1. Advanced Climate Modeling and Downscaling
AI-powered algorithms can refine coarse global climate model outputs into high-resolution local forecasts.
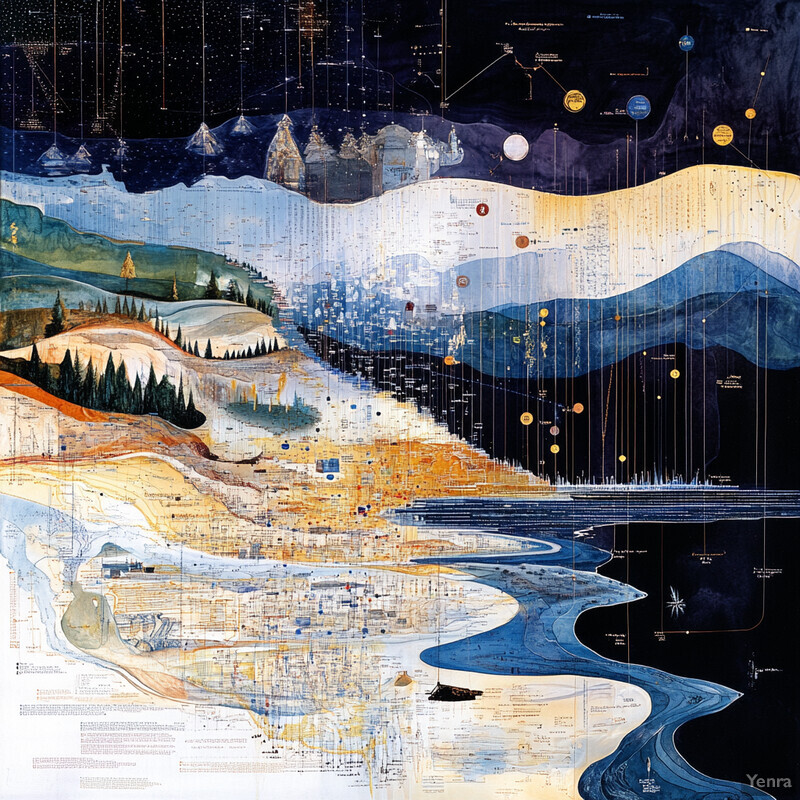
Traditional climate models often operate at coarse spatial and temporal resolutions, which can make it challenging to apply their projections directly to local or regional decision-making. AI-driven techniques such as machine learning–based statistical downscaling bridge this gap. By learning from historical datasets and large-scale climate models, these methods can refine global outputs into highly localized forecasts of temperature, precipitation, and other climate variables. This enables policymakers, city planners, and resource managers to better understand local climate extremes and thresholds, inform urban design, guide agriculture and forestry practices, and ultimately ensure that adaptation measures are appropriately scaled and targeted to the communities most at risk.
2. Early Warning Systems for Extreme Weather
AI-driven analytics can monitor real-time meteorological and oceanographic data to issue rapid, accurate alerts about hurricanes, flash floods, droughts, and heatwaves.
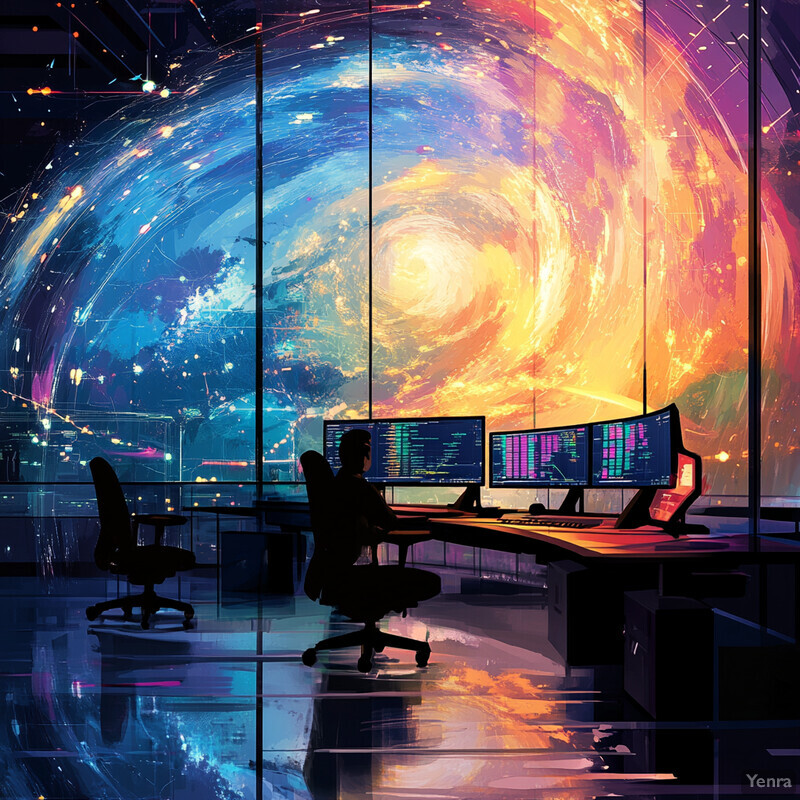
Early warning systems harness AI-driven analytics to detect patterns and anomalies in a vast array of environmental data sources, including satellite imagery, weather station measurements, and oceanographic sensors. These systems rapidly assimilate information and predict the likelihood, intensity, and trajectory of extreme weather events like hurricanes, floods, droughts, or heatwaves. By delivering timely alerts, they empower emergency responders, local governments, and affected populations to take proactive measures. People can evacuate vulnerable areas, reinforce infrastructure, secure essential supplies, or implement water-saving strategies. As climate change intensifies weather extremes, these AI-enhanced warning systems can drastically reduce human and economic tolls while bolstering adaptive resilience.
3. Predictive Analytics for Crop Yields and Agricultural Planning
AI models can integrate weather forecasts, soil conditions, irrigation data, and historical crop performance to predict future yields and inform adaptive planting schedules.
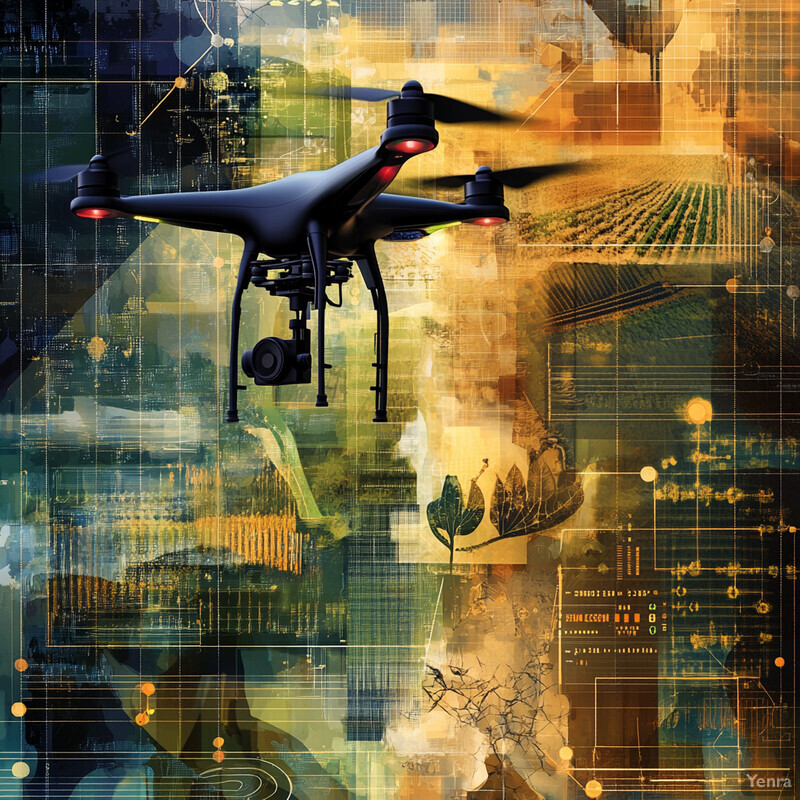
Climate change introduces uncertainty in agricultural production due to shifting rainfall patterns, altered growing seasons, and heightened pest pressures. AI-based predictive analytics synthesize data on local soil quality, historical yields, weather forecasts, and market trends to produce more accurate, actionable insights. Farmers can use these insights to choose more drought-tolerant varieties, adjust planting and harvesting times, or optimize the use of fertilizers and irrigation. By aligning crop selections and resource allocations with predicted conditions, farmers increase their resilience to climate volatility, reduce crop failures, maintain food security, and potentially enhance profitability. This ensures a more adaptive agricultural sector, one better prepared for the challenges ahead.
4. Precision Agriculture and Resource Management
Using AI-assisted remote sensing, drones, and robotic systems, farmers can optimize fertilizer usage, irrigation, and pest control.
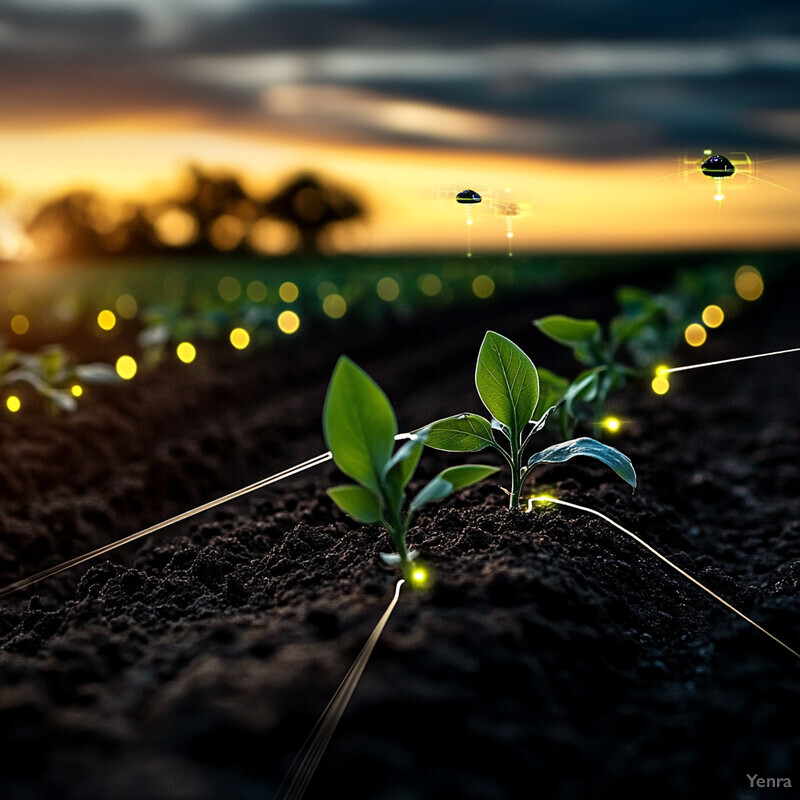
Beyond large-scale forecasts, AI-driven precision agriculture leverages remote sensing, drones, ground sensors, and robotics to make highly localized farm management decisions. Machine learning models can identify nutrient deficiencies, soil moisture levels, and pest infestations at the level of individual fields—even down to specific crop rows. By applying just the right amount of water, fertilizer, or pesticide where and when it’s needed, farmers reduce waste and mitigate environmental damage. Over time, this finely tuned resource management system supports sustained productivity despite shifts in temperature and precipitation. As conditions evolve, precision agriculture practices guided by AI ensure that farms remain productive, efficient, and climate-resilient.
5. Infrastructure Vulnerability Assessment
Machine learning models can analyze engineering data, historical records of failures, and climate projections to identify critical infrastructure that is at high risk.
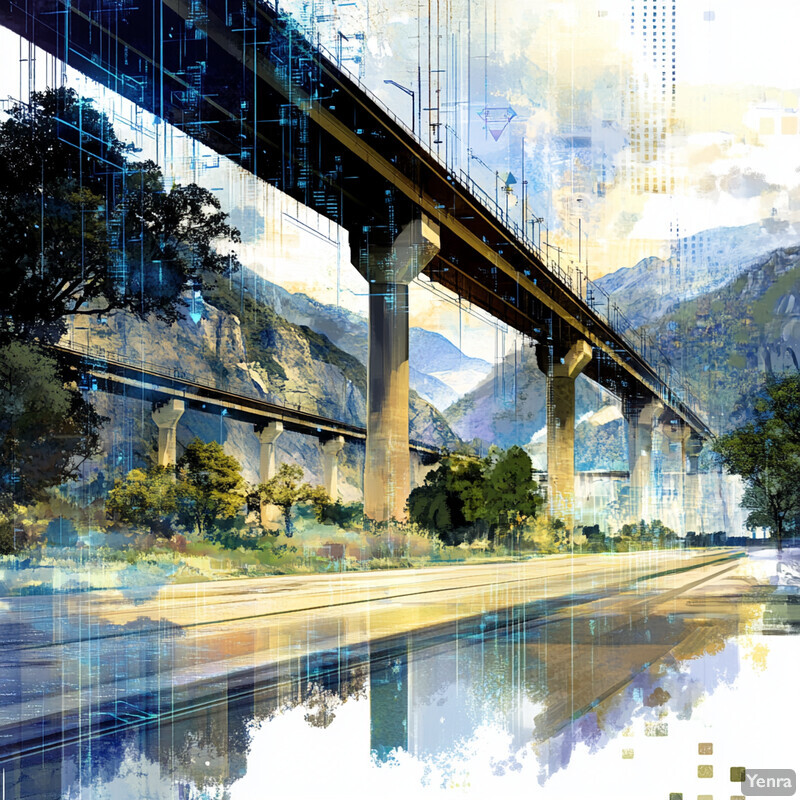
From roads and bridges to levees and dams, critical infrastructure forms the backbone of modern societies. Climate change, however, increases the strain on these structures through more intense storms, flooding, and heat stress. AI models can analyze huge datasets—from engineering blueprints and geotechnical reports to past maintenance records and real-time sensor data—to identify structural weaknesses and forecast future failure points under various climate scenarios. Planners and engineers can then prioritize maintenance, retrofits, or relocations in high-risk areas. By proactively managing infrastructure vulnerabilities, AI helps ensure that essential services remain accessible and functional, enhancing overall community resilience and reducing long-term adaptation costs.
6. Dynamic Flood Mapping and Risk Prediction
AI can integrate satellite imagery, hydrological data, and weather forecasts to continuously update flood risk maps.
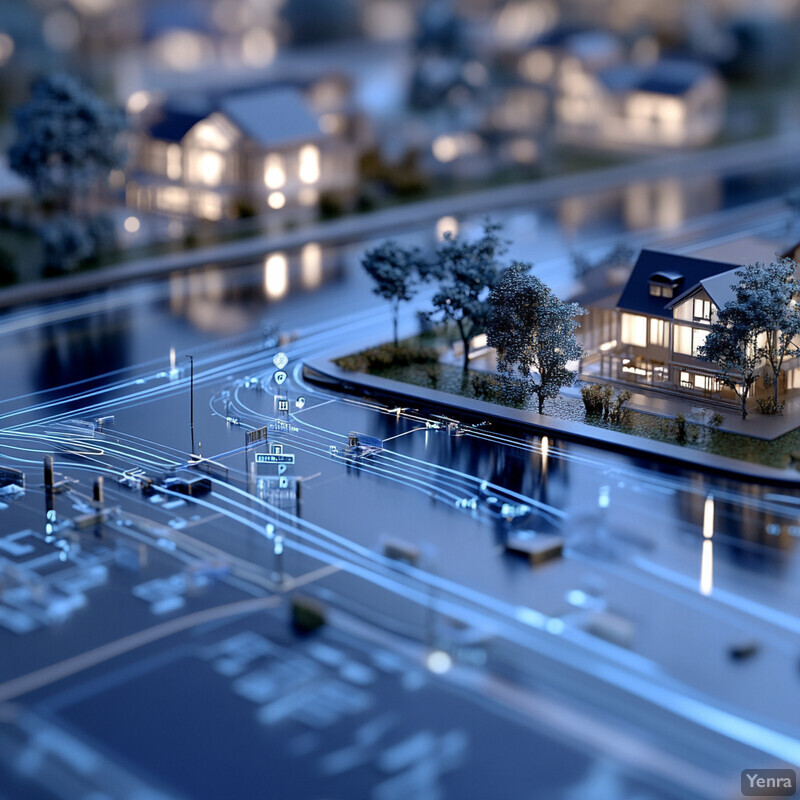
Floods are among the most damaging natural hazards, and their frequency and severity are projected to increase with climate change. AI-enabled flood modeling integrates real-time rainfall data, historical flood records, topographical information, and river flow measurements to continuously update flood-risk maps. These maps enable emergency managers, city planners, and local residents to visualize the changing threats to roads, neighborhoods, or utilities. Armed with this information, municipalities can refine evacuation routes, reinforce flood defenses, steer development away from flood-prone zones, and implement nature-based solutions like wetland restoration. Dynamic, AI-driven flood mapping thus turns complex data streams into actionable plans for safer, more adaptable communities.
7. Coastal Erosion and Sea-Level Rise Modeling
By assimilating coastal topography, sediment transport patterns, storm surge projections, and sea-level rise scenarios, AI helps identify which coastal areas are most vulnerable.
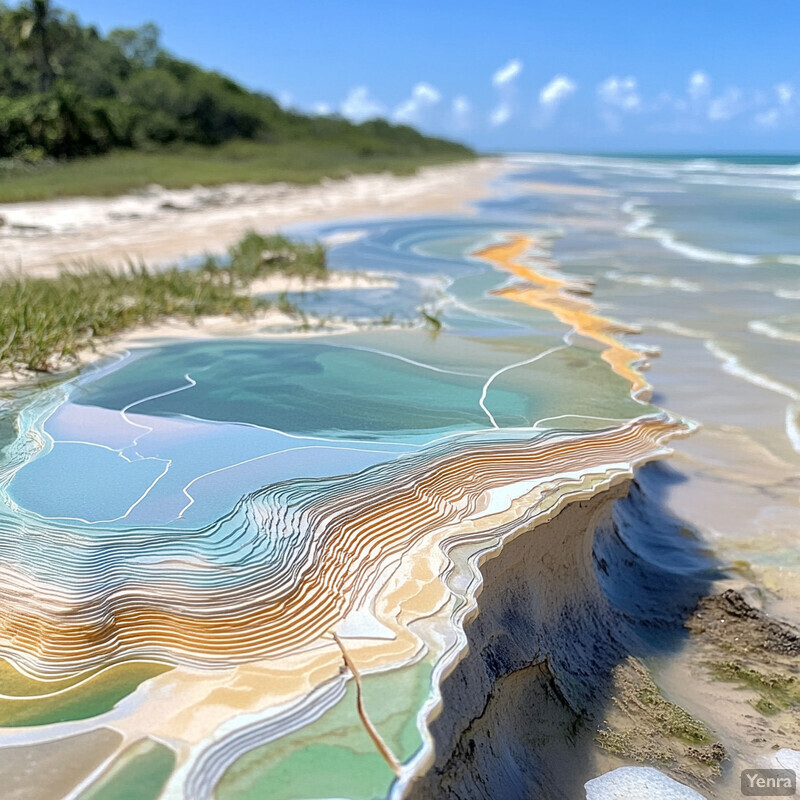
Coastal regions face escalating risks from rising seas, stronger storms, and shifting shorelines. AI-based models can analyze coastal bathymetry, sediment transport patterns, wave dynamics, and historical erosion data to predict how coastlines will evolve under various climate scenarios. Policymakers and stakeholders, from property owners to coastal managers, can use these projections to guide adaptation strategies—such as erecting seawalls, nourishing beaches, installing breakwaters, or restoring mangrove forests. With AI’s ability to incorporate dynamic conditions, forecasts become increasingly accurate over time. This ensures adaptation measures are timely, cost-effective, and aligned with the unique characteristics of each coastal landscape, ultimately preserving valuable ecosystems and human settlements.
8. Disease Vector Tracking Under Climate Shifts
Changes in temperature and rainfall can alter the distribution of vector-borne diseases, and AI-driven models can predict new risk zones.
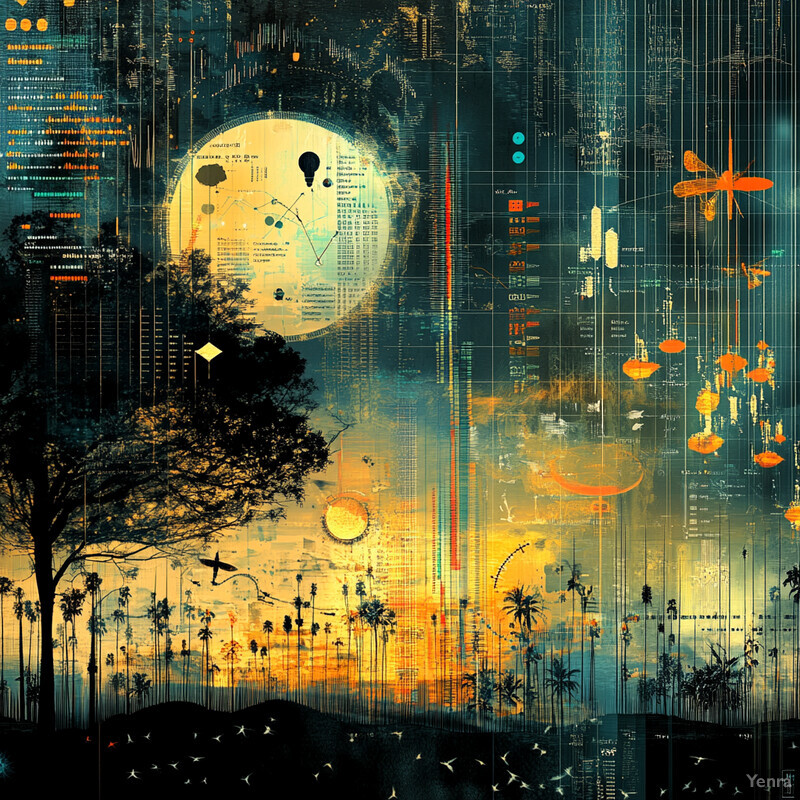
Warmer temperatures, altered precipitation patterns, and changing landscapes can expand the habitats of disease-carrying vectors like mosquitoes or ticks. AI-driven ecological modeling evaluates factors such as climate data, vegetation cover, and human population density to predict where vector-borne diseases might emerge or intensify. Health agencies can then target their interventions—ranging from insecticide spraying and mosquito net distributions to vaccination campaigns—where they are most needed. By staying one step ahead of disease spread, AI-informed public health strategies can reduce the incidence of malaria, dengue, Lyme disease, and other illnesses, safeguarding human well-being in a rapidly changing climate.
9. Real-Time Energy Grid Management
As climate change leads to more unpredictable weather, AI can stabilize the grid by forecasting renewable energy output and electricity demand.
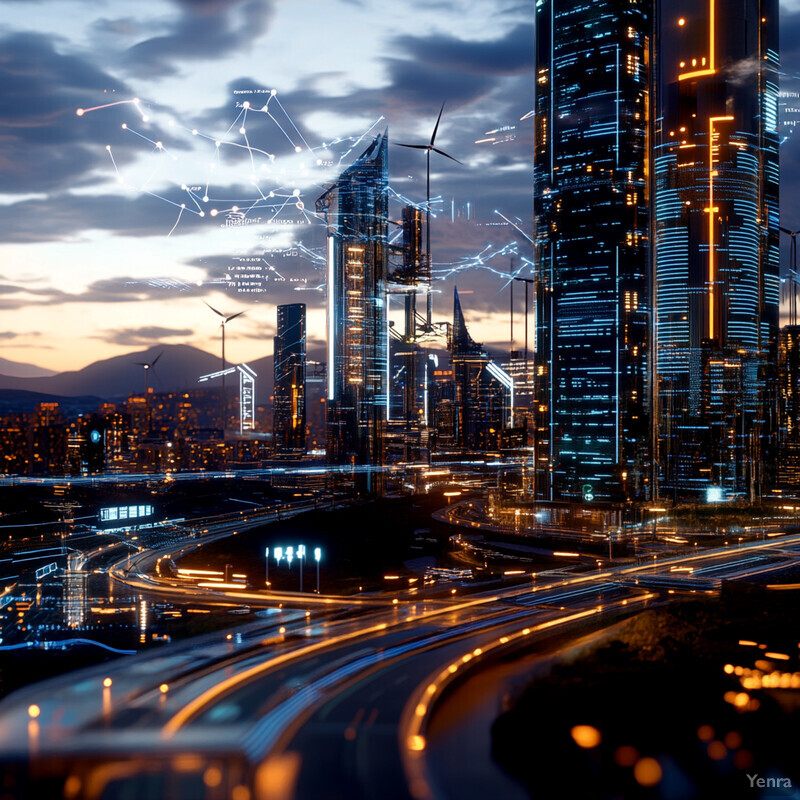
Renewable energy sources like solar and wind are integral to climate adaptation and mitigation, but their intermittent nature challenges traditional grid stability. AI-driven forecasting tools can predict renewable energy generation and electricity demand, balancing supply and load in near real-time. Machine learning optimizes the storage and distribution of electricity, directs energy to where it’s needed most, and rapidly adjusts to changing weather conditions. This ensures more efficient use of renewable sources, reduces reliance on fossil fuels, and enhances grid resilience. By securing a reliable power supply during heatwaves, storms, and other climate extremes, AI helps sustain modern society’s lifeblood: affordable, clean, and stable energy.
10. Water Resource Allocation and Drought Management
Advanced algorithms can track regional water availability, predict future shortages, and optimize water distribution from reservoirs.
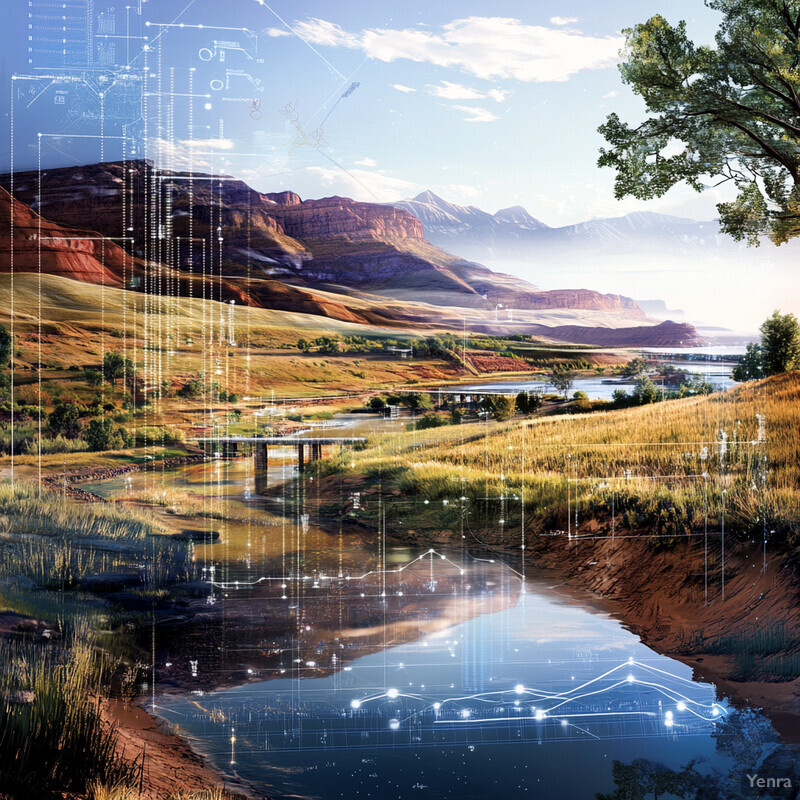
In regions facing water scarcity and drought, efficient allocation of limited water resources is critical. AI systems integrate hydrological models, precipitation forecasts, reservoir levels, and agricultural or industrial consumption patterns to predict water shortages and suggest optimal distribution strategies. Farmers, utilities, and governments can use these data-driven insights to anticipate drought conditions, reduce water wastage, and balance competing demands. Over time, this improves water security, supports stable agricultural output, and ensures that cities remain liveable even as climate patterns shift. By enabling proactive planning and flexible responses, AI contributes to a more sustainable and adaptive water management regime.
11. Soil and Land Degradation Monitoring
AI techniques, including image recognition on satellite data, can identify patterns of soil erosion, land subsidence, or desertification.
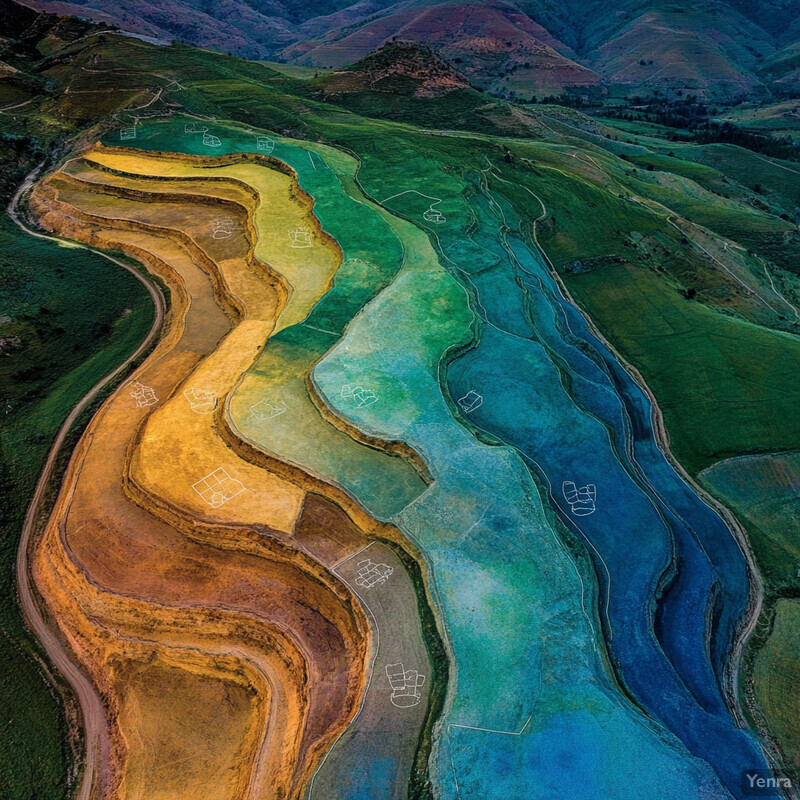
Climate change can intensify soil erosion, desertification, and land subsidence, threatening agriculture, infrastructure, and natural habitats. AI-powered image recognition and remote sensing can detect subtle changes in vegetation, ground movement, or soil moisture that signal land degradation. With early detection, policymakers and land managers can implement rehabilitation measures—such as reforestation, contour farming, terracing, or restoring wetlands—before conditions worsen. This approach not only protects productive lands and ecosystem services but also bolsters resilience by preserving fertile ground for agriculture and maintaining natural buffers against extreme weather. AI thus helps safeguard the fundamental resource—healthy land—that underpins human livelihoods and ecological integrity.
12. Real-Time Urban Climate Resilience Planning
Smart city platforms can integrate local temperature, humidity, and pollution sensors, feeding data into AI models that identify hotspots in need of green infrastructure.
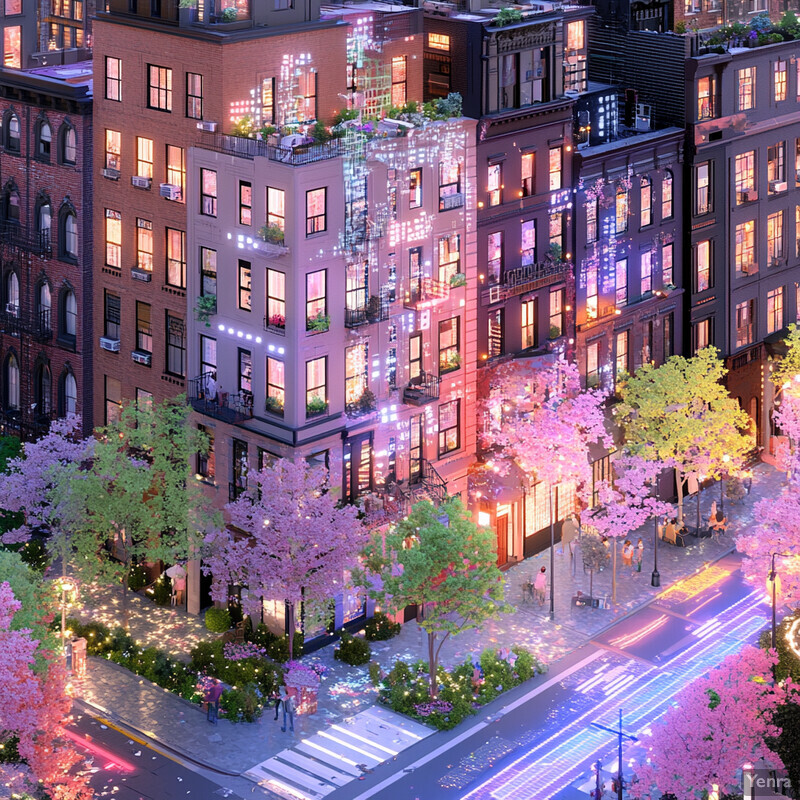
Cities often bear the brunt of climate impacts through heatwaves, urban floods, and pollution. AI-enhanced smart city platforms integrate data from weather stations, air quality sensors, and infrastructure monitors to identify climate stress hotspots. Planners can then deploy targeted interventions, like green roofs, tree planting, reflective pavements, or permeable surfaces, to reduce the urban heat island effect and enhance stormwater management. By coordinating these measures in real-time and adjusting strategies as conditions evolve, city officials foster more comfortable, healthier, and safer urban environments. Ultimately, AI-enabled resilience planning ensures that as our climate changes, our cities remain vibrant and livable.
13. Climate-Informed Supply Chain Optimization
Using forecasting and scenario analysis, AI helps companies reconfigure supply chains to anticipate disruptions from extreme weather.
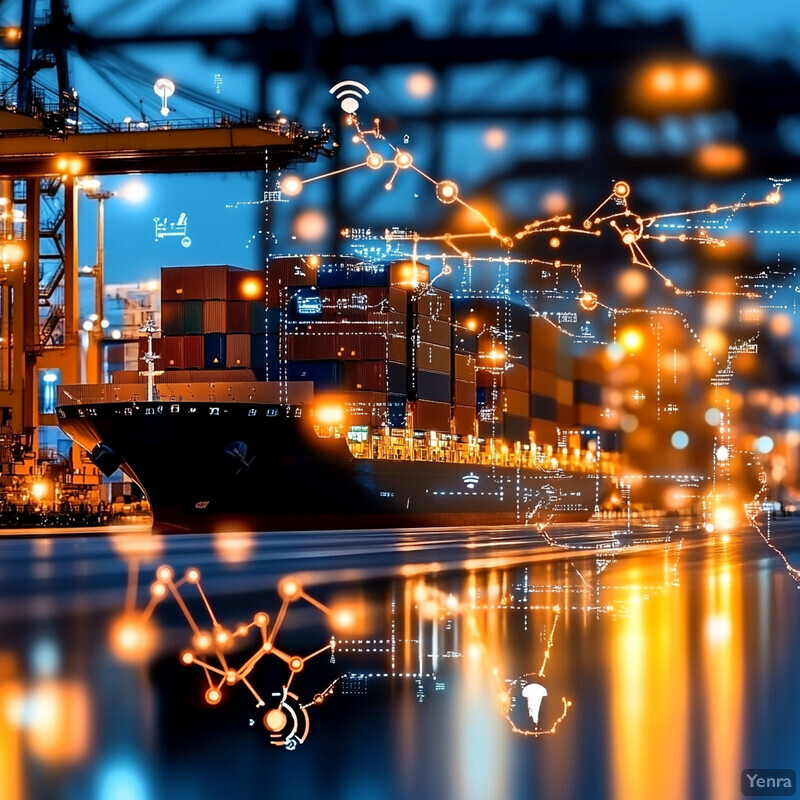
Extreme weather events and shifting climatic patterns can disrupt the flow of goods and services at every stage of a supply chain. AI-driven tools analyze weather forecasts, transportation routes, manufacturing capacities, and inventory levels to predict disruptions and suggest alternative strategies. Businesses can restructure procurement, diversify suppliers, store extra inventory in safer locations, or reroute shipments to avoid flooded roads or closed ports. By fortifying supply chains against climate-related shocks, AI not only prevents financial losses but also stabilizes food, medicine, and resource availability for communities worldwide. This resilience translates into greater economic stability and public well-being.
14. Ecosystem Health and Biodiversity Monitoring
Remote sensing, combined with AI-driven pattern recognition, can track changes in vegetation, wildlife populations, and habitat quality under shifting climate conditions.
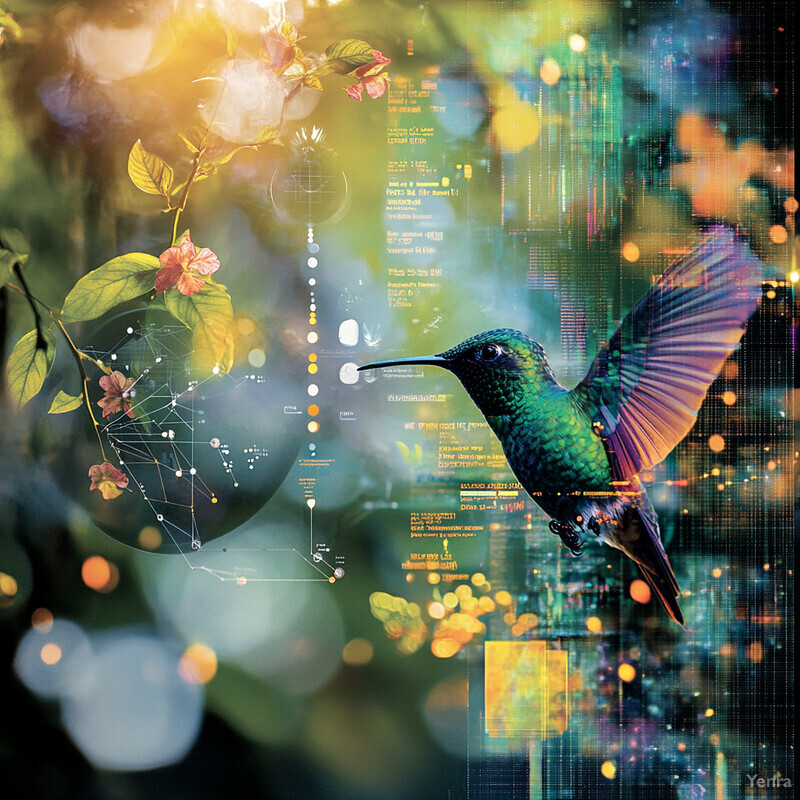
As climate change alters habitats and species distributions, AI-driven pattern recognition from remote sensing and wildlife monitoring data can track shifts in vegetation cover, migration patterns, and population dynamics. Conservationists and land managers can identify which species or ecosystems are most vulnerable and devise adaptive strategies, such as establishing wildlife corridors, reintroducing native species, or protecting key breeding grounds. By guiding targeted interventions, AI helps maintain ecological balance and preserves the ecosystem services—pollination, water purification, carbon storage—that societies depend on. Investing in ecosystem health and biodiversity makes natural systems more resilient, ultimately reinforcing our collective ability to adapt to a changing world.
15. Insurance Risk Analysis and Parametric Coverage
The insurance industry uses AI to model climate-related risks more precisely, enabling the design of parametric insurance policies.
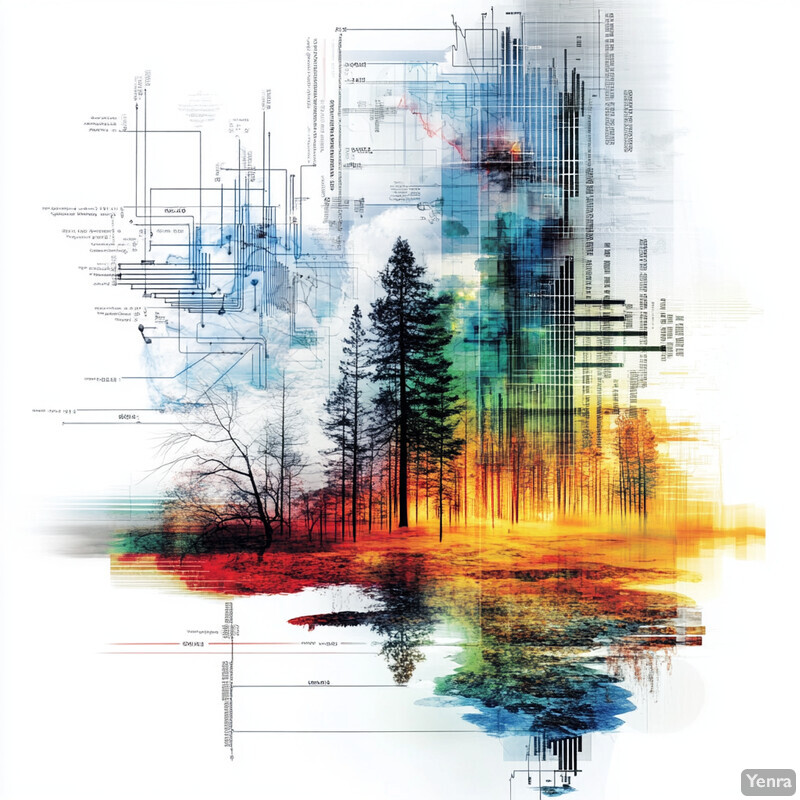
The insurance sector increasingly relies on AI to model climate-related risks more comprehensively. By integrating complex climate projections, historical loss data, and socioeconomic factors, insurers can more accurately price coverage against droughts, floods, or storms. Parametric insurance policies, which trigger payouts when pre-defined environmental conditions are met, speed recovery and reduce bureaucratic hurdles. Communities can rebuild critical infrastructure, restore damaged farmland, or restock emergency supplies more quickly. In this way, AI-enhanced risk analysis not only stabilizes financial markets but also encourages proactive adaptation measures that limit future losses, building a more robust societal response to climate challenges.
16. Forest Fire Detection and Management
Machine learning can analyze satellite imagery and environmental data in near real-time to spot early indications of wildfires.
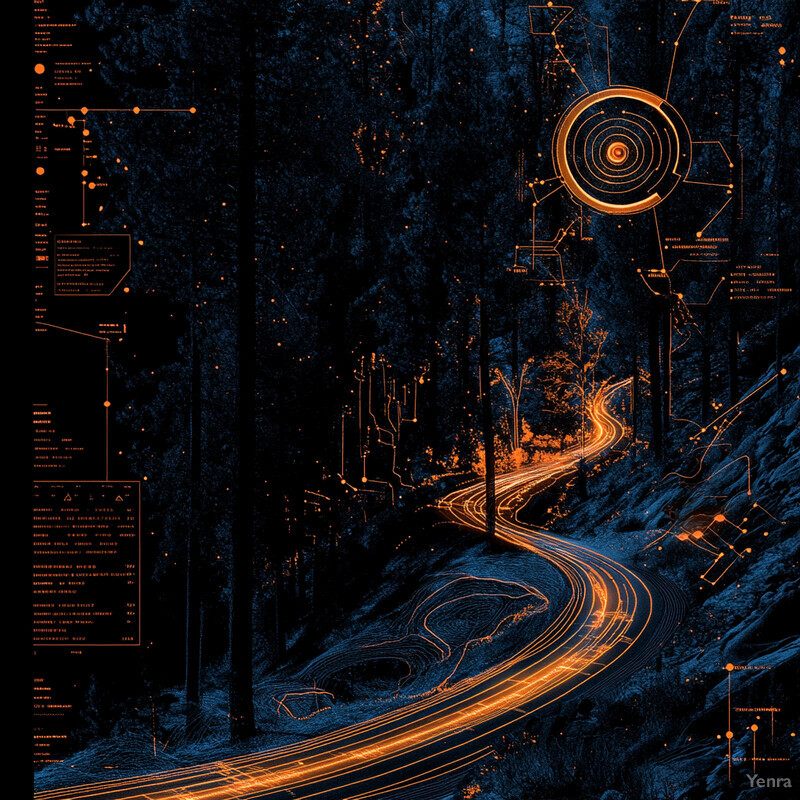
Climate warming and drying conditions have increased the frequency and severity of wildfires. AI systems analyze satellite imagery, weather forecasts, soil moisture data, and vegetation patterns to detect early fire starts and predict fire behavior. This allows firefighting teams to deploy resources more strategically, containing blazes before they become catastrophic. Long-term, AI can suggest controlled burns, strategic thinning, and species selection to enhance forest health and reduce vulnerability. By influencing both immediate response and preventative management, these tools help maintain forests as carbon sinks, habitats, and recreational spaces, ultimately making landscapes more resilient to ongoing climatic shifts.
17. Green Infrastructure Suitability Analysis
AI-based suitability modeling helps planners decide where to place green roofs, permeable pavements, bioswales, and constructed wetlands.
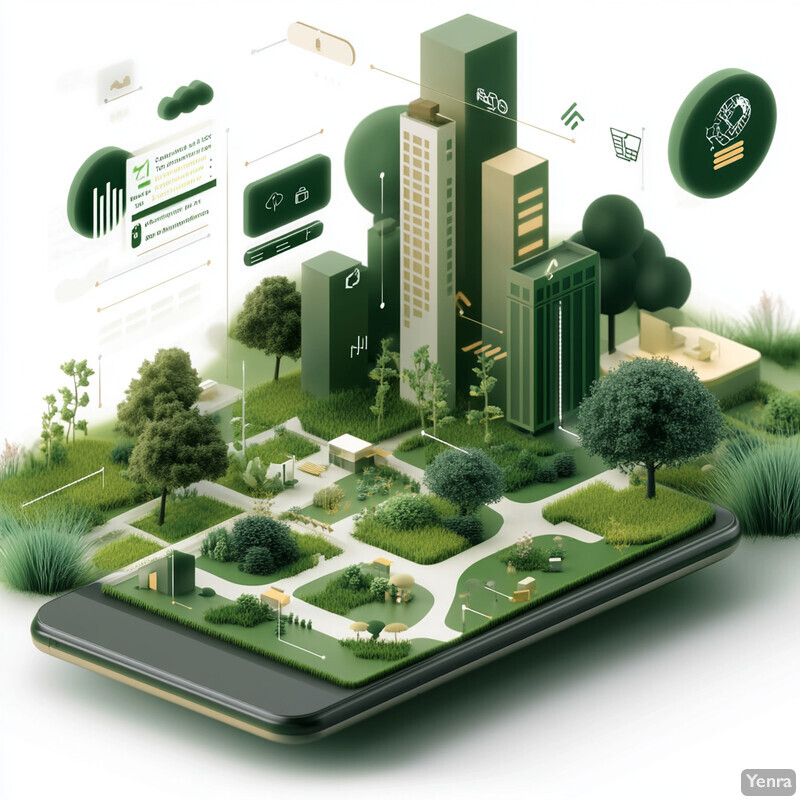
Cities are embracing nature-based solutions—like wetlands, rain gardens, and green roofs—to mitigate flooding, reduce heat, and enhance urban biodiversity. AI-driven modeling can map where these interventions are most likely to yield benefits, factoring in local topography, soil permeability, precipitation patterns, and land-use constraints. By prioritizing the placement and design of green infrastructure, planners maximize ecological functionality and cost-effectiveness. Over time, these urban oases increase public well-being, reduce energy consumption, protect infrastructure, and support wildlife. AI thus acts as a powerful tool for transforming cities into adaptive, climate-resilient landscapes that gracefully blend built and natural environments.
18. Fisheries and Aquaculture Adaptation
Changes in ocean temperature, acidity, and currents affect fish migration and breeding patterns, and AI models can predict these shifts.
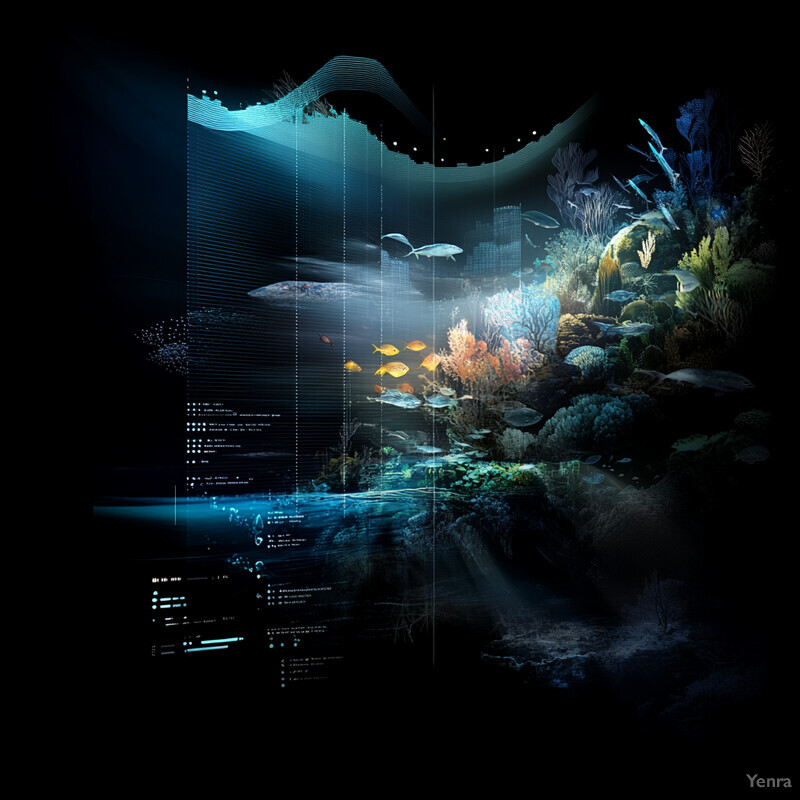
Marine and freshwater ecosystems are highly sensitive to temperature changes, ocean acidification, and shifting currents. AI models combine environmental monitoring with species distribution and lifecycle data to predict changes in fish populations and migration patterns. Fisheries managers can adjust quotas, select more climate-resilient species for aquaculture, or establish marine protected areas to safeguard critical habitats. In turn, these measures ensure stable harvests, protect livelihoods, and maintain a healthy marine food web. By keeping step with the dynamic changes in marine ecosystems, AI empowers more sustainable and flexible fisheries management under escalating climate pressures.
19. Public Health Climate Adaptation Strategies
Integrating climate projections with disease surveillance and demographic data, AI can help public health officials prepare for climate-driven health crises.
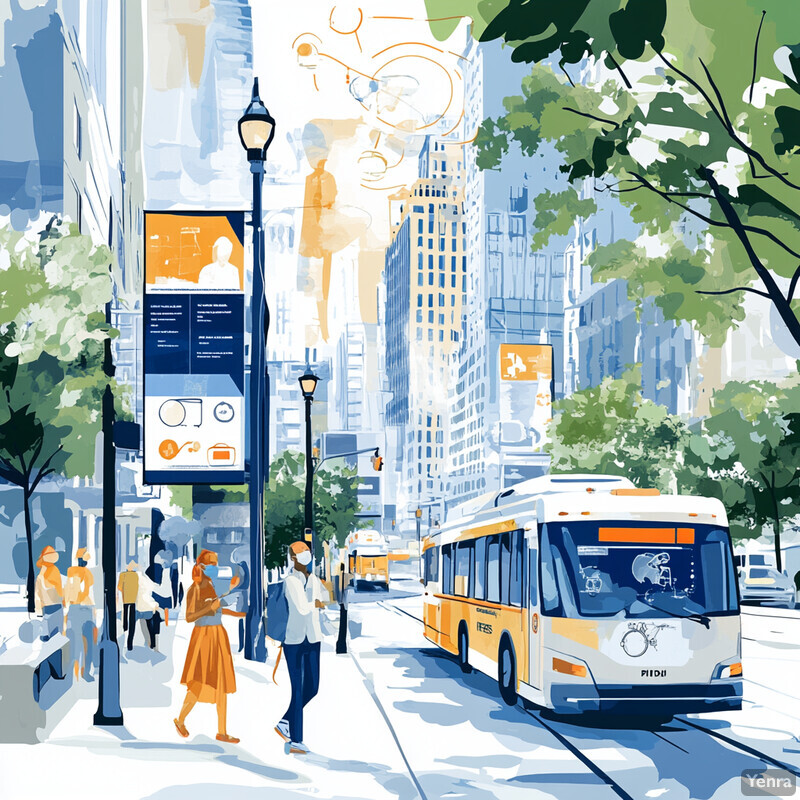
Heatwaves, air quality degradation, and shifting disease patterns affect human health, especially in vulnerable communities. AI-powered platforms integrate climate forecasts, disease surveillance, infrastructure data, and demographic information to anticipate health risks. Officials can bolster healthcare systems, stockpile medicines, or establish cooling centers before extreme events hit. Over the long term, these insights guide urban planning decisions, such as creating green spaces that cool neighborhoods or improving water infrastructure that prevents disease outbreaks. By proactively protecting public health in a changing climate, AI ensures that adaptation strategies focus not only on physical infrastructure but also on safeguarding human lives.
20. Multi-Hazard Resilience Planning
AI tools allow policymakers to evaluate trade-offs among various adaptation measures.
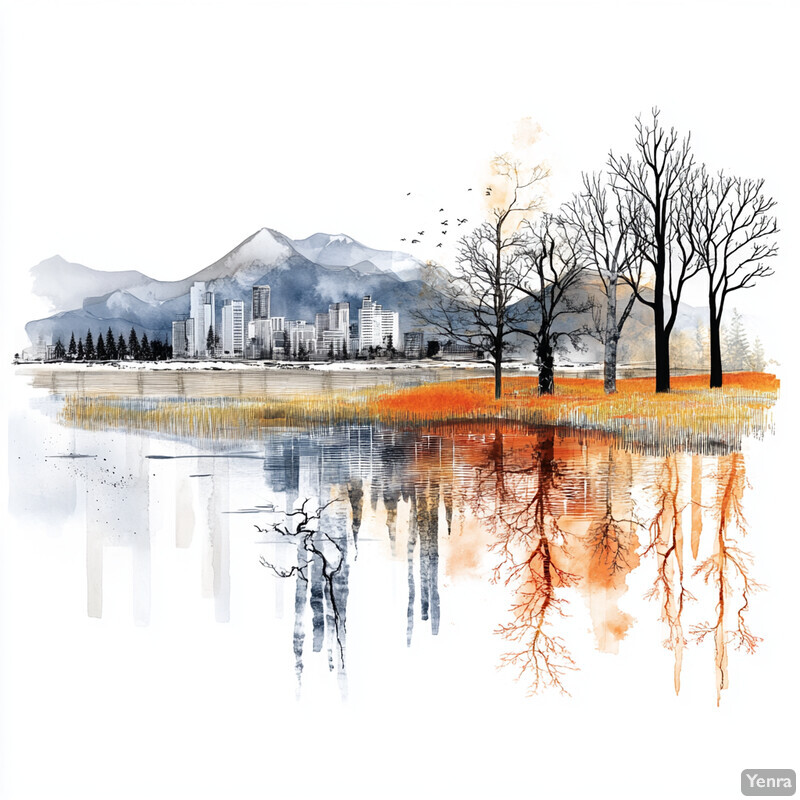
Climate adaptation often involves navigating multiple, interconnected challenges—such as how drought conditions can heighten wildfire risk or how rising seas intensify storm surge impacts. AI-driven decision support tools integrate complex datasets and model compound hazards, helping policymakers understand the interplay between different climate threats. This holistic perspective enables authorities to design adaptation strategies that are robust across multiple scenarios, ensuring that flood defenses, water management systems, agricultural policies, and healthcare provisions work together. By embracing a multi-hazard approach, AI ensures that adaptation is comprehensive, synergistic, and forward-looking, ultimately fostering more sustainable and resilient societies in the face of climate uncertainty.