1. Handwriting Recognition in Historical Documents
AI-powered optical character recognition (OCR) can reliably read old cursive or faded handwriting in census records, parish registers, and other historical documents, reducing manual transcription efforts.
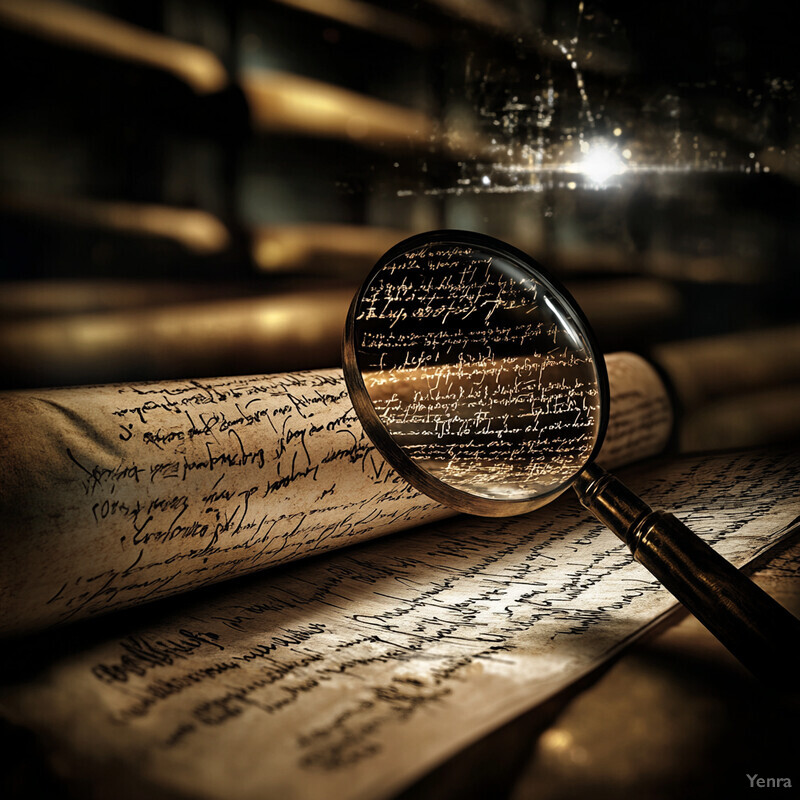
Traditional genealogical research often involves sifting through centuries-old, handwritten records that are faded, incomplete, or penned in archaic scripts. AI-powered handwriting recognition tools leverage advanced optical character recognition (OCR) and deep learning models trained on vast datasets of historical scripts. These models can interpret difficult-to-read cursive lettering and identify letters or symbols that would challenge even the most skilled human transcribers. By doing so, these tools significantly reduce the time needed for manual transcription, increase the accuracy of the recorded data, and make vast archives of historical documents more accessible to researchers worldwide.
2. Automated Transcription of Vital Records
AI tools can streamline the transcription of large volumes of birth, marriage, and death certificates, ensuring faster and more accurate data entry into genealogical databases.
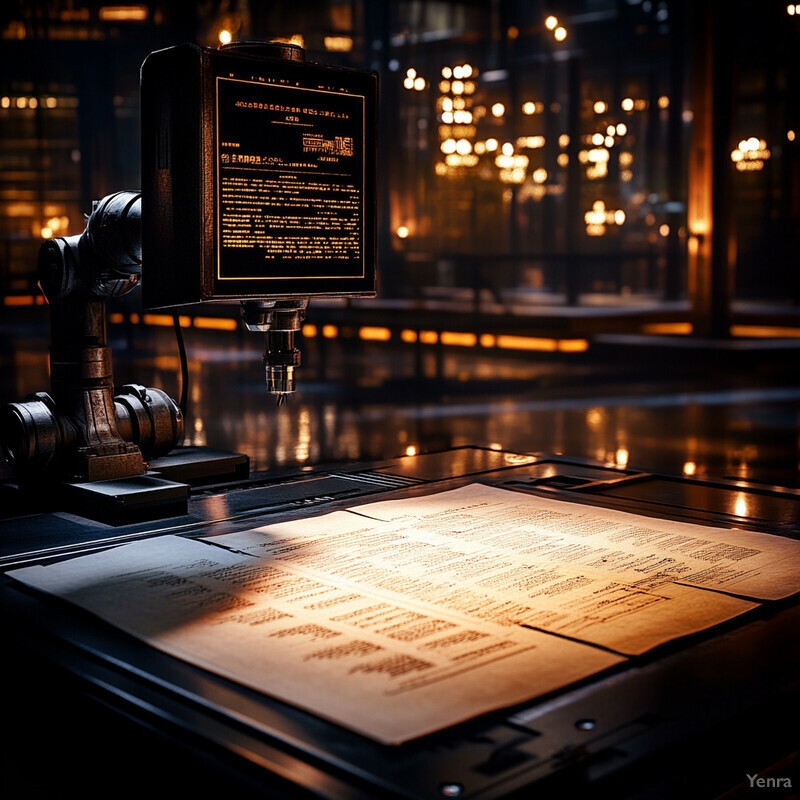
Vital records—such as birth, marriage, and death certificates—are key building blocks in constructing family histories. AI-driven transcription systems can process these documents at scale, extracting essential details (names, dates, locations, relationships) automatically. By applying natural language processing (NLP) techniques and pattern recognition, the systems minimize human error, improve the speed of data extraction, and enrich genealogical databases. This automation allows family historians and genealogical services to focus on analysis and interpretation rather than the laborious initial data-entry tasks.
3. Intelligent Record Linking
Machine learning algorithms can match individuals across different record sets—such as linking a birth record to a later census entry—making it easier to trace a person’s life events across multiple sources.
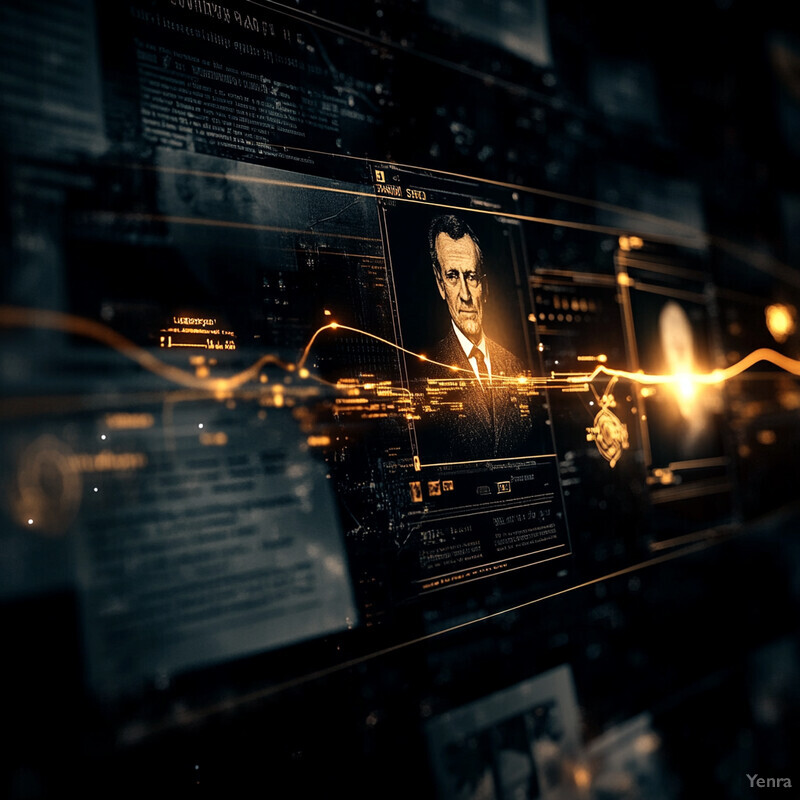
Genealogical research often requires piecing together fragments of a person’s life from disparate sources—census listings, immigration manifests, military enlistment papers, and more. AI algorithms excel at linking records across multiple datasets by comparing known attributes—like names, approximate birthdates, and family associations—and discovering patterns that indicate a match. Over time, these AI-driven processes become even more refined, making it possible to reconstruct a continuous life narrative from scattered documents, which helps researchers confidently identify ancestors and track them through various historical milestones.
4. Enhanced Name Normalization and Variant Detection
AI can reconcile the vast array of name spellings and variations, even across different languages and scripts, helping genealogists recognize that 'Johann Schmidt' and 'John Smith' might be the same ancestor.
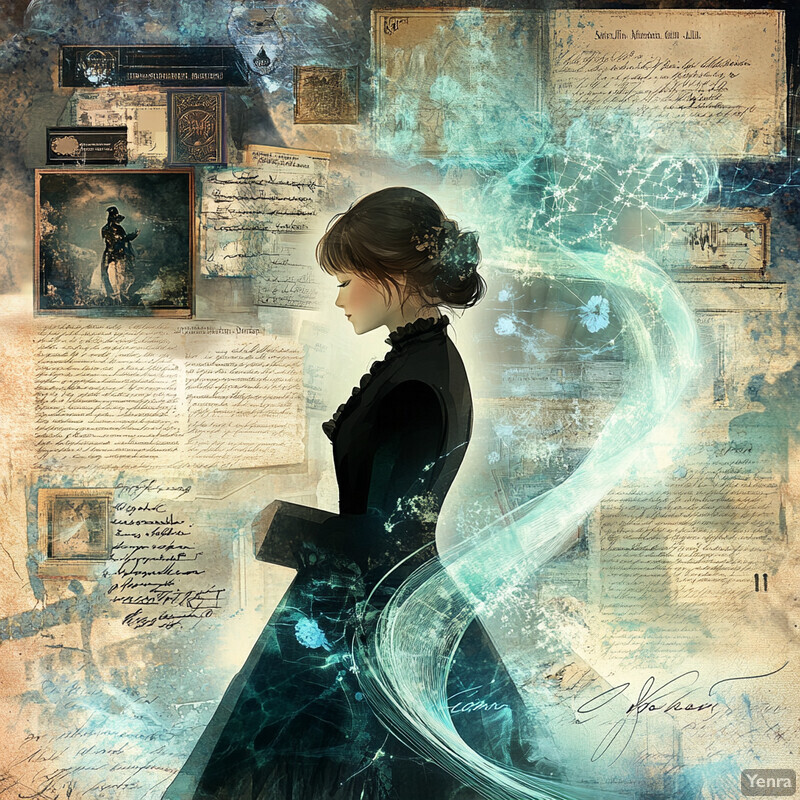
Names evolve and vary across time, cultures, and languages. A single ancestor’s name might appear in different forms—such as “Josef,” “Joseph,” or “Guiseppe”—throughout the historical record. AI-based name normalization tools use phonetic algorithms, linguistic models, and cultural context to unify these variants under a single consistent identifier. By recognizing and grouping variations, the system prevents researchers from missing critical pieces of their family story, ensuring that a slightly misspelled surname doesn’t lead to overlooking an essential document in the genealogical puzzle.
5. Automated Relationship Inference
By analyzing patterns in family trees, AI can infer potential familial relationships—such as suggesting a missing sibling or identifying a likely uncle—improving the accuracy of reconstructed pedigrees.
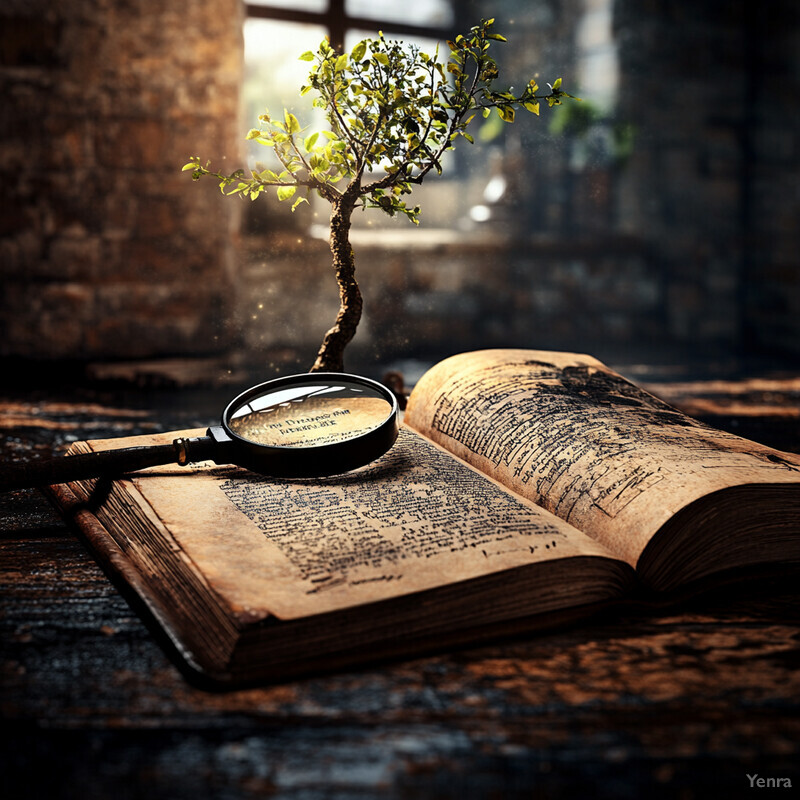
Understanding complex family networks can be challenging, especially when records are incomplete or contradictory. AI systems analyze patterns within family trees—such as birth intervals, sibling clusters, marriage timing, and geographic proximity—to suggest probable familial relationships. For instance, if multiple children are listed with similar surnames and birth years in related census records, the system might propose a previously unknown sibling. This inference accelerates the genealogical discovery process and provides leads for further verification, bringing researchers closer to a clearer picture of their ancestral lineage.
6. Language Translation and Standardization
Advanced natural language processing (NLP) tools can automatically translate old Latin, German Gothic script, or other languages, making it simpler to extract meaning from global genealogical sources.
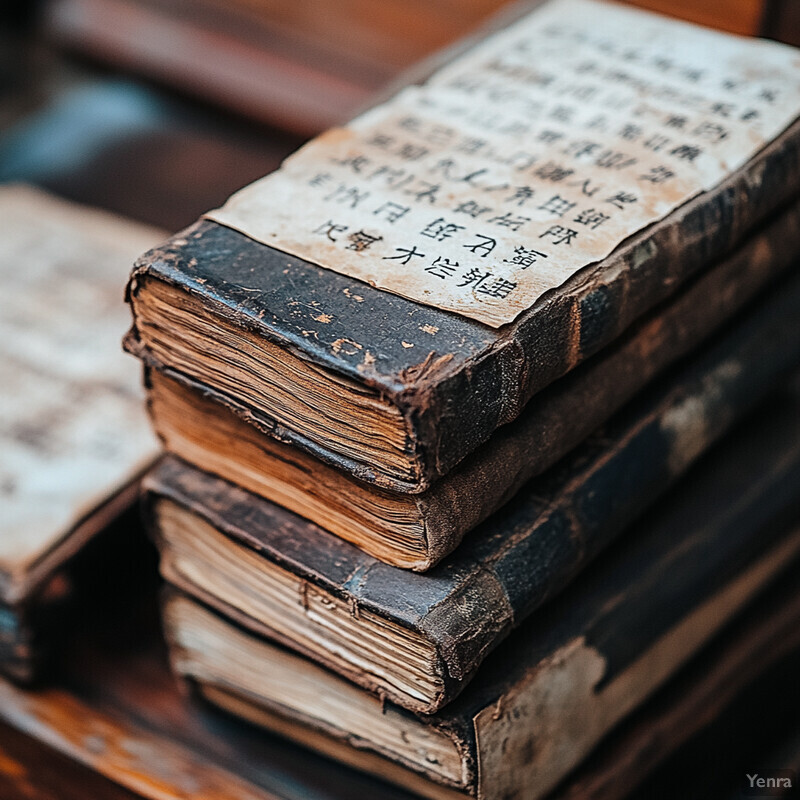
Genealogical documents often come from regions with different languages, dialects, and historical writing systems. AI-driven translation models can process old Latin church registers, German Gothic script records, or French civil documents, automatically converting them into a researcher’s preferred language. Alongside translation, AI also handles the standardization of dates, measurements, and place names, making it much easier for genealogists to interpret and compare data across linguistic and cultural boundaries, and thus broadening the scope of accessible historical records.
7. Historical Contextualization
AI can provide automatic annotations of events, geographic migration patterns, and socio-political circumstances surrounding an ancestor’s life, adding rich historical context to genealogical research.
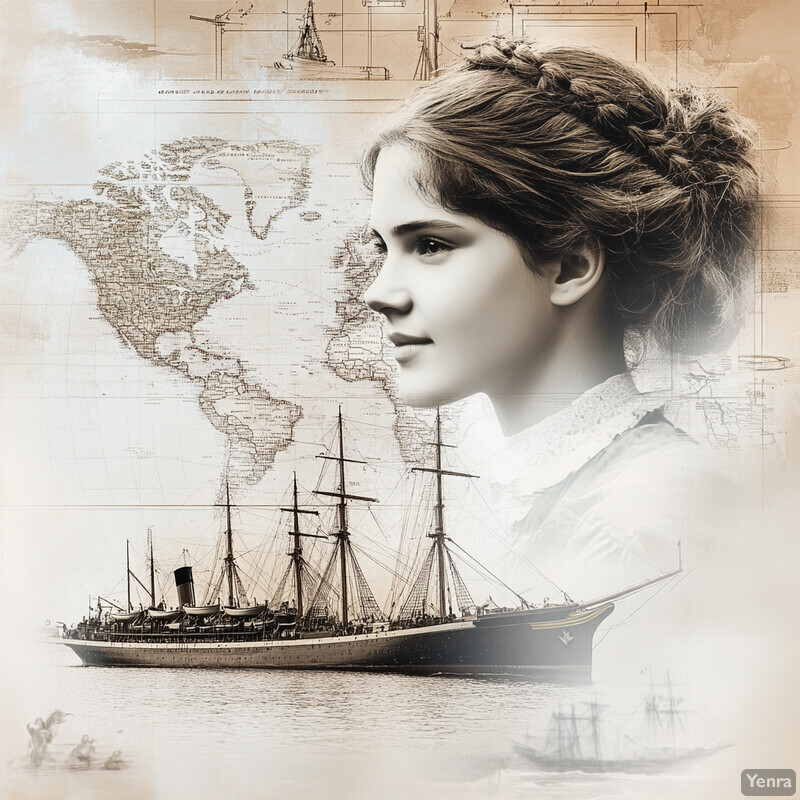
Family events—births, deaths, migrations—rarely occur in a vacuum. AI tools can enrich genealogical research by providing contextual information drawn from historical sources. By analyzing patterns and timelines, the system might identify that ancestors emigrated during a particular famine, or that a family’s move coincided with the opening of a new railway line. Automatically adding context helps genealogists understand not just who their ancestors were, but also why they made certain decisions, offering richer narratives that link personal family stories to larger historical currents.
8. Smart Search Recommendations
Sophisticated AI-driven search systems can understand queries semantically, suggesting relevant records or likely matches that a simple keyword search would miss.
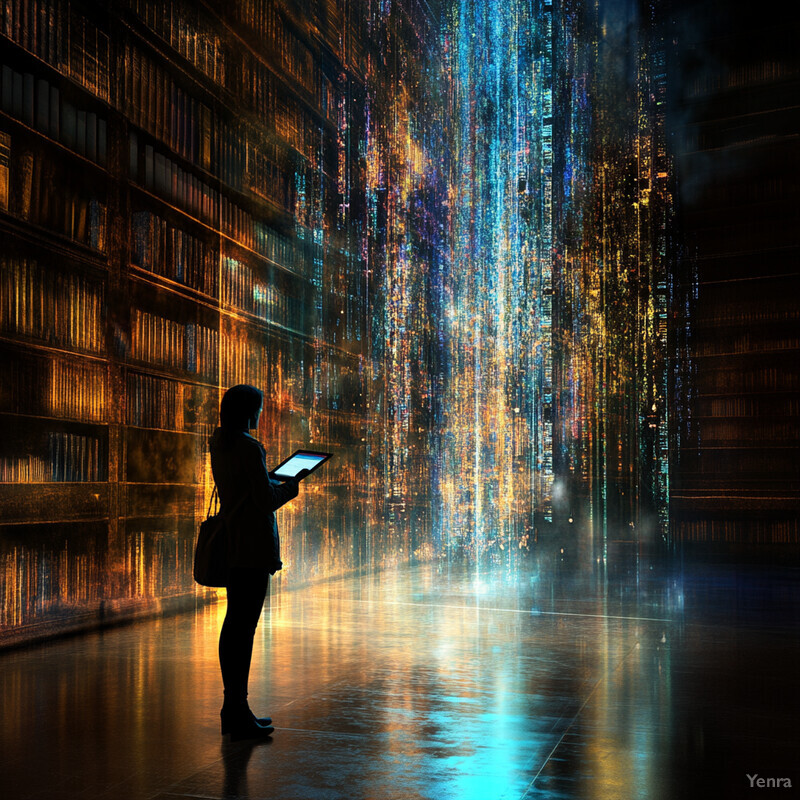
Instead of relying on basic keyword searches, AI-driven search engines in genealogical databases use semantic understanding to interpret user queries. For example, if someone searches for an ancestor’s birth record in “Prussia,” the system might also suggest documents from areas that were historically Prussian territory but are now part of Germany or Poland. By understanding geography, time periods, related surnames, and document types, these AI-enhanced systems can point researchers towards unexpected but relevant sources, ultimately speeding up discovery and reducing dead ends.
9. Pattern Recognition in Large Datasets
Machine learning can analyze patterns in massive genealogical databases, identifying common migration routes, surname distributions, and family clusters that guide researchers to their ancestors’ origins.
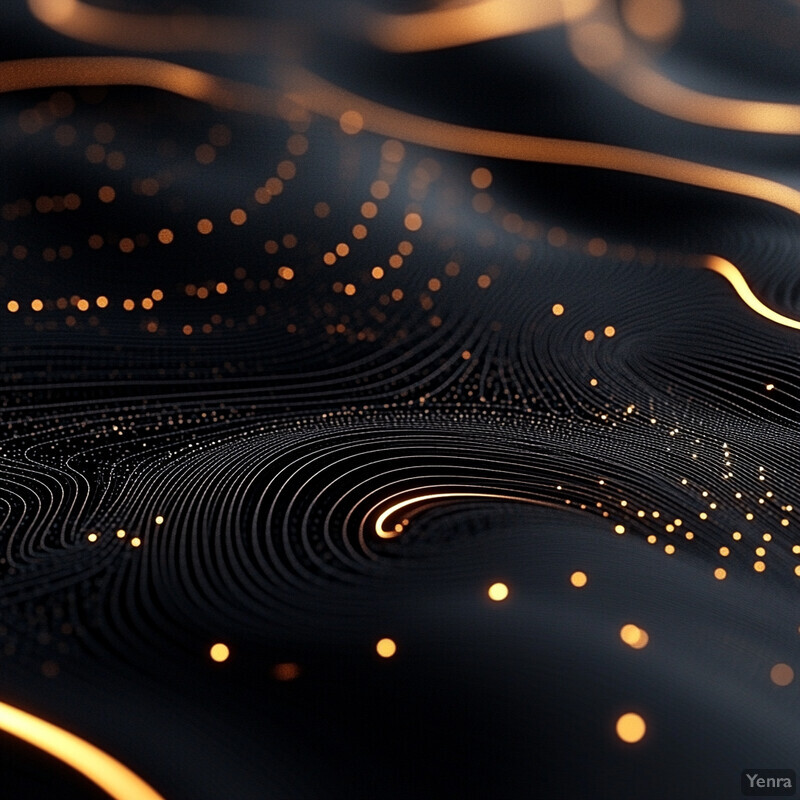
Massive genealogical databases contain hidden patterns and relationships that can be difficult to detect with human eyes alone. AI excels at processing large volumes of data, identifying migration patterns, surname distributions, and familial clustering. The system may reveal that a certain surname line frequently moved from one village to another every generation or that a particular profession persisted in a family over multiple centuries. This pattern recognition allows researchers to form new hypotheses, direct their searches more strategically, and gain deeper insights into their ancestors’ lifestyles and societal roles.
10. Automated Data Quality Checks
AI can flag inconsistent or improbable data—such as a recorded birthdate after a deathdate—alerting researchers to potential errors or transcription mistakes that need review.
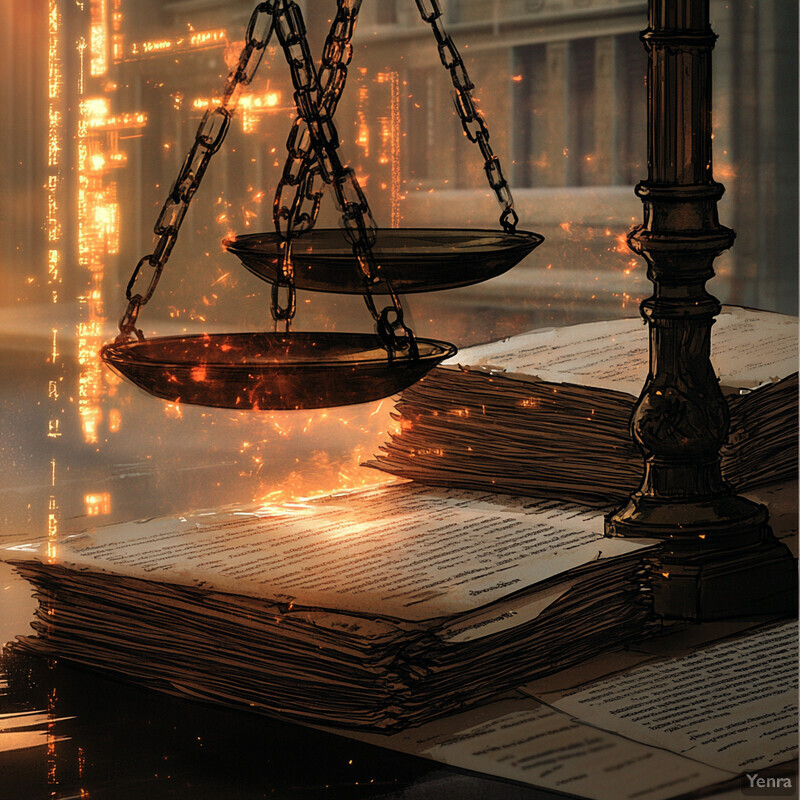
Genealogical data often originates from multiple sources, each with its own potential for transcription errors or contradictory details. AI-driven quality checks act as intelligent filters that flag inconsistencies—like children born before the recorded birthdates of their parents or individuals who appear in records after their supposed death. By highlighting these improbable details, AI gives genealogists cues to re-examine questionable documents or faulty assumptions, significantly improving the accuracy and reliability of reconstructed family trees.
11. Facial Recognition in Old Photographs
Emerging AI-driven image analysis tools can compare old family photos, identifying individuals who appear in multiple images and potentially confirming their identities.
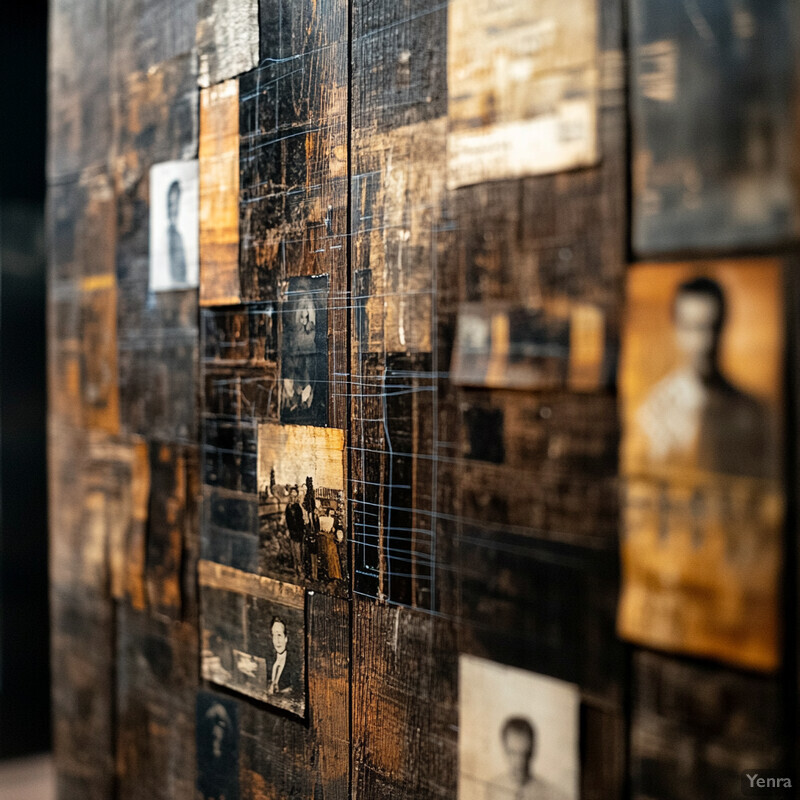
Photographs provide a vivid, personal window into the past, but identifying the people in them can be a longstanding challenge. AI-based facial recognition algorithms can now analyze the features of individuals in old images, comparing them to known reference photos. Over time, this can confirm identities, group images by person, and help genealogists track an ancestor’s appearance across decades. Such tools transform obscure, unlabeled family snapshots into valuable documentary evidence, reinforcing connections between images and historical records.
12. Document Classification and Tagging
AI can classify historical documents by type (e.g., census, military record, immigration manifest) and tag them with keywords, making browsing and retrieval much more efficient.
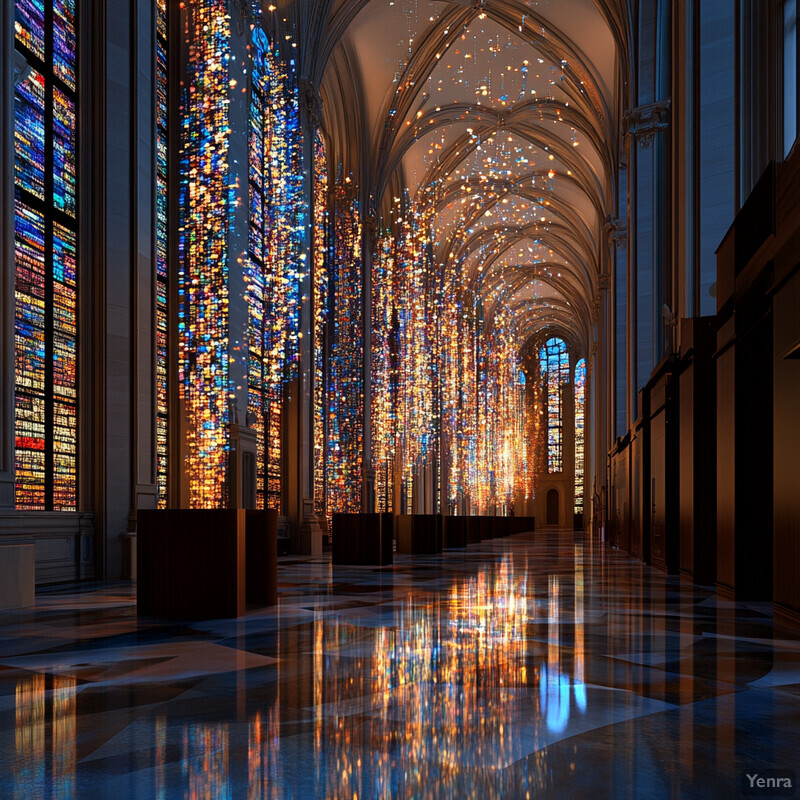
Historical archives contain a wide variety of documents—census pages, immigration records, probate inventories, military draft cards—and organizing them can be a monumental task. AI can automatically classify documents by type, language, date range, and geographic location, and then apply relevant keywords or categories. By making it easier to navigate these databases, researchers spend less time sifting through irrelevant material and more time focusing on their areas of interest. This efficiency in classification not only aids professionals but also empowers amateur genealogists to explore more records independently.
13. Geo-Referencing Historical Places
AI can automatically link historical place names (often no longer in use) to current map coordinates, aiding genealogists in visualizing ancestral migrations and land holdings.
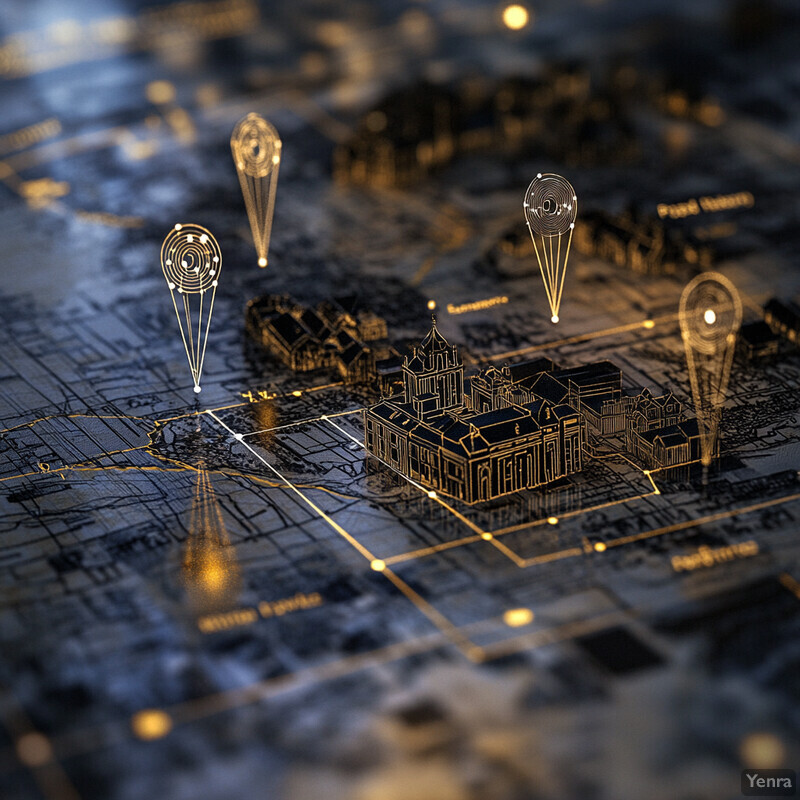
Place names frequently change over time due to political shifts, spelling variations, and local dialects. AI systems can correlate historical place names with modern geographic coordinates, maps, and standardized place directories. This geo-referencing helps genealogists visualize ancestral journeys, identify the ancestral homestead on a modern map, and understand historical migration patterns. It transforms abstract references in old records into tangible, present-day geographical contexts, making it simpler to appreciate the physical reality of one’s family history.
14. Inferring Missing Data
Predictive modeling can fill in gaps in family trees by suggesting plausible birth years, marriage intervals, or migration timelines based on patterns found in similar family structures.
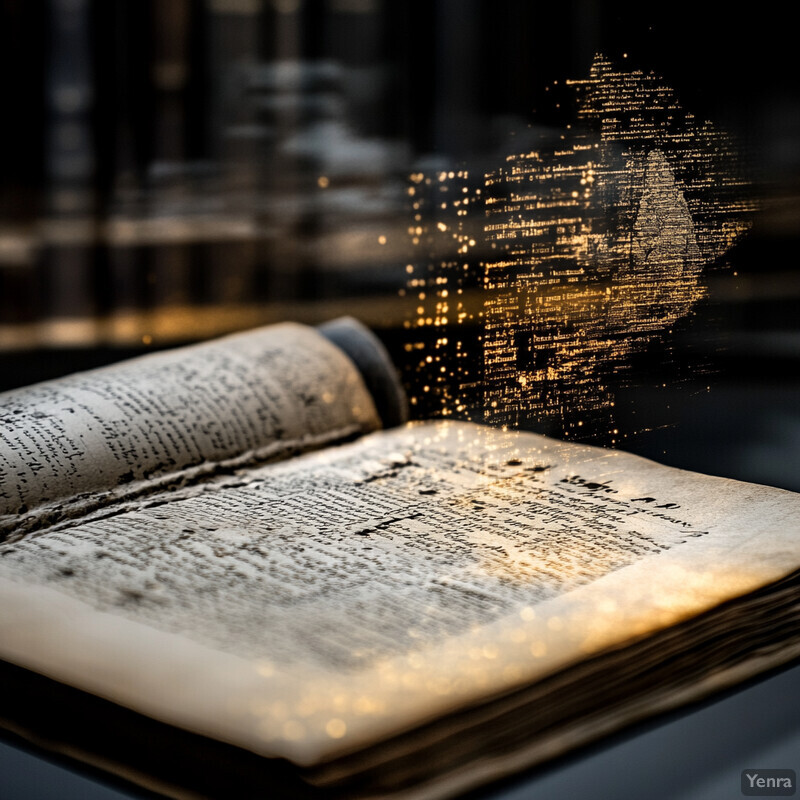
Not all genealogical documents are complete; some have missing birthdates, others lack evidence of migration, and many omit the maiden names of female ancestors. AI can predict and suggest missing details by modeling patterns observed in similar records. For instance, if all siblings in a family were born about two years apart in the same village, the AI might estimate a missing sibling’s birth year within a reasonable range. While these inferences are not definitive, they provide invaluable leads for researchers to pursue further and guide logical next steps in the research process.
15. Multimodal Analysis of Text and Images
By combining textual and visual AI techniques, researchers can extract genealogical data from newspapers, gravestone inscriptions, and old postcards without needing separate manual efforts.
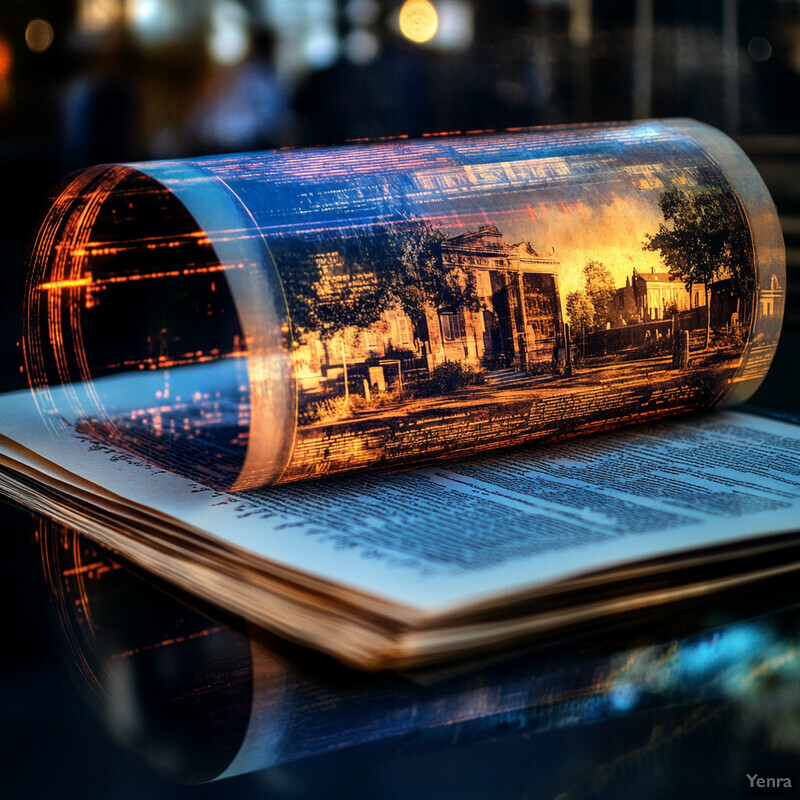
Genealogical clues come in many forms: tombstone inscriptions, newspaper clippings, postcards, and ledger sheets. AI’s multimodal analysis capabilities allow it to process and integrate textual and visual information simultaneously. For example, it can read an obituary’s text while also interpreting the accompanying family photograph to confirm identities. By synthesizing multiple data types, AI breaks down silos that previously required separate manual efforts, giving genealogists more cohesive and compelling evidence for their ancestral narratives.
16. Expert Virtual Assistants
AI chatbots trained specifically in genealogy can guide users through complex research steps, suggest search strategies, and highlight overlooked records, acting as virtual research assistants.
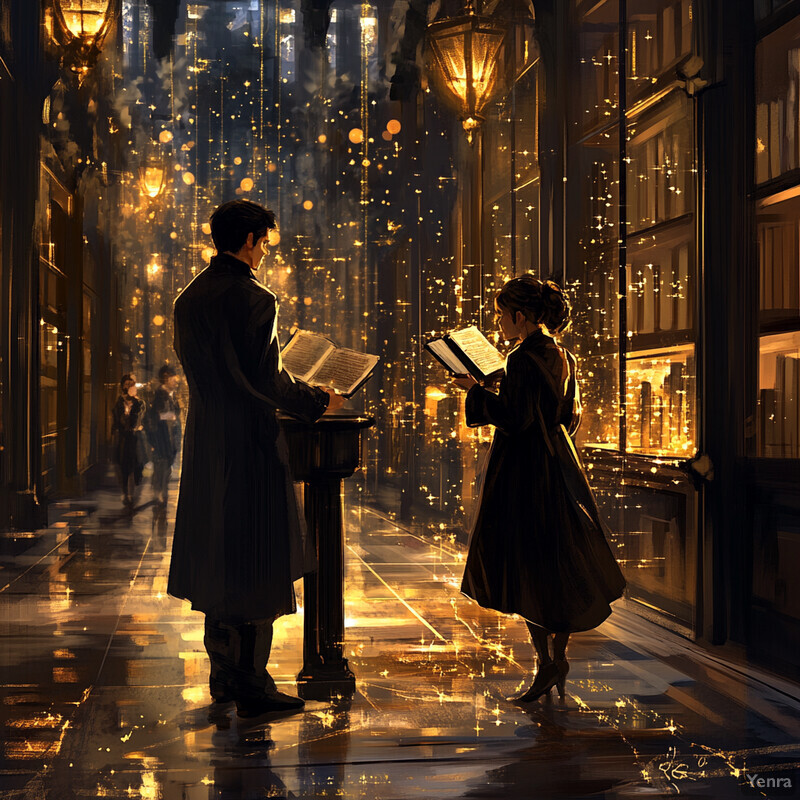
With AI-driven virtual assistants, genealogists can now access on-demand expertise. These digital helpers, trained on extensive genealogical knowledge and research techniques, can suggest possible record sets to explore, explain the historical significance of certain events, and highlight commonly overlooked sources. As a result, beginners gain a guided learning experience, and experienced genealogists can streamline their workflows. This democratization of expert guidance empowers all researchers to advance in their projects without waiting for specialized, human expert intervention.
17. Continuous Machine Learning Updates
As more records and genealogies are added, AI models can continuously retrain, improving their accuracy and ensuring that newly digitized documents are integrated efficiently.
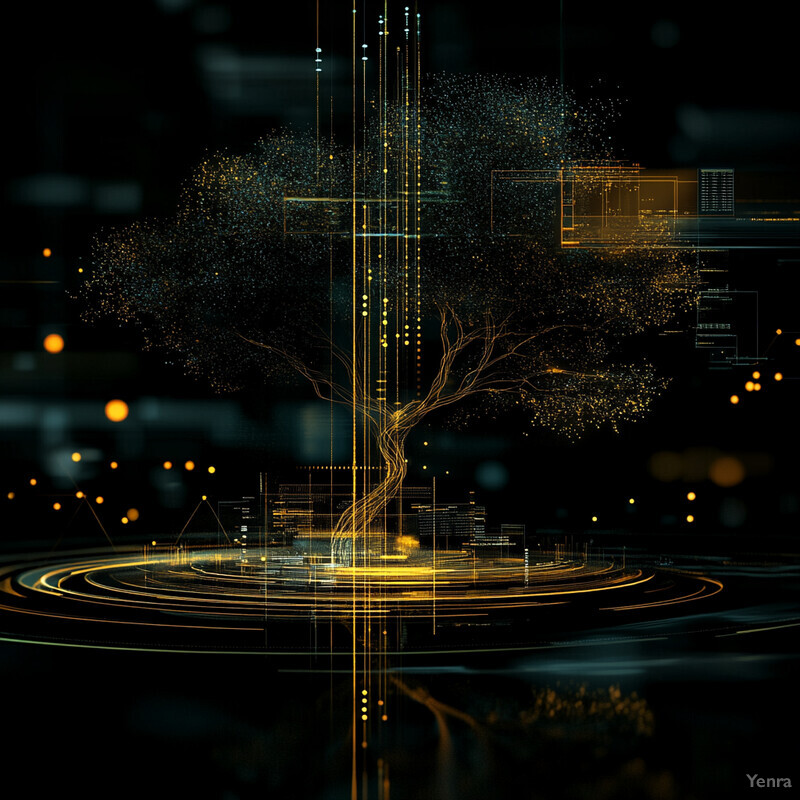
AI systems improve through iteration. As new historical documents are digitized, corrections are made to existing datasets, and user feedback refines search accuracy, the underlying machine learning models evolve and improve. Over time, these improvements compound, leading to more reliable transcription accuracy, better record linking, and more insightful predictions. This continuous feedback loop ensures that genealogical research tools remain state-of-the-art and adaptable to the ever-expanding universe of historical records.
18. Sophisticated Identity Resolution
Algorithms can merge multiple partial identities into a single ancestor profile, discerning that various partial records refer to the same individual, even if scattered or incomplete.
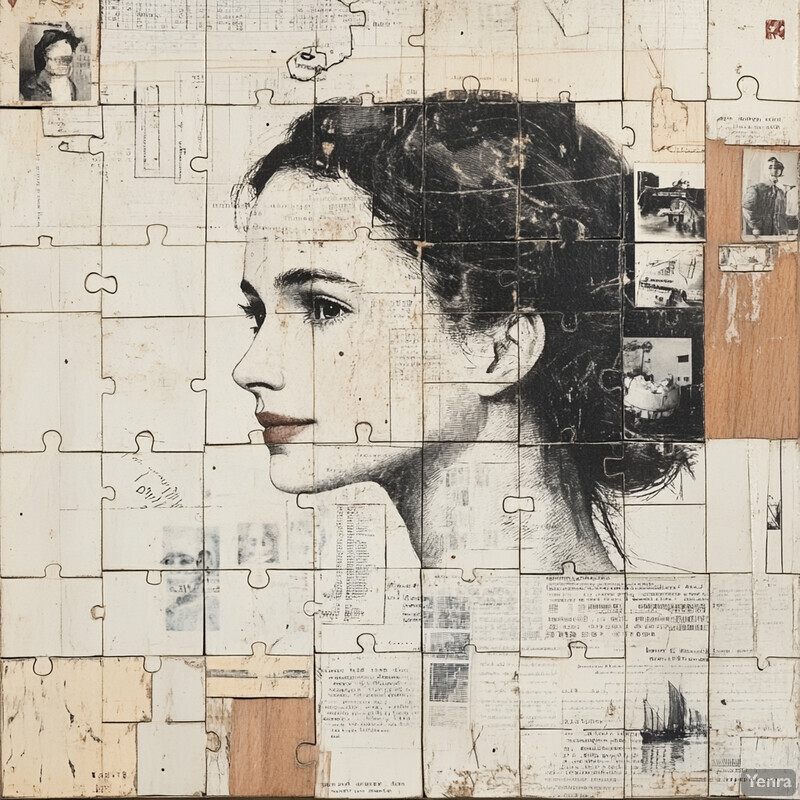
Historical documents might refer to the same individual by slightly different names, birthdates, or residences. AI excels at reconciling these fragmented identities into a single coherent ancestor profile. By comparing metadata (dates, locations, occupations, family ties) and applying probabilistic models, AI determines the likelihood that various references point to one person. This identity resolution reduces duplication in genealogical databases, clarifies ancestral lines, and makes it easier for researchers to confidently trace a single individual’s trajectory through history.
19. Integration with Genetic Data
AI can correlate genealogical records with DNA test results, pinpointing genetic connections and helping users discover new relatives or confirm uncertain parentage lines.
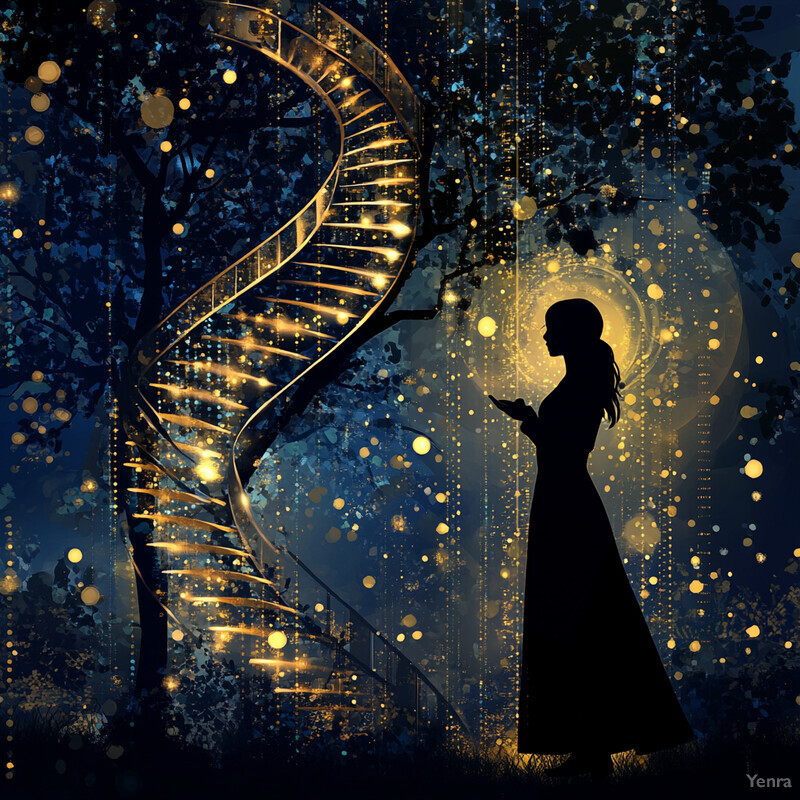
Modern genealogical research often incorporates DNA testing results, which reveal genetic matches and ancestral origins at a population level. AI can integrate these genetic insights with historical records to pinpoint specific family lines, confirm hypothesized relationships, and identify unknown relatives. By combining documentary evidence with genetic markers, AI helps resolve ancestral riddles that traditional records alone might fail to clarify. The result is a more holistic, science-driven approach to uncovering family histories.
20. Automated Narrative Generation
Advanced NLP can generate readable family history narratives from structured genealogical data—providing personalized family stories that integrate verified events, places, and relationships.
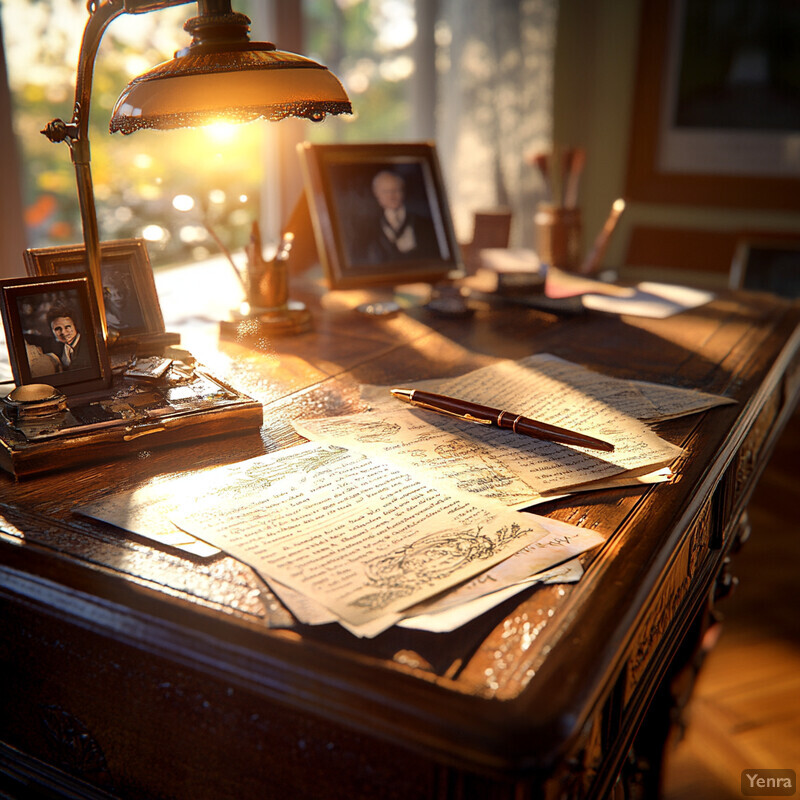
Transforming raw genealogical data into a compelling family narrative can be difficult. Advanced AI-driven natural language generation tools take structured data—names, dates, places, events—and craft coherent, readable stories that highlight significant life events, migrations, and cultural contexts. This automated storytelling not only saves time but also makes the research more engaging and accessible. Family members and future generations can enjoy a well-rounded narrative of their ancestry, combining factual accuracy with the human interest of personal history.