1. Enhanced Remote Sensing Analysis
AI-driven image recognition and classification algorithms can automatically process satellite, aerial, and drone imagery to identify changes in land use, vegetation cover, and water quality, reducing the time and effort required for manual interpretation.
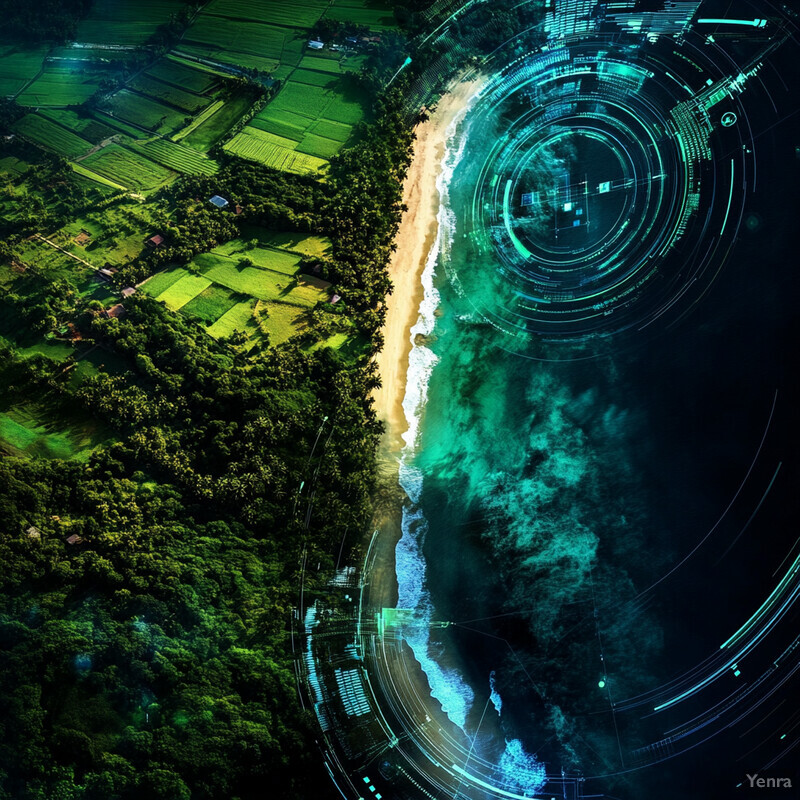
Artificial Intelligence (AI) tools significantly improve the interpretation and utilization of remote sensing data obtained from satellites, drones, and airborne sensors. By applying deep learning algorithms to multispectral or hyperspectral imagery, AI can automatically classify land cover, detect subtle environmental changes, and identify areas of concern such as deforestation or erosion. This automation reduces the manual effort traditionally required in analyzing large datasets, ensures more consistent interpretation, and increases the speed at which decision-makers receive actionable information. The result is a more nuanced understanding of baseline environmental conditions, allowing project developers and regulators to pinpoint sensitive areas and implement protective measures during Environmental Impact Assessments (EIAs).
2. Predictive Modeling of Ecosystem Impacts
Advanced machine learning models can integrate historical environmental data, climate forecasts, and ecological parameters to predict potential impacts on ecosystems with greater accuracy and reliability.
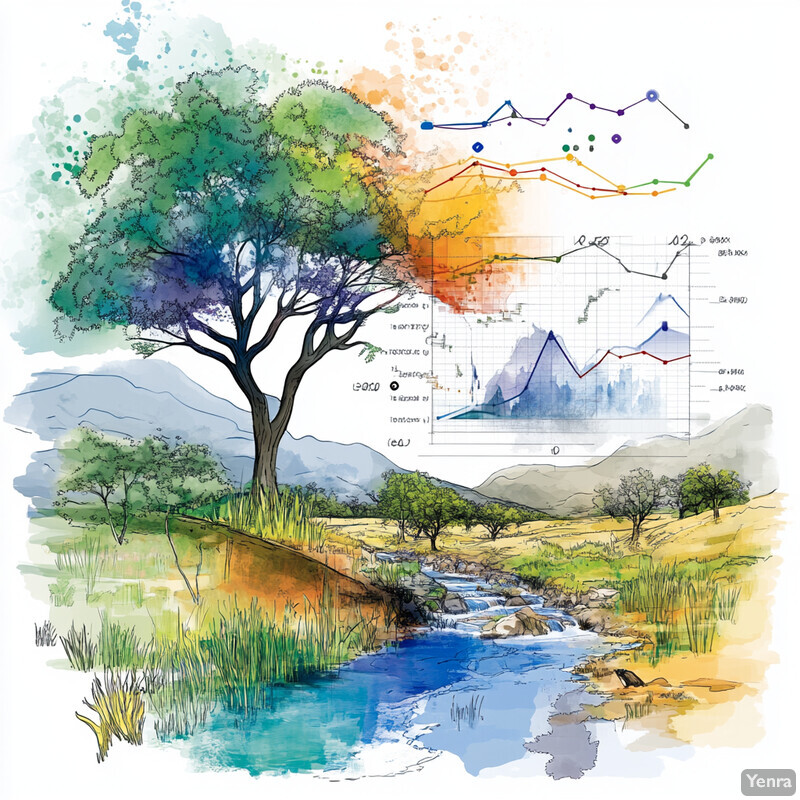
AI-driven predictive models use extensive historical datasets combined with current and projected conditions—such as climate patterns, species distributions, and habitat quality—to forecast how proposed projects might affect ecosystems. These models can integrate variables like temperature, precipitation, and anthropogenic stressors to simulate potential changes in species composition or habitat fragmentation over time. By running multiple scenarios, EIAs gain a robust view of the range and likelihood of ecological outcomes. This foresight equips stakeholders with the information needed to develop proactive mitigation strategies and long-term conservation plans, ensuring that interventions are both cost-effective and ecologically sound.
3. Automated Species Identification
Using AI-powered image and audio recognition, field data on flora and fauna can be rapidly analyzed to determine species presence, abundance, and distribution, improving biodiversity assessments.
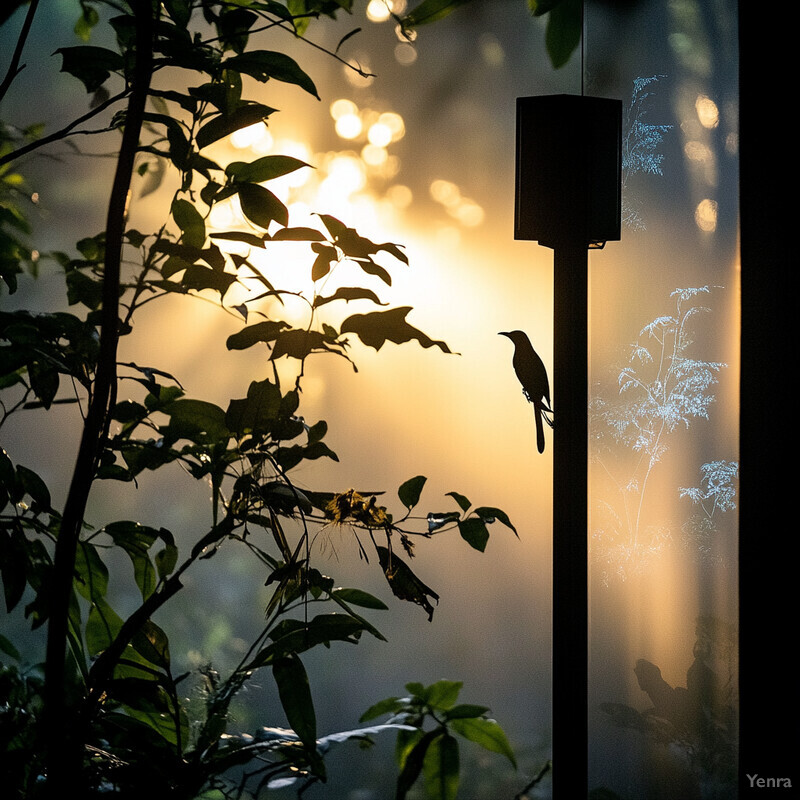
Traditionally, identifying species on-site or in recorded data requires specialized expertise and time-consuming fieldwork. AI now leverages computer vision and machine learning algorithms to rapidly process images and audio recordings, accurately identifying species with fewer human errors. For example, camera-trap images or bird calls can be automatically analyzed to determine species presence and abundance. This increases the scalability and efficiency of biodiversity assessments, allowing EIAs to encompass broader survey areas, capture seasonal variations, and track shifts in community composition. More comprehensive species data lead to better-informed assessments and enhanced protection for vulnerable wildlife populations.
4. Real-Time Environmental Monitoring
AI can process continuous streams of sensor data—such as water quality, air quality, and soil condition—enabling dynamic and adaptive Environmental Impact Assessments (EIAs) that reflect current conditions rather than static snapshots.
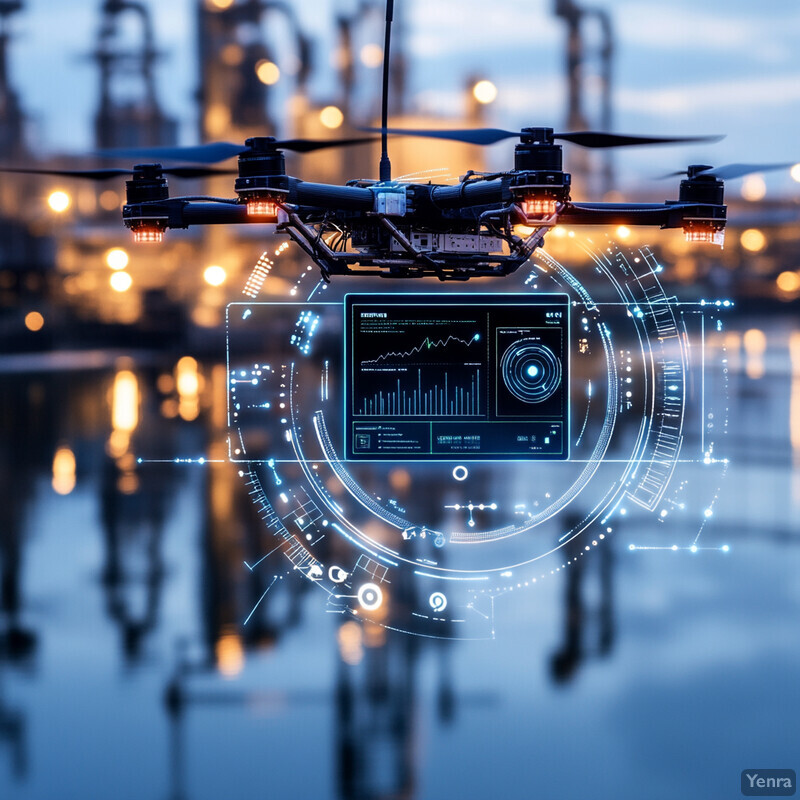
By integrating AI with sensor networks, environmental parameters—such as water turbidity, pH, air pollutant concentrations, and noise levels—can be continuously monitored and analyzed in real-time. Machine learning models identify anomalous patterns, detect threshold exceedances, and alert stakeholders immediately. This dynamic approach moves beyond static, one-time assessments, ensuring that EIAs reflect ongoing conditions rather than outdated data points. The continuous feedback allows project developers to implement timely mitigation measures, regulators to enforce compliance effectively, and communities to have ongoing insight into the environmental health of their region.
5. Enhanced Climate Risk Assessment
By incorporating climate models and big data sets, AI can better forecast how proposed projects may interact with future temperature, precipitation, and extreme weather events, improving the climate resilience component of EIAs.
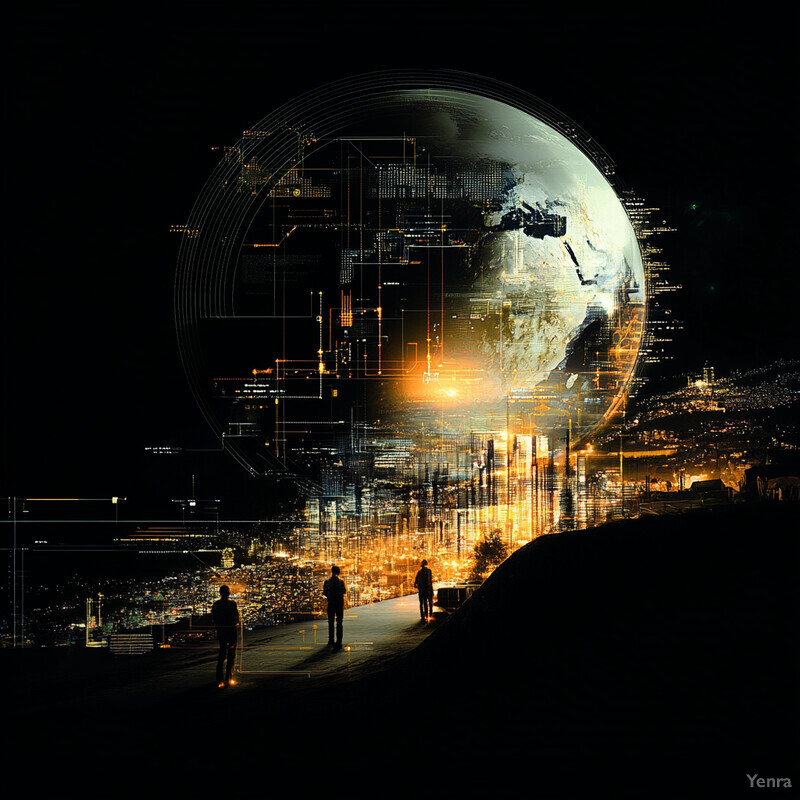
As climate change intensifies, understanding how future climatic conditions could interact with proposed developments becomes essential. AI can integrate global circulation models, historical climate records, and project-specific spatial data to estimate the future risks related to extreme weather events, shifting precipitation patterns, and rising temperatures. With this analysis, EIAs become forward-looking tools that help decision-makers anticipate challenges like drought stress, flood vulnerability, and habitat shifts. By incorporating climate scenarios, planners can design projects that are more resilient, minimize environmental damage, and ensure long-term sustainability even under changing climatic conditions.
6. Cumulative Impact Analysis
Machine learning can integrate data from multiple projects and environmental factors in a given region, helping to identify cumulative impacts that might be overlooked when considering each project individually.
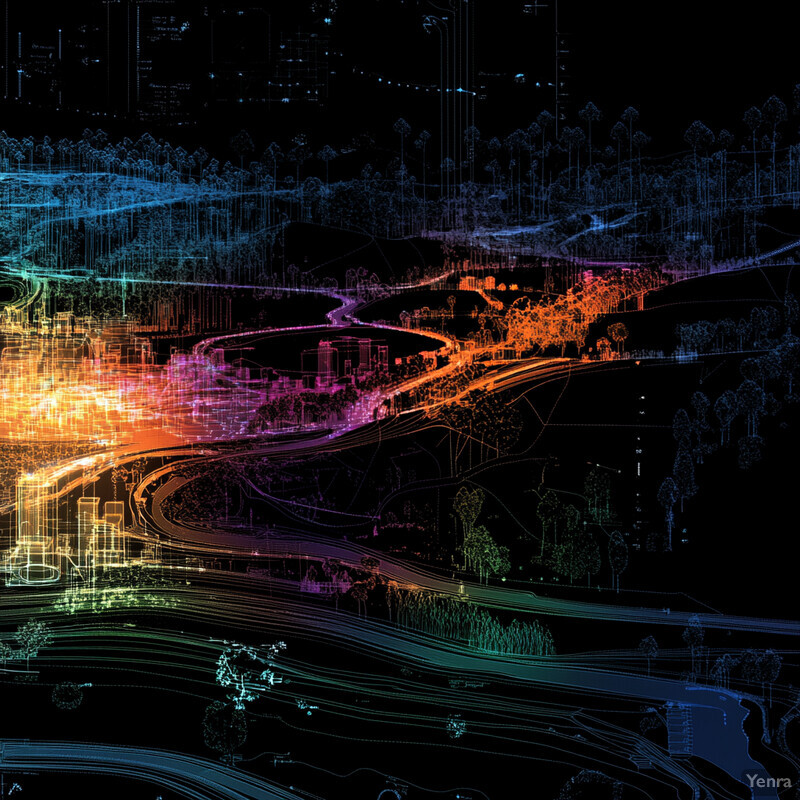
Environmental impacts rarely occur in isolation. AI systems can combine datasets from multiple projects, policies, and landscape changes to identify cumulative impacts that may only become evident at broader scales or over longer periods. By comparing current and past conditions, as well as potential future scenarios, machine learning models can highlight underlying trends and interactions that might be overlooked in a single-project assessment. Such holistic insights help policymakers, stakeholders, and environmental managers understand the bigger picture, leading to more informed decision-making and effective regional planning strategies that mitigate broad-scale ecosystem degradation.
7. Adaptive Habitat Modeling
AI algorithms can model habitat fragmentation and quality changes over time, assisting planners and conservationists in pinpointing areas likely to be most affected by development and guiding mitigation strategies.
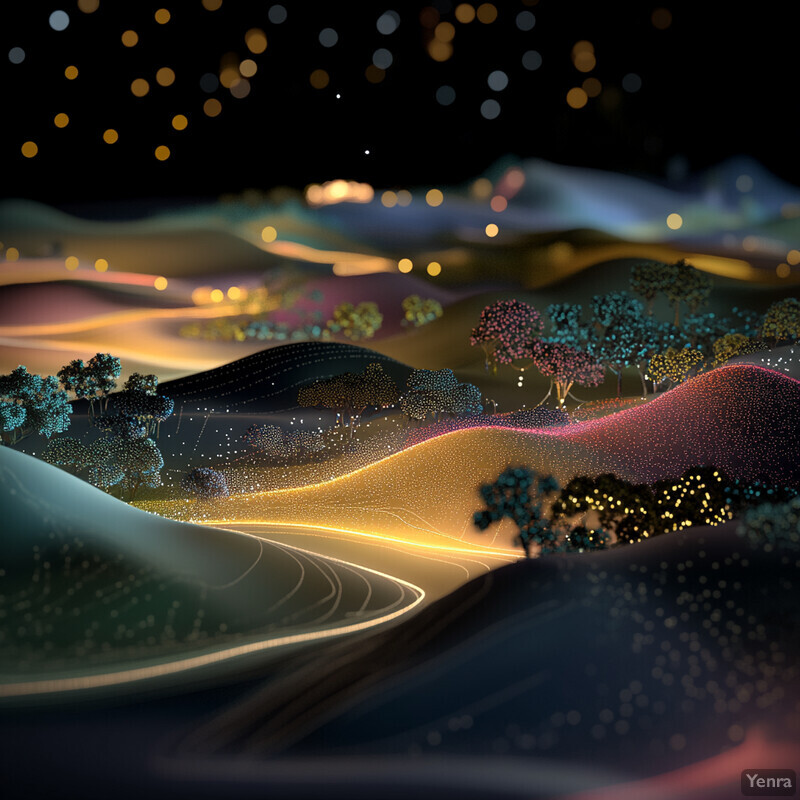
Habitats are dynamic, and their quality can fluctuate based on numerous factors such as invasive species, land use changes, and pollution. AI-driven habitat models incorporate diverse datasets—remote sensing imagery, climate projections, topographical maps, and species occurrences—to track and predict changes in habitat structure and suitability. These models can guide EIAs in identifying current and future high-value habitats that should be preserved or restored. By providing a spatially explicit, evolving picture of habitat quality, projects can be designed or modified to avoid critical habitats, thus maintaining ecological integrity and reducing the likelihood of biodiversity loss.
8. Improved Groundwater and Surface Water Modeling
AI techniques can refine hydrological models, predicting changes in groundwater recharge, surface run-off, and flood risk more precisely, vital for water resource management in EIAs.
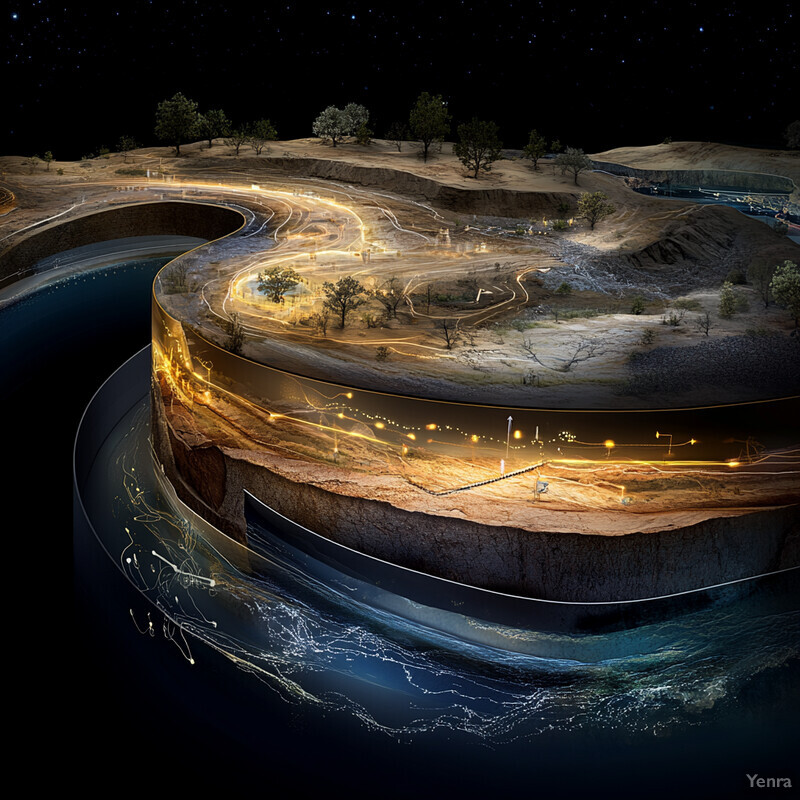
Water resources are often at the heart of environmental assessments, influencing ecosystems, agriculture, and community health. AI enhances hydrological models by rapidly processing large volumes of climate, geological, and hydrodynamic data. The resulting models predict changes in groundwater levels, river flows, sediment transport, and pollutant dispersion under various development scenarios. This capability provides a scientific foundation for understanding how construction, resource extraction, or infrastructure projects might affect watershed health, enabling targeted mitigation efforts that safeguard drinking water supplies, aquatic habitats, and downstream ecosystems.
9. Early Detection of Pollution Events
Advanced anomaly detection algorithms can quickly identify unusual pollutant levels or emissions, enabling earlier intervention and mitigation measures during and after project development.
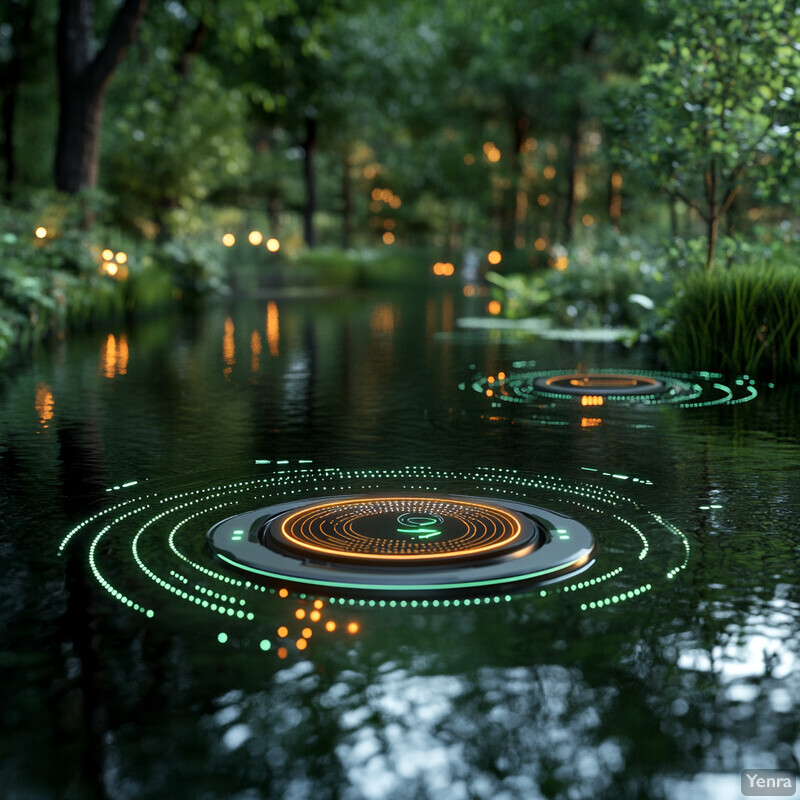
One of the greatest advantages AI brings to EIAs is the capacity for early warning systems. By continuously processing sensor data on air, water, and soil quality, machine learning algorithms can identify subtle deviations from baseline conditions. These algorithms learn what "normal" looks like and can detect anomalies—such as sudden increases in pollutant levels—far earlier than manual inspection would allow. Early detection empowers stakeholders to respond proactively, deploying containment measures or halting activities that may be causing contamination, ultimately limiting ecological damage and potentially avoiding regulatory violations and costly clean-ups.
10. Streamlined Data Integration
AI can integrate diverse data sources—remote sensing imagery, field measurements, geological surveys, climate models, and biodiversity inventories—into coherent analytical frameworks for more holistic EIAs.
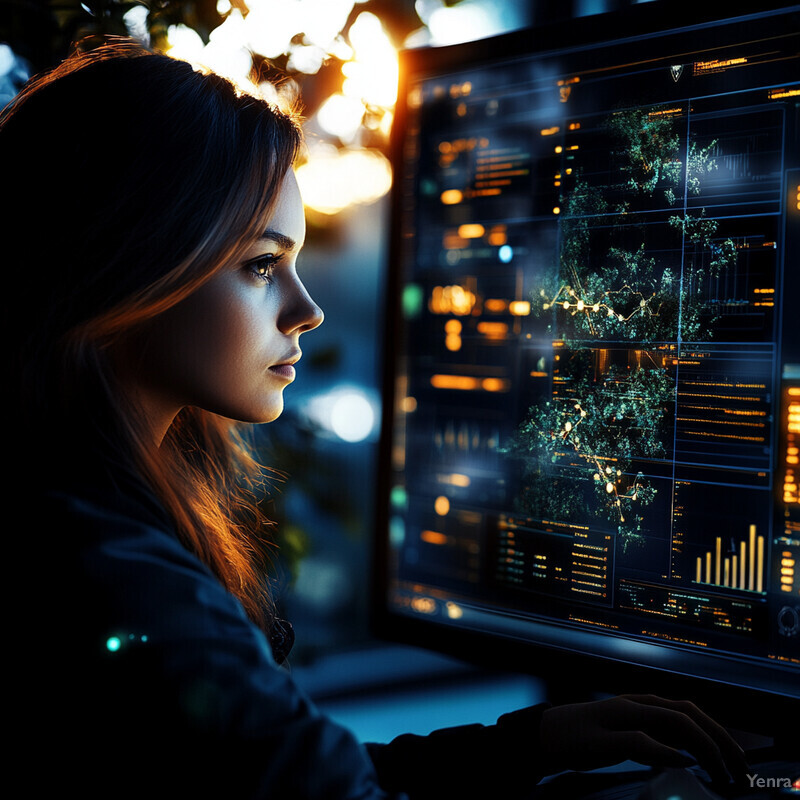
Effective EIAs require synthesizing diverse datasets—biological surveys, geological reports, historical land use records, climate models, and socio-economic indicators—into a coherent whole. AI excels at processing and integrating these complex, high-volume data streams. Using sophisticated data fusion techniques, machine learning can transform disparate, unstructured inputs into standardized, analyzable formats. This streamlining reduces the time and labor required, minimizes errors in manual data handling, and allows environmental practitioners to focus on interpretation and decision-making rather than data wrangling. The result is more holistic, data-rich EIAs that are both timely and accurate.
11. Optimized Site Selection
By running complex spatial and environmental constraints through AI optimization algorithms, developers can choose project sites with the least environmental impact, minimizing ecological harm from the outset.
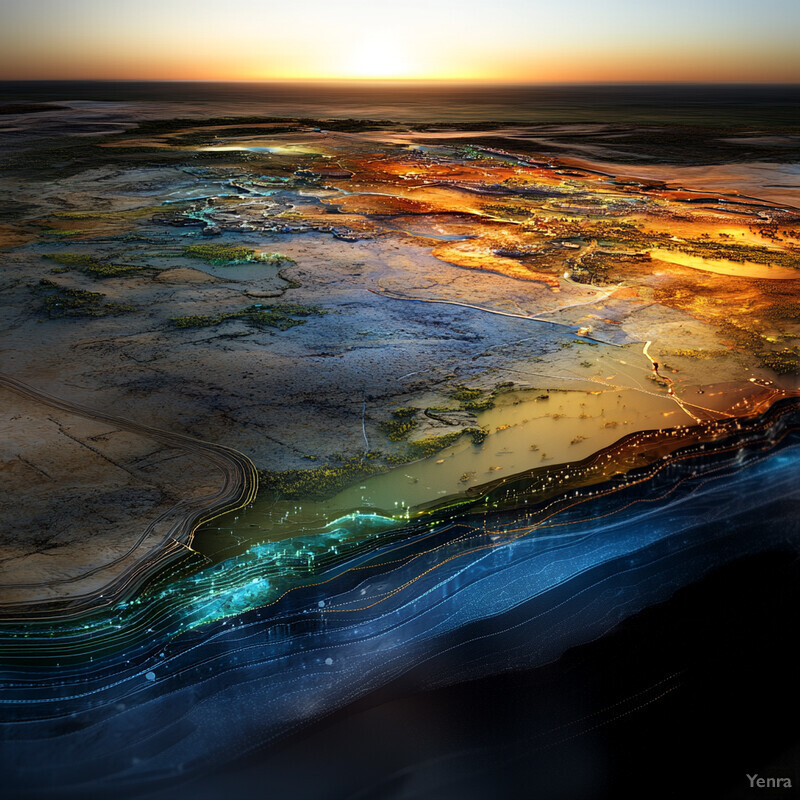
In large-scale development projects, selecting a site that minimizes environmental harm is a critical goal. AI optimization algorithms can rapidly evaluate multiple spatial layers—terrain stability, biodiversity hotspots, water resources, and protected areas—alongside infrastructure needs and economic constraints. By running countless scenarios, these algorithms identify locations that meet project requirements while reducing impacts on sensitive ecosystems. This scientifically guided site selection process helps prevent costly redesigns, streamlines permit approvals, and protects vital natural resources before project activities even begin.
12. Enhanced Scenario Testing
AI-driven scenario analysis allows multiple future conditions (e.g., differing regulatory frameworks, climate conditions, or mitigation strategies) to be tested rapidly, helping decision-makers weigh outcomes more effectively.
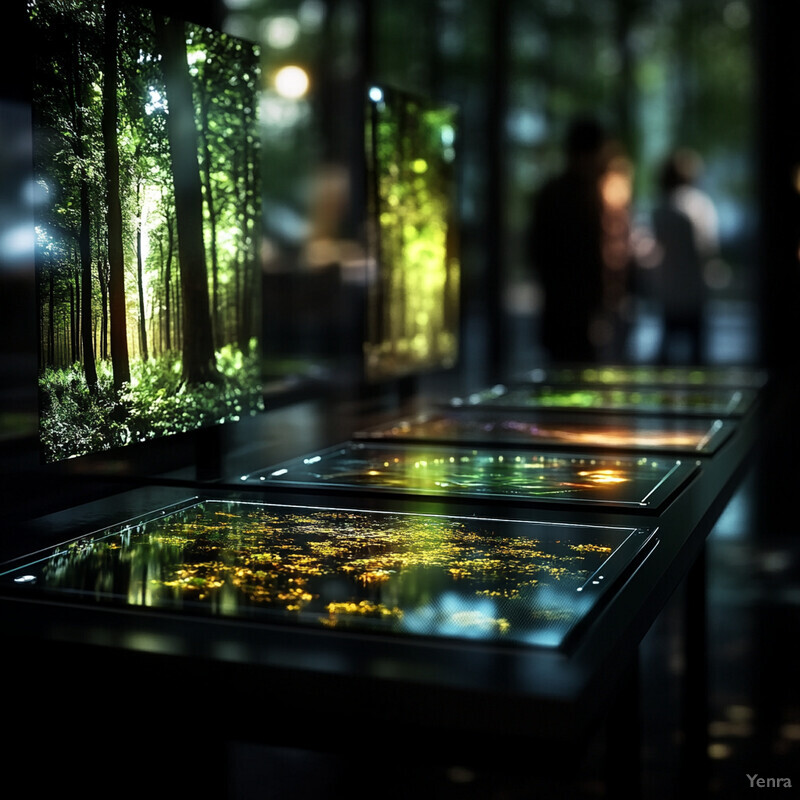
Complex environmental and socio-economic systems demand robust scenario analysis. AI-based simulation platforms can create and test numerous “what-if” scenarios, accounting for variations in regulatory frameworks, mitigation strategies, and future environmental conditions. These tools let decision-makers visualize the consequences of different actions, whether it’s building a dam, creating a wind farm, or expanding agricultural areas. By comparing outcomes side-by-side, EIAs can identify the path that best achieves sustainable development goals, balances community interests, and preserves ecological integrity under uncertain future conditions.
13. NLP for Document Analysis
Natural Language Processing (NLP) can help process large volumes of technical reports, policy documents, and EIA submissions, extracting key insights, flagging compliance issues, and summarizing lengthy texts for quicker review.
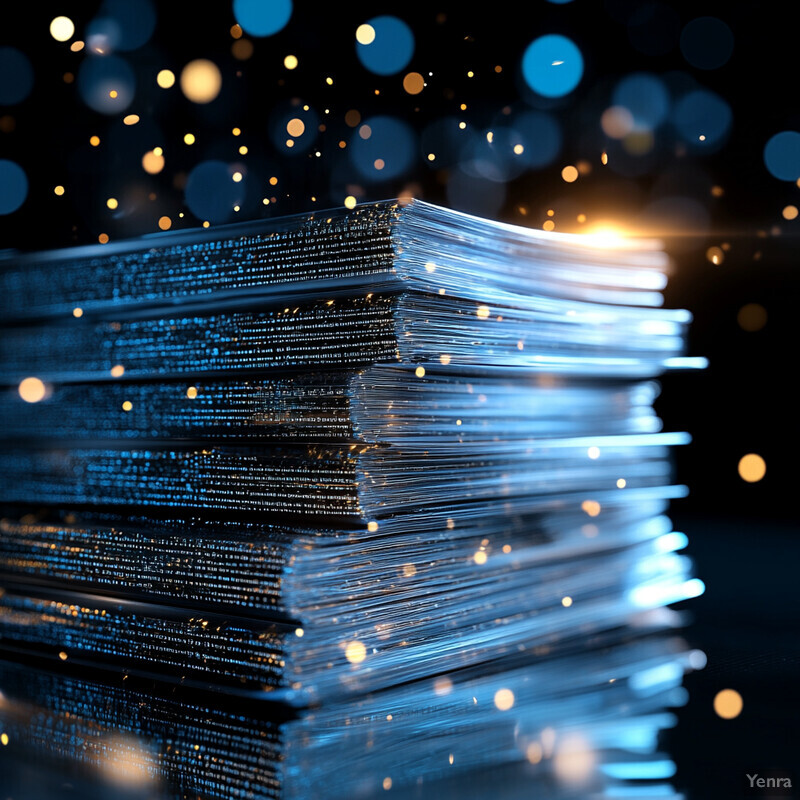
Natural Language Processing (NLP) algorithms simplify the review of extensive documentation associated with EIAs. AI can comb through scientific papers, regulatory texts, technical reports, community feedback, and policy documents, extracting key findings, summarizing complex information, and highlighting compliance issues or knowledge gaps. This capability reduces administrative burdens and human error, ensuring that decision-makers have quick, reliable access to essential information. As a result, stakeholders can spend more time on meaningful evaluation and less time on repetitive data mining, accelerating the EIA review and approval process.
14. Biodiversity Forecasting Under Climate Change
AI models can estimate how species ranges and population sizes might shift in response to a new development under different climate scenarios, guiding more proactive and sustainable planning.
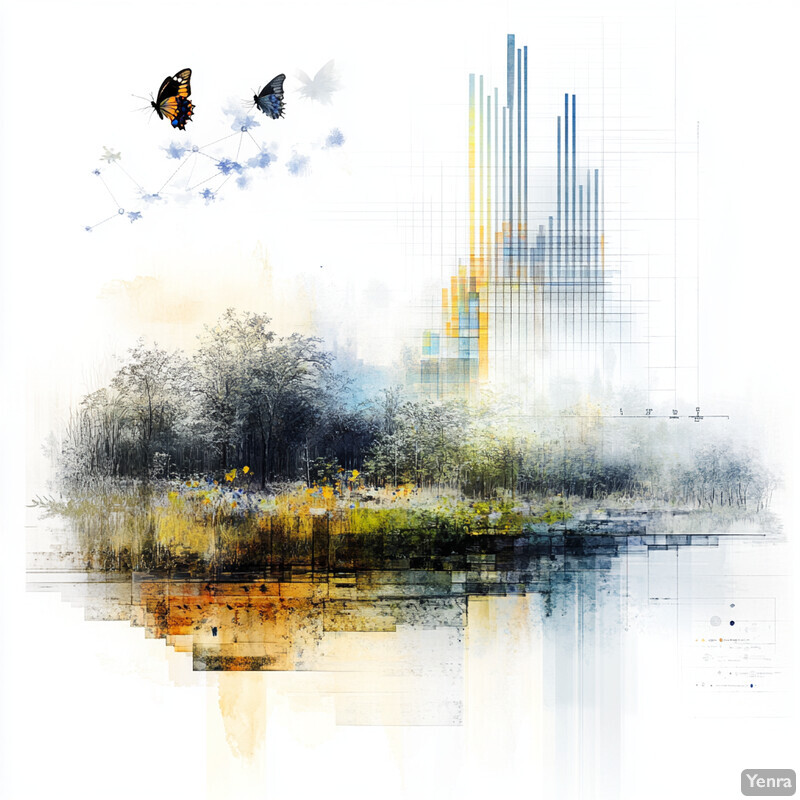
By blending ecological, biological, and climate data, AI models can predict shifts in species distributions, community composition, and overall biodiversity in response to future climatic conditions. These forecasts help EIAs account not only for current environmental baselines but also for how ecosystems might evolve. Understanding future species migrations or the potential decline of certain keystone species informs more adaptive and forward-looking conservation strategies. This proactive approach ensures that development projects incorporate resilience measures to safeguard biodiversity against climate-driven changes.
15. Social-Environmental Data Fusion
AI can incorporate socio-economic indicators (like demographic data, community health data) along with environmental metrics to assess broader socio-environmental implications, leading to more equitable outcomes.
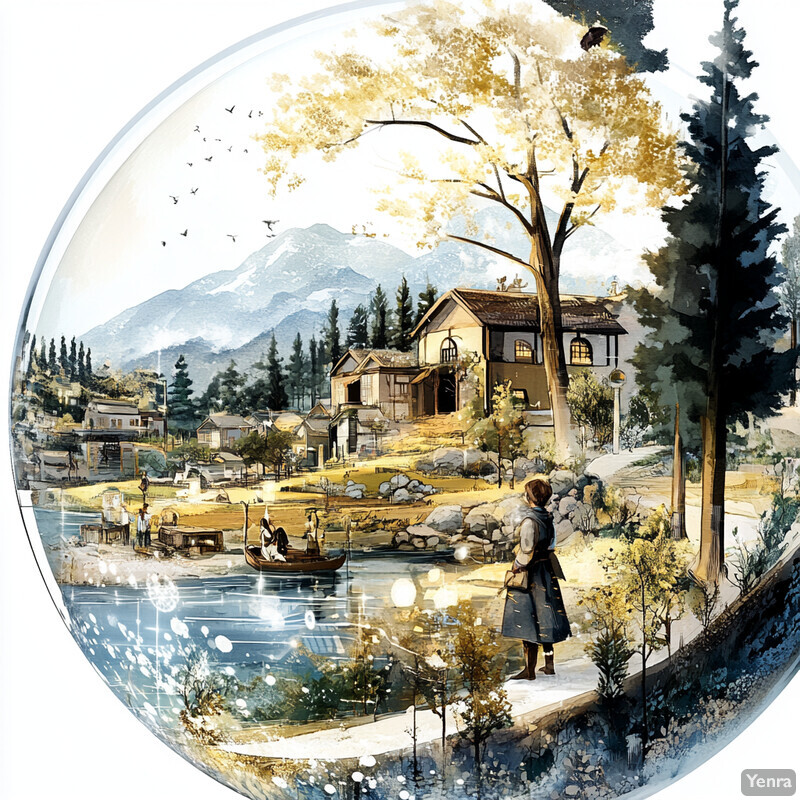
Human and environmental systems are interconnected. AI can integrate socio-economic indicators—like population densities, public health statistics, cultural values, and economic activities—alongside environmental data. This fusion creates more holistic EIAs that consider both ecological and human well-being. By identifying how environmental changes might affect local communities, such as through alterations in resource availability or exposure to pollutants, EIAs can propose strategies that are not only environmentally sustainable but also socially equitable. This ensures that development projects support healthy, resilient communities in harmony with their natural surroundings.
16. Improved Accuracy in Air Quality Predictions
Machine learning models can predict pollutant dispersion patterns, helping identify who and what might be affected by changes in local air quality associated with the proposed project activities.
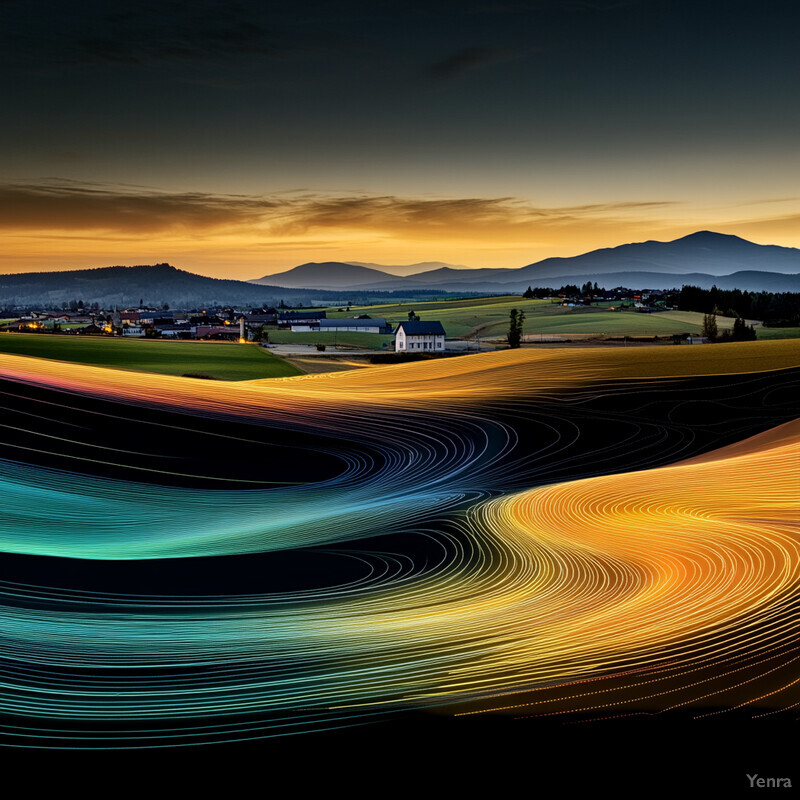
Accurate air quality modeling is vital for assessing the impacts of industrial facilities, infrastructure projects, and transportation expansions. AI-driven predictive models integrate real-time sensor data, historical emission records, meteorological conditions, and geographic characteristics to forecast pollutant dispersion patterns. These forecasts help EIAs identify population centers at risk, evaluate the effectiveness of proposed mitigation measures, and ensure compliance with air quality standards. Over time, improved accuracy in modeling leads to better health outcomes, enhanced regulatory compliance, and more transparent engagement with the public on environmental health matters.
17. Acoustic and Bioacoustic Monitoring
Deep learning applied to audio recordings from project areas can track changes in wildlife activity, migratory bird patterns, or marine mammals’ presence, offering richer insights into the ecological soundscape.
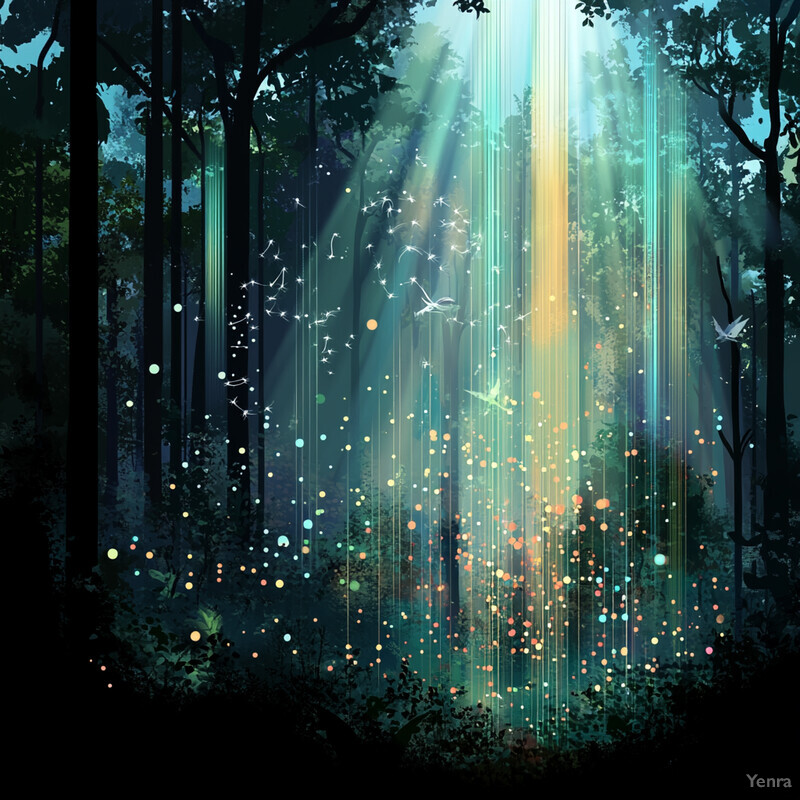
Soundscapes offer valuable insights into ecological health. AI-powered analysis of audio recordings—captured by underwater microphones for marine environments or acoustic sensors in forests—can identify species-specific calls, detect changes in wildlife activity, and even track migratory patterns. Such bioacoustic monitoring helps EIAs assess the presence of sensitive or endangered species, evaluate noise pollution impacts, and gauge ecosystem vitality. By listening in on nature’s soundtracks, stakeholders gain an additional dimension of data to guide more responsible project planning and to maintain the rich acoustic tapestry that many ecosystems rely upon.
18. Reduced Fieldwork Costs and Times
By automating data analysis tasks, AI reduces the need for extensive field surveys, saving time, money, and resources, while still maintaining—or even increasing—data quality and thoroughness.
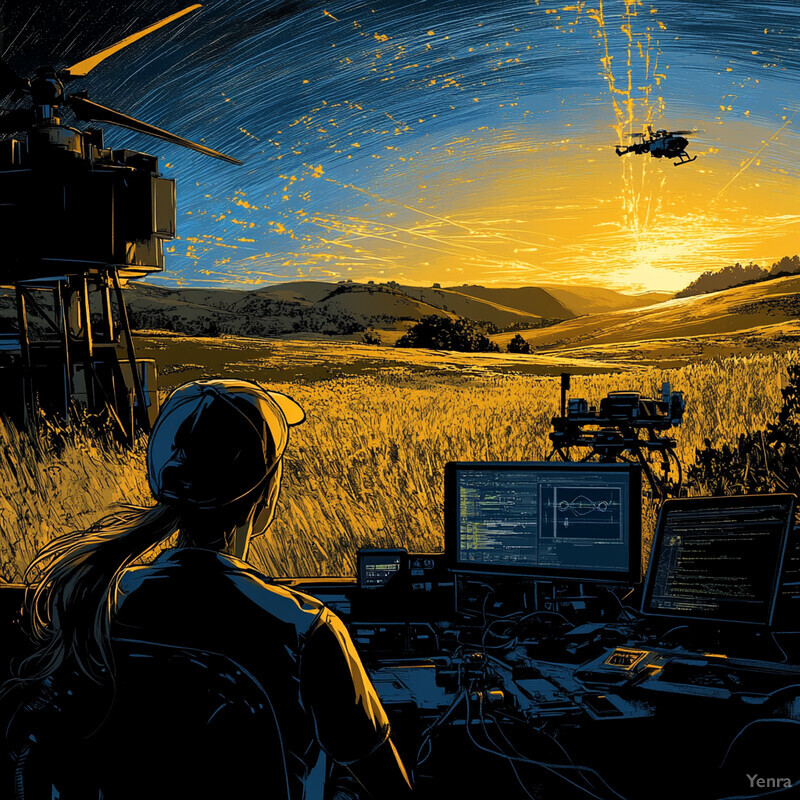
Field surveys are time-consuming, labor-intensive, and often expensive. By automating certain data processing tasks and refining the accuracy of predictive models, AI reduces the need for extensive and repeated on-site inspections. Remote sensing data processed by AI can provide continuous updates, while automated species recognition tools lessen the need for specialized personnel in the field. Although some ground-truthing remains essential, these efficiencies free up resources, making EIAs more cost-effective and accessible. As a result, project proponents can allocate more funding toward mitigation measures, restoration efforts, or enhanced community engagement.
19. Transparent Decision Support Tools
AI-powered dashboards and visualization tools can present complex EIA data in intuitive formats, aiding stakeholders, the public, and regulators in understanding potential impacts and fostering trust.
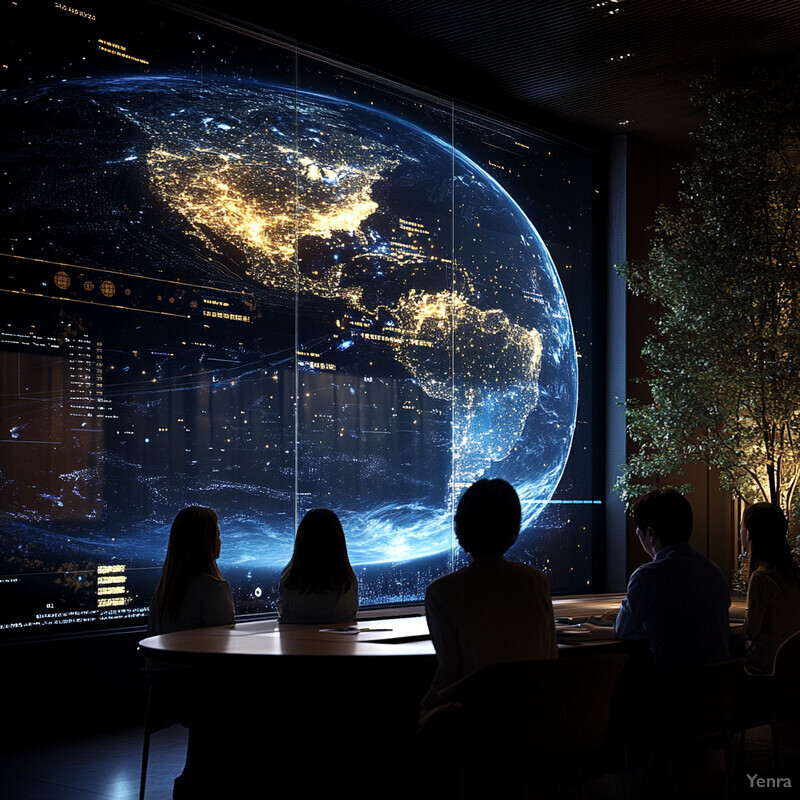
Complex environmental data can be overwhelming for policymakers, stakeholders, and the public. AI-driven visualization and dashboard tools transform raw data into intuitive maps, graphs, and interactive models, making it easier to understand proposed project impacts. This transparency helps build trust among all parties involved—regulatory agencies, communities, NGOs, and project developers—by presenting complex technical analyses in a user-friendly format. Informed and engaged stakeholders are more likely to find common ground, support sustainable outcomes, and contribute constructively throughout the EIA process.
20. Continuous Improvement Through Feedback Loops
As projects evolve, AI models can update impact assessments with new data (e.g., from sensors or ongoing monitoring), creating adaptive EIAs that evolve over time to reflect real-world conditions and effectiveness of mitigation measures.
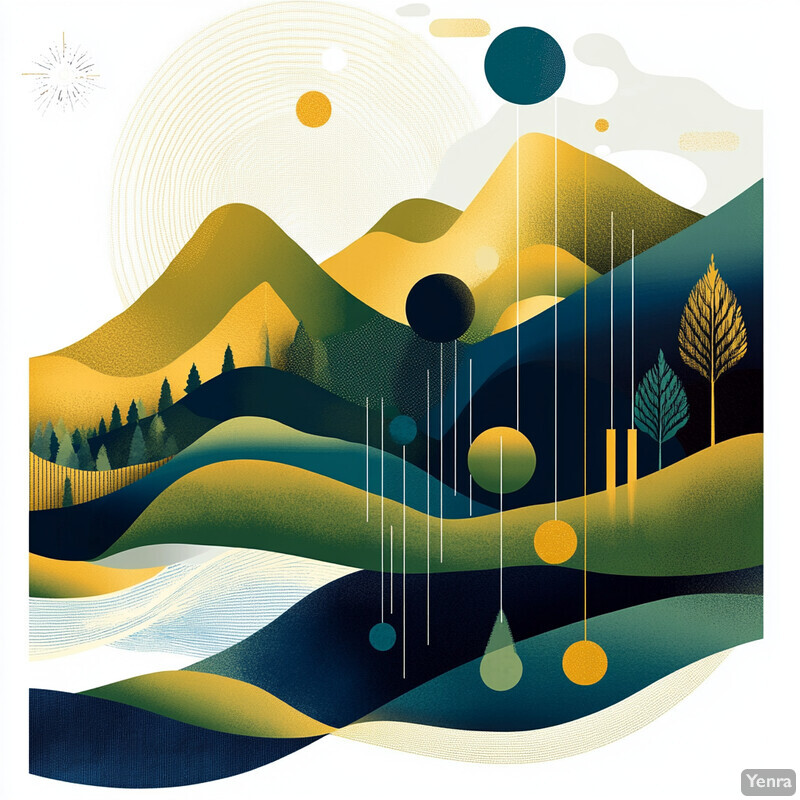
AI-based EIAs are not static documents; they are dynamic systems that evolve as new data and insights come to light. Machine learning models can incorporate feedback from ongoing monitoring, updated climate projections, or observed changes in species populations. These continuous feedback loops keep impact assessments relevant, ensuring that any unexpected outcomes—such as unforeseen species declines or pollutant leakage—can be quickly addressed. This adaptive approach moves EIAs from one-time evaluations toward living tools that guide long-term environmental stewardship and sustainable project management.