1. Automated Data Classification
AI-driven tools can automatically identify and classify data according to sensitivity and compliance requirements (e.g., identifying personally identifiable information or protected health information), thereby streamlining data governance processes.
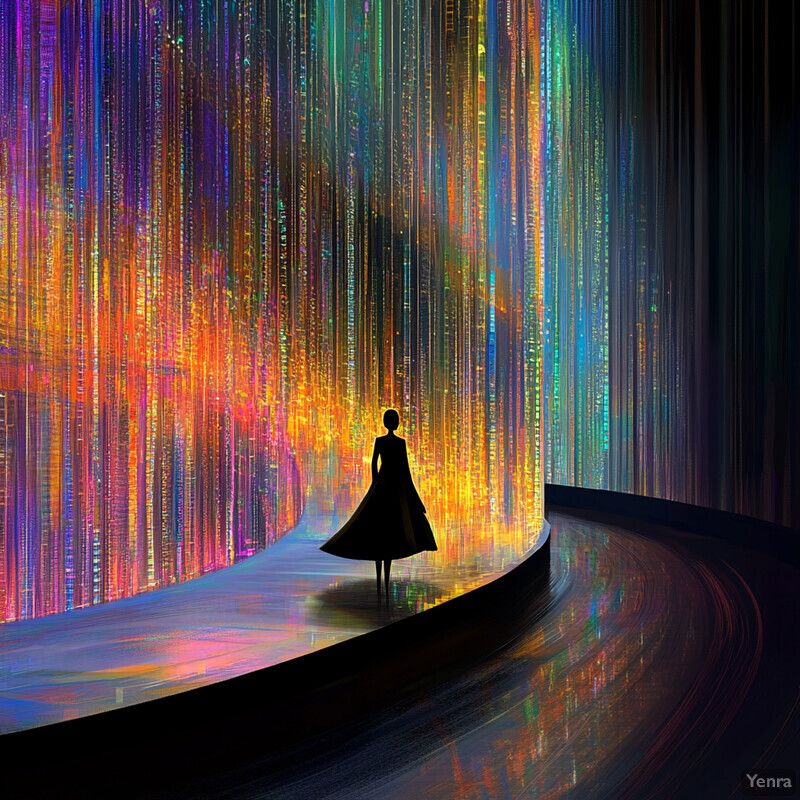
AI-driven data classification tools leverage machine learning algorithms to sift through vast amounts of organizational data—both structured and unstructured—and categorize it based on sensitivity levels and regulatory requirements. By continually learning from patterns, these systems can distinguish personally identifiable information (PII) or protected health information (PHI) from general business data with increasing accuracy. This automated classification minimizes human error, reduces the time spent on manual labeling, and ensures that sensitive data is appropriately flagged for protections like encryption or access restrictions. Ultimately, this supports a more robust data governance framework, enabling organizations to maintain compliance more easily while reducing operational costs and risks associated with mismanaged data.
2. Sensitive Data Redaction
Natural Language Processing (NLP) models can detect and anonymize sensitive information in documents, ensuring that only privacy-compliant data is shared or stored, reducing the risk of inadvertent data leaks.
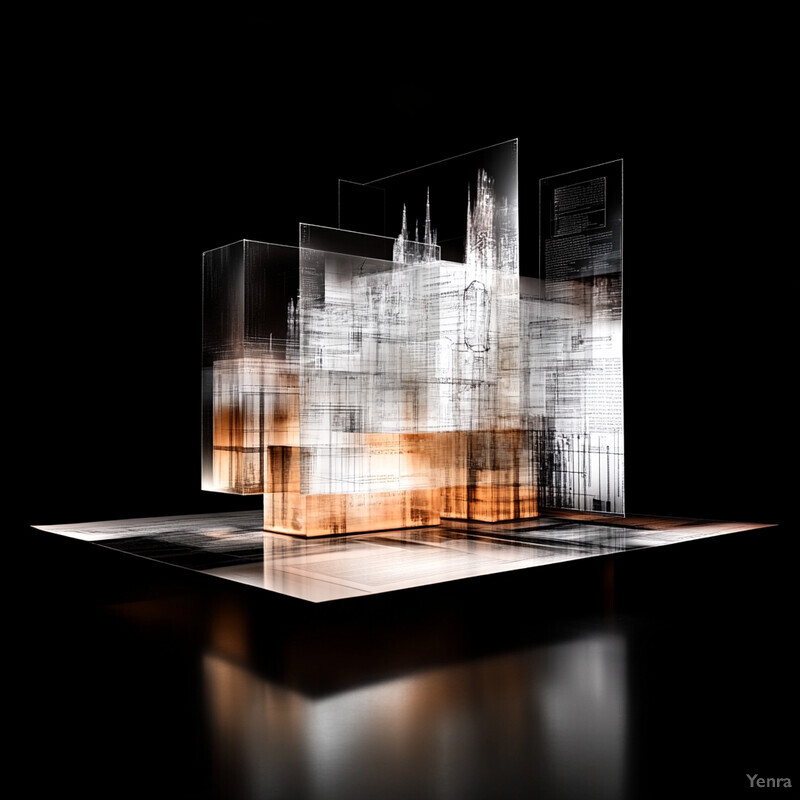
Natural Language Processing (NLP) models and other advanced AI techniques can scan through unstructured text—such as documents, emails, and recorded transcripts—and automatically identify content that might violate privacy regulations. This includes names, social security numbers, credit card details, and other identifiable elements. Once identified, these tools can effectively obscure or remove this information, ensuring that any data leaving secure environments is appropriately sanitized. This automated redaction process not only helps organizations comply with data protection regulations like the GDPR or HIPAA but also streamlines workflows, allowing data sets to be safely shared for analytics, research, or other legitimate business purposes without endangering individuals’ privacy.
3. Adaptive Access Control
Machine learning algorithms can dynamically adjust user access rights based on behavioral patterns, context, and risk scoring, ensuring that sensitive data is only accessible under secure and compliant conditions.
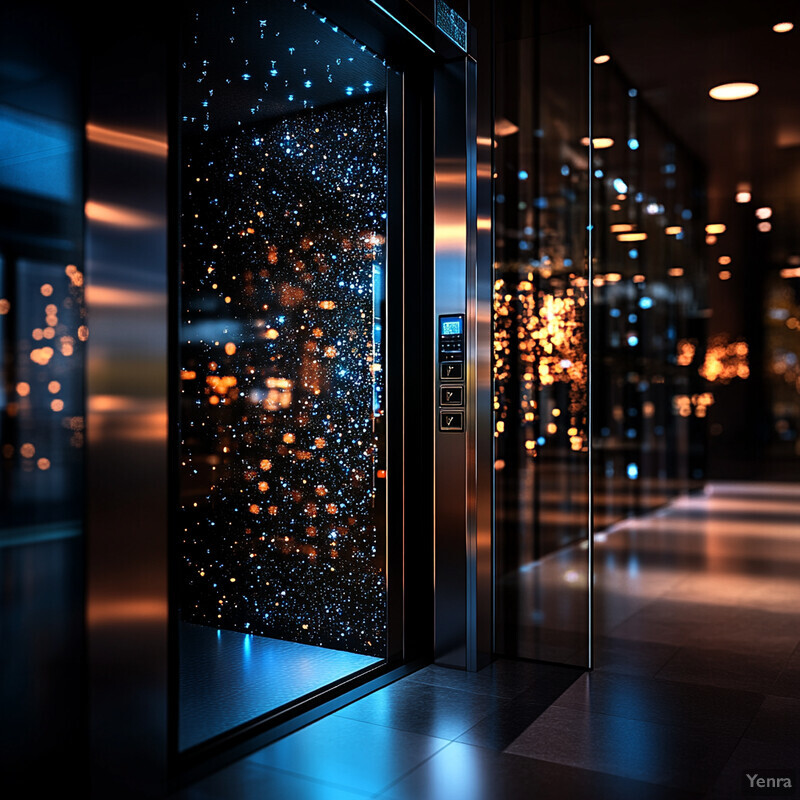
Modern AI-based access control systems go beyond static user roles and permissions by employing continuous risk assessment. They analyze behavioral patterns, user context, device reputation, and real-time network conditions to determine whether granting access to sensitive data is justified at that moment. For example, if a user who typically accesses a database from a secure office network suddenly attempts to log in from a suspicious IP address at an unusual time, the system may require additional authentication or block access. This adaptive approach to permissions enhances data privacy by ensuring only the right individuals access the right data under the right conditions, directly supporting compliance mandates that require strict control of sensitive information.
4. Real-Time Privacy Policy Enforcement
AI can continuously monitor data flows in real-time, automatically blocking or flagging activities that violate privacy and regulatory policies before a breach or compliance incident occurs.
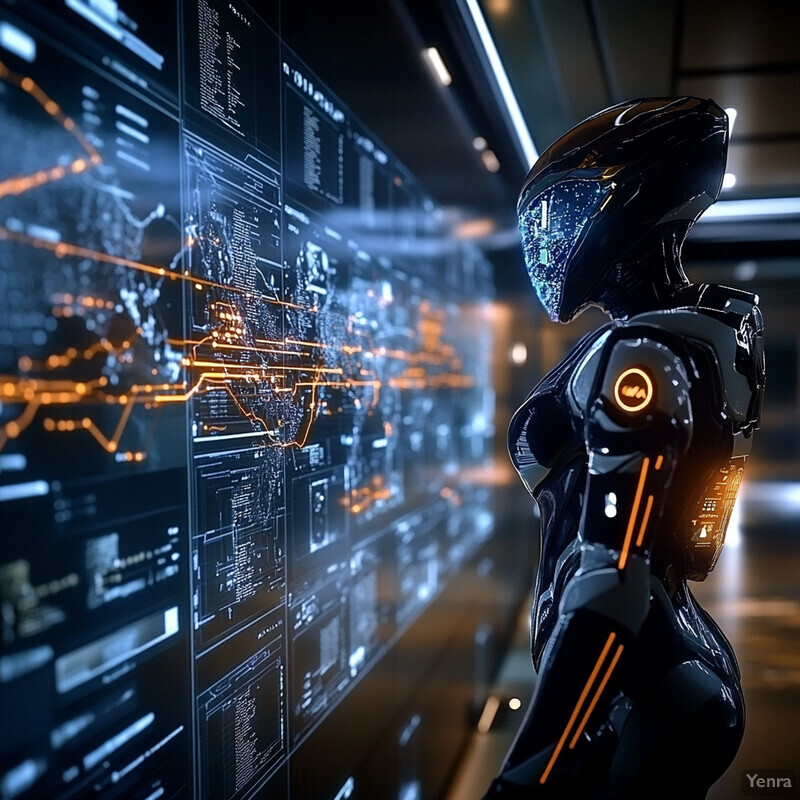
AI-driven compliance engines can continuously monitor network traffic, database queries, and user activities against established privacy and data protection policies. As these tools operate in real-time, they can immediately flag—or even automatically block—policy violations before sensitive data is improperly shared or exposed. By doing so, organizations can enforce compliance requirements proactively rather than relying on reactive audits. This kind of enforcement reduces the window of opportunity for data breaches, helps maintain strict adherence to regulatory frameworks (like CCPA or GDPR), and offers clear audit trails that demonstrate an organization’s proactive stance on privacy enforcement to regulators and stakeholders.
5. Automated Compliance Reporting
AI-based systems can generate detailed compliance reports and audit trails with minimal human intervention, reducing manual overhead and increasing accuracy in demonstrating adherence to regulations.
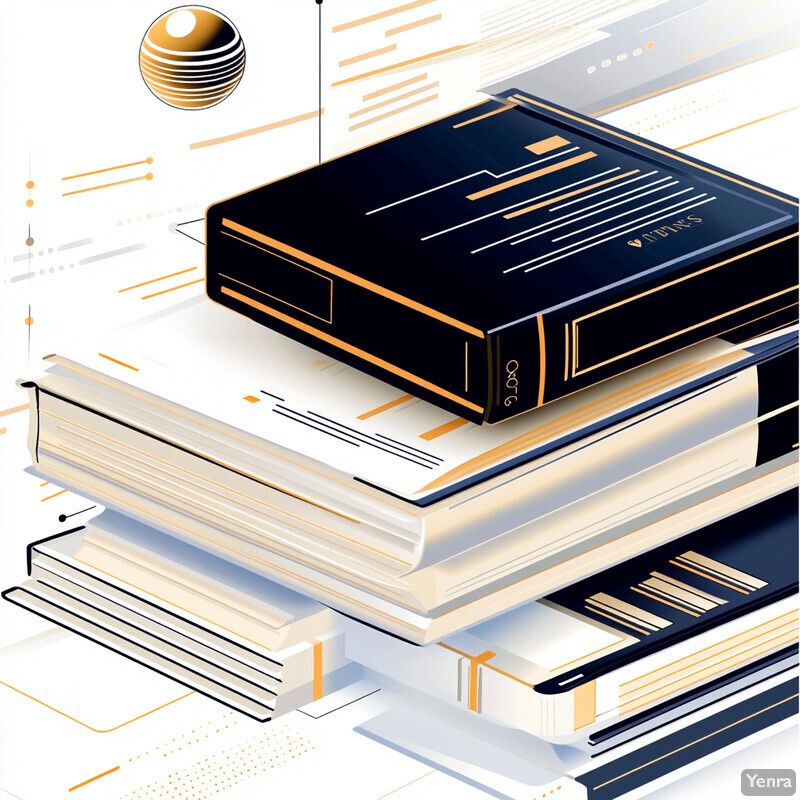
Traditionally, compliance reporting involves manual compilation of records, audit logs, and user activities—a tedious and error-prone process. AI-based tools simplify this by automatically aggregating and analyzing relevant data, generating comprehensive compliance reports that highlight adherence to key regulations, potential policy violations, and remediation actions taken. These reports can be customized to meet specific regulatory standards and can be generated on-demand or scheduled regularly. As a result, organizations gain a transparent, auditable record of their compliance posture, reducing the administrative burden and human effort required, while also improving the accuracy and reliability of compliance documentation.
6. Proactive Data Breach Detection
By analyzing network traffic, user behavior, and system logs, AI systems can detect anomalous patterns indicative of potential breaches earlier, limiting unauthorized data exposure and aiding compliance.
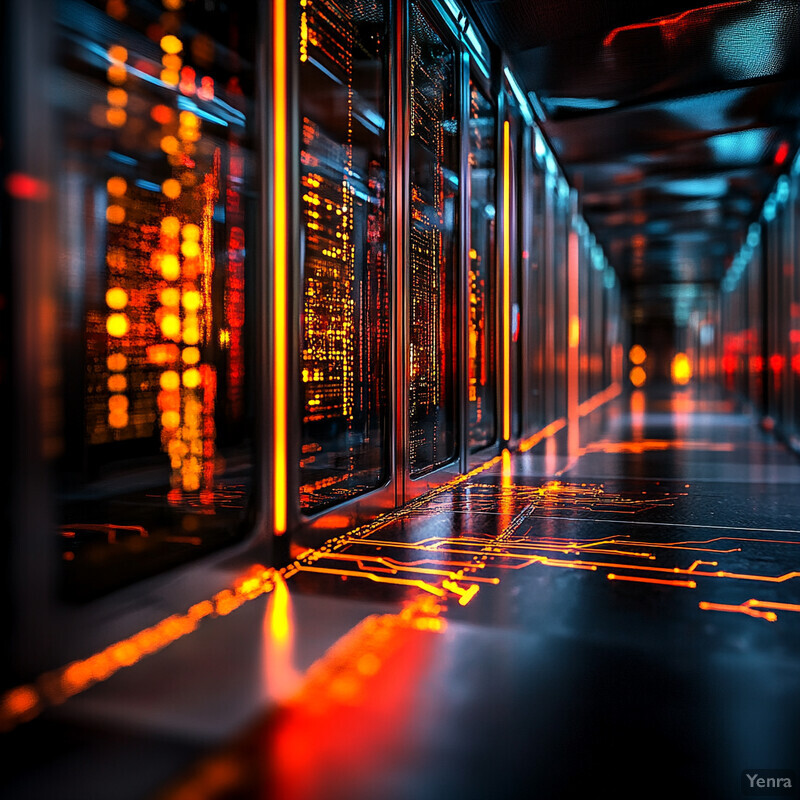
AI enhances data security and compliance by detecting unusual patterns in data access and system usage that could indicate a breach or malicious activity. These machine learning models are trained to differentiate between normal user behavior and anomalies that might represent unauthorized access attempts, insider threats, or data exfiltration. By identifying these signs early, organizations can intervene before significant damage occurs. This early-warning capability is instrumental in maintaining compliance with regulations that require timely breach notifications and minimized data exposure. Proactive detection not only helps avoid the hefty fines and reputational damage associated with breaches but also assures regulators and customers that privacy safeguards are continuously monitored and improved.
7. Context-Aware Consent Management
AI can interpret different legal jurisdictions and data usage scenarios to ensure that consent gathering and usage align with regulations like GDPR, automatically updating consent forms or prompting for re-consent when needed.
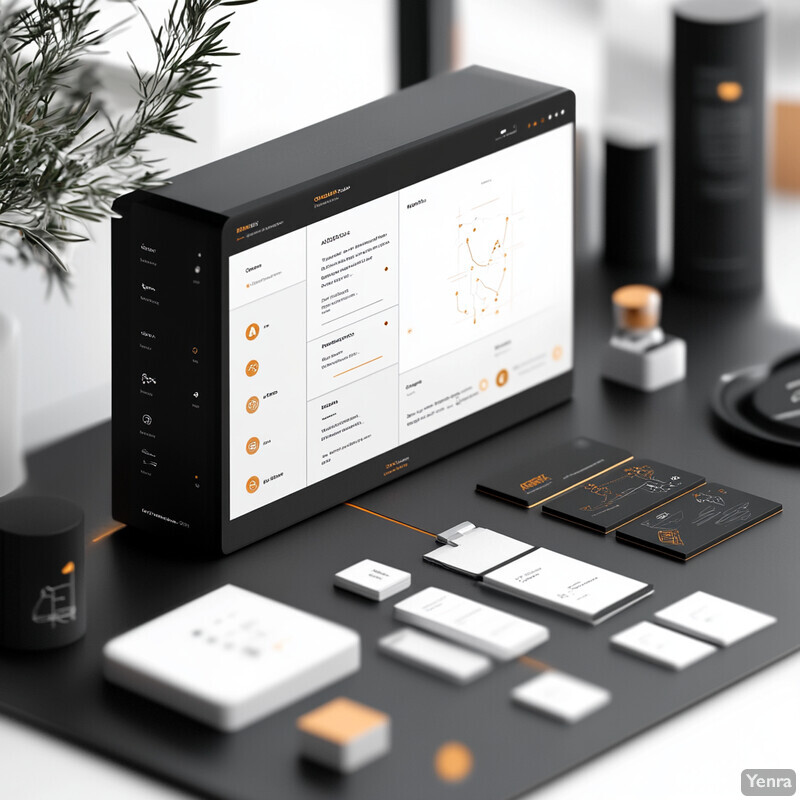
Consent requirements differ based on jurisdiction, the nature of collected data, and the intended use of that data. AI-driven systems help organizations navigate these complexities by dynamically determining when and how user consent should be obtained or refreshed. They consider factors like local regulations, user preferences, and historical user interaction patterns to present contextually appropriate consent notices. This ensures that data collection and usage always align with relevant laws—such as GDPR’s explicit and informed consent mandates—and that user rights are respected. With automated consent management in place, organizations maintain a consistent and compliant approach to data handling, building trust with their users and regulatory bodies alike.
8. Privacy-by-Design Recommendations
During software development, AI-powered code analysis tools can identify privacy risks and suggest design modifications that adhere to best practices and regulatory guidelines, embedding compliance early in the lifecycle.
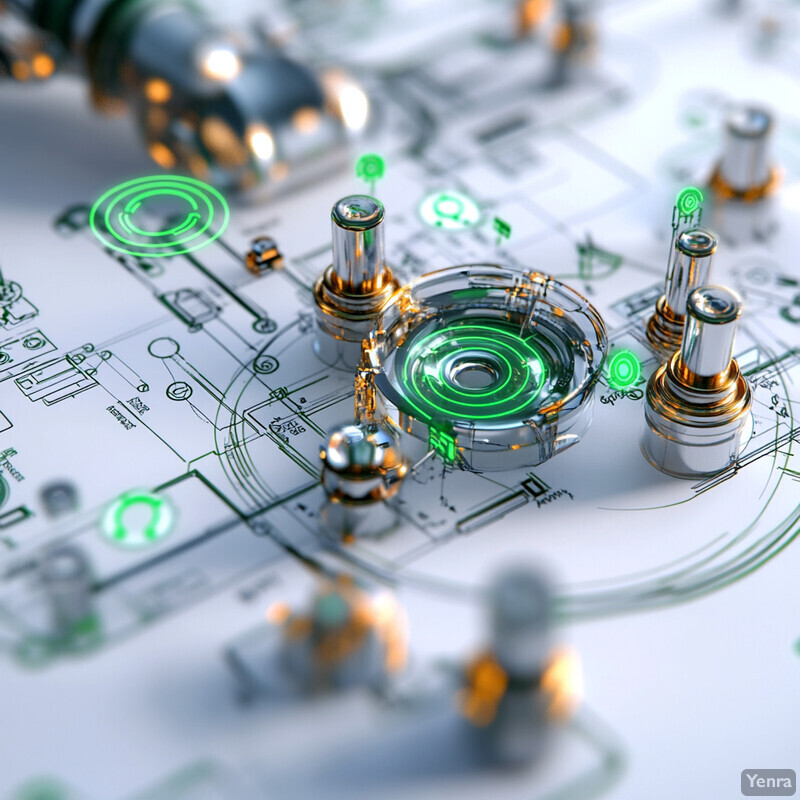
Incorporating privacy considerations from the start of the software development lifecycle is a core principle of modern data protection frameworks. AI-powered code analysis and architectural evaluation tools can identify potential privacy risks in software designs and offer recommendations to mitigate them before deployment. These tools might suggest encrypting certain data fields, prompting pseudonymization of sensitive attributes, or advising adjustments to data retention strategies. By injecting privacy best practices early in development and continually refining these approaches with feedback loops, organizations reduce the likelihood of future compliance issues and make it simpler to maintain strong data protection postures over time.
9. Risk Scoring and Prioritization
Machine learning models can assess the potential privacy risk of various data-processing activities, helping organizations prioritize resources for the most vulnerable areas and ensure compliance with evolving regulations.
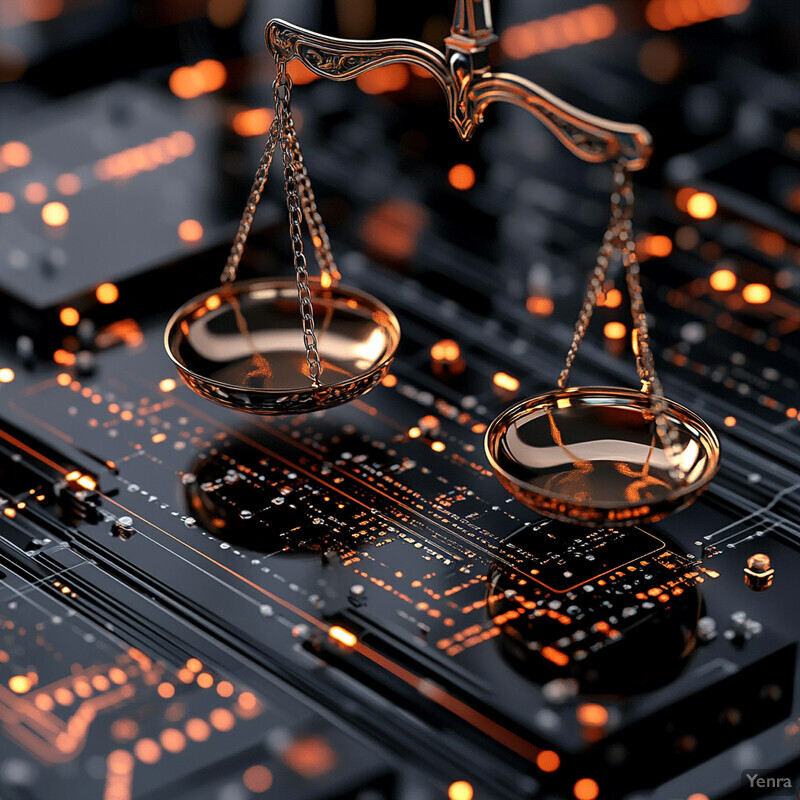
One of the most challenging aspects of data governance and compliance is determining which processes, datasets, or applications pose the highest privacy risks. AI-based risk scoring models evaluate factors like data sensitivity, access frequency, external sharing, and past incidents to assign risk scores to data assets or activities. Armed with these insights, compliance officers can prioritize their efforts, focusing resources on the most vulnerable or impactful areas. This data-driven prioritization ensures that organizations address compliance gaps efficiently and effectively, improving their overall data protection strategy and aligning their controls with the highest return on privacy investment.
10. Automated Data Minimization
AI-driven systems can identify redundant or unnecessary data and recommend safe deletion or sanitization strategies, ensuring organizations handle only the minimal amount of personal data required by law.
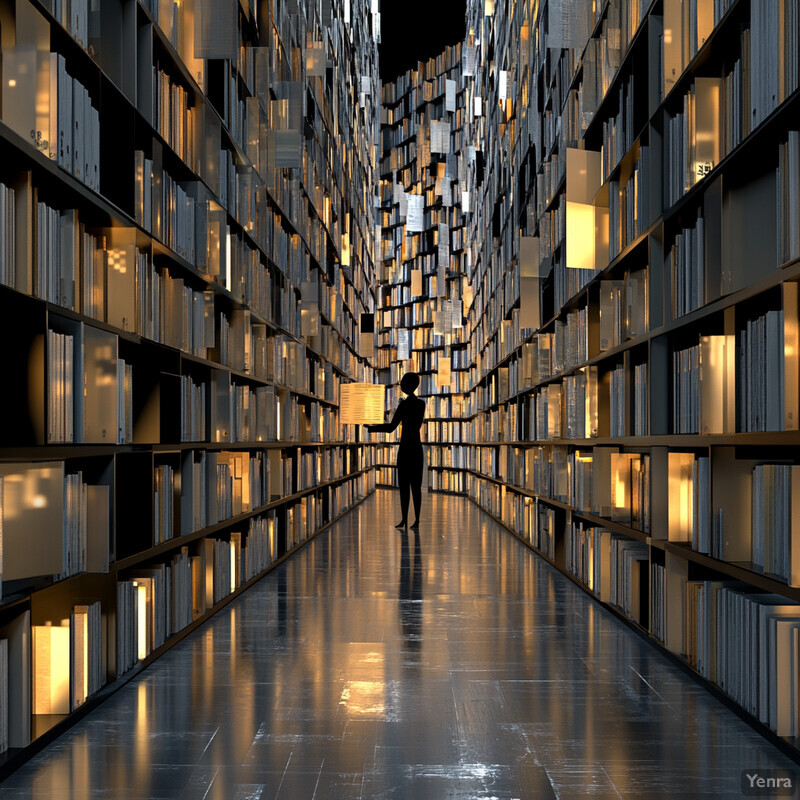
Data protection regulations often emphasize the principle of data minimization, requiring that organizations only collect and retain the minimal amount of personal data needed to achieve their purposes. AI-driven tools can examine storage systems, identify redundant or outdated data, and recommend safe deletion or aggregation methods. These recommendations help organizations avoid unnecessary data retention, reducing the attack surface and compliance risks associated with holding excessive personal information. Automated data minimization not only aligns with legal obligations like GDPR’s “data minimization” principle but also streamlines database management and lowers storage costs over the long term.
11. Synthetic Data Generation for Compliance Testing
AI can generate synthetic, privacy-preserving datasets that mirror the properties of real data without containing identifiable information, supporting compliance-friendly testing and analytics.
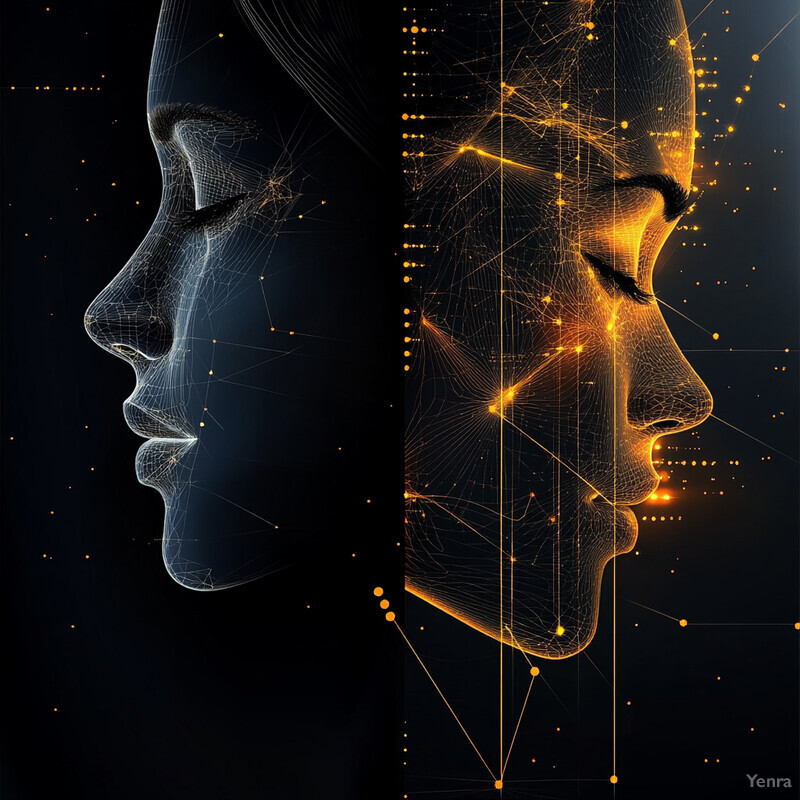
For testing analytics models, product features, or new data-driven services, developers often need access to realistic data. However, using real customer data in testing environments can raise privacy risks. AI-fueled synthetic data generation creates artificial datasets that closely resemble the statistical properties of genuine data—without including identifiable information. This ensures developers, data scientists, and quality assurance teams can perform robust testing, training, and validation without exposing sensitive information. By preserving realism while protecting privacy, synthetic data supports compliance in scenarios where the use of live data would otherwise jeopardize privacy regulations.
12. Support for Regulatory Updates
AI models can track global regulatory changes, interpret the implications, and suggest updates to privacy policies and compliance frameworks, aiding organizations in remaining compliant as laws evolve.
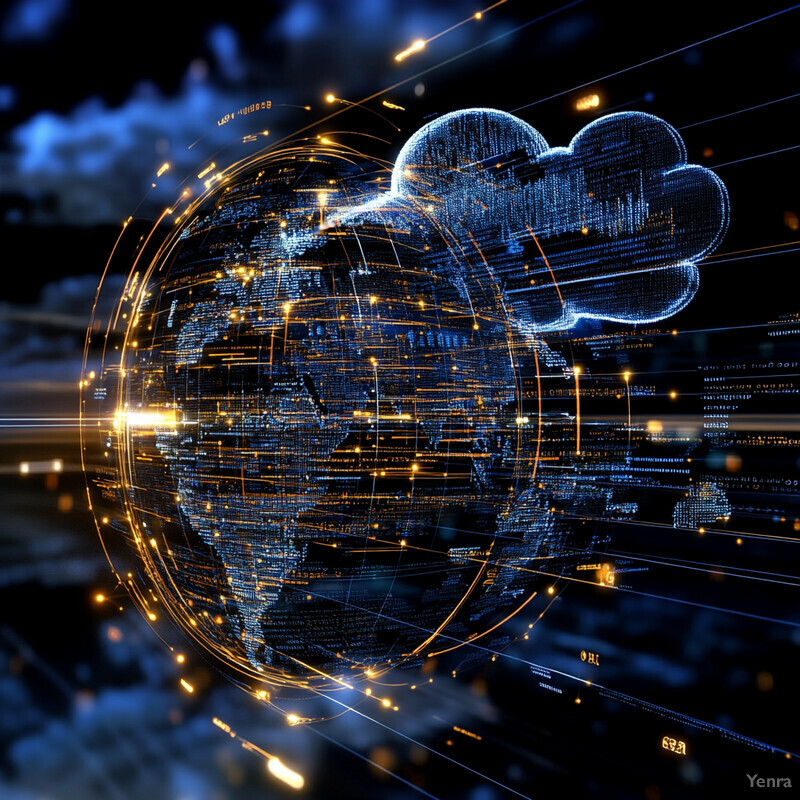
The legal landscape surrounding data privacy is dynamic and ever-evolving. AI tools can regularly scan legal repositories, regulatory bulletins, and official announcements across multiple jurisdictions to track changes in compliance requirements. By interpreting these regulations and mapping them to current policies and workflows, the AI can suggest necessary adjustments—such as updating privacy notices, altering data retention schedules, or refining consent mechanisms. This ongoing vigilance helps organizations remain compliant even as new laws come into effect, mitigating the risk of penalties and ensuring that data-handling practices remain in harmony with the latest standards.
13. Deepfake and Identity Fraud Detection
Advanced AI models can identify manipulated content, fraudulent activity, or impersonation attempts, maintaining data integrity and compliance with identity verification mandates.
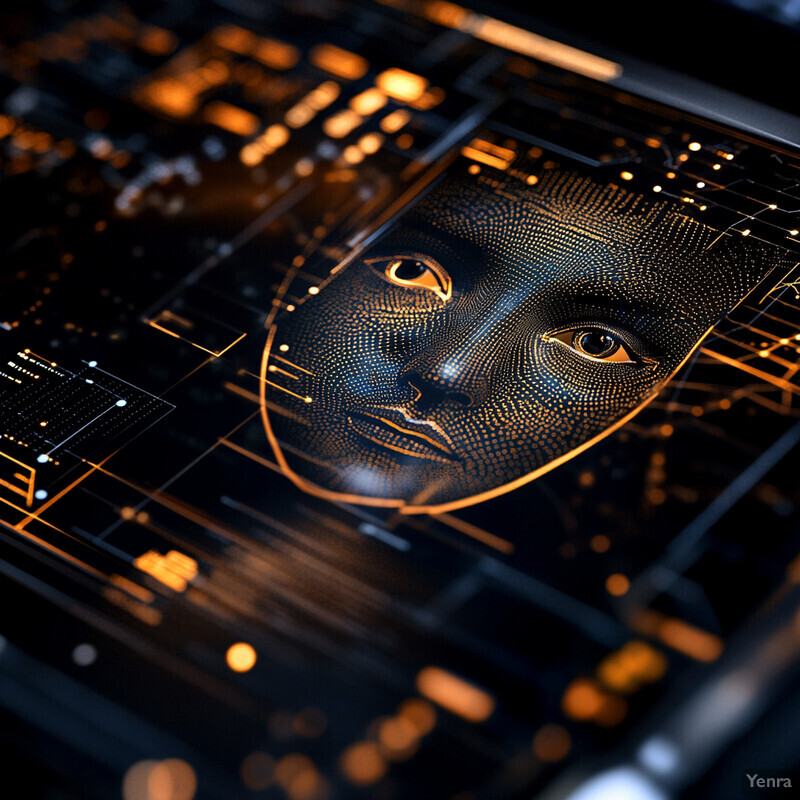
As the sophistication of deepfakes and impersonation attacks grows, so does the need for advanced defenses. AI-based detection systems analyze subtle visual, audio, and behavioral cues to identify manipulated content or fraudulent activities. By preventing identity fraud, impersonation, and malicious manipulation, these tools help maintain data integrity and user trust. Compliance frameworks often mandate strong identity verification and secure access controls, and AI-driven deepfake detection strengthens these compliance measures by ensuring that only legitimate, verified users can interact with sensitive systems and data.
14. Integrated Data Encryption and Tokenization Advice
AI-driven recommendations help determine the appropriate level and method of data encryption or tokenization, ensuring compliance with data protection standards and reducing the risk of exposure.
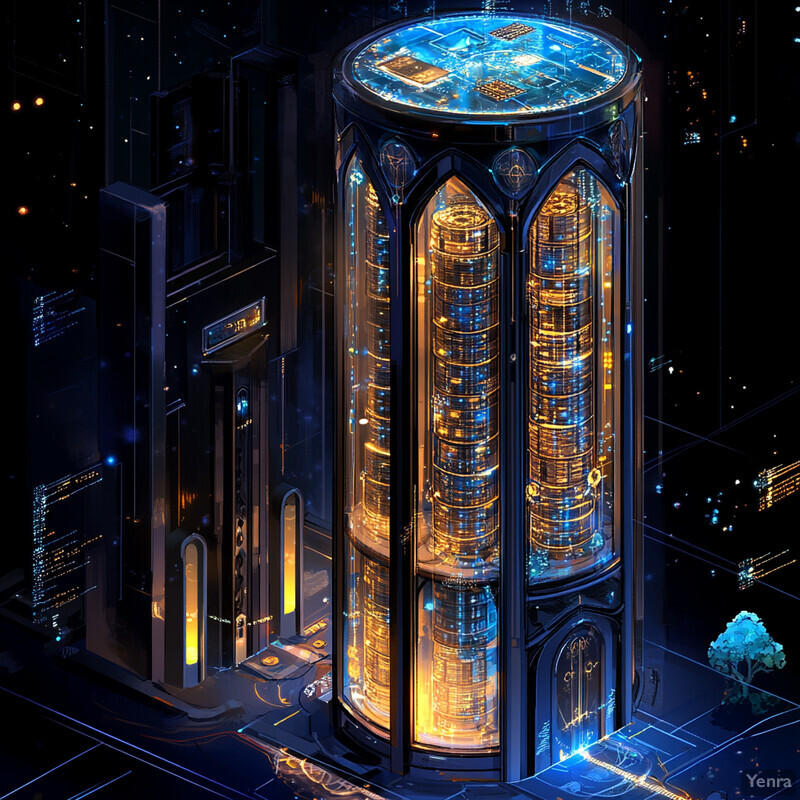
Deciding how to protect data—whether through encryption, tokenization, or anonymization—can be complex. AI advisory systems can evaluate data sensitivity, regulatory mandates, and environmental constraints to recommend the appropriate level and method of data protection. They may suggest strong encryption algorithms for especially sensitive data or tokenization strategies for data that must remain partially identifiable for certain workflows. By aligning encryption and tokenization approaches with regulatory requirements and best practices, these AI systems help organizations implement robust security and privacy controls that stand up to audits and reduce compliance headaches.
15. Automated Vendor Risk Management
AI systems can evaluate third-party vendors’ privacy practices, detect possible compliance issues, and continuously monitor vendors to ensure they maintain appropriate privacy standards over time.
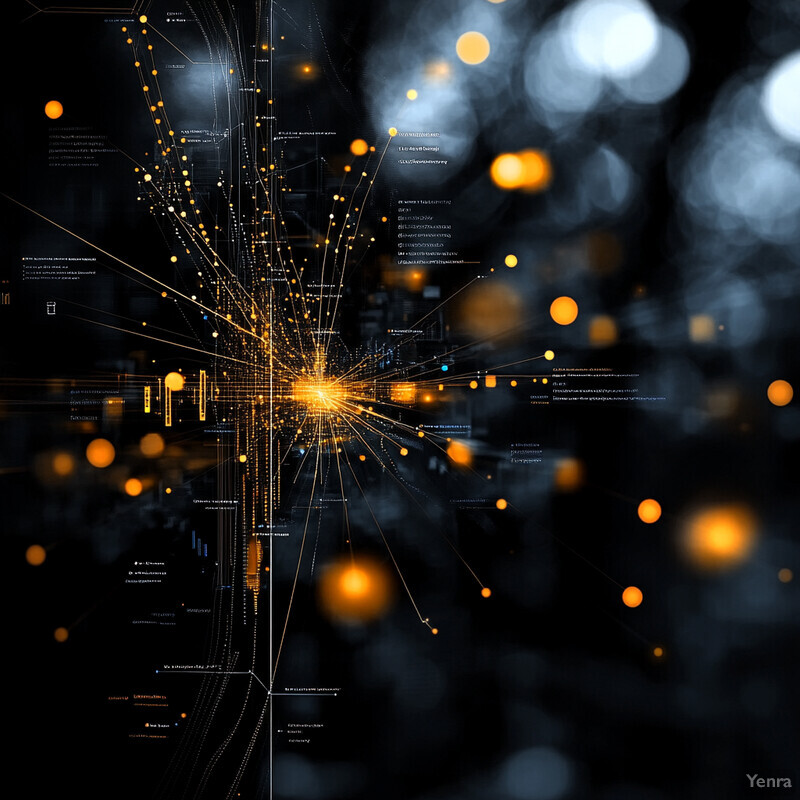
Many organizations outsource data-processing tasks to third-party vendors, creating extended ecosystems of compliance obligations. AI-driven vendor risk management platforms continuously monitor these vendors’ cybersecurity posture, regulatory adherence, and incident history. If a vendor’s risk profile changes—due to a discovered vulnerability or a known compliance violation—the AI system can alert compliance officers who can take timely action, such as renegotiating terms, requesting remediation steps, or even terminating the contract. By proactively identifying and mitigating vendor-related risks, organizations can maintain strong compliance postures and protect their supply chains from data breaches and regulatory penalties.
16. Behavioral Analytics for Insider Threats
By analyzing user activities for unusual behavior, AI-based monitoring can alert compliance officers to potential insider threats to data privacy, allowing for timely intervention and reducing regulatory risks.
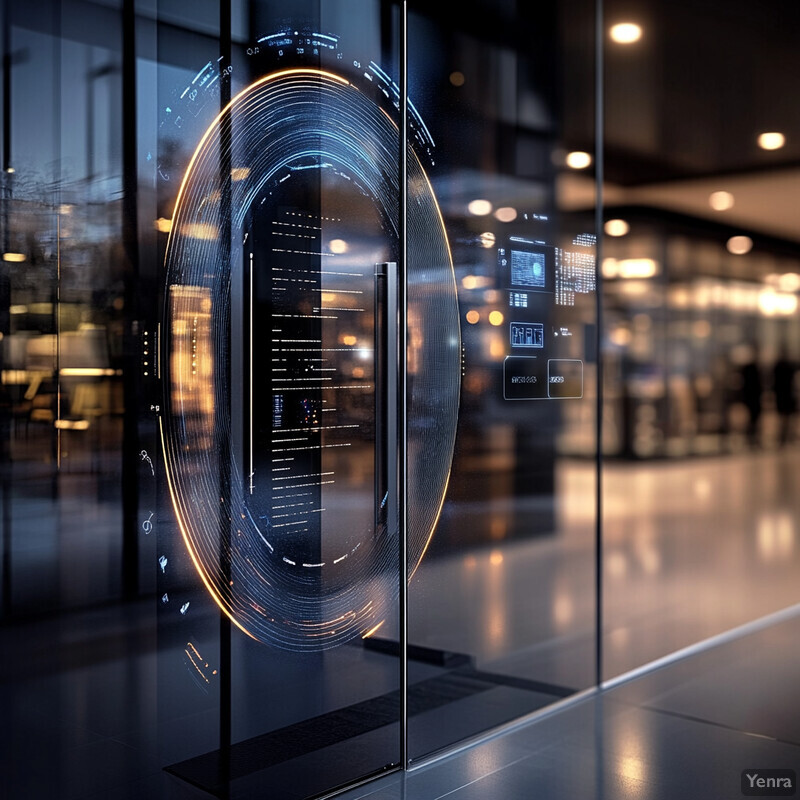
Insider threats—where employees or contractors misuse their legitimate access—pose a significant compliance risk. AI-based behavioral analytics tools scrutinize user activities, looking for suspicious patterns such as unusual login times, abrupt spikes in data downloads, or attempts to access previously untouched sensitive files. By correlating these anomalies with established norms, AI systems can alert compliance and security teams to potential insider threats. Early detection of such activities prevents unauthorized data exposure, reduces the risk of regulatory non-compliance, and ensures that only authorized, trustworthy individuals can work with sensitive information.
17. Language and Jurisdictional Variance Handling
NLP and other AI models can parse legal texts in multiple languages, adapting compliance strategies to local data protection laws, ensuring that global organizations can maintain compliance everywhere they operate.
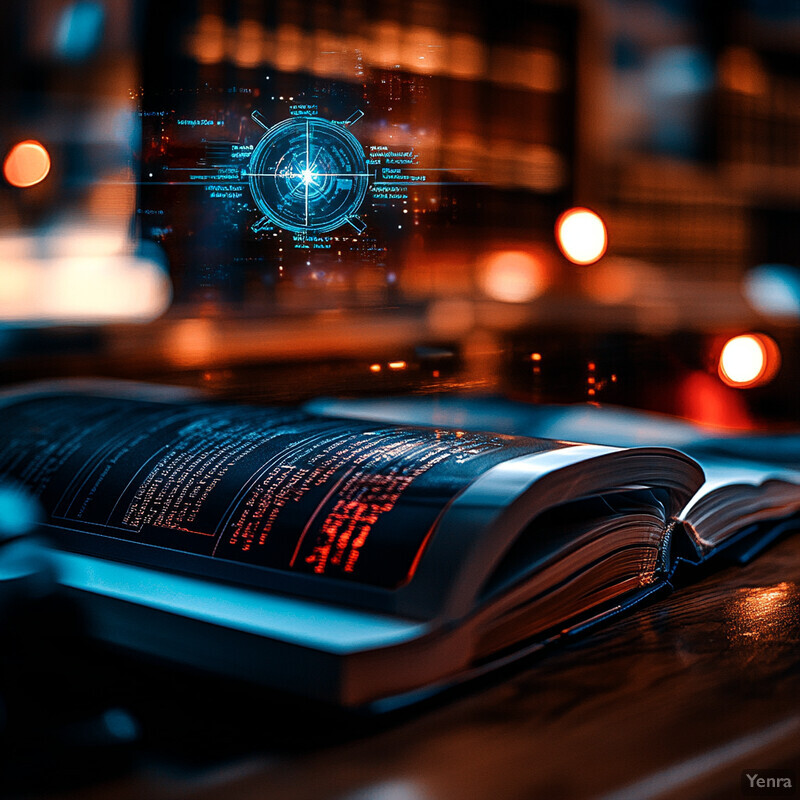
Global organizations must navigate a patchwork of data protection laws that vary by country, region, and even industry. NLP-based AI systems can parse legal texts and guidance in multiple languages, automatically mapping them to an organization’s data policies. By harmonizing these diverse requirements, the AI tools help maintain consistent compliance across borders. This level of nuance ensures that data-handling practices respect local privacy rights, consent rules, and reporting obligations, thereby preventing costly legal disputes and allowing multinational entities to operate with confidence in multiple jurisdictions.
18. Enhanced Incident Response
When a privacy breach occurs, AI can automate parts of the incident response—prioritizing tasks, suggesting remediation steps, generating required notifications to regulators, and documenting the process for audits.
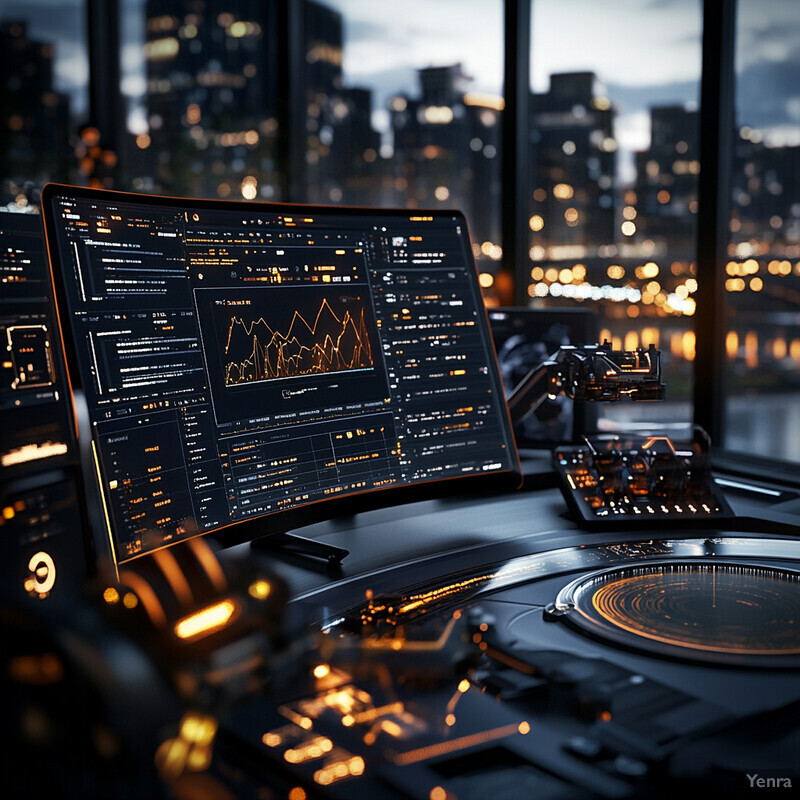
When a data breach or privacy incident occurs, swift and structured action is critical to meet compliance obligations and mitigate damage. AI-supported incident response platforms streamline this process by analyzing the nature of the breach, identifying affected data, recommending immediate containment steps, and suggesting communication strategies for notifying regulators and impacted individuals. By optimizing the order and urgency of response activities, these systems reduce the time it takes to restore compliance and trust. Detailed audit trails of the incident response process further assure regulators that the organization managed the incident responsibly and transparently.
19. Improved Employee Training and Education
AI-driven personalized learning platforms can tailor privacy and compliance training modules to individual employee roles and knowledge gaps, ensuring the workforce is up-to-date with the latest regulations.
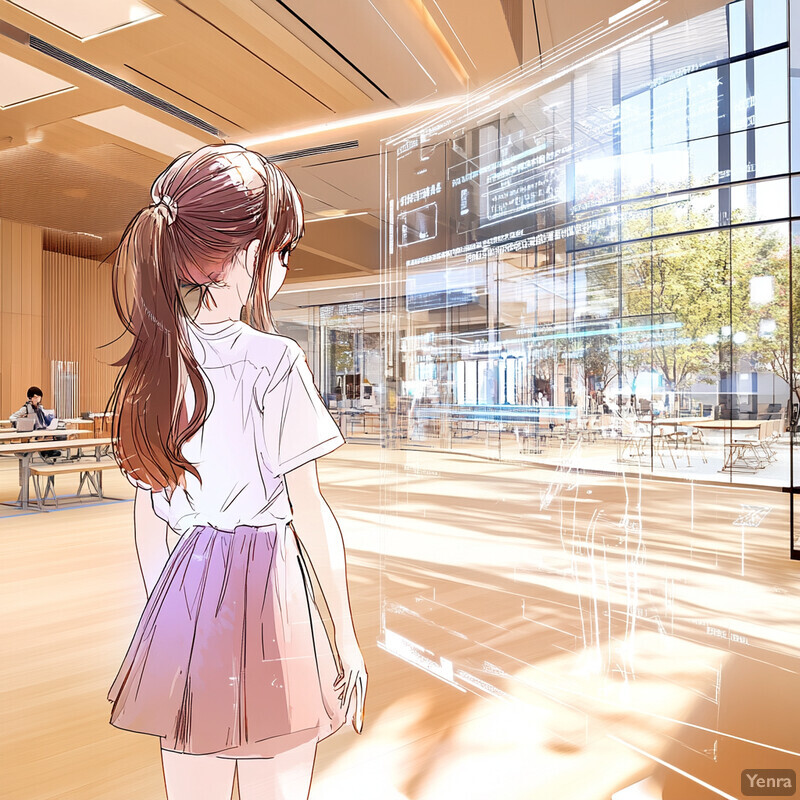
Human errors remain a leading cause of data breaches and compliance failures. AI-driven training programs customize educational modules based on an employee’s role, past performance in compliance quizzes, and observed behavior (e.g., frequency of clicking suspicious links). These adaptive learning systems ensure that each team member gains a strong understanding of the latest data privacy policies and best practices, while also reinforcing areas of weakness. As employees become more knowledgeable and vigilant, the organization’s overall compliance posture improves, reducing the risk of accidental data leaks and regulatory violations.
20. Continuous Improvement Through Feedback Loops
AI systems can gather outcomes from audits, investigations, and reported incidents, then refine models and rules to improve future detection, prevention, and compliance strategies, creating a virtuous cycle of advancement in data privacy.
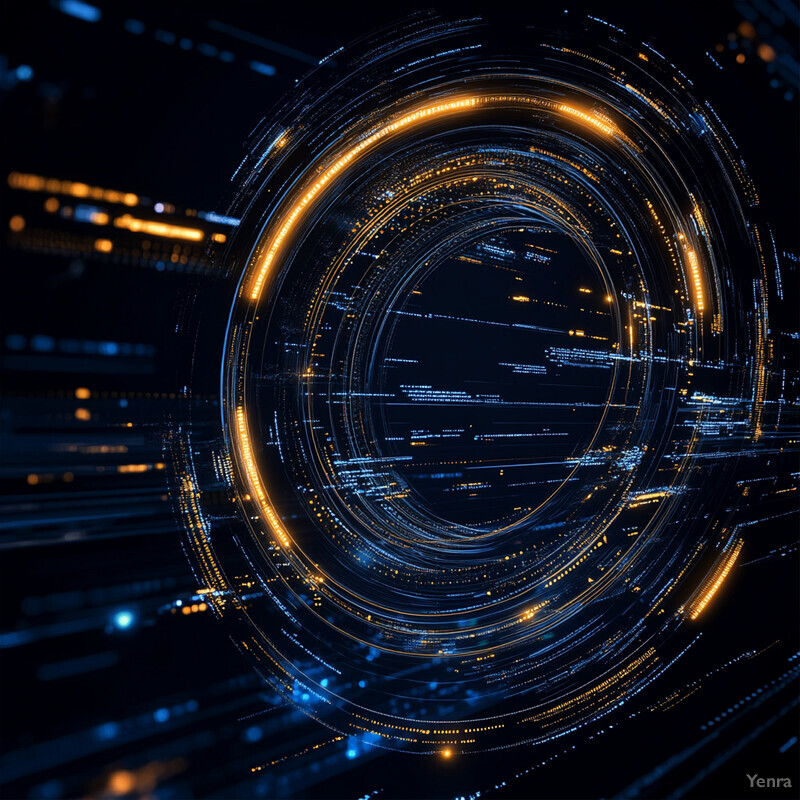
One of the great strengths of AI is its capacity to learn from experience. Compliance and privacy tools can integrate feedback from audits, investigations, and post-incident analyses directly into their models. This creates a virtuous cycle: each compliance review, breach investigation, or regulatory update informs the AI’s decision-making processes and detection strategies. Over time, the system becomes more adept at anticipating privacy risks, identifying vulnerabilities, and recommending effective controls. Continuous improvement helps organizations stay ahead of evolving threats and rapidly changing regulations, ensuring a more resilient and compliant data ecosystem.