1. Personalized Prosthetic Design
AI-driven algorithms can analyze patient-specific anatomical, biomechanical, and lifestyle data to create custom prosthetic designs tailored to individual limb geometries and movement patterns.
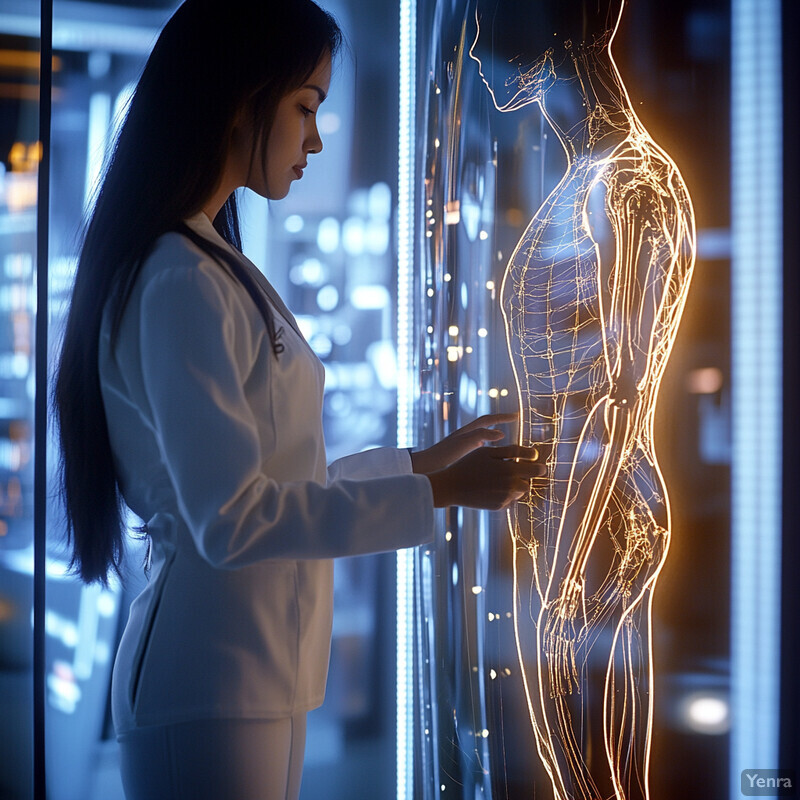
AI techniques, particularly advanced machine learning algorithms, can leverage detailed data about an individual’s anatomy, joint mechanics, residual limb geometry, and mobility habits to craft truly personalized prosthetic solutions. By integrating high-resolution scans, motion capture data, and feedback from wearable sensors, these algorithms can generate 3D models that precisely fit the user’s unique residual limb shape. Instead of relying on standardized templates or expert intuition alone, AI tools can quickly converge on a design that optimizes comfort, load distribution, and alignment. This personalized approach not only reduces pressure points and chafing but also improves the prosthetic’s mechanical efficiency, allowing patients to move more naturally. Over time, the system can iterate and refine these models based on patient feedback, clinical evaluations, and performance analytics, continually improving both comfort and functionality.
2. Optimized Material Selection
Machine learning models can predict how various materials (e.g., carbon fiber composites, titanium alloys) will behave under complex biomechanical loads, aiding in the selection of materials that balance weight, strength, and flexibility.
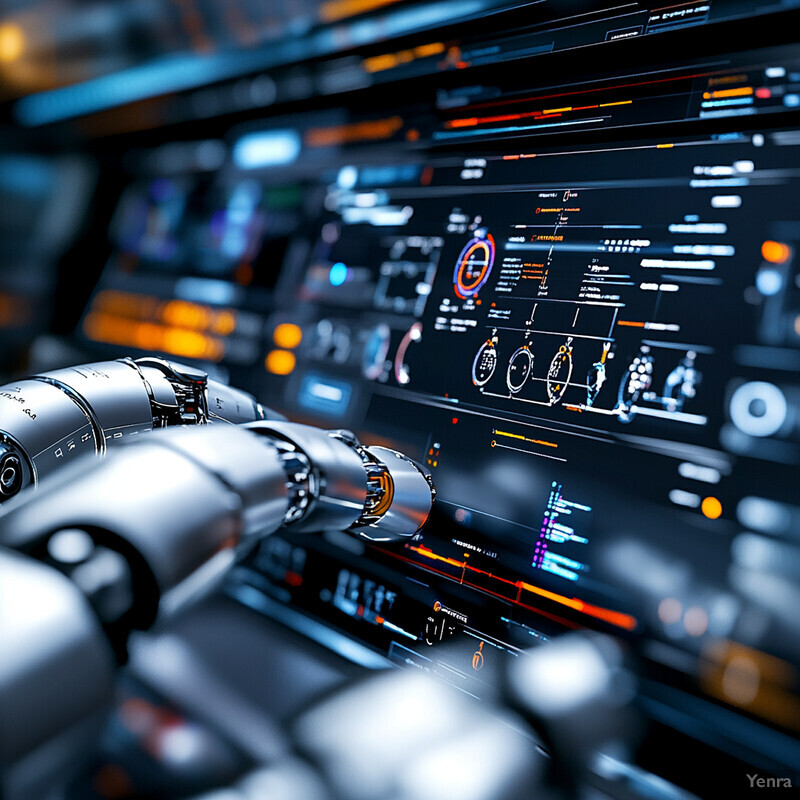
Selecting materials for prosthetics involves balancing factors such as weight, strength, durability, and flexibility—complex considerations that AI can streamline. Machine learning models can be trained on vast datasets of material properties, simulation results, and historical performance metrics to predict how candidate materials will behave under a wide range of biomechanical loads. By doing so, these systems help engineers and clinicians identify the optimal combination of composites, polymers, or metals that meet the patient’s performance needs while also withstanding stresses and reducing fatigue. Such insights can lead to prosthetics that are lighter yet more robust, improving both user comfort and device longevity. Ultimately, AI-driven material selection can expedite the design cycle and enable more cost-effective production while ensuring prosthetics achieve their intended mechanical and ergonomic goals.
3. Data-Driven Shape Modeling
Advanced AI techniques, such as generative adversarial networks (GANs), can generate accurate 3D prosthetic socket models from residual limb scans, ensuring a better fit and reducing patient discomfort.
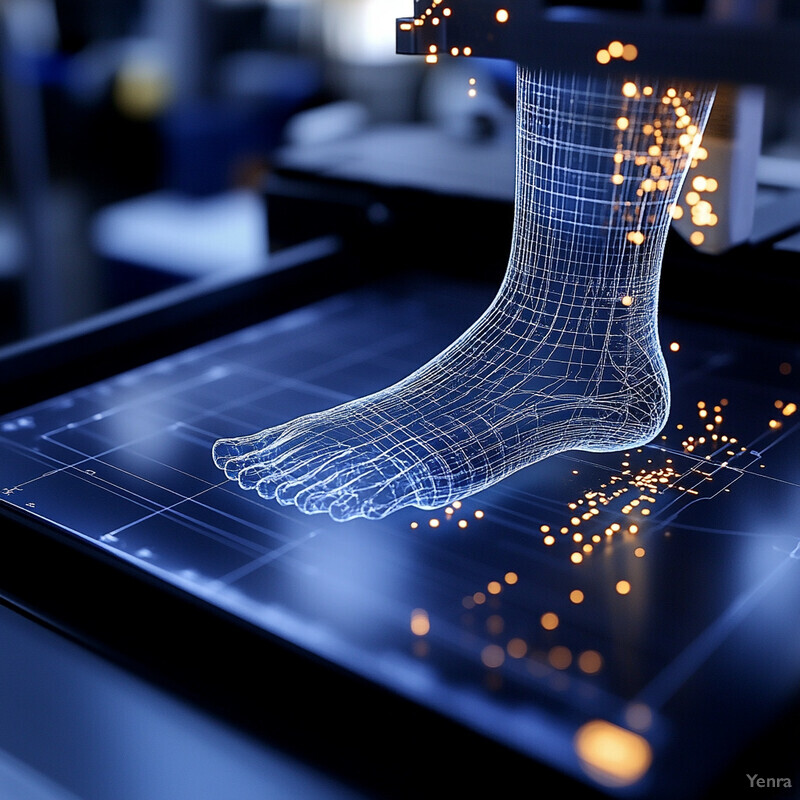
Traditional prosthetic socket fabrication relies heavily on manual casting and trial-and-error adjustments to achieve a secure, comfortable fit. AI-powered generative models, like GANs, can automate much of this process by converting patient-specific residual limb scans into refined 3D shapes. These models learn from large databases of successful prosthetic fits, correlating limb geometry and tissue density with ideal socket contours. As a result, the prosthetist can rapidly produce sockets that minimize pressure hotspots and enhance weight distribution, improving comfort and stability. This data-driven approach reduces the time required for iterative fittings and can lead to more satisfactory user outcomes, enabling patients to adapt more easily to their prosthetic devices.
4. Dynamic Gait Simulation
Deep learning-based biomechanical models can simulate a user’s gait under different conditions, predicting how the prosthetic will perform when walking, running, or climbing stairs. This helps designers refine limb alignment and joint stiffness.
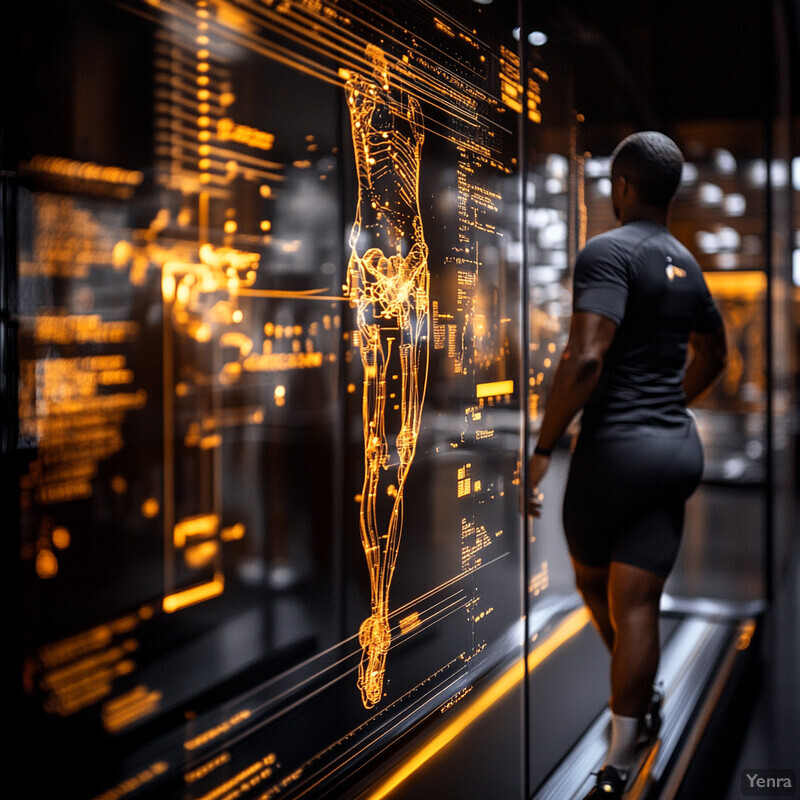
One of the most critical aspects of prosthetic design is how it influences the user’s gait. AI-driven dynamic simulations leverage deep learning and biomechanical modeling to predict how a prosthetic will affect walking, running, or navigating uneven terrain. By analyzing motion capture data, joint kinetics, and muscle activation patterns, these models help engineers visualize and fine-tune joint stiffness, alignment, and energy return before the prosthetic is ever constructed. Such simulations allow for rapid adjustments to the virtual prototype, ensuring the final device promotes a more natural and efficient stride. The result is a prosthetic that feels more like an extension of the user’s own body, reducing energy expenditure, injury risk, and overall discomfort.
5. Real-Time Control Adjustments
Reinforcement learning algorithms can continuously adapt prosthetic joint control parameters, such as damping and stiffness, in response to changing user dynamics and environmental conditions.
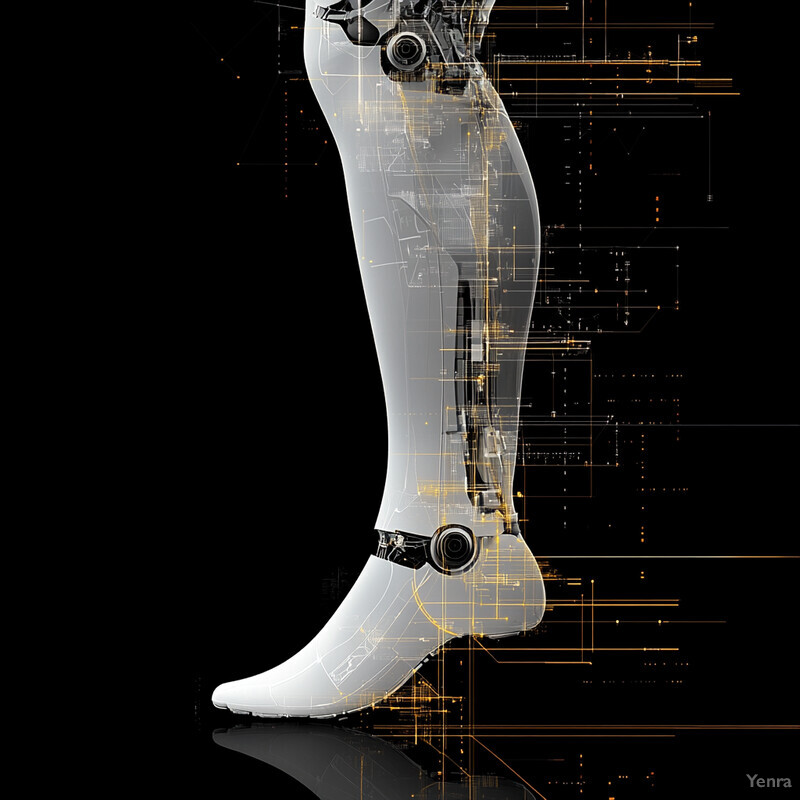
In real-world scenarios, a prosthetic must adapt continuously to changing conditions—altered walking speeds, diverse terrains, and user fatigue. Reinforcement learning algorithms can monitor sensor inputs in real-time and adjust joint control parameters, such as stiffness and damping, on the fly. By continually learning from the user’s movements and feedback, the prosthetic can become more responsive and intuitive over time. For example, when transitioning from a flat surface to stairs, the AI controller could instantly alter the prosthetic’s torque profile for improved stability and safety. This capability ensures that users enjoy a fluid, personalized experience and that their prosthetic remains supportive even as environmental and personal factors fluctuate.
6. EMG Pattern Recognition
AI models can interpret electromyographic (EMG) signals from residual muscles, enabling more intuitive and responsive myoelectric prosthetic control by translating subtle muscle activity into precise hand or foot movements.
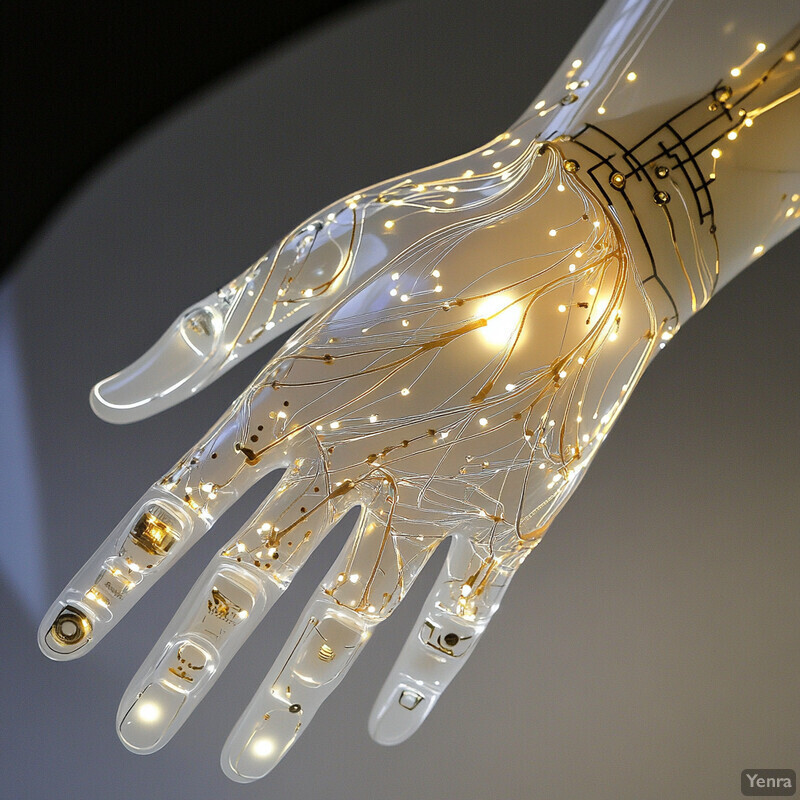
Myoelectric prosthetics rely on muscle signals recorded via electromyography (EMG) to drive limb movement. However, translating raw EMG data into precise and intuitive control commands is challenging. AI-driven pattern recognition systems can analyze complex EMG signals, filter out noise, and identify distinct muscle activation signatures corresponding to desired movements, such as flexing a hand or pointing a foot. These models learn to map subtle muscle patterns into a rich set of output commands, enabling users to perform complex tasks like grasping delicate objects or adjusting their grip strength naturally. As the AI refines its understanding of the user’s unique EMG signals, the prosthetic control becomes more seamless, enhancing the user’s sense of agency and reducing mental effort.
7. Predictive Maintenance and Lifespan Modeling
Machine learning can forecast when a prosthetic component might fail or need maintenance by analyzing stress distribution, user habits, and environmental conditions.
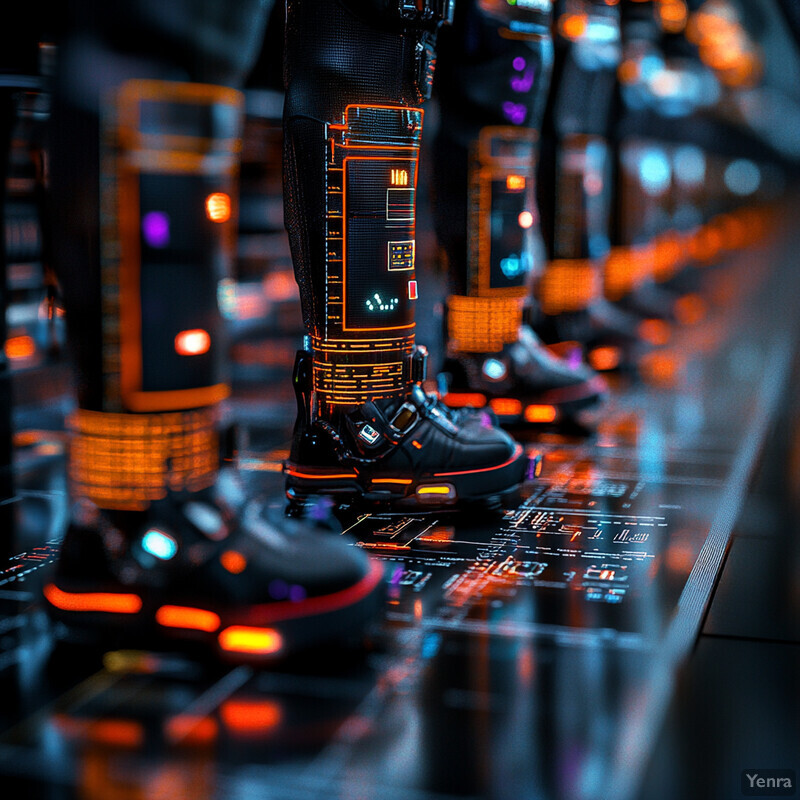
Prosthetics, like any mechanical device, are subject to wear and tear. AI-based predictive maintenance models use sensor data—recording stress, vibrations, and temperature changes—to forecast when components will likely fail or need servicing. By learning from historical maintenance records, material fatigue data, and user feedback, these algorithms can estimate a device’s remaining lifespan and suggest proactive interventions. This minimizes downtime and ensures the prosthetic remains dependable. Patients benefit from timely repairs or component replacements, avoiding sudden device malfunctions and maintaining consistent mobility and quality of life.
8. Digital Twin Environments
AI creates digital twins of patients and prosthetic devices, allowing researchers and clinicians to run virtual experiments, refine designs, and anticipate device performance before physical prototypes are built.
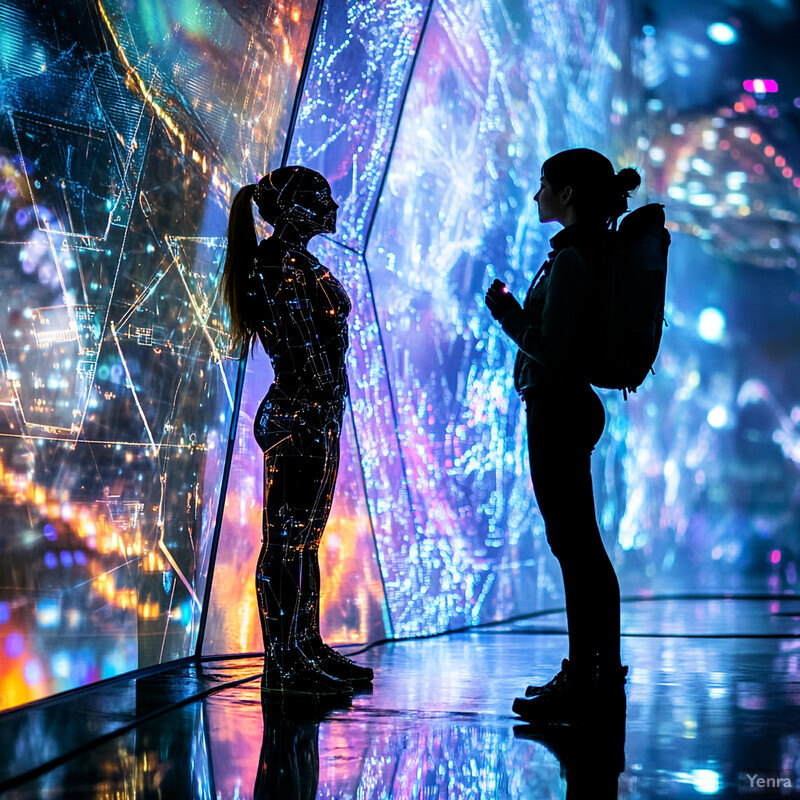
A digital twin is a virtual replica of a physical entity—in this case, the prosthetic and its user—built using AI and simulation technologies. Engineers, clinicians, and researchers can run tests and design iterations in these digital environments without subjecting the patient to physical trials. By adjusting parameters like component materials, joint angles, or even user gait patterns, the digital twin can predict the impact on comfort, efficiency, and endurance. This iterative, simulation-based approach accelerates innovation, reduces prototyping costs, and provides valuable insights that lead to more refined and robust prosthetic solutions. Ultimately, digital twins serve as powerful tools for anticipating user needs and optimizing prosthetic performance before real-world implementation.
9. Neural Interface Optimization
Advanced AI algorithms can improve the interface between the nervous system and robotic prosthetics, optimizing signal interpretation and feedback loops for smoother, more natural movements.
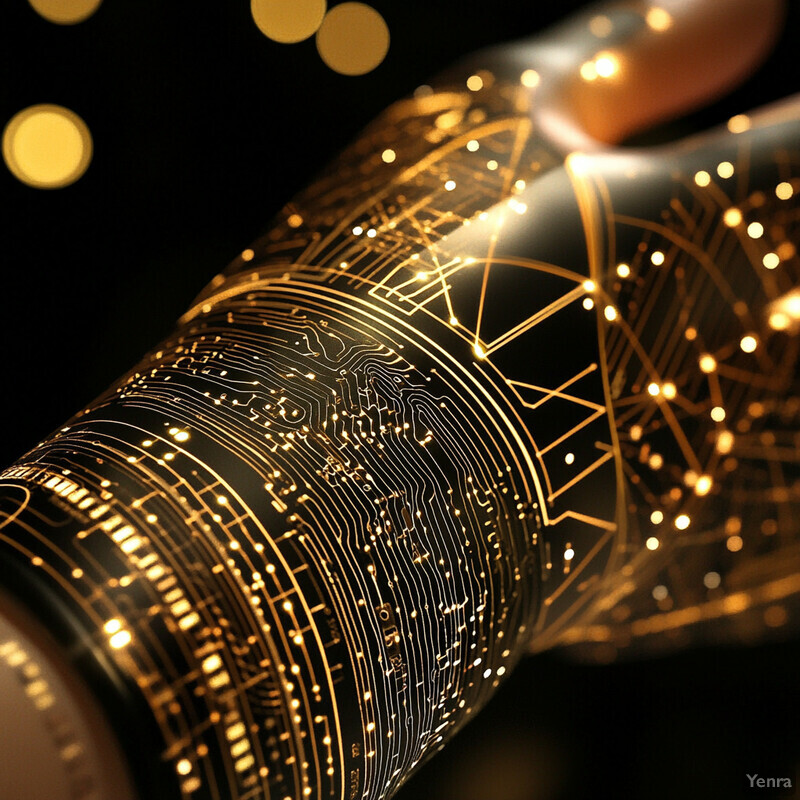
As neural interfaces for prosthetics advance, integrating the nervous system directly with robotic limbs becomes possible. AI algorithms play a crucial role in interpreting complex neural signals and converting them into meaningful control commands. By applying machine learning to neural data, these systems learn the user’s intent more accurately, bridging the gap between biological and mechanical components. This leads to smoother, faster, and more natural movements as the prosthetic essentially becomes “wired” into the patient’s nervous system. Moreover, AI-driven optimization of the neural interface can continuously improve signal quality and reduce noise, ensuring that the prosthetic can respond as closely as possible to the user’s will.
10. Enhanced Comfort and Fit
By applying deep learning to pressure sensor data within sockets, AI can identify hotspots of friction or discomfort, guiding iterative design tweaks for a better fit and reduced patient pain.
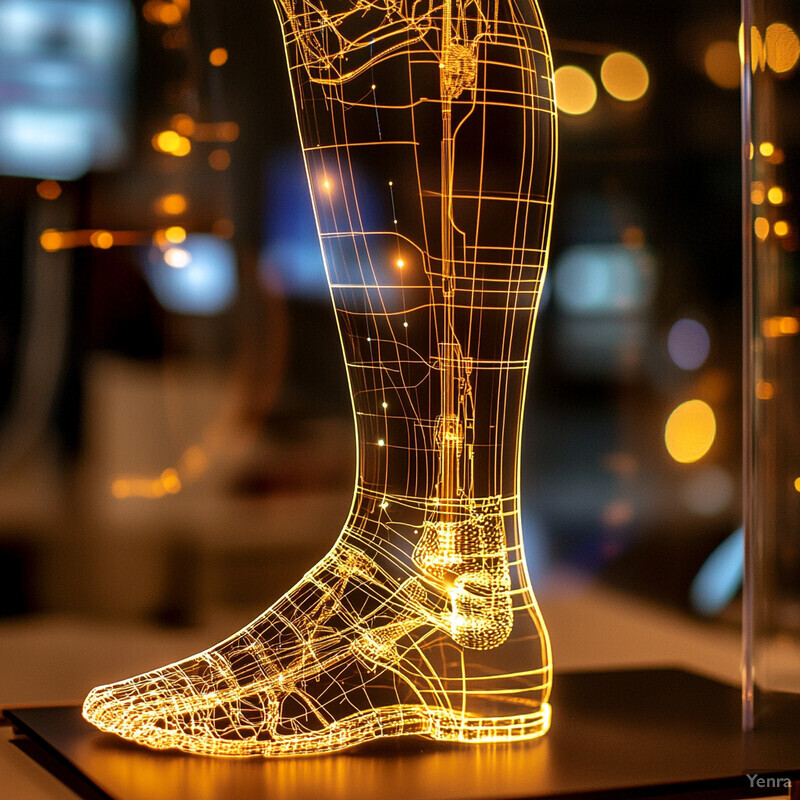
Ensuring a prosthetic fits comfortably requires careful attention to pressure distribution and friction hotspots. AI can gather data from pressure sensors embedded in the prosthetic socket and identify patterns of discomfort or excessive shear forces. By analyzing these patterns, machine learning models can suggest refinements in socket shape or recommend different liner materials to mitigate problems. Over time, iterative improvements lead to a more ergonomically sound interface that reduces pain, chafing, and skin irritation. As comfort improves, patients are more likely to use the prosthetic regularly, fostering better rehabilitation outcomes and improved mobility.
11. Motion Intent Prediction
AI-based intent recognition systems can predict user movements before they occur. This foresight allows the prosthetic to preemptively adjust joint torques for more fluid and effortless motion.
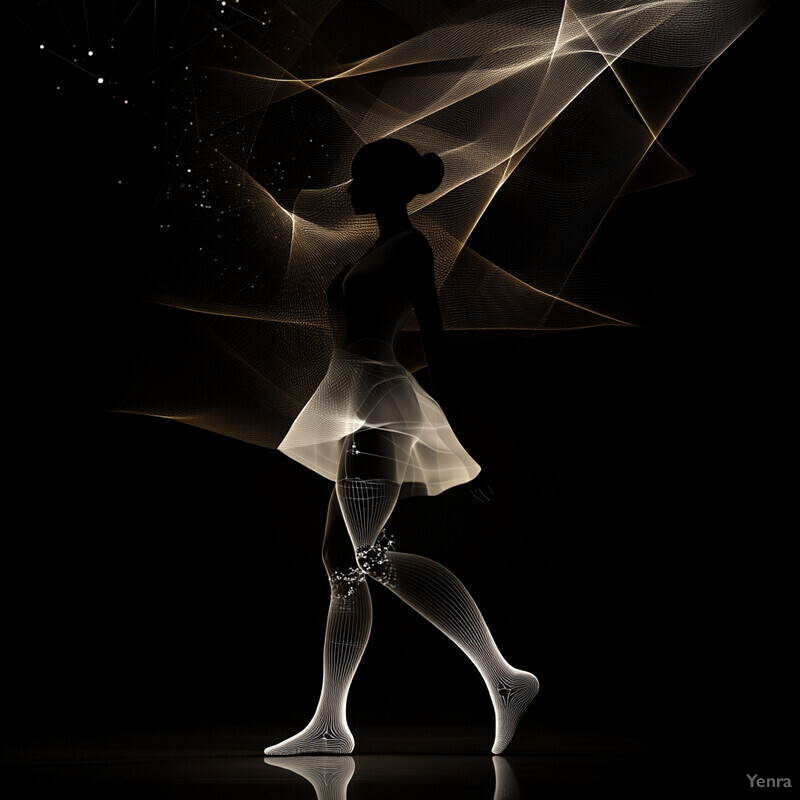
Anticipating user movements before they occur can drastically improve prosthetic responsiveness. AI models trained on historical movement data can learn to detect subtle cues in EMG signals, posture shifts, or biomechanical states that signal the user’s next action. By predicting a step, stride adjustment, or arm movement fractionally ahead of time, the prosthetic can preemptively configure its actuators to produce more fluid, efficient motion. This proactive approach enhances naturalness and stability, especially in challenging scenarios like quickly adjusting to sudden obstacles or changing gait speed. Over time, the prosthetic “learns” the user’s unique behavioral patterns, reducing cognitive load and helping them feel more confident and secure in daily activities.
12. Adaptive Impedance Control
Utilizing machine learning, prosthetic devices can modulate impedance in real time, altering stiffness and damping properties to enhance stability and adaptability to uneven terrains.
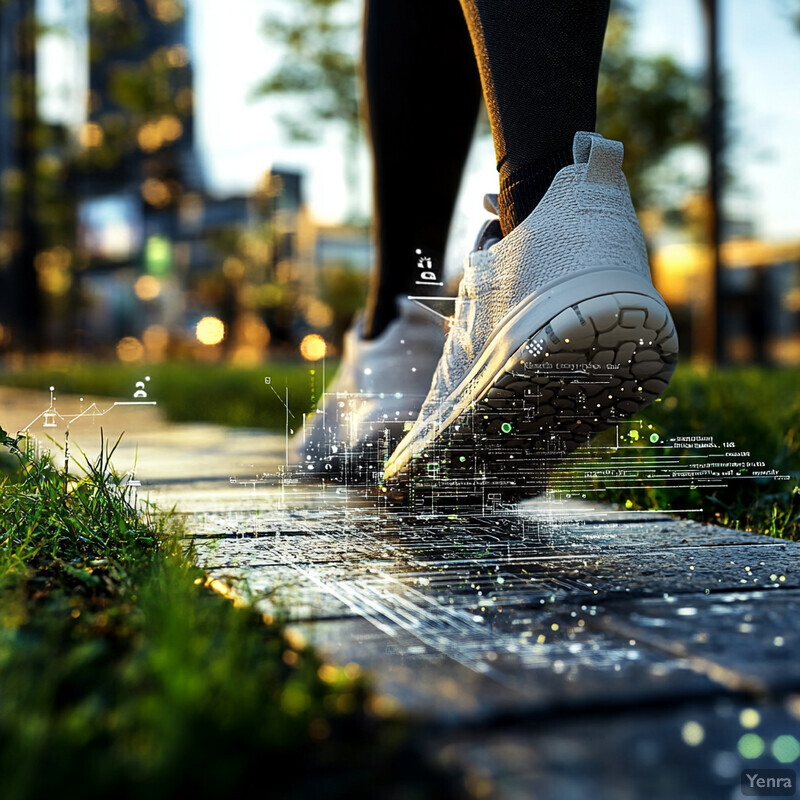
Impedance control refers to modulating joint stiffness and damping properties to achieve smooth, stable limb movements. AI can monitor user feedback and environmental conditions—like ground slope or surface irregularities—and adjust impedance parameters in real time. By doing so, the prosthetic can provide the right balance of stability and flexibility, ensuring stable support on uneven ground but also allowing a gentle, shock-absorbing gait on flat surfaces. These adaptive changes enhance comfort, reduce the risk of falls, and help users maintain a natural walking style even as conditions vary. Ultimately, adaptive impedance control supports a more intuitive user experience, allowing individuals to focus less on controlling their prosthetic and more on living actively.
13. Complex Joint Modeling
AI techniques can model the intricate interactions of multiple joints under load, providing insights into how altering one joint’s stiffness or alignment affects the overall biomechanics of the prosthetic-user system.
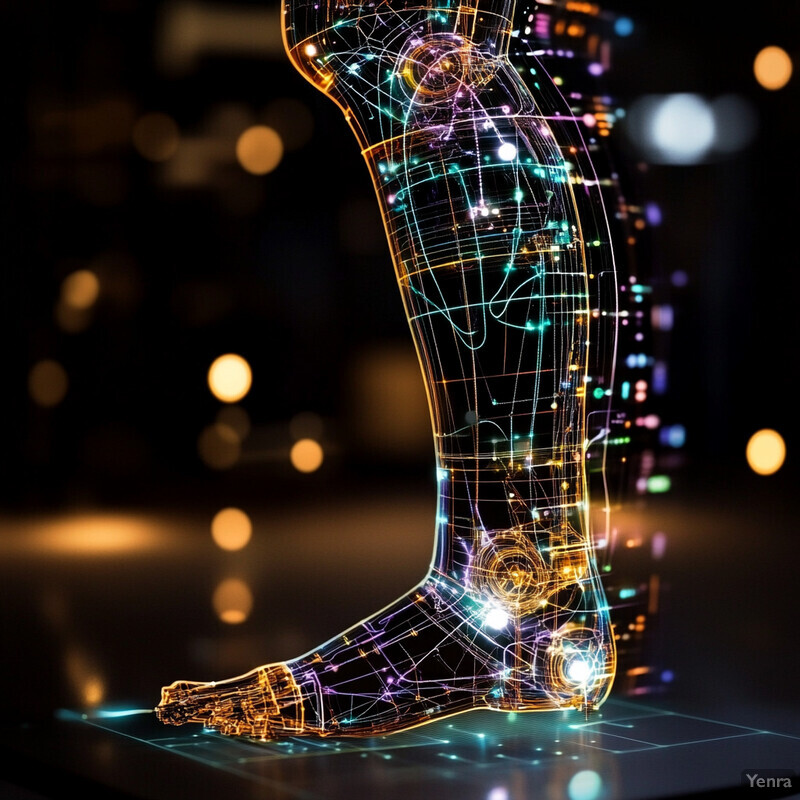
Human movement is a symphony of interacting joints and tissues, and prosthetics must integrate seamlessly into this biomechanical orchestra. AI can model these intricate interactions, learning how altering one joint’s parameters affects forces and angles in others. By processing large, multivariate datasets from motion capture, electromyography, and force sensors, these models can uncover hidden relationships that guide prosthetic joint design. This holistic perspective ensures that when adjustments are made—like stiffening an ankle component or changing knee alignment—the resulting biomechanical effects across the limb are well understood. The outcome is a prosthetic that meshes naturally with the user’s entire musculoskeletal system, promoting healthier long-term biomechanics.
14. Virtual Rehabilitation Tools
AI-driven simulations help therapists and patients explore different exercises and training routines, enabling personalized rehabilitation protocols that improve prosthetic use and user confidence over time.
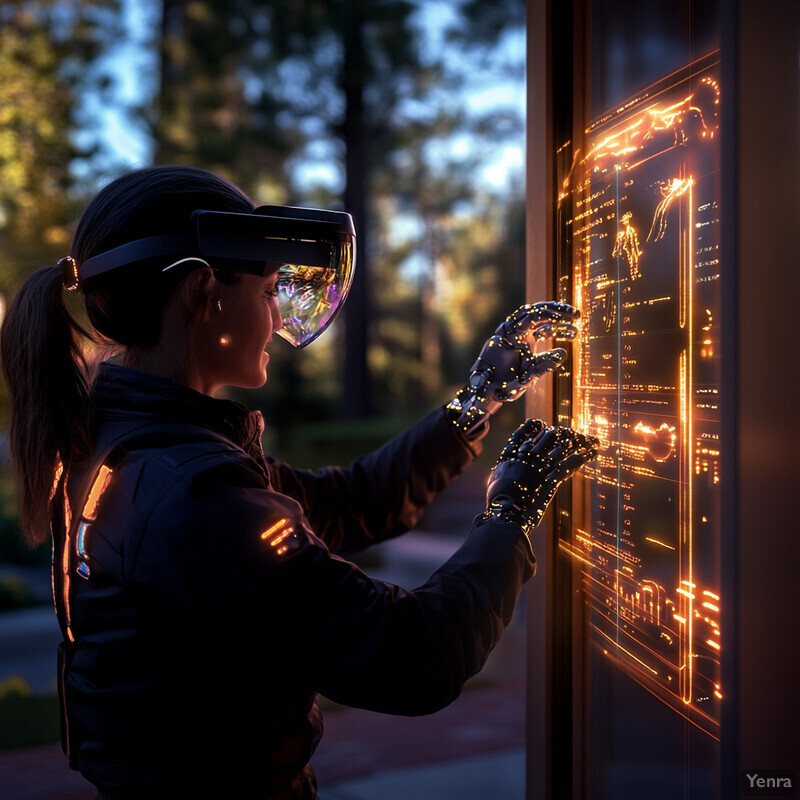
Rehabilitation following prosthetic fitting often involves repetitive exercises and careful monitoring by a therapist. AI-driven virtual environments can simulate various exercises and track user progress in real time, providing instant feedback on movement quality and alignment. Patients can practice walking simulations, balance training, or object manipulation exercises in a safe, controlled virtual space, while machine learning algorithms assess improvements in posture and motor control. Over time, the AI can adapt these rehabilitation routines to individual needs, making them more challenging as the user’s abilities improve. This personalized, data-driven approach to rehabilitation accelerates skill acquisition, enhances patient engagement, and leads to better overall prosthetic use.
15. Feature Extraction from Wearable Sensors
Sophisticated AI methods can process large amounts of multi-modal sensor data—force plates, accelerometers, inertial measurement units—to extract meaningful features and insights for refining prosthetic control strategies.
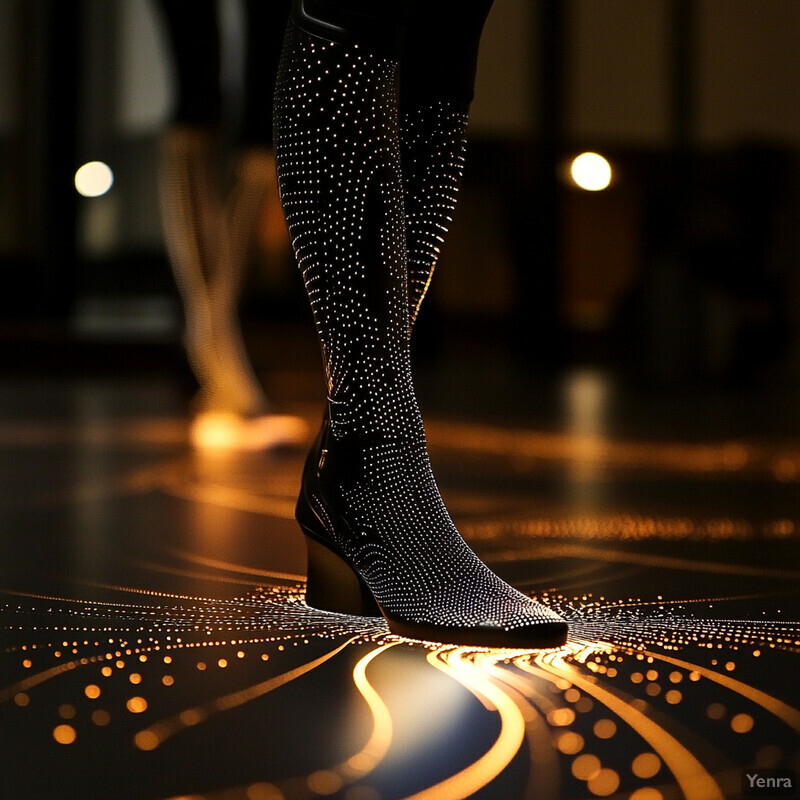
Wearable sensors—including force plates, accelerometers, and inertial measurement units—generate a continuous stream of rich biomechanical data. AI-driven signal processing techniques can distill this data into meaningful features, such as gait stability metrics, joint angle trajectories, or energy expenditure indicators. By highlighting key patterns and correlations, these features guide engineers and clinicians in refining prosthetic hardware and software parameters. The end result is a prosthetic more finely tuned to the user’s biomechanics, offering improved stability, efficiency, and responsiveness. As more data is collected and analyzed, the prosthetic’s control system becomes increasingly adept at responding to the user’s unique movement patterns and evolving physical capabilities.
16. User-Environment Interaction Prediction
Machine learning models can learn patterns of how prosthesis users interact with varying environmental factors (stairs, slopes, uneven ground) and adjust prosthetic settings accordingly for enhanced stability.
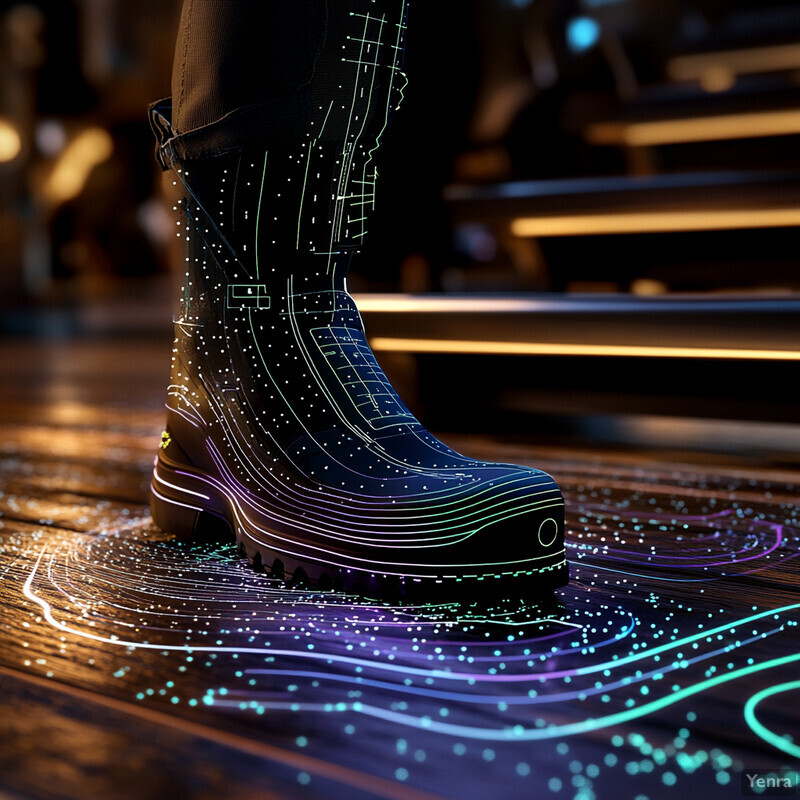
When navigating real-life environments, prosthetic users must adapt to a range of conditions—from climbing stairs to walking on grass or gravel. AI can predict how a user interacts with different terrains, inclines, and obstacles by combining sensor data and historical usage patterns. These predictive models can then adjust prosthetic control strategies, choosing appropriate stiffness, torque, or damping levels to ensure stable, confident movement. By anticipating challenges before the user encounters them, the prosthetic reduces the likelihood of stumbles or falls, instilling greater confidence in the wearer. Over time, as the AI learns from new experiences, these environment-aware adjustments become more refined, further enhancing mobility and independence.
17. Robust Error Detection and Correction
AI systems can identify anomalies in prosthetic function, such as joint misalignments or actuator drift, and automatically correct these errors on the fly.
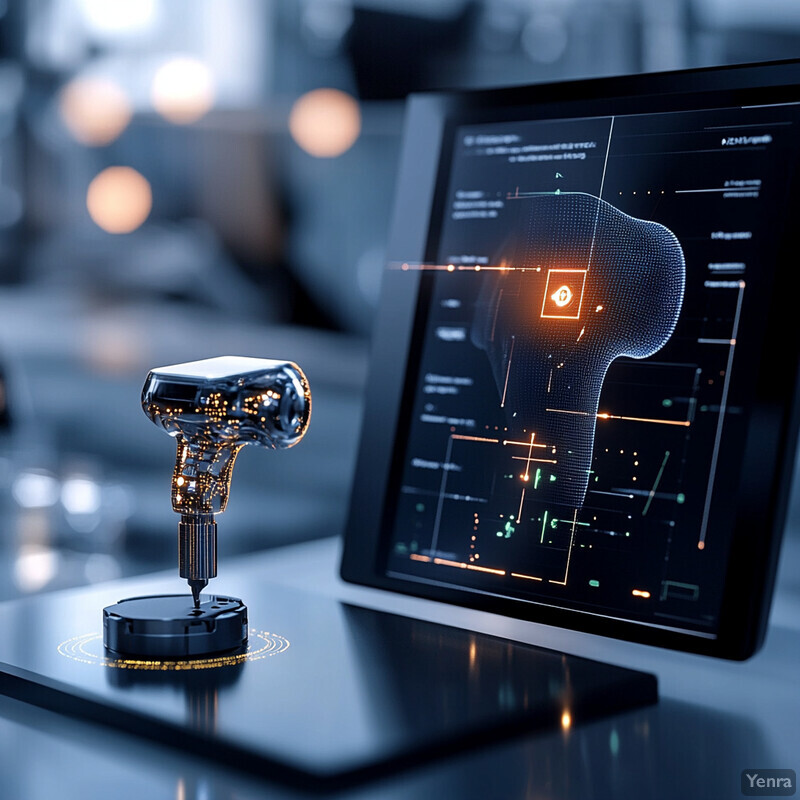
Mechanical devices, especially those worn daily, can experience calibration drift, misalignments, or sensor faults. AI-powered monitoring systems can detect these errors early by comparing real-time sensor data against statistical models of normal operation. When anomalies occur, the AI can trigger corrective measures—like recalibrating joint alignment or adjusting control algorithms—ensuring the prosthetic remains reliable and safe. This proactive approach minimizes downtime and user frustration, as minor issues are resolved before they cause significant discomfort or performance degradation. In essence, AI helps maintain a high standard of prosthetic function over the device’s entire service life.
18. Population-Level Design Insights
By analyzing data from large user populations, AI can identify common biomechanical trends and design principles that apply across many patients, leading to more universally effective prosthetic templates.
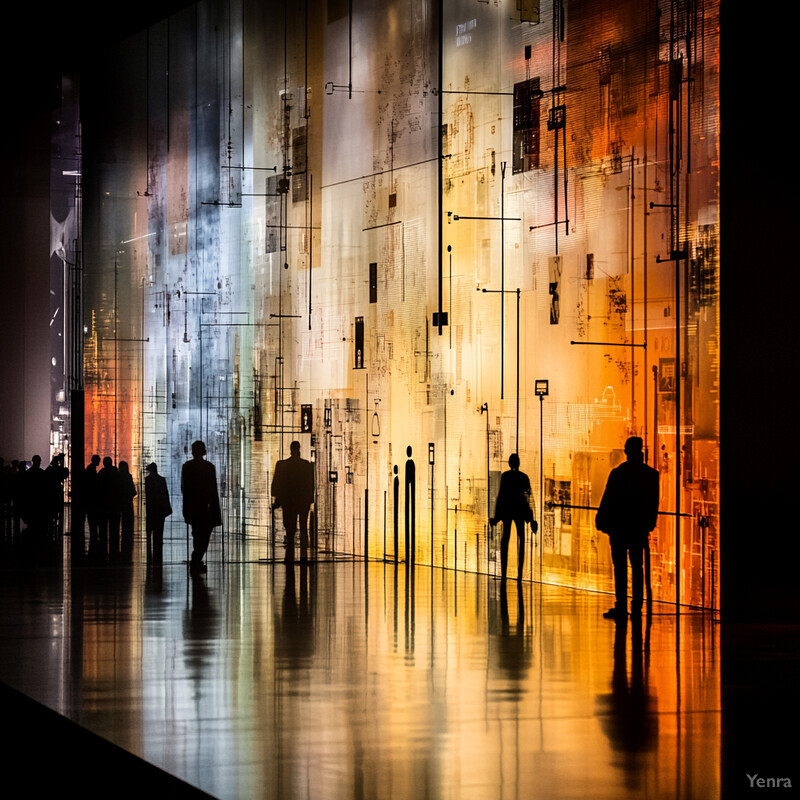
No two patients are identical, but analyzing data from large patient populations can reveal valuable trends in prosthetic design and performance. By applying AI to aggregated datasets, researchers can identify which design elements—such as foot shape, joint stiffness profiles, or socket materials—tend to produce the best outcomes across different user groups. These insights serve as a blueprint for refining general prosthetic templates and guiding custom solutions. The resulting knowledge transfer accelerates innovation, reduces guesswork, and leads to prosthetics that are more likely to achieve optimal comfort, durability, and functionality for a broad range of patients.
19. Rapid Prototyping Through Simulation
AI accelerates the prosthetic design cycle by rapidly simulating multiple design iterations and testing their biomechanical performance digitally, drastically reducing time and cost.
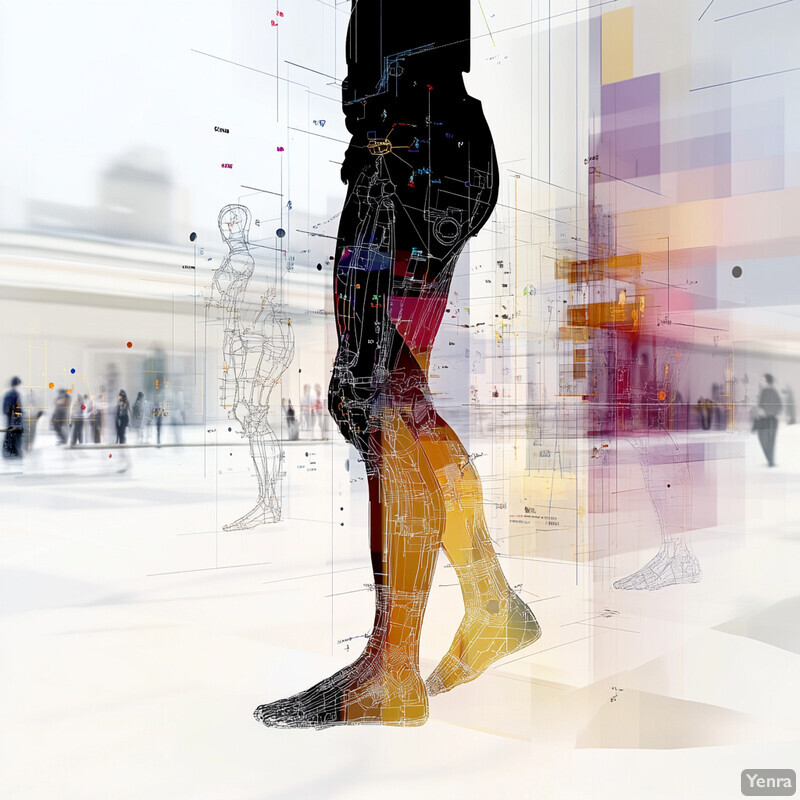
Prototyping prosthetic designs involves iterative cycles of testing and refinement. AI accelerates this process by simulating multiple design configurations digitally, assessing their biomechanical performance before any physical component is manufactured. This reduces material waste, lowers costs, and significantly shortens the development timeline. Instead of manually building and testing each prototype, designers can rely on AI-driven simulations to rapidly converge on the most promising options. Once the best candidate emerges, the final prototype and subsequent device are much closer to the ideal solution, ensuring patients benefit from innovative prosthetics sooner.
20. Longitudinal Performance Tracking
Over time, AI models can learn from continuous usage data, adapting to changes in a patient’s gait, muscle strength, or activity levels and suggesting adjustments that maintain optimal prosthetic function.
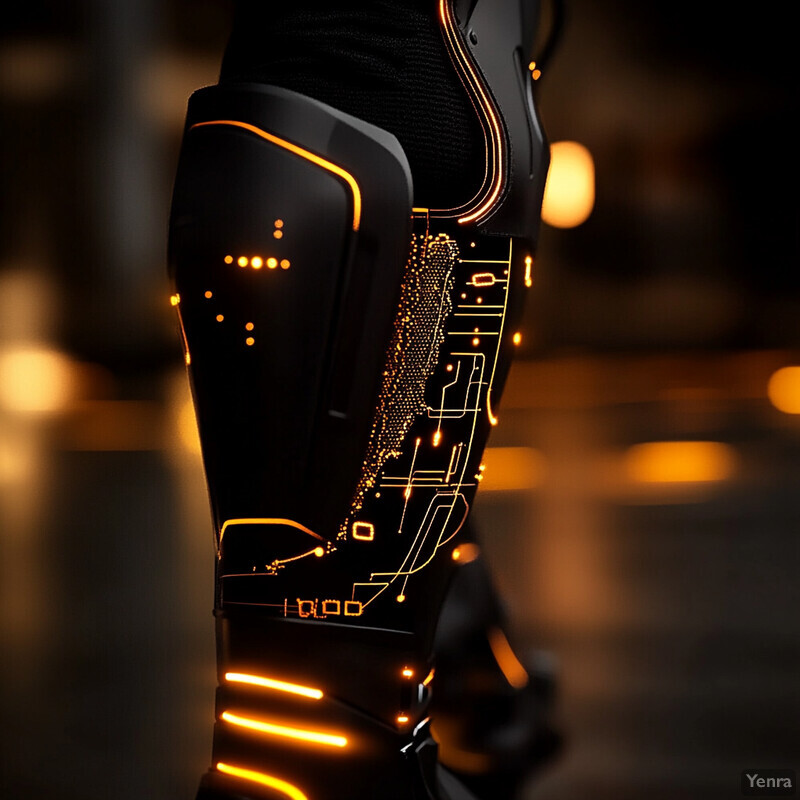
As a patient’s condition evolves—muscle strength changes, gait patterns shift, or new environmental demands arise—the ideal prosthetic configuration may need to be updated. AI systems can track changes in performance over months and years, analyzing sensor data and usage metrics to identify gradual trends. When these trends suggest the prosthetic needs recalibration or component upgrades, the AI can alert clinicians and users proactively. Continuous longitudinal tracking ensures that the prosthetic remains aligned with the user’s current capabilities, maintaining optimal comfort, functionality, and support. In this way, AI not only enhances initial fitting and performance but also ensures longevity and adaptability over the prosthetic’s entire lifecycle.