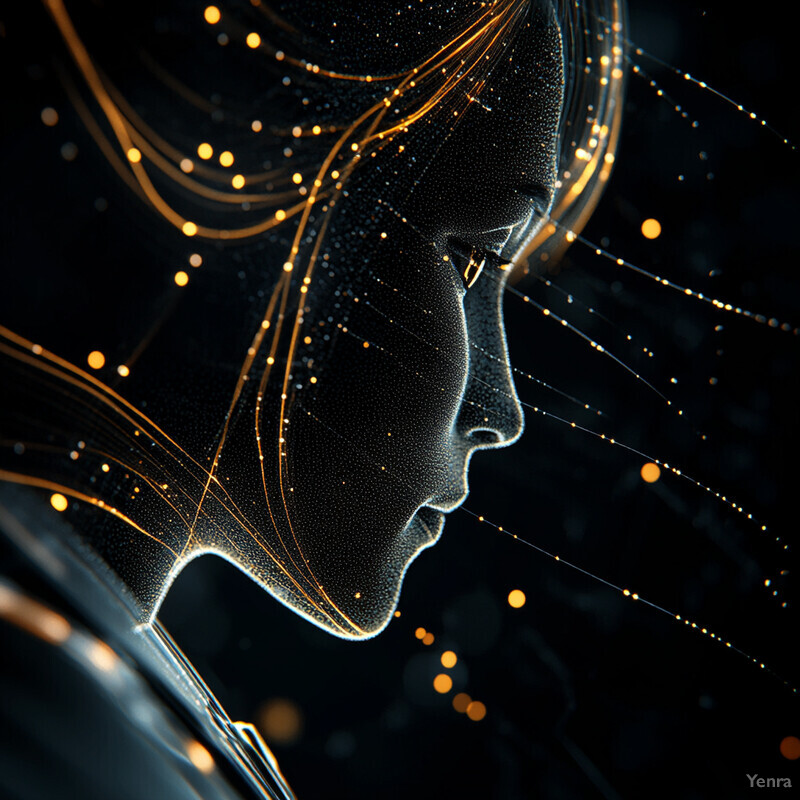
AI can simulate the decision-making processes of seasoned professionals who possess decades of experience and specialized knowledge. By embedding expert rules, strategies, and reasoning into the simulation, novices can train in scenarios that mimic working alongside a top-tier mentor. For example, a junior surgeon can “consult” the AI mid-operation simulation, receiving guidance as if a world-renowned surgeon were present. As a result, learners can tap into expert intelligence anytime, accelerating the transfer of hard-earned wisdom and best practices.
10. Emotion and Stress Level Detection
By integrating biometric sensors and emotion recognition, AI can gauge trainees’ mental states and adjust scenarios accordingly, helping learners practice coping strategies under stress.
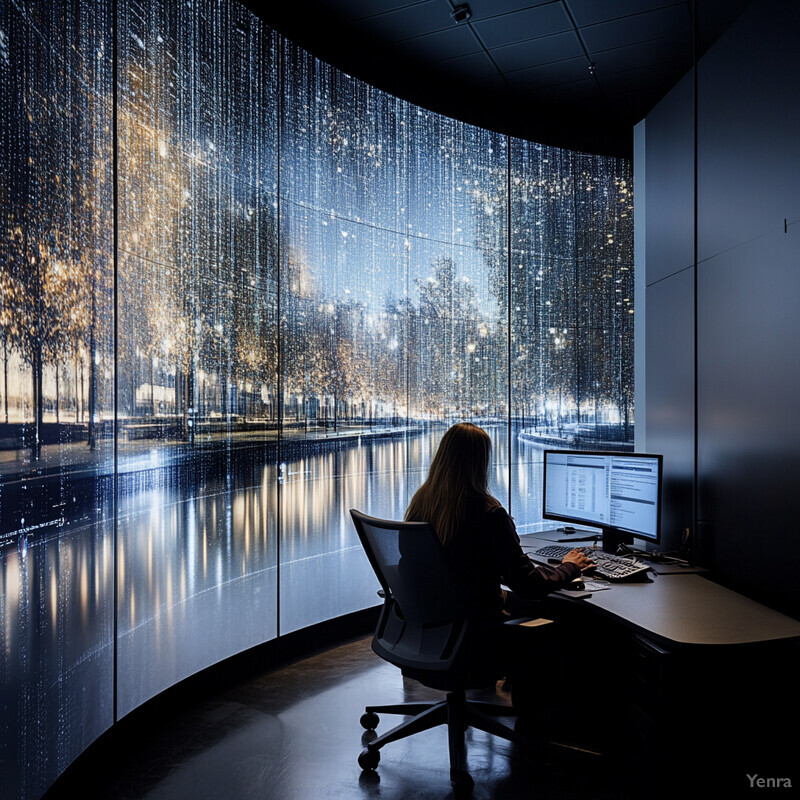
Integrating biometric sensors and advanced analytics, AI can detect subtle cues like heart rate variability, pupil dilation, and facial expressions. In a crisis management training scenario, if the simulation detects a spike in stress indicators, it may adjust the difficulty or provide gentle hints to help the trainee regroup. Conversely, if the learner seems too comfortable, the system may raise the stakes. This enables a tailored training environment that not only refines technical skills but also teaches learners how to perform optimally under pressure.
11. Contextual Cue Generation
AI can insert subtle hints or guidance at key moments, like highlighting an important detail in the environment, to keep learners on track without spoon-feeding them the solution.
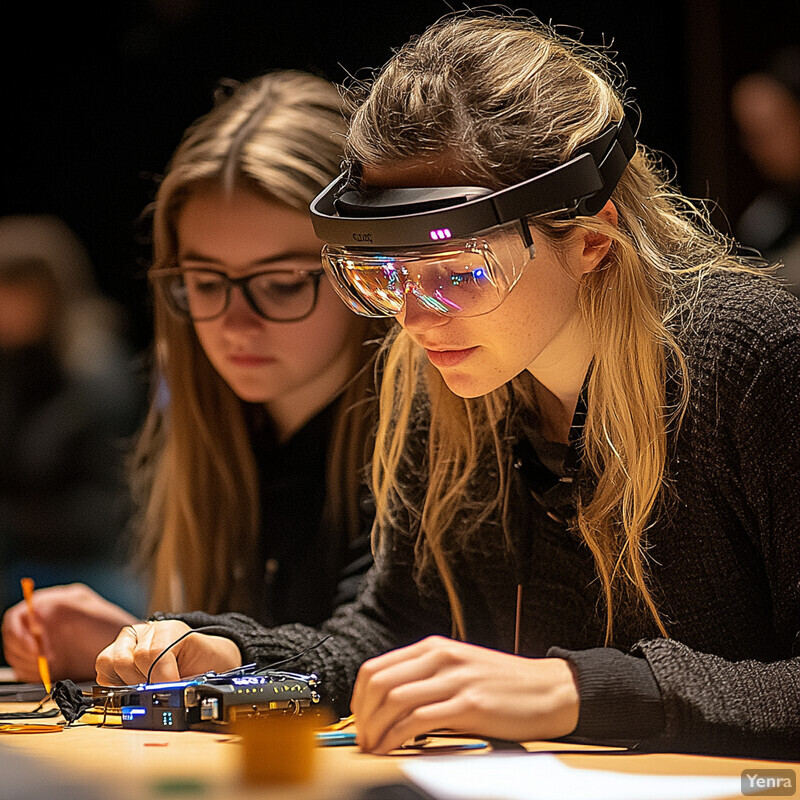
Rather than offering flat, generic hints, AI can provide subtle guidance that is sensitive to the scenario’s narrative and the trainee’s current state. If a user is practicing aircraft maintenance and misses a crucial inspection step, the simulation might highlight the overlooked component or generate a contextual clue—like a faint buzzing sound near a malfunctioning instrument. These fine-grained nudges help learners discover solutions on their own, reinforcing problem-solving skills without diluting the training intensity.
12. Adaptive Narrative Building
Complex training environments—like those for emergency response or high-stakes negotiations—benefit from AI that dynamically evolves storylines and events based on trainee actions and responses.
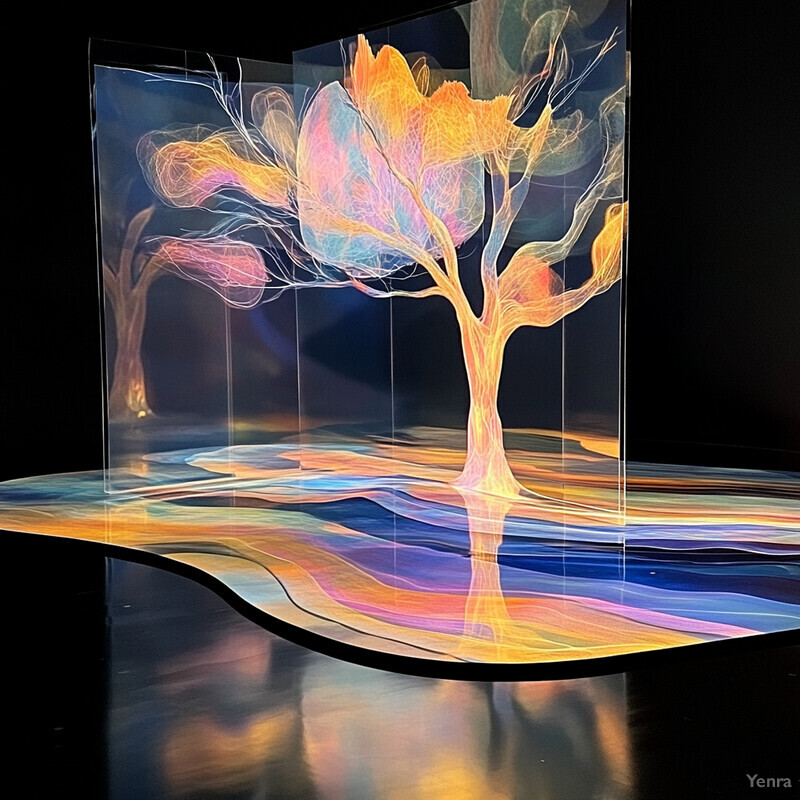
Training simulations can evolve dynamically as learners make choices, making the experience feel like an interactive story rather than a predetermined lesson. In a public safety training scenario, how a trainee responds to a hazardous materials spill might influence subsequent events, characters’ behavior, and resource availability. The simulation’s narrative adapts based on user performance, creating a more engaging and meaningful journey. Such adaptability helps trainees understand that their decisions carry weight, better preparing them for the nuanced reality of their profession.
13. Realistic AI-Driven Adversaries
In tactical and security training, AI opponents can learn and adapt tactics over time, challenging trainees to refine their strategies against unpredictable and intelligent foes.
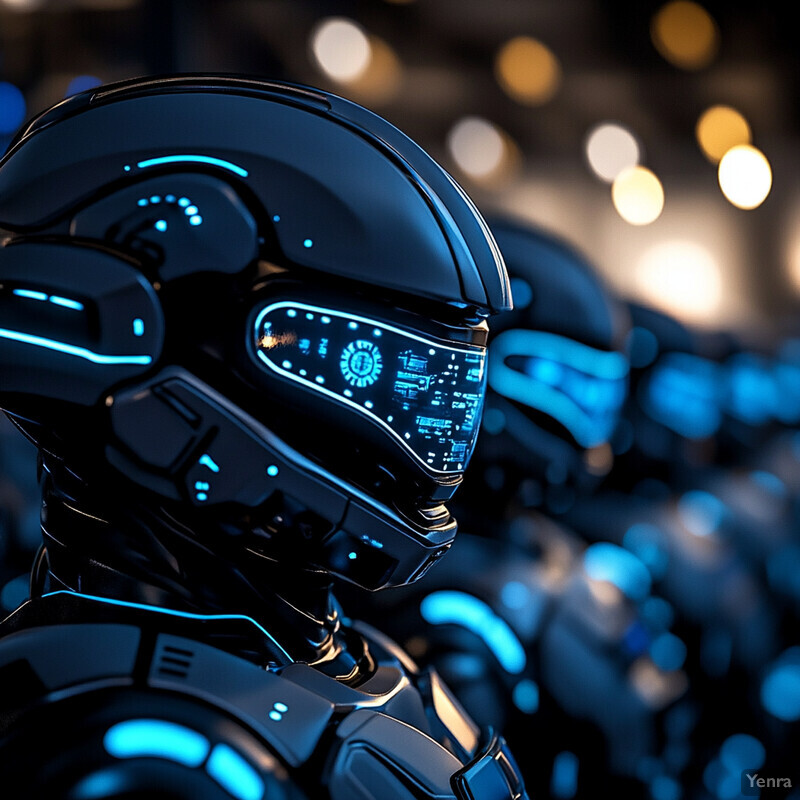
In fields such as military tactics, law enforcement, or cybersecurity, trainees must learn to outsmart intelligent adversaries. AI can simulate opponents who adapt to the trainee’s strategies, changing their tactics over time. If a trainee repeatedly uses a particular approach, the AI adversary can learn and counter it, forcing the trainee to continuously innovate. This kind of dynamic challenge ensures that learners are not only tested on their knowledge but also on their capacity to think critically and adapt quickly under pressure.
14. Scalable Group Training Simulations
AI can coordinate multiple virtual participants in team-based exercises, simulating entire squads, departments, or communities, making large-scale training scenarios more accessible and cost-effective.
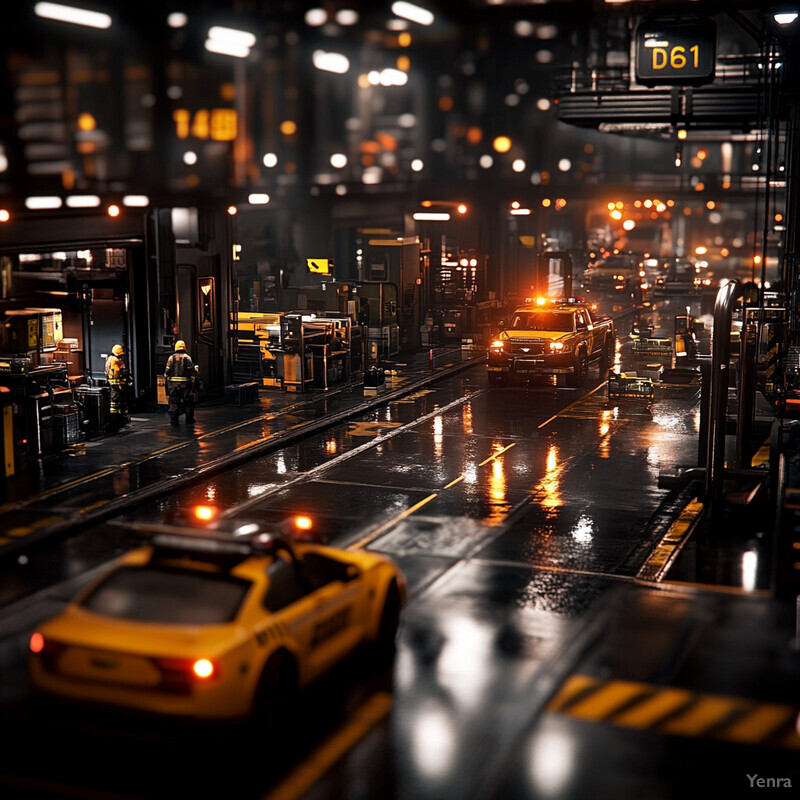
AI can manage complex multi-participant environments, coordinating large groups of trainees and virtual entities in shared simulations. For example, an emergency response exercise might involve dozens of firefighters, paramedics, and police officers, each represented by AI or human trainees. The AI can ensure realistic interdependencies—like a virtual fire spreading or simulated traffic congestion—while maintaining a coherent training narrative. This scalability is essential for organizations that need to rehearse large-scale operations without incurring massive costs or logistical hurdles.
15. Cultural and Language Adaptations
AI can modify training content to reflect different cultural norms or languages, ensuring that trainees worldwide receive realistic and context-relevant immersive experiences.
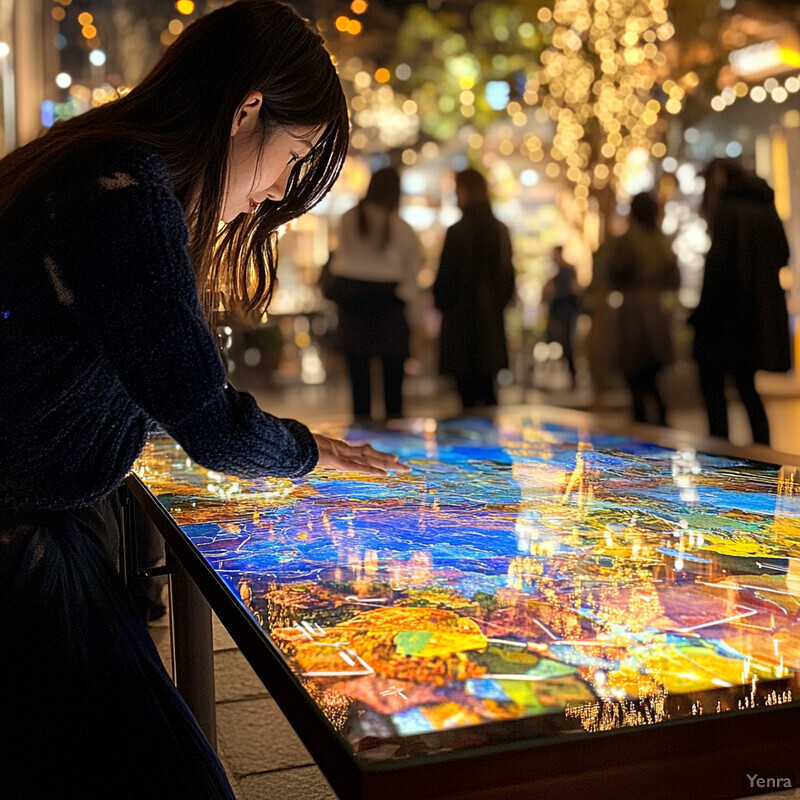
AI allows simulations to be easily localized, providing trainees worldwide with environments that reflect their cultural norms, language, and regional challenges. Healthcare simulations for international aid workers, for example, can incorporate culturally sensitive communication styles, dietary considerations, and local disease profiles. By making the training experience contextually relevant, learners are better prepared to transfer their new skills to their specific real-world environments.
16. Integration with Wearable Tech and Sensors
AI can process data from motion-capture suits, eye-tracking, or haptic feedback devices to refine the realism of simulations and ensure learners are practicing correct physical techniques.
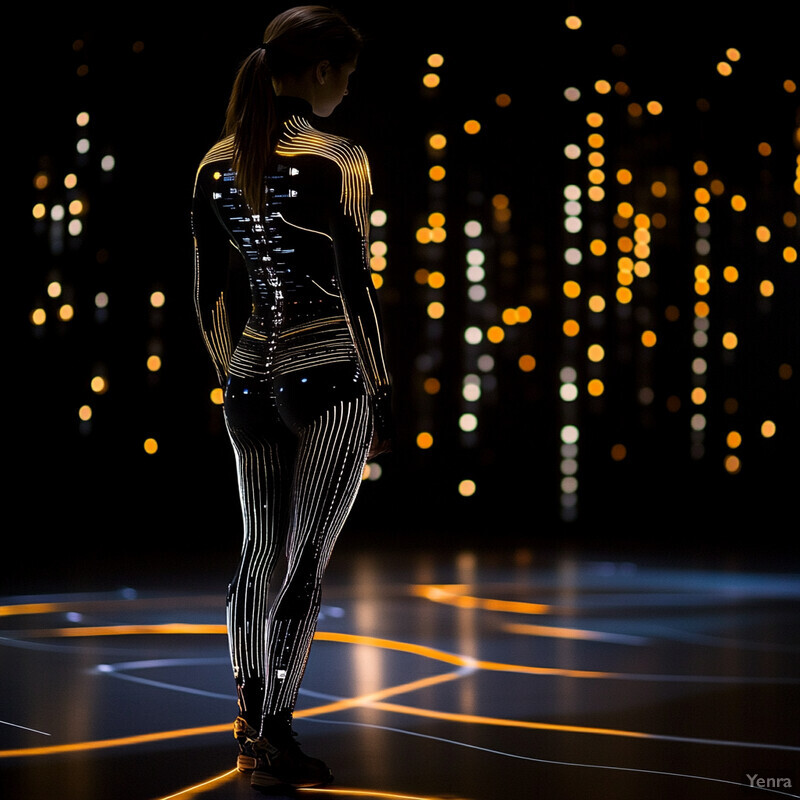
By analyzing data from motion-capture suits, haptic gloves, or eye-tracking devices, AI can provide feedback on physical techniques and cognitive processes. A manufacturing technician might wear AR glasses and gloves that track the precision of their movements in assembling a complex machine. The AI uses this data to detect inefficient gestures or fatigue, offering suggestions for improvement. Such direct, measurable feedback ensures that learners refine not just their theoretical knowledge but also the physical mastery of their craft.
17. Learning from Trainee Behaviors
AI systems can collect and analyze data from thousands of training sessions, continuously refining the simulation models and pedagogical strategies used, leading to ever-improving training outcomes.
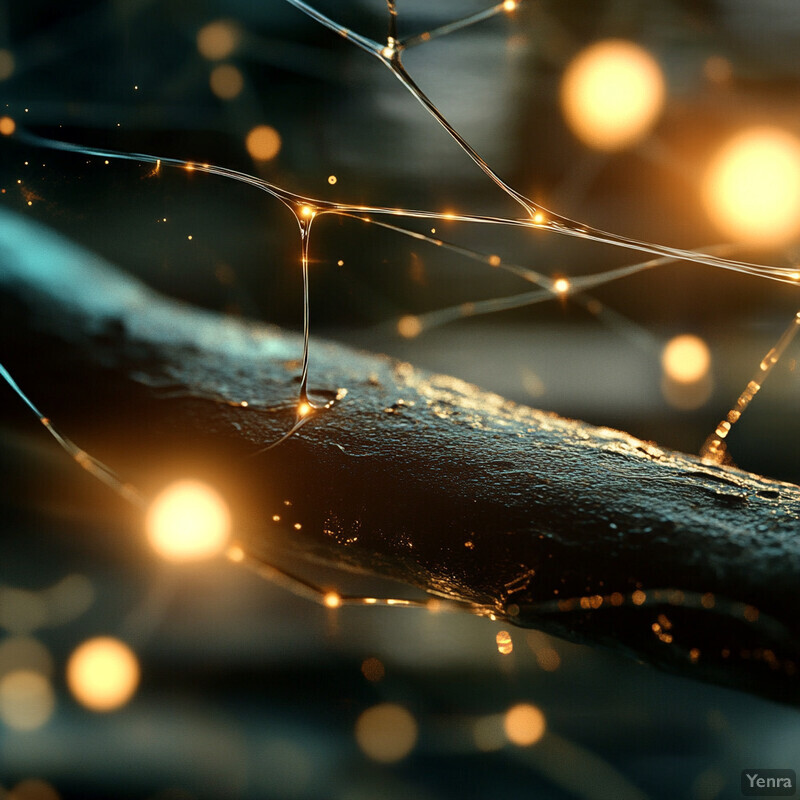
Every interaction a learner has within the simulation generates valuable data. AI can aggregate these data points from thousands of sessions to identify best practices, common pitfalls, and training elements that produce the greatest skill retention. With this iterative refinement, the simulation improves over time. As more learners train, the AI’s curriculum adjusts, scenarios become more realistic, and feedback more tailored. This virtuous cycle leads to constantly evolving and improving training systems that remain state-of-the-art.
18. Resource Optimization
By intelligently allocating computing resources—such as rendering high-detail images only when necessary—AI can maintain smooth, high-fidelity simulations on limited hardware.
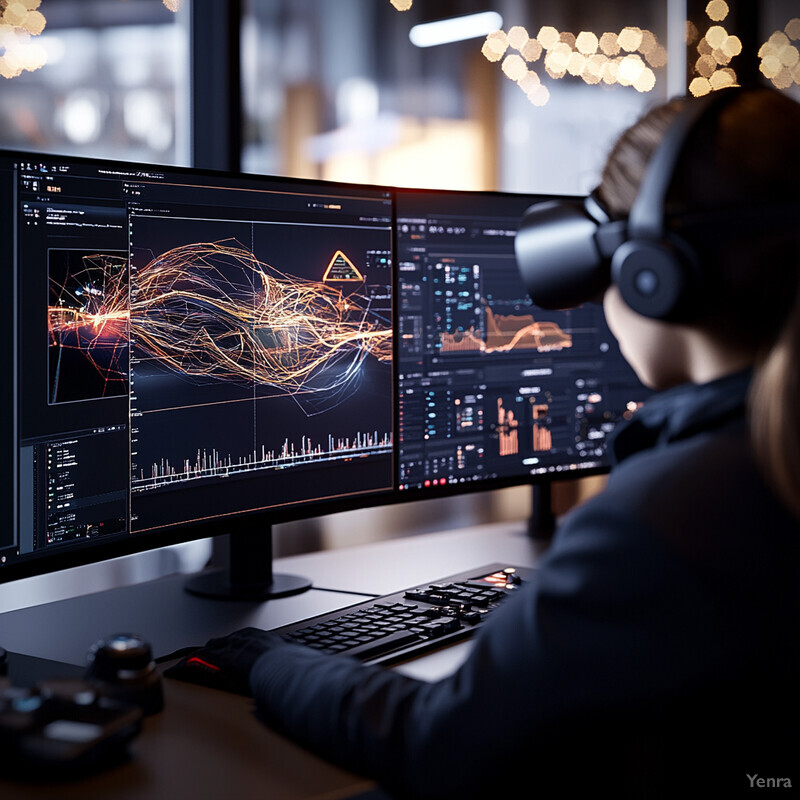
Running high-fidelity simulations, particularly in VR or AR environments, can be resource-intensive. AI can intelligently manage graphics rendering, physics calculations, and network traffic to ensure the user experiences seamless performance. For example, the system may reduce rendering complexity in peripheral vision areas or dynamically prioritize essential simulation elements. This optimization ensures that training runs smoothly, even on modest hardware, lowering barriers to access and expanding the reach of advanced immersive training tools.
19. Intelligent Tutoring Systems
AI-driven virtual tutors or coaches can engage learners in Socratic dialogues, step-by-step reasoning, and guided problem-solving, mirroring the support of a human mentor.
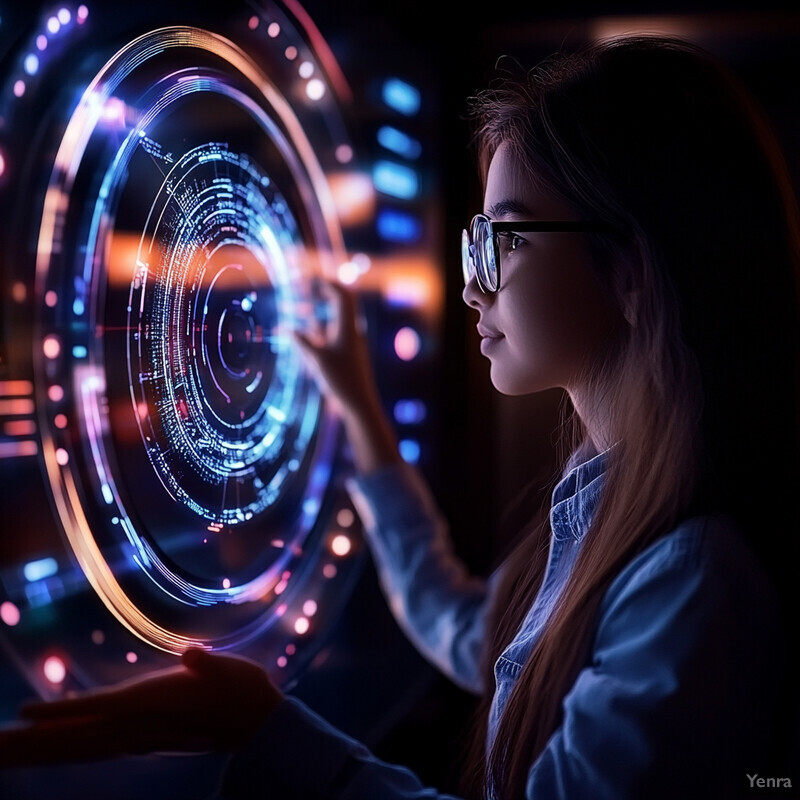
AI can serve as a knowledgeable virtual tutor, guiding trainees through complex tasks step-by-step. If a learner is studying mechanical maintenance, the tutoring system might engage them in a Socratic dialogue, prompting them to think critically rather than just providing answers. As the trainee responds, the AI adapts its questioning technique, uses metaphors, or leverages previous answers to deepen understanding. These intelligent tutors replicate the personalized instruction quality of human mentors, making expert-level guidance widely available and cost-effective.
20. Multimodal Interaction and Feedback
AI can integrate visual, auditory, and haptic cues to create a fully immersive learning environment, providing comprehensive feedback that improves both technical and soft skills.
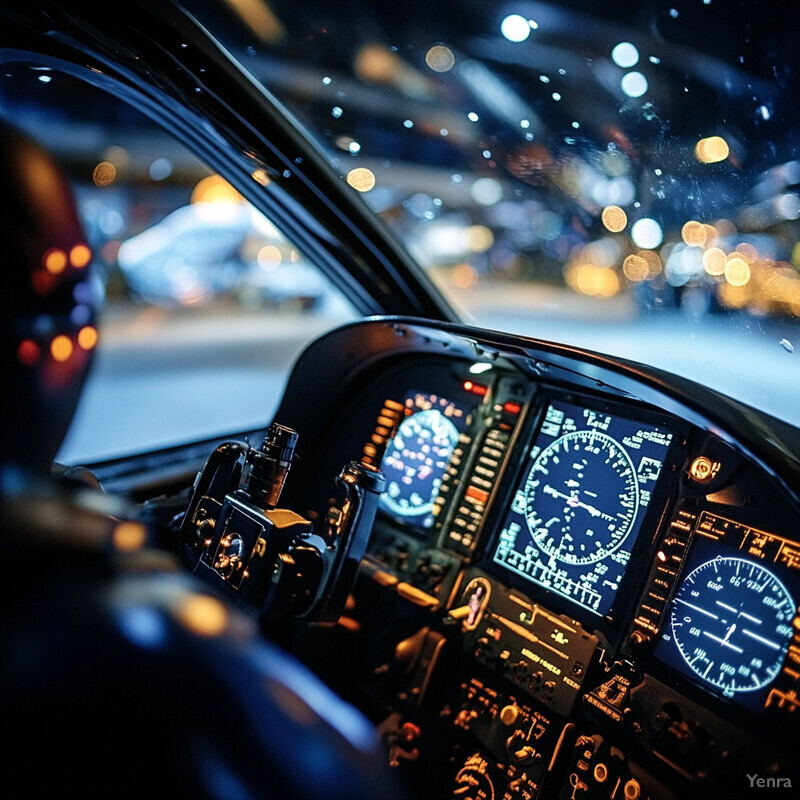
AI can combine visual, auditory, and haptic cues to create deeply immersive learning experiences. Consider a flight simulation that uses subtle vibrations in a joystick to signal turbulence, realistic engine audio cues, and visual changes in the cockpit instrumentation. The AI processes the trainee’s reactions—how quickly they adjust controls, how steadily they maintain altitude—and tailors future challenges to enhance learning. By engaging multiple senses and responding to real-time performance, AI-driven multimodal feedback ensures comprehensive skill development that encompasses both cognitive and motor abilities.