1. Automated Artifact Identification and Classification
AI-driven image recognition tools can identify, label, and categorize vast collections of cultural items, making it easier to organize and present them within virtual museum spaces.
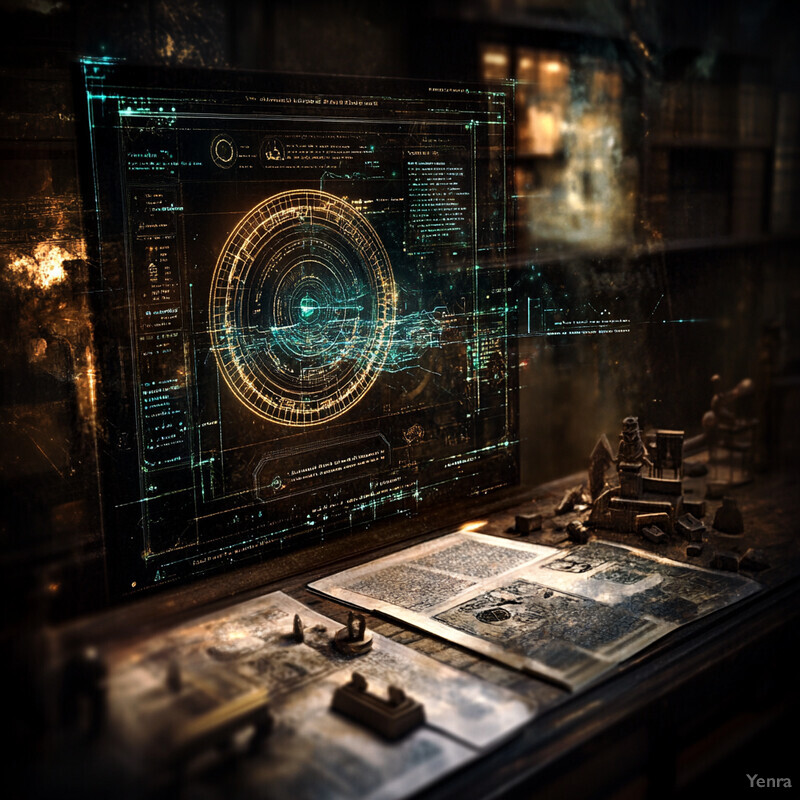
AI-driven image recognition technologies can rapidly scan through massive image databases to identify cultural artifacts and classify them based on style, period, region, or material. By analyzing intricate patterns such as brushstrokes in paintings, decorative motifs in pottery, or inscriptions on sculptures, these systems can assign accurate metadata that often surpasses what manual cataloging can achieve in a given timeframe. This automated classification not only streamlines the curation process, freeing curators and researchers to focus on higher-level interpretive work, but also ensures visitors have quick and reliable access to well-organized collections within virtual museum environments.
2. High-Resolution 3D Reconstructions
Through AI-enabled photogrammetry and 3D modeling, damaged or incomplete cultural artifacts can be virtually reconstructed with greater accuracy, preserving their form for future generations.
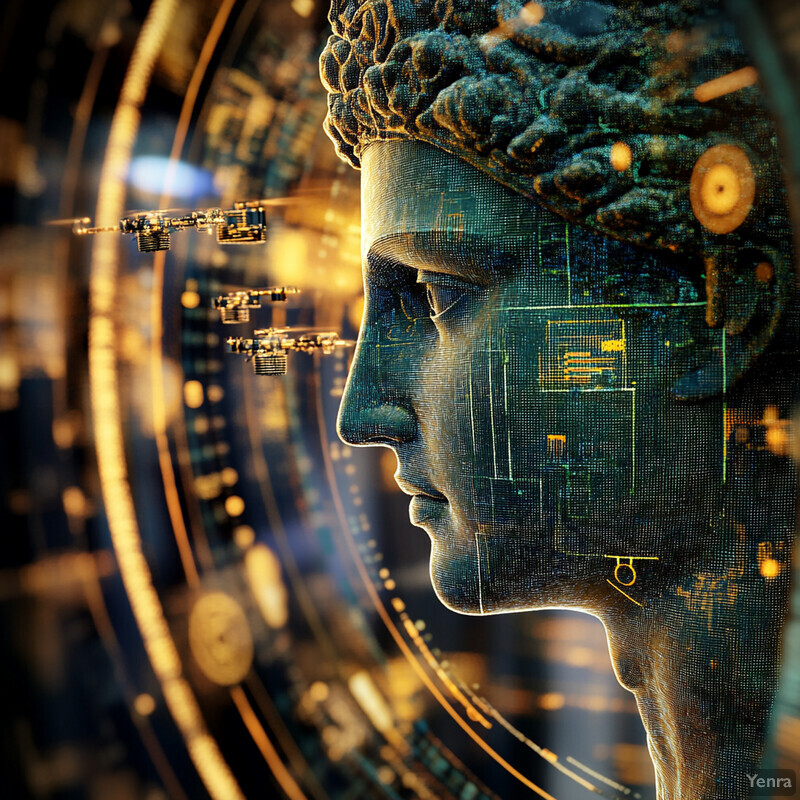
Modern AI algorithms utilize photogrammetry and deep learning techniques to produce high-fidelity 3D models of artifacts, monuments, and entire cultural sites. By stitching together thousands of 2D images and combining them with historical records, these reconstructions can restore details that may have faded from the physical originals. This technology allows visitors to virtually “walk around” an artifact or immerse themselves in a historical environment, experiencing cultural heritage from angles and perspectives not possible in traditional museum settings. These dynamic representations are invaluable for research, preservation planning, and public engagement, as they ensure a permanent, digital record resilient to time and environmental factors.
3. Virtual Restoration of Art and Textiles
Machine learning algorithms can analyze historical records and existing fragments to suggest how faded paintings, murals, or tapestries may have originally appeared, offering digitally restored versions in a virtual museum setting.
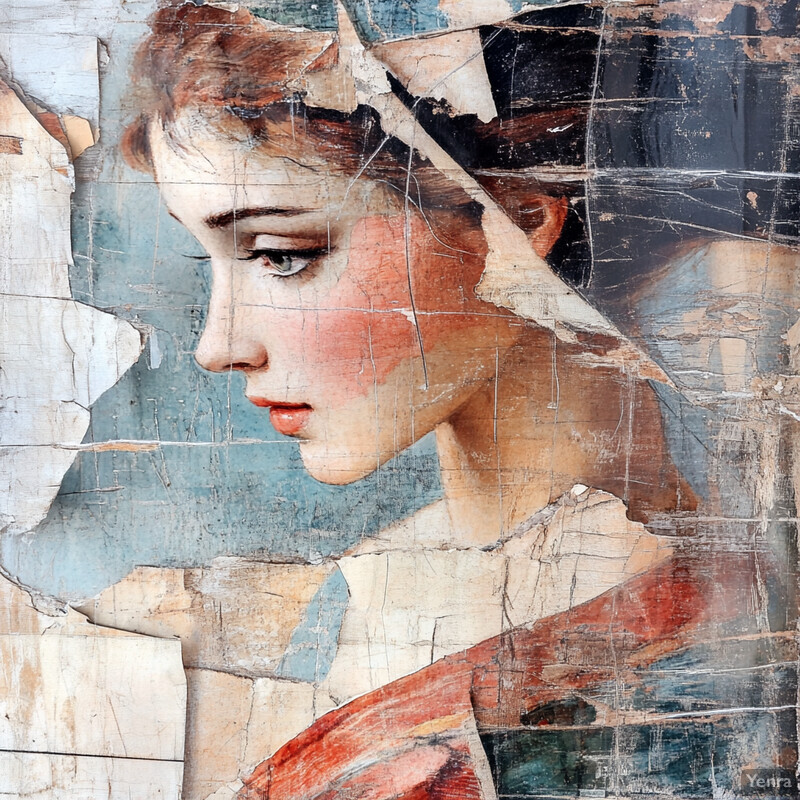
Artificial intelligence can analyze patterns, pigments, and textures in aging paintings, manuscripts, or textiles and then simulate how these objects might have appeared when new. By comparing present-day conditions to reference images, chemical composition data, and stylistic norms of the period, AI-driven restoration tools fill in cracks, reconstruct lost fragments, and revive faded colors. Visitors to virtual museums can thus see culturally significant objects in their original splendor without compromising the integrity of the real artifact. This approach helps historians, conservators, and art lovers better understand the aesthetics and craftsmanship that defined earlier eras, ultimately deepening the appreciation of cultural legacies.
4. Historical Contextualization and Storytelling
Natural language processing (NLP) models can generate rich narrative contexts and interpretations, allowing virtual museum exhibits to tell stories that deepen user understanding of cultural artifacts and traditions.
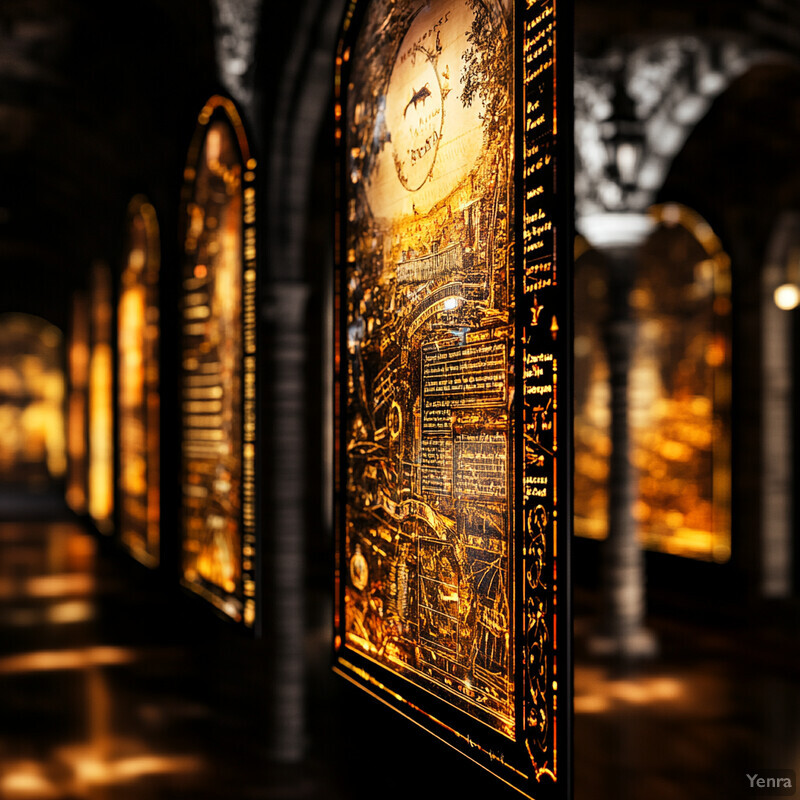
AI-enabled natural language processing (NLP) and knowledge graphs can piece together vast amounts of historical data—from texts, archives, and scholarly articles—to create immersive storytelling experiences around artifacts and cultural sites. These systems interpret complex narratives and condense them into clear, engaging explanations that highlight the cultural significance of each object. In virtual museums, users can access rich context on demand, learning about the people who created the artifact, the cultural influences that shaped it, and its journey through time. This deeper level of storytelling transforms artifacts from static objects into active participants in the narrative of human civilization.
5. Personalized Curatorial Experiences
Recommendation algorithms can tailor virtual museum tours to each visitor’s interests, guiding them through relevant collections and cultural themes, thus enhancing engagement and learning.
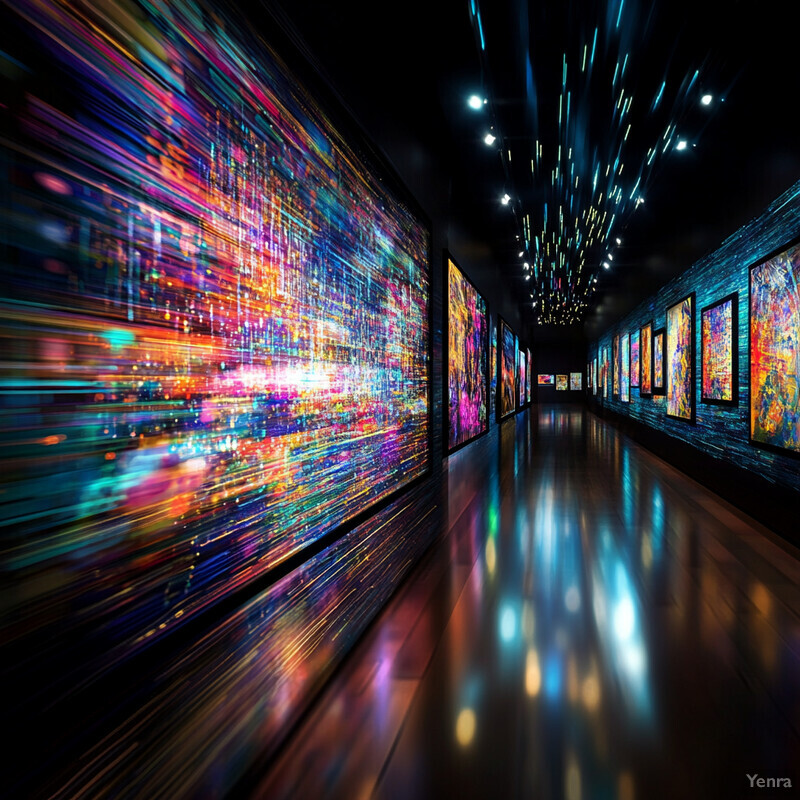
By analyzing user preferences, browsing patterns, and interests, AI recommendation engines can dynamically tailor virtual museum tours to each visitor. Such systems track user engagement—what artworks they linger on, what keywords they search for—and then suggest related artifacts, themes, or historical periods. Personalized recommendations create more meaningful and memorable interactions by focusing on what resonates with the user. This shifts the traditional model of a one-size-fits-all museum layout to a visitor-centric approach, ensuring audiences remain engaged, curious, and motivated to explore cultural heritage at their own pace and according to their unique tastes.
6. Dynamic Linguistic Translations
AI-powered real-time translation provides multilingual access to exhibit information, making cultural heritage more inclusive and accessible to a global audience.
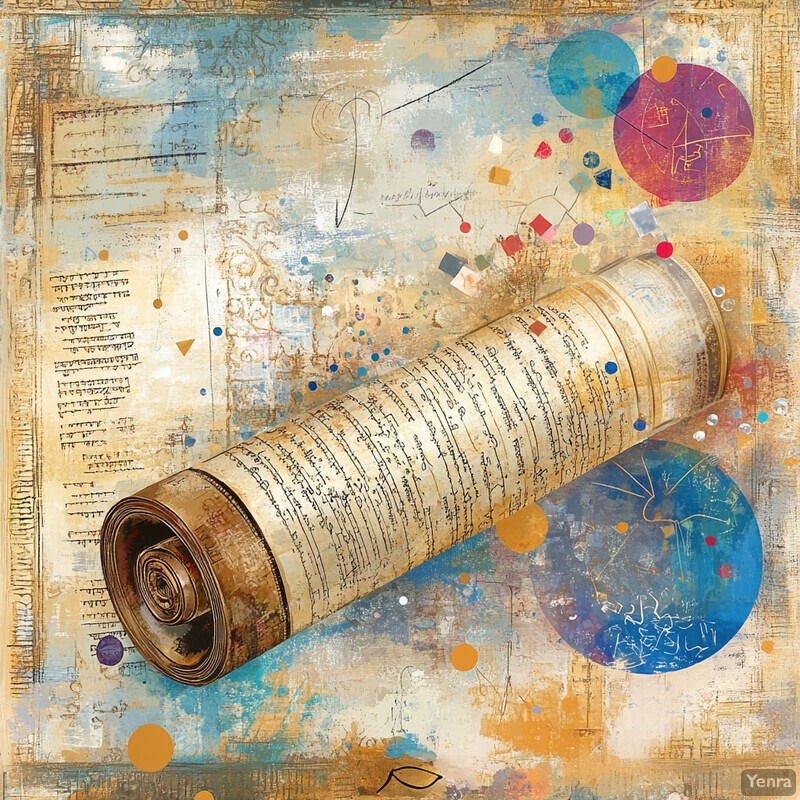
In a globally connected world, ensuring cultural heritage is accessible across language barriers is crucial. AI-driven translation tools, powered by advanced NLP models, can instantly convert exhibit descriptions, audio tours, and educational materials into numerous languages. As visitors navigate a virtual museum, they can seamlessly switch between language options, ensuring that the richness of cultural narratives is not lost in translation. This technology enables people from different linguistic backgrounds to appreciate the same exhibit on equal footing, fostering global understanding and cultural exchange in the digital realm.
7. Intangible Cultural Heritage Preservation
Speech recognition and NLP tools can capture and preserve oral traditions, folklore, music, and dance interpretations, enabling users to experience intangible heritage in virtual spaces.
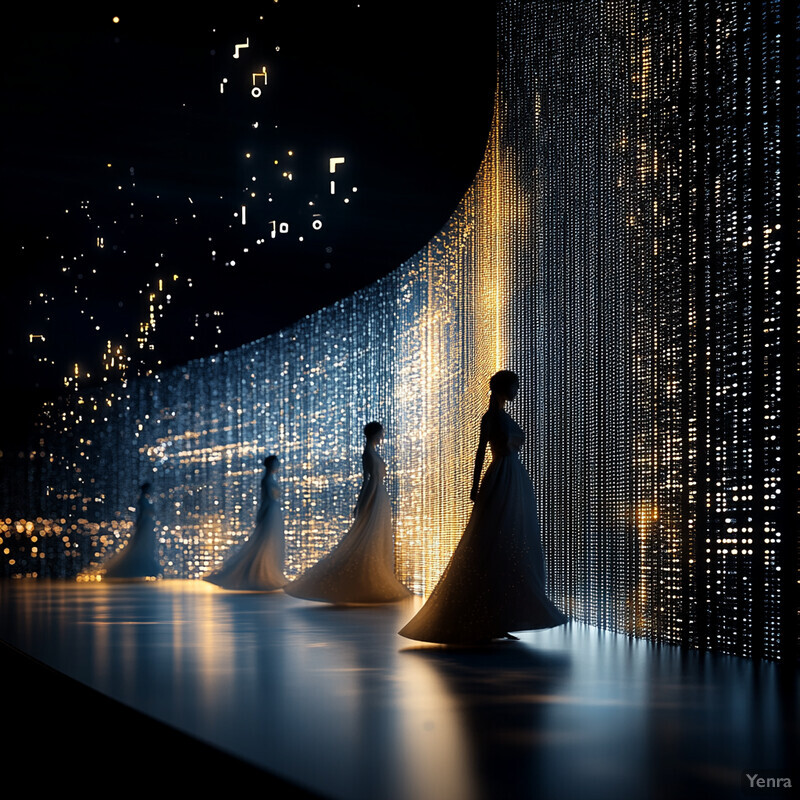
Traditional songs, dances, rituals, and oral histories are forms of cultural heritage that are notoriously difficult to preserve in conventional museums. AI systems equipped with speech recognition, gesture detection, and pattern analysis can capture and digitize these ephemeral practices. In virtual museum platforms, visitors can witness digital recreations or listen to recordings of storytellers, observe choreographies of ancestral dances, or immerse themselves in traditional ceremonies. By preserving intangible cultural heritage in digital form, AI ensures that future generations can continue to learn from and be inspired by cultural expressions that would otherwise fade away over time.
8. Semantic Search and Discovery
Advanced AI-driven search tools allow users to find artifacts not just by keywords but by concepts, enabling more meaningful exploration of cultural collections.
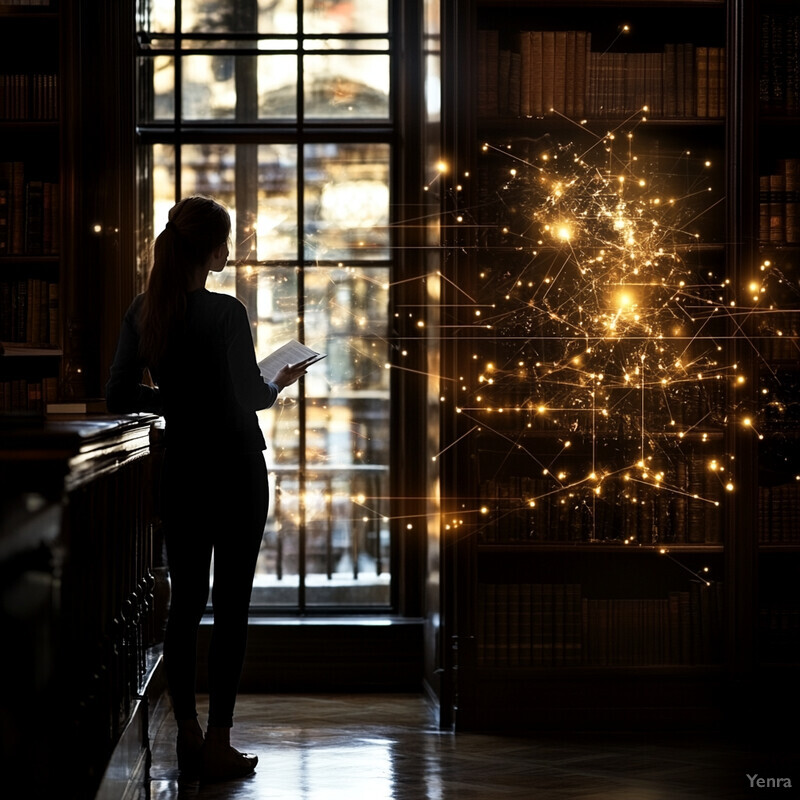
Simple keyword searches often fail to uncover the depth and breadth of cultural artifacts housed in digital archives. AI-based semantic search tools go beyond literal keyword matching to understand the meaning and context behind user queries. By leveraging knowledge graphs and sophisticated language models, these systems help users discover unexpected connections among objects, themes, and historical periods. As a result, visitors can easily find relevant artifacts, gain new insights, and navigate cultural collections more intuitively, enhancing the overall educational and exploratory experience within virtual museum environments.
9. Authenticity Verification with AI Forensics
Machine learning can help verify the authenticity of digitized cultural artifacts by analyzing patterns, materials, and historical documents, ensuring that virtual displays accurately represent heritage objects.
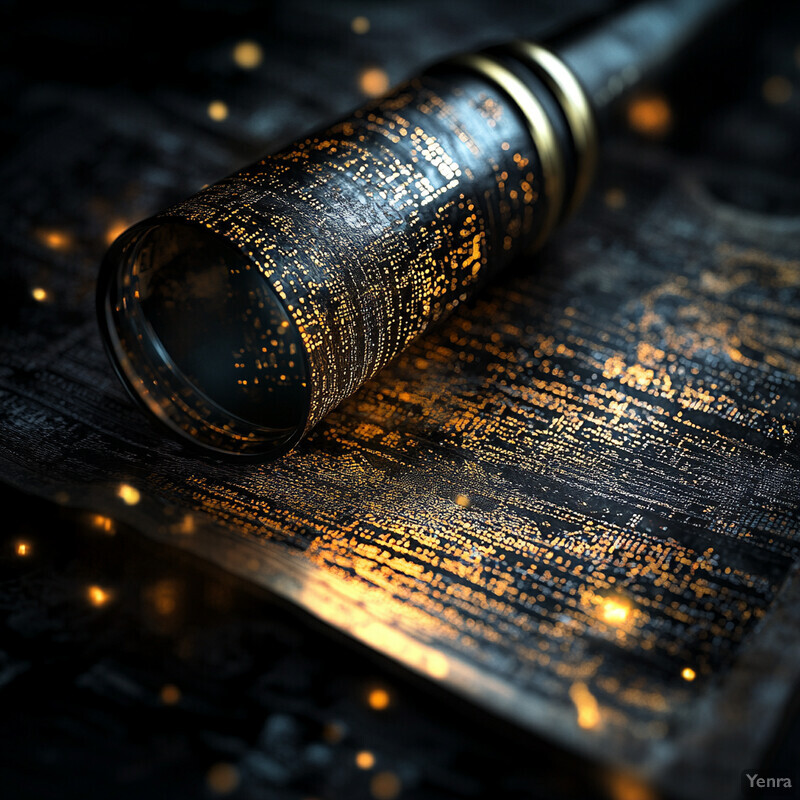
Determining the authenticity of cultural artifacts is crucial for building trust in virtual exhibitions. AI-driven forensic analysis tools can scrutinize digital images for inconsistencies in style, material composition, or historical context. By comparing features against known genuine pieces and existing scholarly data, these tools help curators and researchers authenticate or debunk questionable claims about an artifact’s origin. Ensuring the integrity of displayed objects in virtual museums maintains scholarly credibility and reassures visitors that what they see has been rigorously vetted, bolstering public confidence and academic rigor in digital cultural platforms.
10. Interactive Educational Modules
AI can power adaptive learning modules that quiz visitors, offer tailored explanations, or highlight related artifacts, enhancing both learning outcomes and user engagement.
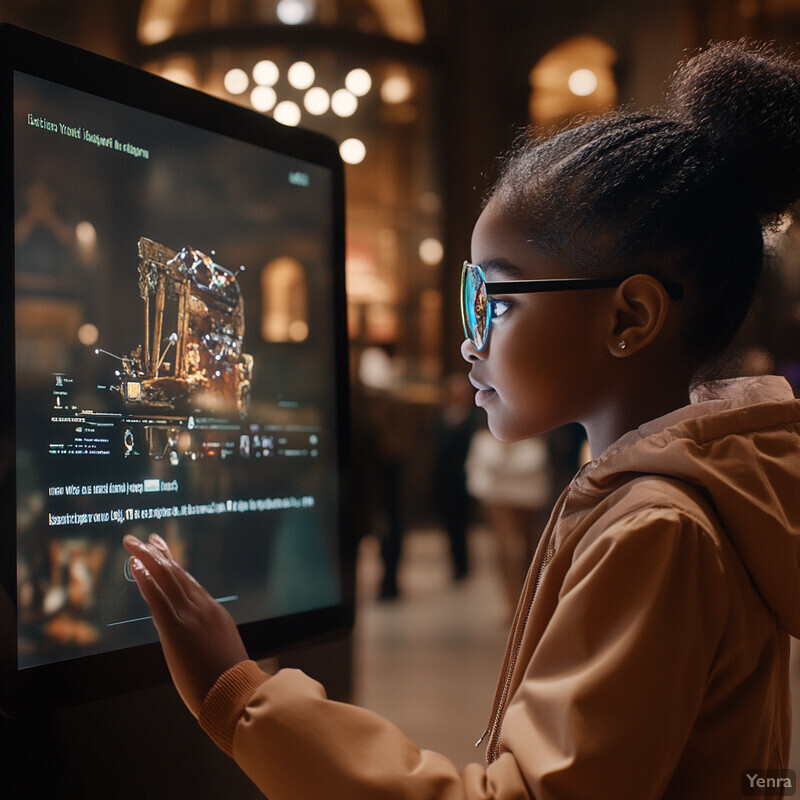
AI can transform passive viewing experiences into active learning opportunities. Through adaptive tutoring systems and quiz-based interactions, virtual museum platforms can offer personalized learning paths to visitors. By assessing the user’s prior knowledge, learning speed, and engagement patterns, AI modules adjust the difficulty and content of lessons. Users might receive interactive prompts, visual comparisons, or critical thinking exercises related to displayed artifacts. This approach not only makes cultural learning more enjoyable and accessible but also improves retention and understanding, turning museums into dynamic educational ecosystems.
11. Predictive Preservation Modeling
By analyzing patterns of deterioration in similar artifacts, AI models can suggest digital ‘restoration’ processes or highlight urgent preservation needs for physical objects, informing physical and virtual museum strategies.
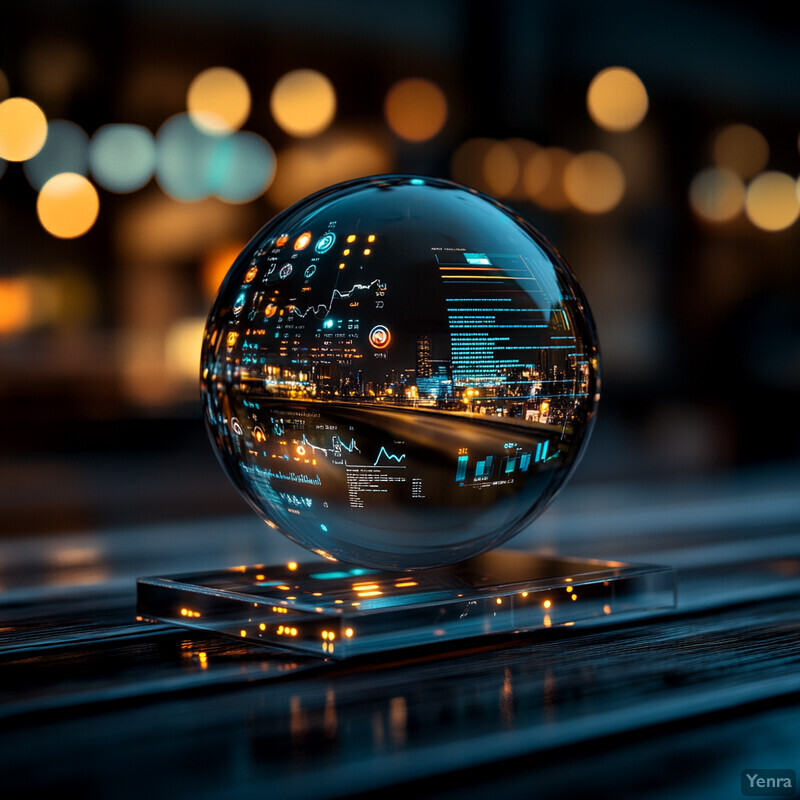
Conservators and curators often face the challenge of predicting the future conditions of artifacts to manage conservation efforts effectively. AI-powered predictive models analyze patterns of deterioration, environmental data, and usage metrics to forecast how certain objects might age or degrade over time. Virtual museums can integrate these predictions to highlight objects needing urgent digital preservation or restoration efforts. Such foresight helps cultural institutions allocate resources efficiently, ensuring that both physical and digital representations of cultural heritage remain accessible for future generations.
12. Robust Metadata Generation
Computer vision and NLP can auto-generate detailed metadata, descriptions, and thematic connections between artifacts, speeding up curation processes and improving virtual exhibit organization.
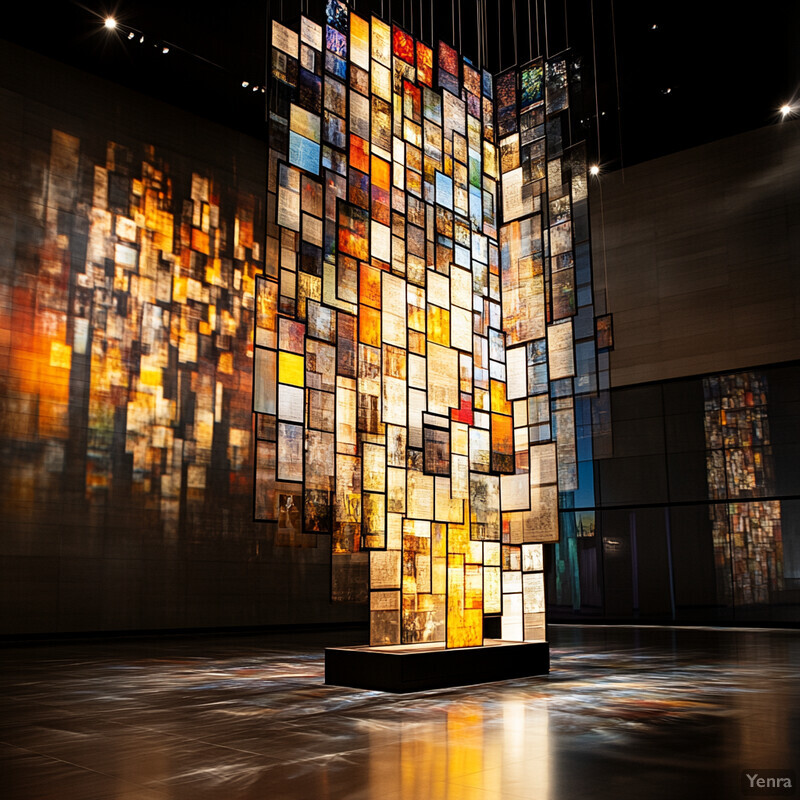
A critical aspect of any well-organized archive or exhibition is detailed, accurate metadata. AI can automatically generate rich metadata by identifying symbols, materials, subjects, and historical references within an artifact’s imagery or documentation. These extensive descriptions facilitate better search, curation, and cross-referencing within virtual museum databases. With reliable and structured metadata, users can move effortlessly from one artifact to another, uncover thematic links, and engage deeply with the cultural narrative presented across collections.
13. Enhanced Accessibility through Assistive AI
Beyond translations, AI can provide captioning, audio guides, and even sign language avatars, ensuring that virtual museums are accessible to individuals with varying abilities.
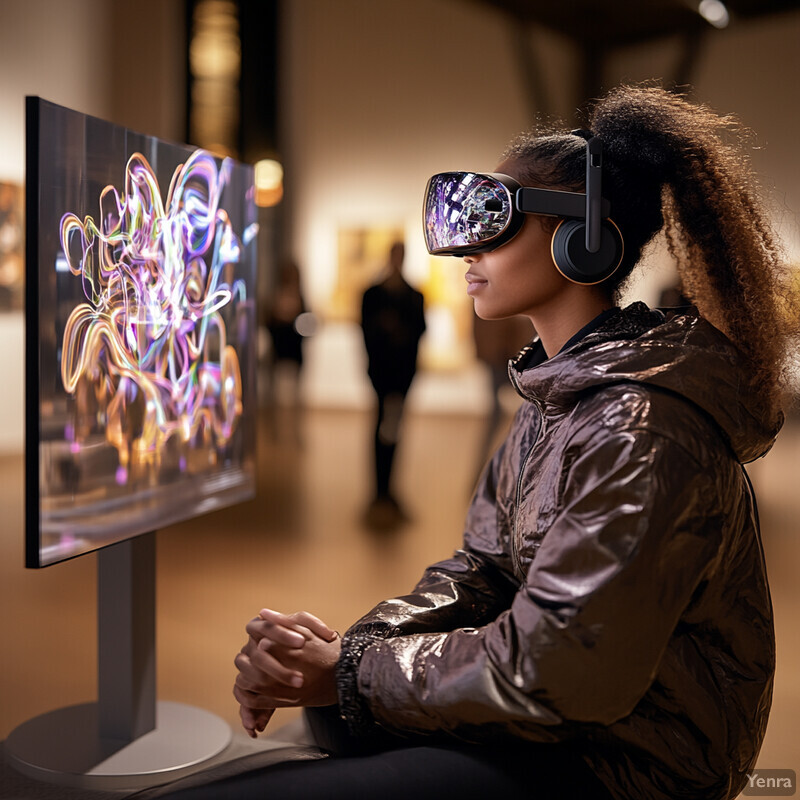
Ensuring that virtual museums cater to visitors of all abilities is essential for inclusivity. AI can provide assistive features such as automated captions for videos, audio descriptions for images, and even sign language interpretation through avatar-based systems. These tools help people with visual, auditory, or other disabilities experience cultural heritage on more equal terms. By leveraging machine learning’s ability to adapt and improve, virtual museums can continuously refine these assistive technologies, ultimately making digital cultural experiences universally accessible.
14. Recreating Historical Environments
AI can use historical data to reconstruct entire cultural settings—ancient cities, lost landscapes, or traditional homes—allowing users to experience cultural heritage in situ within virtual reality.
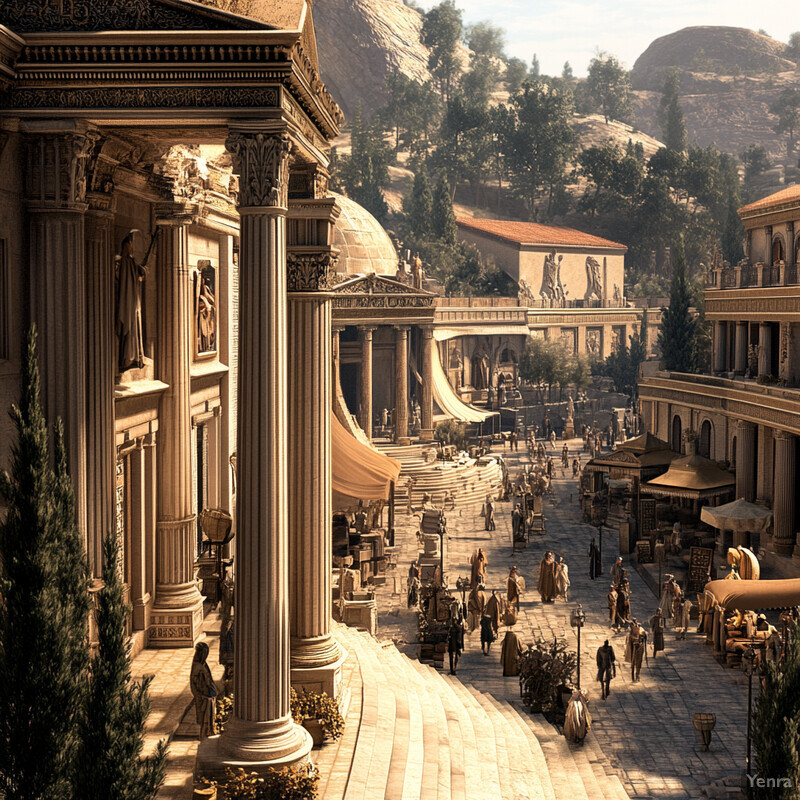
Beyond displaying individual objects, virtual museums can harness AI to reconstruct entire historical or cultural landscapes. Machine learning models incorporate archaeological findings, historical texts, and environmental data to rebuild lost cities, sacred spaces, or cultural hubs in fully immersive 3D environments. Visitors can virtually “step into” ancient marketplaces, navigate through traditional village layouts, or observe cultural exchanges that took place centuries ago. These reconstructions not only provide a more holistic understanding of cultural contexts but also offer educators and researchers a dynamic platform for analyzing and interpreting historical ecosystems.
15. Cultural Network Analyses
Graph-based AI tools can link people, places, events, and artifacts across time, helping virtual museums present interconnected cultural narratives rather than isolated objects.
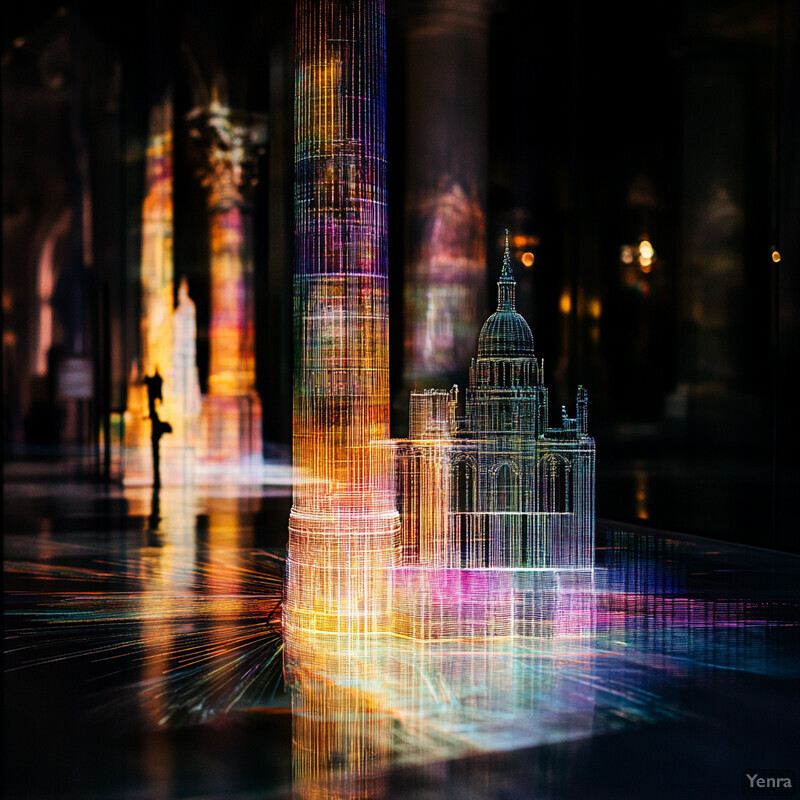
Artifacts do not exist in isolation; they are part of a vast network of cultural relationships. AI-based network analysis tools can connect objects, people, places, events, and ideas across different time periods and geographies. By visualizing these complex webs, virtual museums offer visitors the chance to see how cultural influences spread, how technologies evolved, or how artistic styles intermingled. This networked perspective transforms collections into interconnected stories, helping visitors appreciate cultural heritage as a vibrant, dynamic tapestry rather than a static assortment of unrelated pieces.
16. Sentiment and Engagement Analytics
By analyzing user interactions and feedback, AI can gauge emotional responses and interests, helping curators refine virtual exhibits for greater cultural impact and resonance.
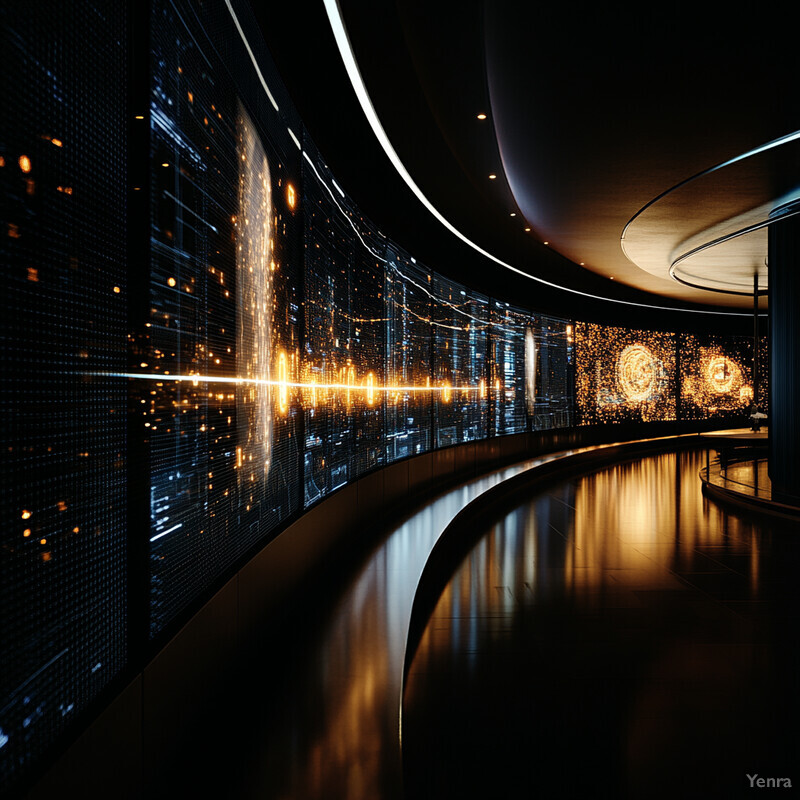
Virtual museum platforms can use AI to monitor user interactions—such as which exhibits attract the most attention, how long visitors spend viewing certain artifacts, or which narratives generate the most positive feedback. These analytics, enhanced by sentiment analysis of user comments and reviews, enable curators to understand visitor preferences and emotional reactions. Using these insights, institutions can refine their exhibitions, highlight resonant themes, and create more compelling storytelling strategies, continually improving the visitor experience.
17. Temporal Progression Simulations
Machine learning can simulate how certain artifacts, architectural sites, or cultural practices evolved over centuries, providing interactive timelines that bring history to life.
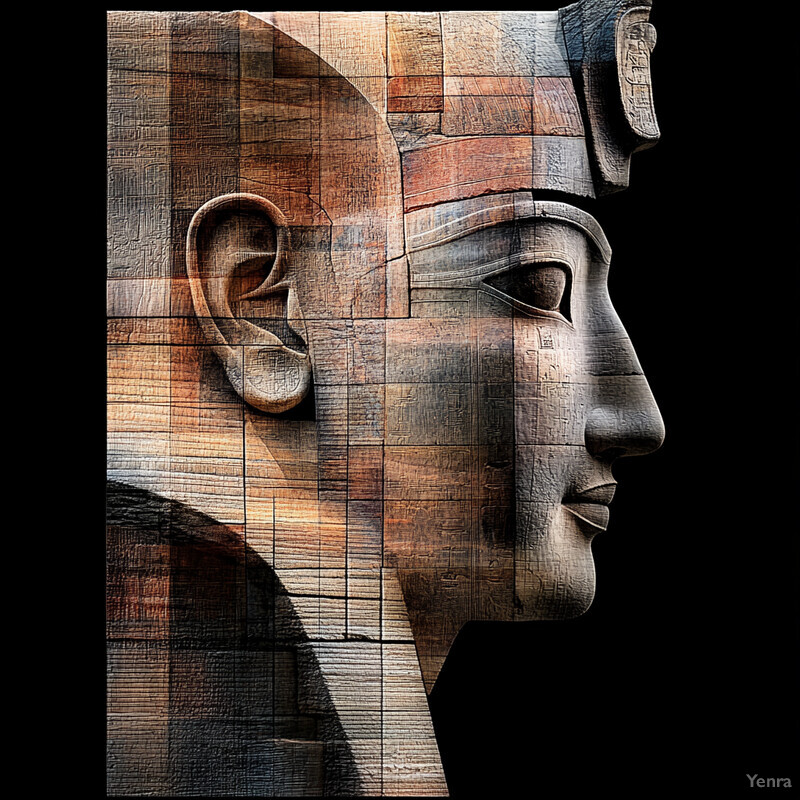
AI-driven models can illustrate how cultural artifacts, architectural styles, or artistic movements developed over long periods. By processing historical data and simulating changes over time, virtual museum visitors can witness the gradual transformation of a cultural practice or the evolution of an art form. These “time-travel” experiences add a dynamic narrative dimension to the museum’s offerings, enabling users to appreciate the processes of cultural adaptation, resilience, and innovation that have shaped our collective heritage.
18. Generative AI for Missing Elements
AI models can ‘fill in the gaps’ by generating probable missing patterns, designs, or textual content for incomplete artifacts, enhancing the user’s ability to visualize lost cultural elements.
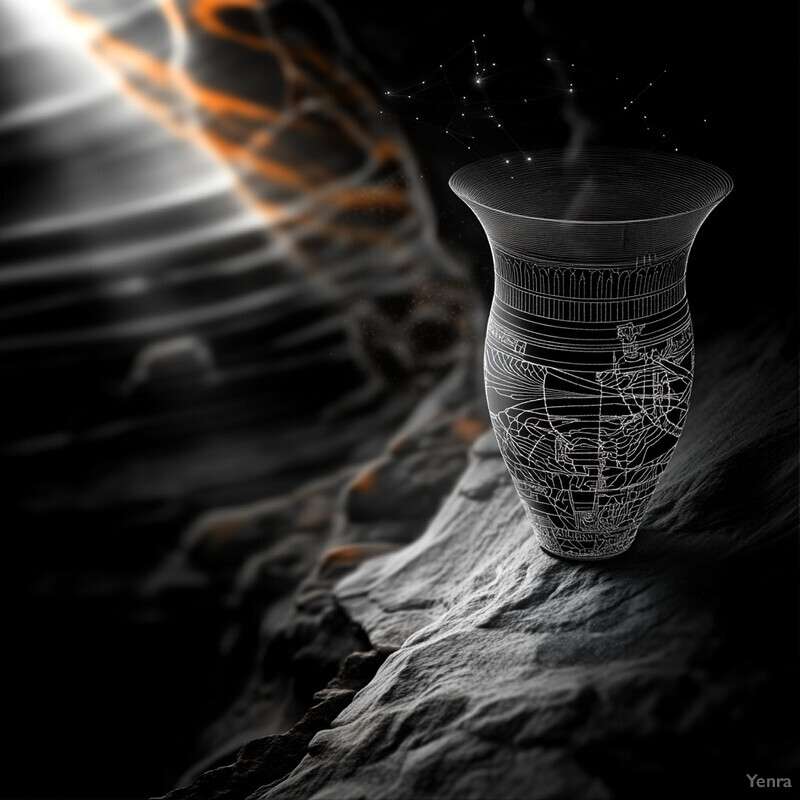
Cultural artifacts are often incomplete due to damage or loss of components. Generative AI models can hypothesize plausible reconstructions of missing parts by analyzing stylistic patterns and historical analogs. For instance, a broken pottery shard might be digitally “completed” with a pattern generated from similar pieces of the same era. While clearly marked as speculative, these digital reconstructions help visitors visualize objects as they might have once existed, enriching their understanding and deepening their connection to the past.
19. Virtual Docent Chatbots
NLP-driven conversational agents can guide visitors through digital exhibits, answering questions in real-time and providing personalized insights and anecdotes about artifacts.
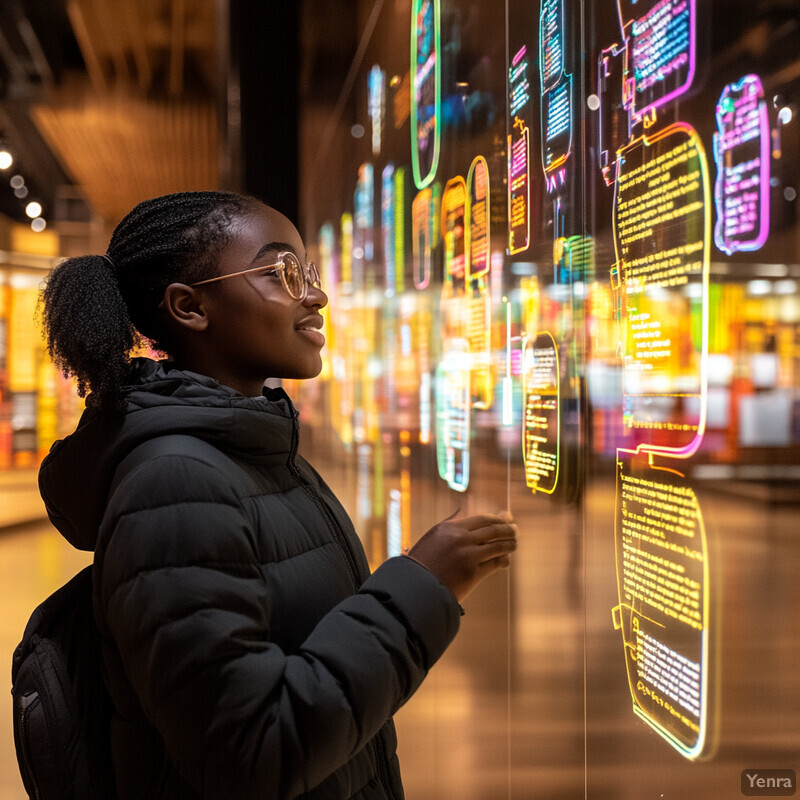
One of the most engaging ways to navigate a museum is through personal interaction with knowledgeable guides. In virtual spaces, AI-powered chatbots serve as digital docents, answering user queries, suggesting related exhibits, and providing in-depth explanations. With NLP and context-aware dialogue systems, these chatbots can handle a wide range of questions, from basic historical facts to more complex cultural interpretations. This personalized, conversational approach helps humanize the virtual museum experience, making it feel more welcoming, interactive, and intellectually stimulating.
20. Data-Driven Curatorial Decision Making
By analyzing user interactions, trending themes, and visitor interests, AI can help curators make informed decisions on what artifacts to highlight next, continuously evolving virtual museum experiences.
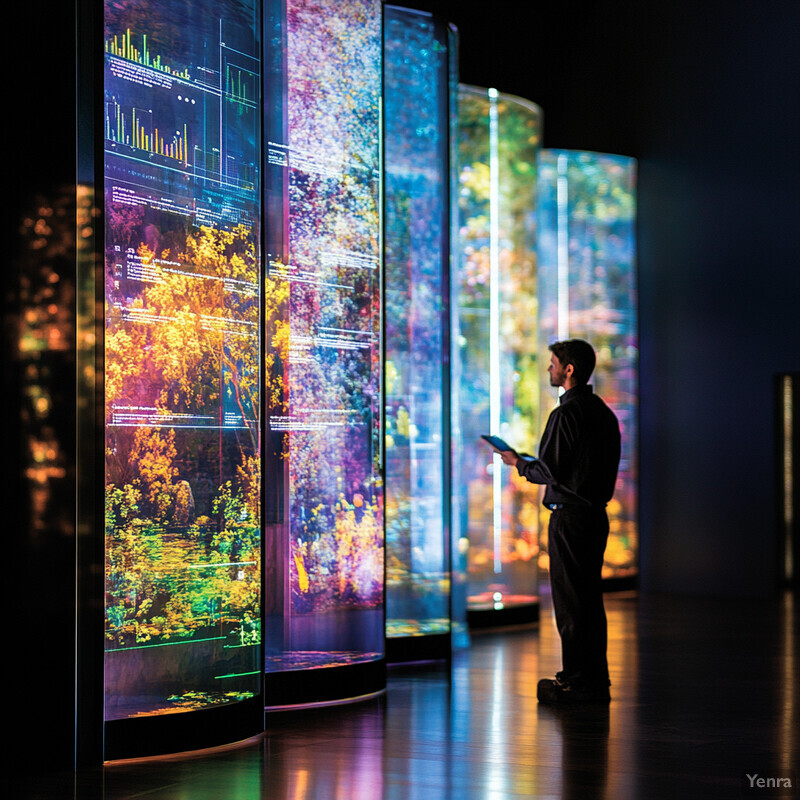
AI analytics can synthesize vast amounts of visitor engagement data, revealing patterns and trends that might otherwise go unnoticed. Armed with these insights, museum curators can identify which themes captivate audiences most, which exhibits prompt meaningful learning, or which narratives spark lively discussion. Over time, curators can use AI-driven recommendations to shape more relevant and inspiring digital exhibitions. By continuously adapting the virtual museum’s offerings based on data-driven feedback, cultural institutions can remain responsive to public interests, ensuring that cultural heritage stays vibrant and resonant in the digital age.