1. Automated Species Identification
AI algorithms, especially deep learning models, can efficiently analyze large acoustic datasets to recognize vocalizations from multiple species, reducing the need for time-consuming manual classification.
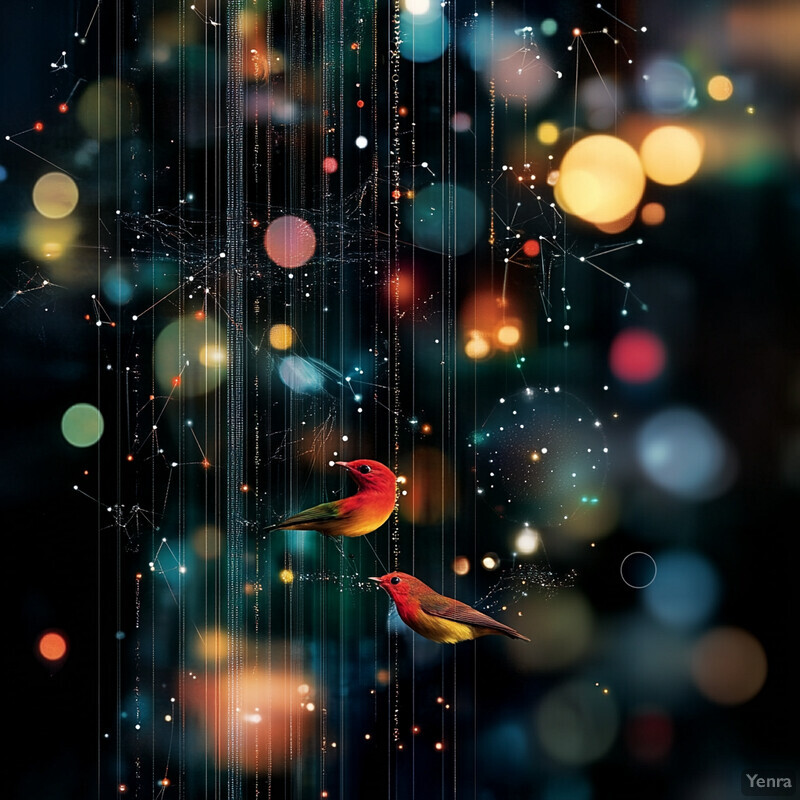
Traditionally, identifying species by their vocalizations required extensive domain expertise and tedious manual listening. With advanced AI models, particularly deep learning neural networks trained on large datasets of annotated calls, researchers can now automatically recognize species-specific vocal signals with remarkable accuracy. This enables rapid processing of extensive field recordings, allowing scientists to identify rare or elusive species even in complex soundscapes. Such automation not only saves time and effort but also reduces observer bias, leading to more consistent and reproducible results. As a consequence, automated species identification aids in monitoring biodiversity, informing conservation priorities, and accelerating ecological research.
2. Enhanced Signal Denoising
Advanced signal processing models can separate animal calls from background noise in real time, improving data quality and allowing researchers to record in challenging acoustic environments.
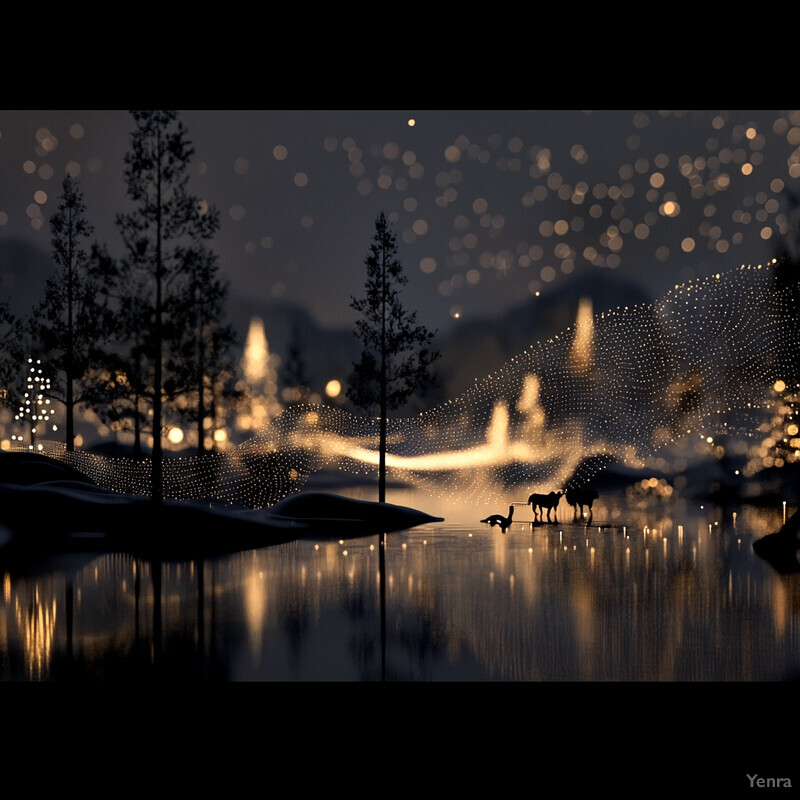
Field recordings often include a cacophony of non-target sounds—wind, rain, human-made noise, and other environmental interferences—that can mask important animal calls. AI-powered signal processing techniques, including advanced filtering, source separation algorithms, and deep learning-based denoising models, help isolate target species vocalizations from background noise. By improving the signal-to-noise ratio, researchers can more confidently detect and analyze faint or subtle calls that would otherwise be lost. This technology ensures higher-quality datasets, ultimately allowing for better assessments of species presence, behavior, and ecosystem health under real-world, noisy conditions.
3. Efficient Call Detection
Machine learning methods help detect and segment individual calls or songs from continuous audio recordings, enabling more precise event-based analyses.
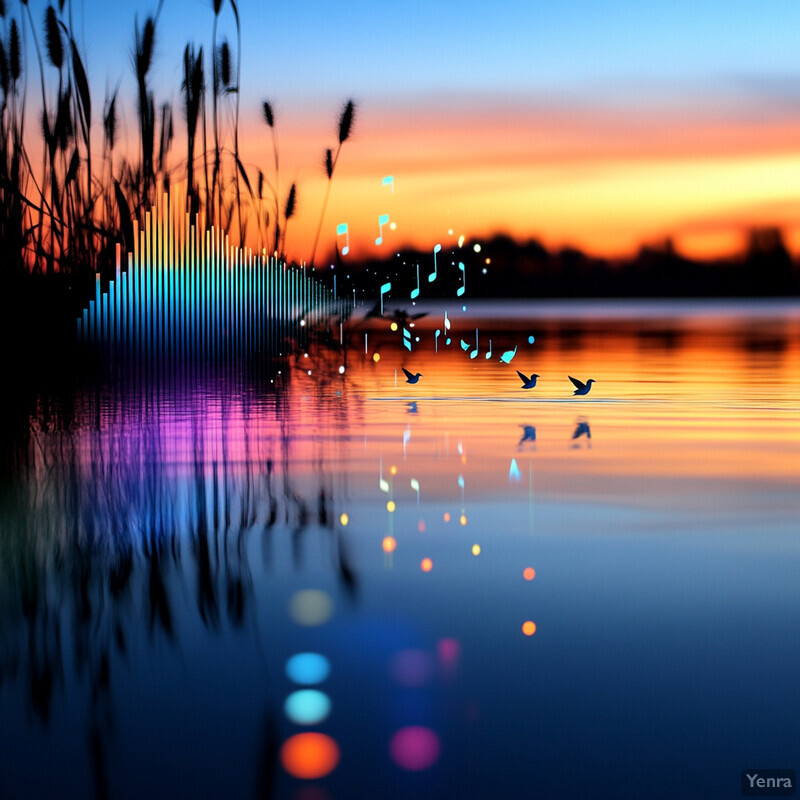
Before the rise of AI, identifying calls within hours or days of audio recordings required painstaking manual scanning. Now, machine learning-driven call detectors rapidly pinpoint the start and end of vocal events. By leveraging algorithms trained on representative samples, these tools can automatically parse continuous recordings, extract relevant segments, and flag those for further review. This efficiency frees researchers from the cumbersome task of manually combing through large datasets, enabling larger spatial and temporal coverage of ecosystems. As a result, scientists can more readily detect subtle ecological patterns and track species activity over time.
4. Acoustic Event Classification
AI can classify complex acoustic events, such as mating calls, alarm signals, or territorial songs, to better understand animal behaviors and ecological interactions.
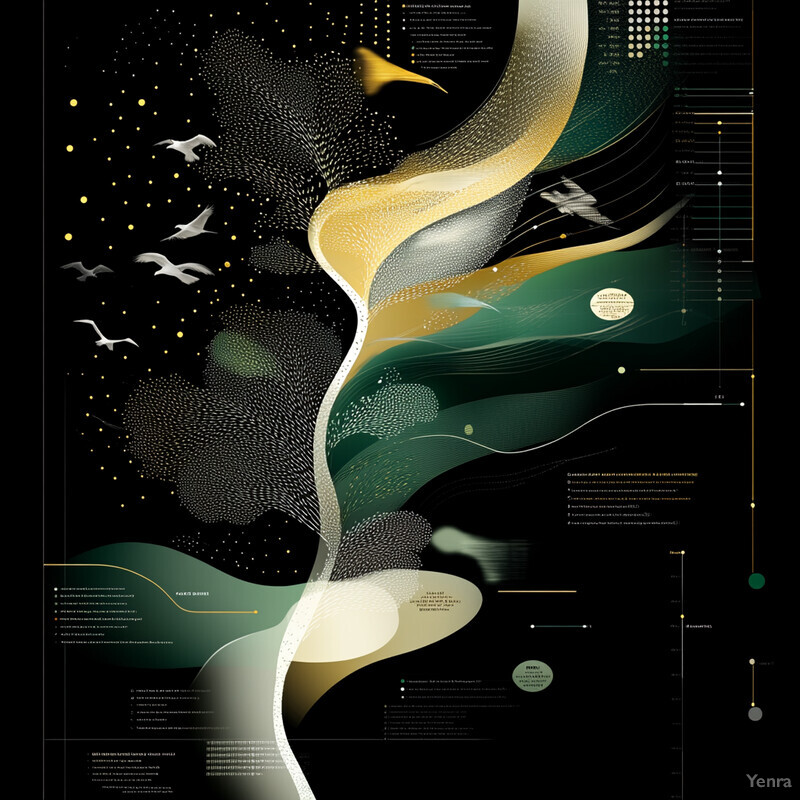
Beyond detecting simple calls, AI has enabled detailed classification of entire acoustic events. This can involve distinguishing between different behavioral contexts—such as alarm calls, mating songs, or territorial signals—and differentiating among multiple species vocalizing simultaneously. By training deep learning models on labeled acoustic events, researchers can categorize a multitude of complex sounds. This nuanced understanding supports more in-depth ecological interpretations, helping to reveal how species communicate, how they respond to environmental changes, and how individual events contribute to broader ecosystem dynamics.
5. Multi-species Monitoring
AI models can simultaneously handle multiple taxa, allowing researchers to gather broad-scale biodiversity data from a single set of recordings.
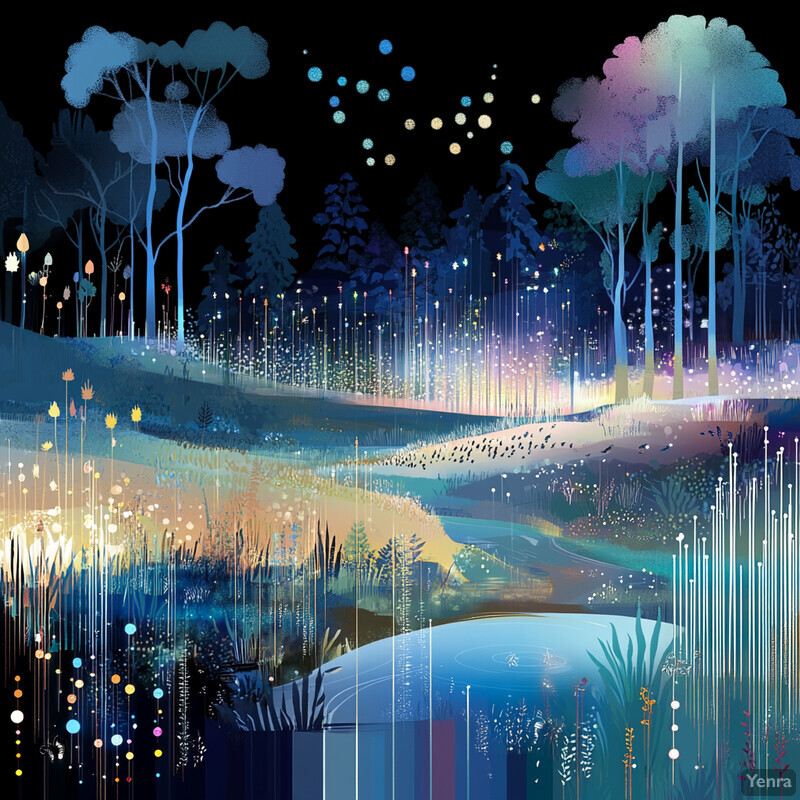
One of the biggest challenges in bioacoustics is dealing with ecosystems where dozens or even hundreds of species vocalize concurrently. AI-driven multi-species classification models can handle this complexity by identifying multiple taxa from a single recording, thus providing a holistic snapshot of community composition. This simultaneous monitoring approach saves researchers from needing multiple specialized systems and allows for integrative biodiversity assessments. As a result, conservationists and ecologists can track ecosystem health more comprehensively and efficiently, detecting changes in species assemblages and identifying emerging ecological threats at a broader scale.
6. Adaptive Sampling Strategies
Intelligent sensors, guided by AI-driven insights, can decide when and where to record based on learned patterns of animal activity, optimizing resource usage.
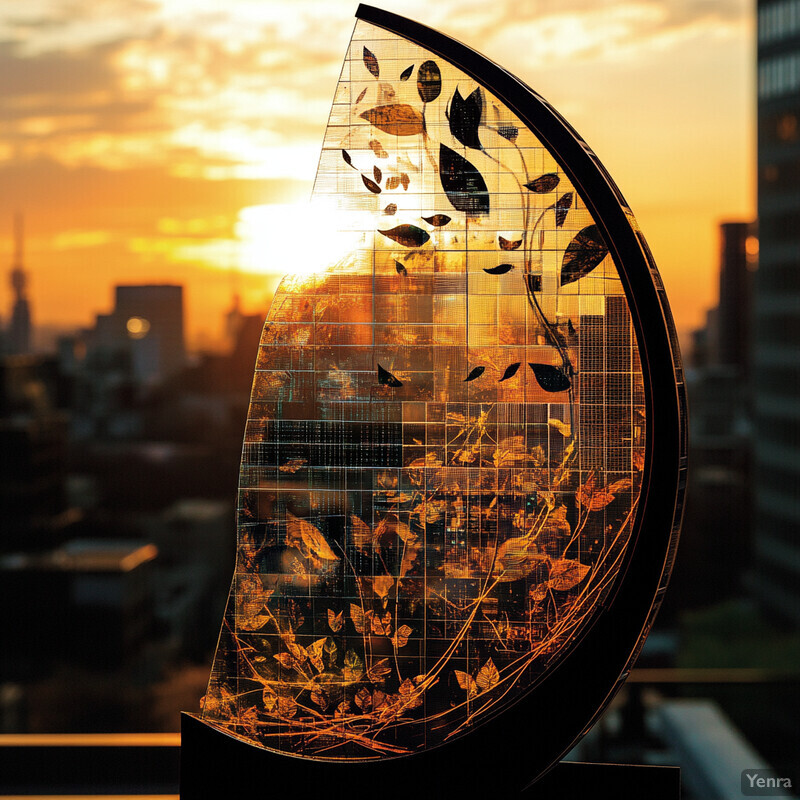
Instead of passively recording sounds around the clock, modern acoustic sensors guided by AI can dynamically alter their recording schedules in response to real-time patterns. For example, if the AI detects a certain species call or peak activity periods, it can activate more frequent or targeted recording intervals. This adaptability conserves battery life, storage space, and field effort, making long-term studies more sustainable. In essence, sensors become “smart” listeners that hone in on events of interest, ensuring that data collection is both resource-efficient and more likely to capture critical ecological information.
7. Real-time Monitoring
On-device AI models can process recordings as they are collected, providing immediate feedback for field researchers.
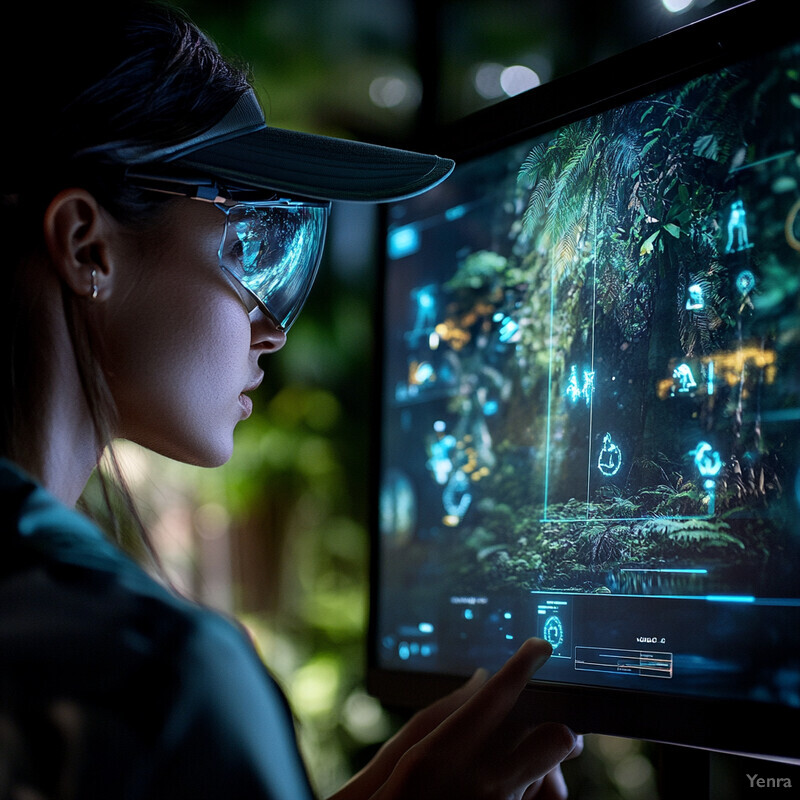
With the computational power of edge devices and embedded AI models, it is now possible to process and interpret acoustic data as it is being captured in the field. By conducting near-instantaneous analysis, these systems can alert researchers when certain target species vocalize or when unusual acoustic events occur. This real-time feedback lets field teams adjust their sampling efforts on the fly, respond to threats such as poaching or invasive species, and even engage in immediate mitigation strategies. Real-time monitoring thus transforms data collection from a static, retrospective process into a dynamic, interactive tool for conservation and research.
8. Temporal and Spatial Pattern Analysis
AI-driven analytics can uncover subtle temporal and spatial patterns in vocal activity, helping to understand species behaviors across time and habitats.
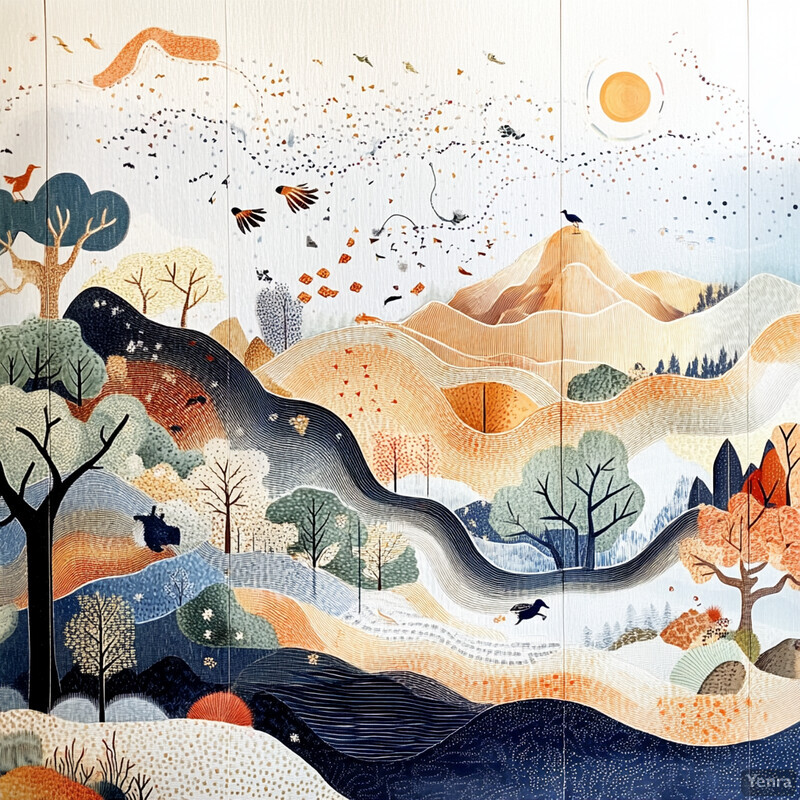
Understanding when and where species vocalize is key to unraveling their ecology. AI-driven analytics can reveal daily, seasonal, and annual patterns in acoustic activity, as well as differences in species presence across habitats. By integrating advanced modeling techniques, these systems can correlate vocalization patterns with environmental factors—such as temperature, precipitation, or vegetation structure. The resulting insights help ecologists understand the drivers behind acoustic behaviors, predict how global changes might alter vocal activity, and ultimately guide targeted conservation actions based on identified spatial and temporal trends.
9. Automated Quality Control
Algorithmic methods can assess recording quality, filtering out poor-quality segments and ensuring more reliable datasets.
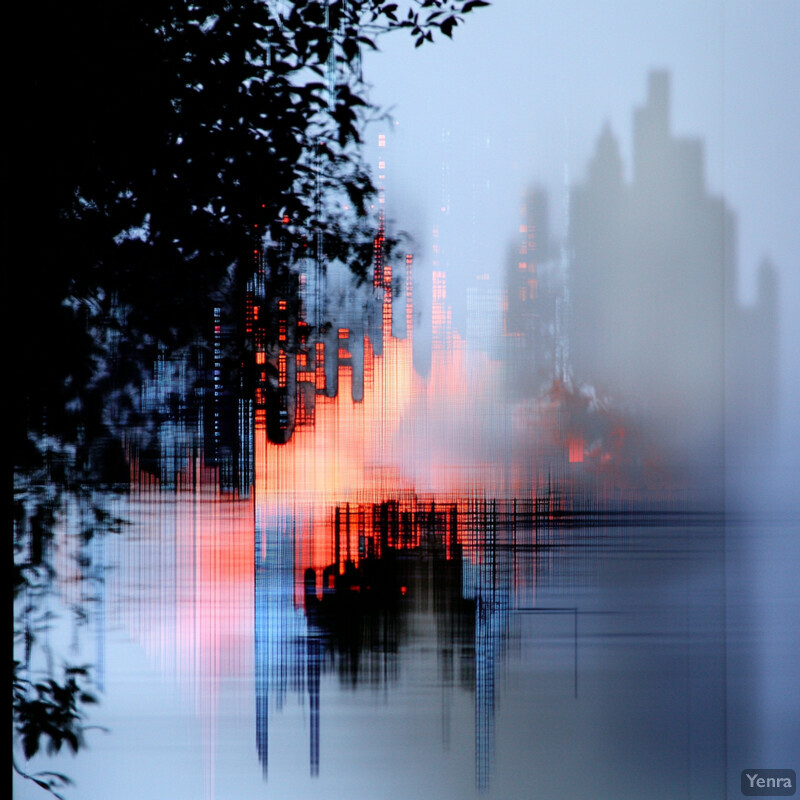
Large acoustic datasets often contain corrupted files, equipment malfunctions, or low-quality segments that interfere with subsequent analyses. AI-powered quality control systems automatically assess recording quality, flagging problems like microphone distortion, mechanical interference, or unusually high noise floors. By filtering out problematic data upfront, researchers improve the reliability of their downstream analyses. Consequently, more consistent datasets lead to more robust ecological insights, ensuring that time and resources are spent on interpreting meaningful data rather than sifting through unusable recordings.
10. Population Density Estimation
By modeling the relationship between call rates and population numbers, AI can help estimate animal densities for conservation planning.
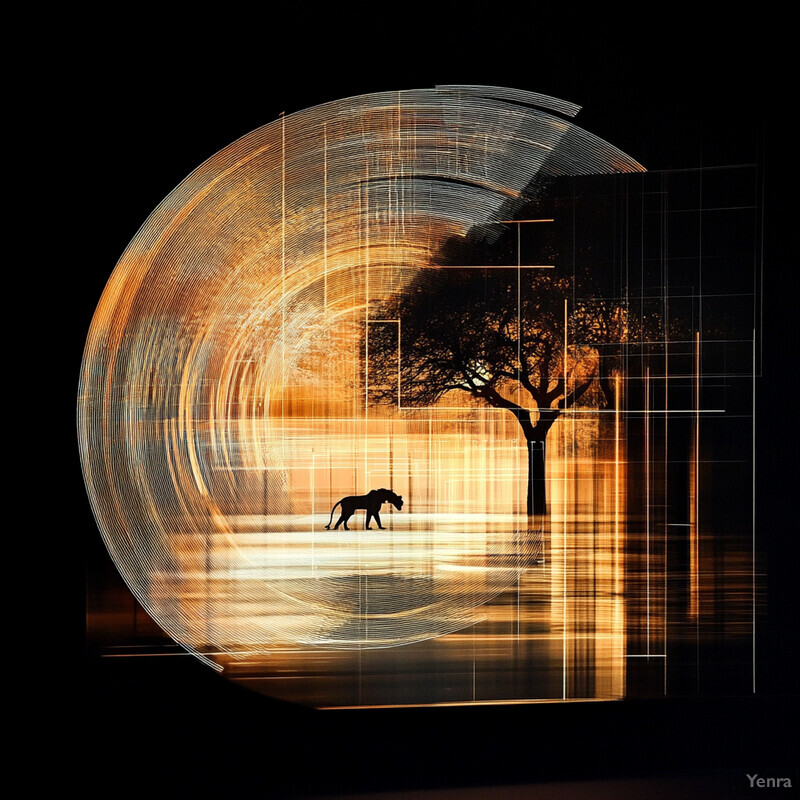
Some acoustic signals correlate with population densities, allowing researchers to infer how many individuals of a species might inhabit a given area. With AI-driven models, scientists can establish statistical relationships between call rates and actual population counts, often validated through field surveys. Over time, these models can track population trends, provide early warnings of declines, and inform management strategies for at-risk species. Population density estimation from acoustics thus becomes more accurate, scalable, and accessible, supporting data-driven conservation and reducing the need for intrusive or labor-intensive survey methods.
11. Vocal Repertoire Characterization
AI-driven clustering can group call types, helping to describe a species’ full vocal repertoire and understand their communication systems.
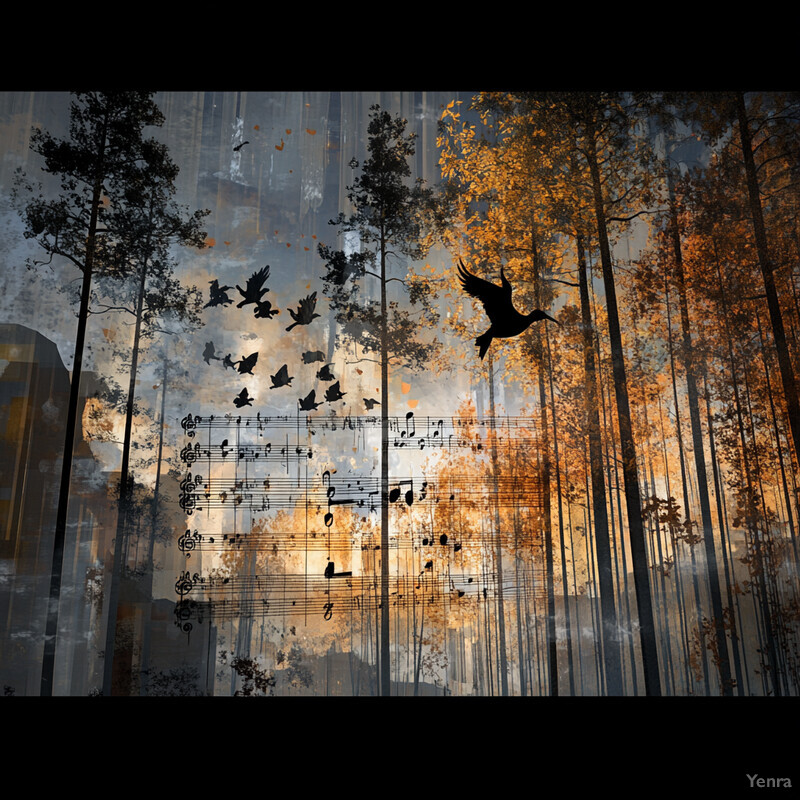
Many species produce a variety of call types—different signals for courtship, territory defense, alarm, or social bonding. Clustering algorithms and deep learning models can identify and group similar call types from large datasets. By systematically characterizing a species’ full vocal repertoire, researchers gain deeper insights into communication complexity, social structures, and behavioral ecology. Such comprehensive catalogs provide baseline references for future studies, help detect shifts in vocal behaviors over time, and may shed light on how animals adapt their communication strategies in changing environments.
12. Behavioral Context Recognition
Contextual machine learning models can link specific acoustic signals to environmental conditions or behaviors, aiding interpretation.
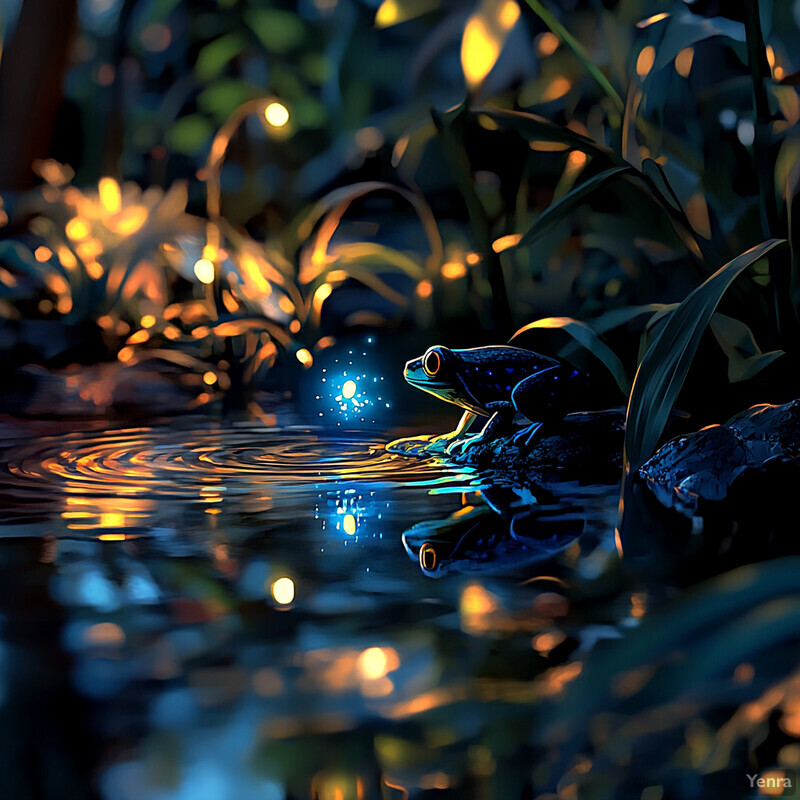
By integrating acoustic data with information about the environment and animal activities, AI models can infer the behavioral context underlying specific vocalizations. For instance, certain calls might coincide with feeding events, mating displays, or predator presence. Drawing on context-rich datasets, machine learning models can discern these connections, allowing researchers to interpret the “meaning” behind the sounds. This level of understanding is critical for behavioral ecology, as it clarifies how animals respond to their surroundings, how they communicate essential information, and how these patterns may shift in response to environmental changes.
13. Long-term Trend Analysis
AI can detect subtle changes in call frequency or amplitude over long periods, revealing shifts in population health or habitat conditions.
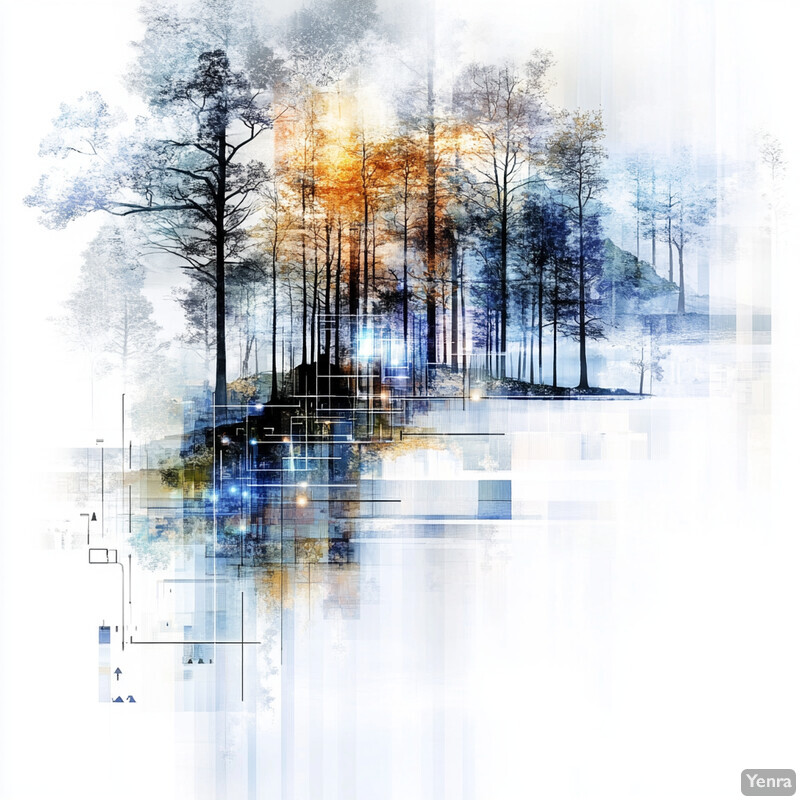
Over years or even decades, subtle changes in call frequency, duration, or amplitude can signal significant ecological shifts—such as habitat degradation, climate-driven migrations, or anthropogenic disturbances. AI-powered trend detection tools can analyze massive historical datasets to identify these gradual patterns. By recognizing long-term trends, researchers can distinguish natural variability from concerning shifts in ecosystem health. Such insights are crucial for predicting future changes, implementing proactive conservation measures, and ensuring that policies and management plans align with ecological realities.
14. Anomaly Detection
ML algorithms can highlight unusual acoustic events—such as invasive species calls—triggering early interventions.
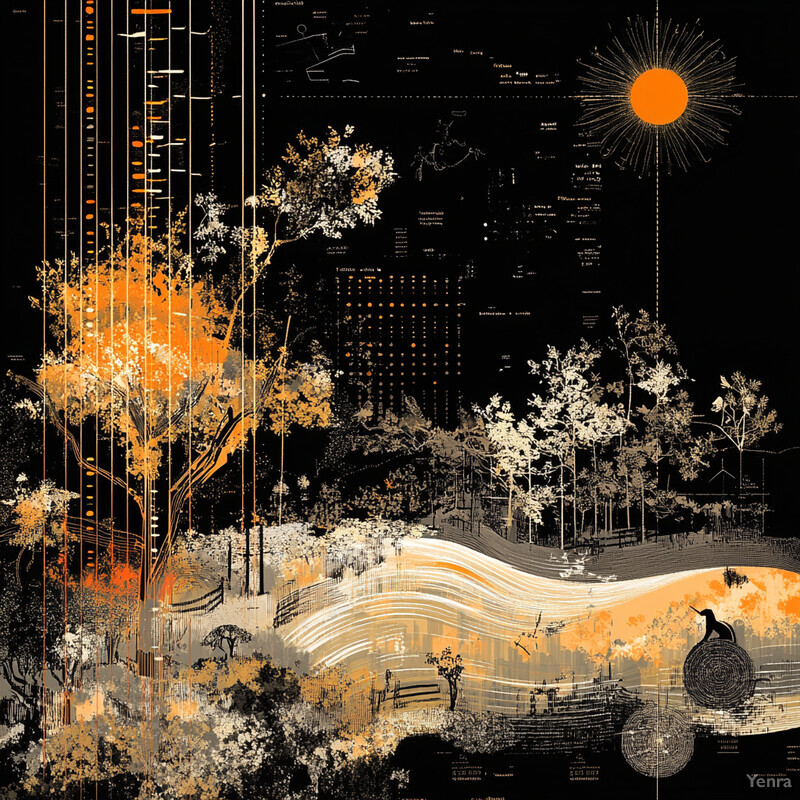
Not all changes in acoustic environments follow predictable patterns. Sometimes, sudden anomalies—such as an invasive species’ call, an unexpected predator’s presence, or a rare vocalization type—can have outsized ecological importance. AI-based anomaly detection systems are trained to spot these irregular events. Early detection of anomalies allows quick intervention, such as eradicating invasive species before they establish or mitigating unforeseen anthropogenic disturbances. In this way, anomaly detection serves as an acoustic early warning system, enhancing the responsiveness and agility of conservation and management efforts.
15. Predictive Modeling
AI models can predict future occurrences of certain acoustic events based on historical trends, aiding proactive management.
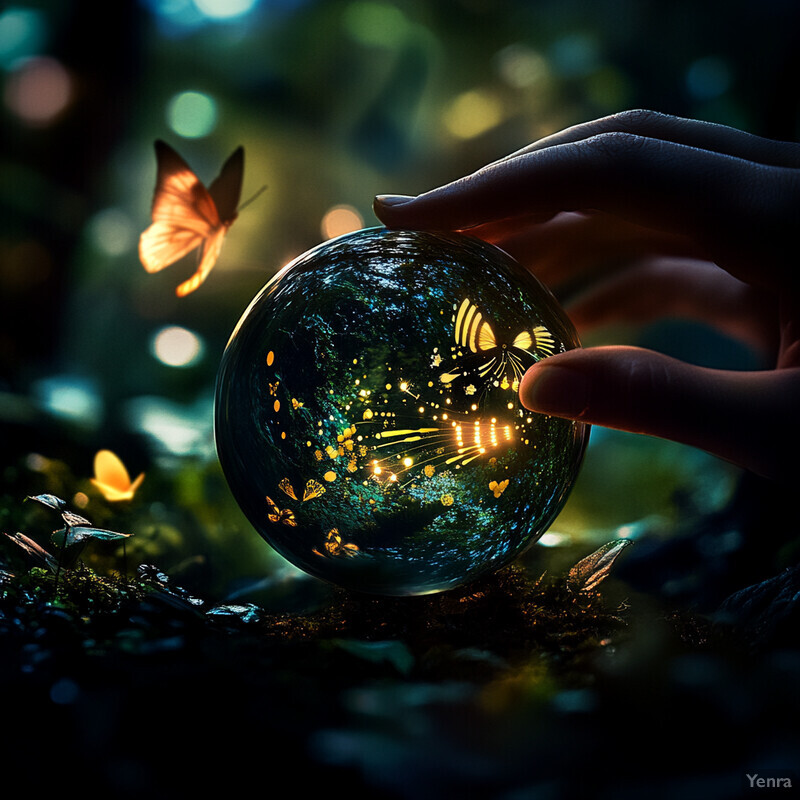
Historical acoustic data combined with AI-powered predictive modeling can forecast future occurrences of certain acoustic events. By recognizing patterns and correlating them with known drivers—like seasonal migrations, breeding cycles, or climatic shifts—models can predict when and where particular species will vocalize. This foresight aids in proactive management actions, such as timing protective measures or habitat restorations to coincide with critical life history events. Predictive modeling thus helps conservation practitioners anticipate ecological needs rather than simply reacting to changes after they occur.
16. Transfer Learning and Domain Adaptation
Pre-trained acoustic models can be adapted to new species or environments with minimal extra data, speeding up large-scale bioacoustic monitoring.
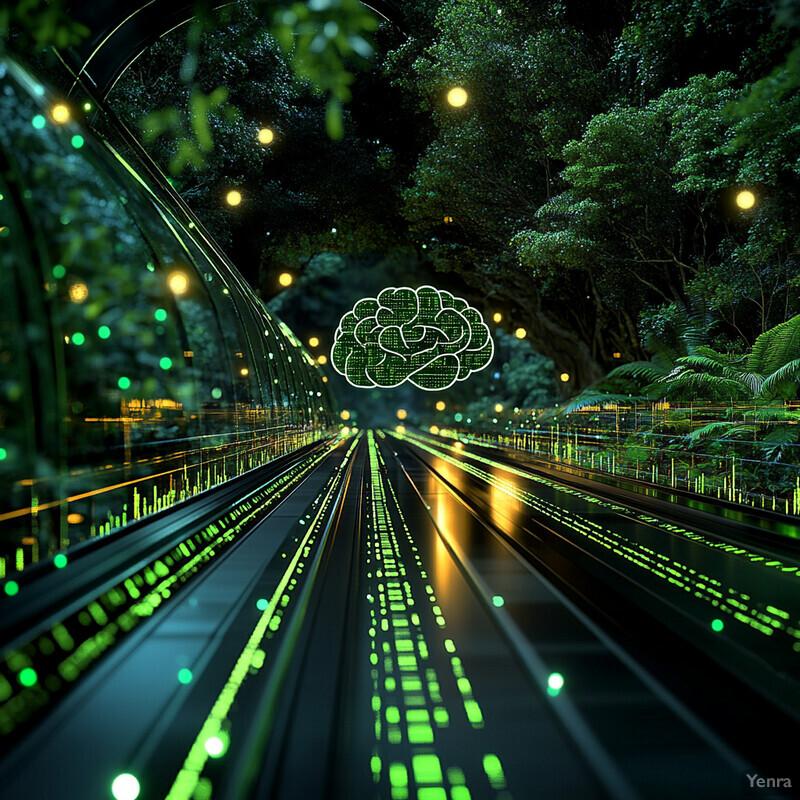
One challenge in bioacoustics is the variability of conditions across different habitats, recording equipment, and species communities. Transfer learning techniques allow models trained on one dataset to adapt and perform well on new, less-studied systems with limited additional data. This flexibility dramatically accelerates scaling up bioacoustic monitoring initiatives across regions, taxa, and conditions. Instead of starting from scratch each time, researchers leverage pre-trained models, reducing the amount of labeled data, time, and expertise needed to launch effective acoustic monitoring campaigns in new domains.
17. Integration with Other Modalities
By combining acoustic data with environmental parameters or camera traps, AI provides a holistic view of ecosystems.
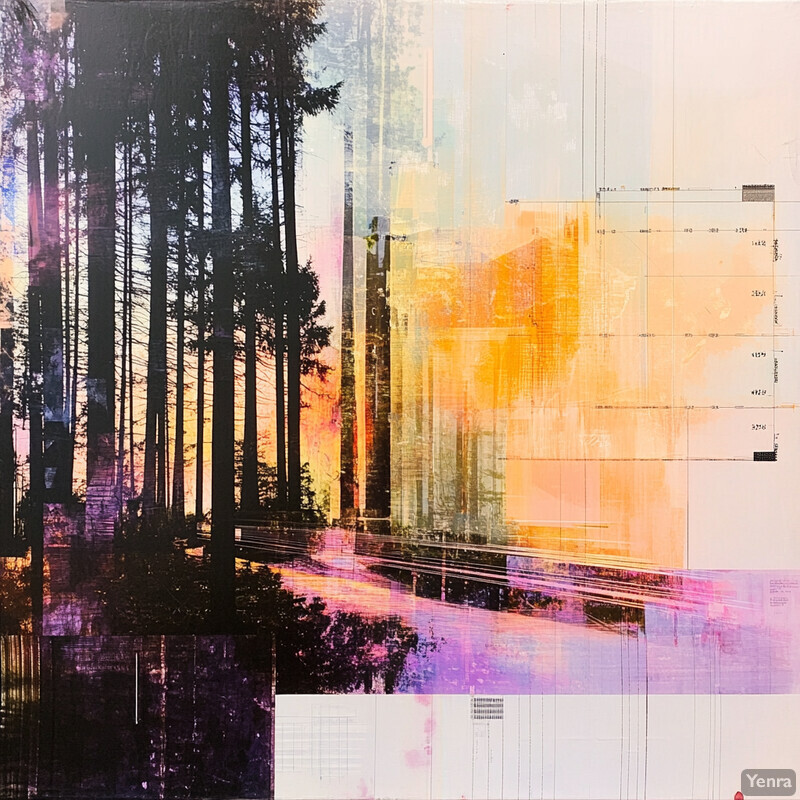
Acoustic data alone provides valuable insights, but it can become even more informative when integrated with other data streams like satellite imagery, camera trap photos, weather data, and habitat surveys. AI models can correlate acoustic signals with environmental conditions, species presence in camera traps, or vegetation indices, forming a multidimensional understanding of ecosystems. Such integrated analyses foster holistic ecological assessments, help pinpoint the interplay between acoustic patterns and habitat characteristics, and facilitate more robust, data-driven conservation strategies.
18. Crowdsourcing and Citizen Science Support
AI-based audio annotation tools can guide citizen scientists to correctly identify calls, improving data reliability and public engagement.
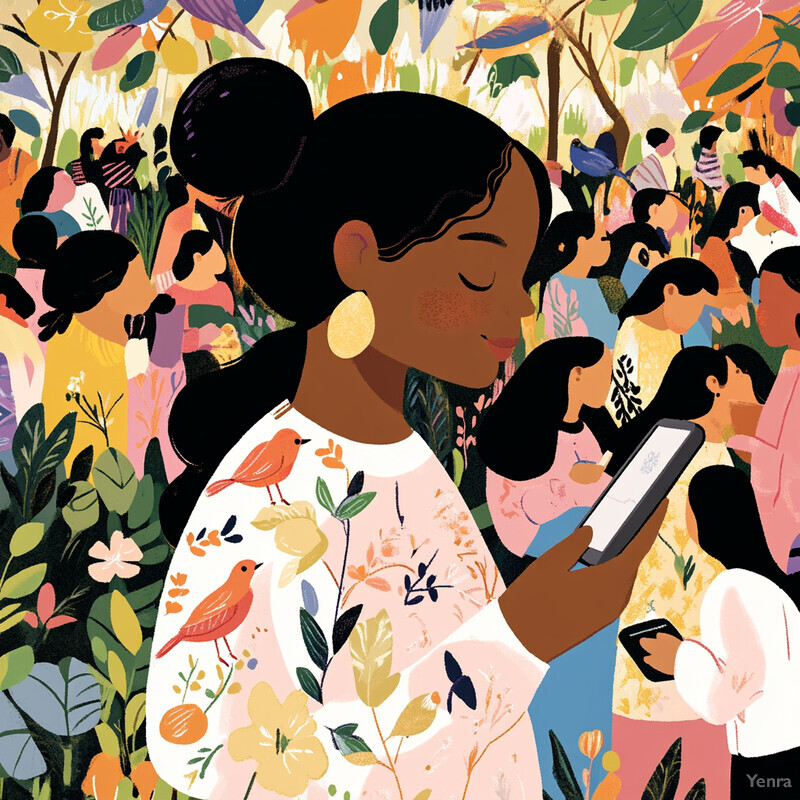
Engaging the public in biodiversity research is a powerful tool for large-scale data collection and awareness. However, citizen scientists can find acoustic data difficult to interpret. AI-supported platforms can guide volunteers by providing suggestions, highlighting likely species matches, or confirming expert identifications. This mentorship ensures more accurate annotations, improves data reliability, and maintains participant motivation. By democratizing the identification process, AI broadens the scope and impact of citizen science initiatives, enhancing scientific data quality and public engagement in conservation efforts.
19. Resource Management and Policy Guidance
AI-driven insights from bioacoustics can inform environmental regulations, protected area management, and targeted conservation measures.
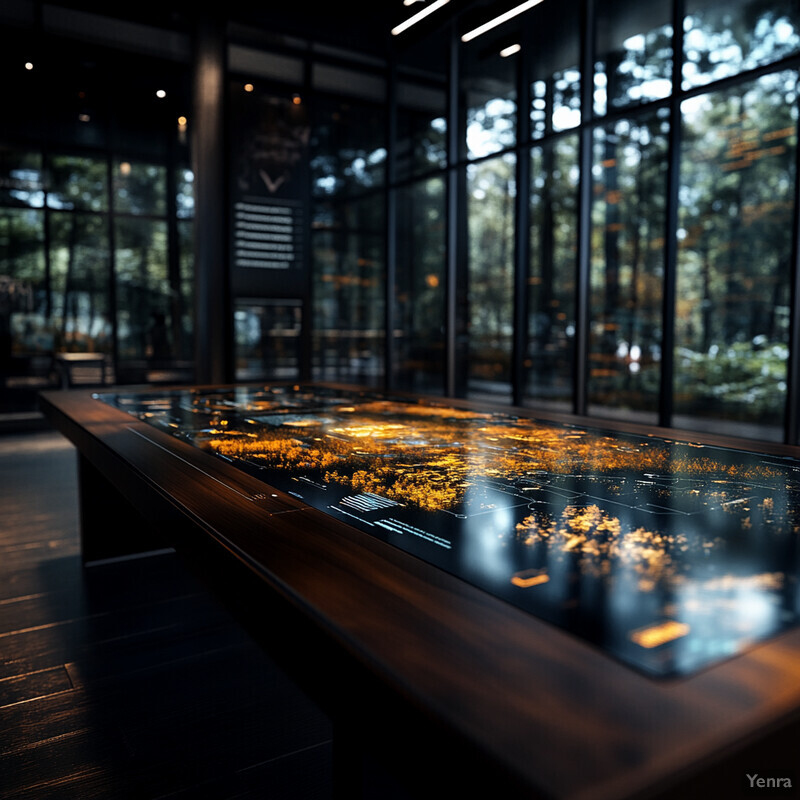
Policymakers and land managers require evidence-based recommendations to ensure sustainable ecosystem stewardship. AI-driven analyses of acoustic data can pinpoint critical habitats, identify keystone species, and detect early signs of ecological imbalance. The insights gained inform management decisions such as where to establish protected areas, how to mitigate noise pollution, or when to enforce seasonal activity restrictions. By turning raw acoustic data into actionable conservation intelligence, AI guides resource allocation, regulatory frameworks, and long-term environmental policies that better reflect ecological realities.
20. Reduced Human Bias and Labor
By automating tedious classification tasks, AI allows researchers to focus on higher-level ecological questions and reduces observer bias.
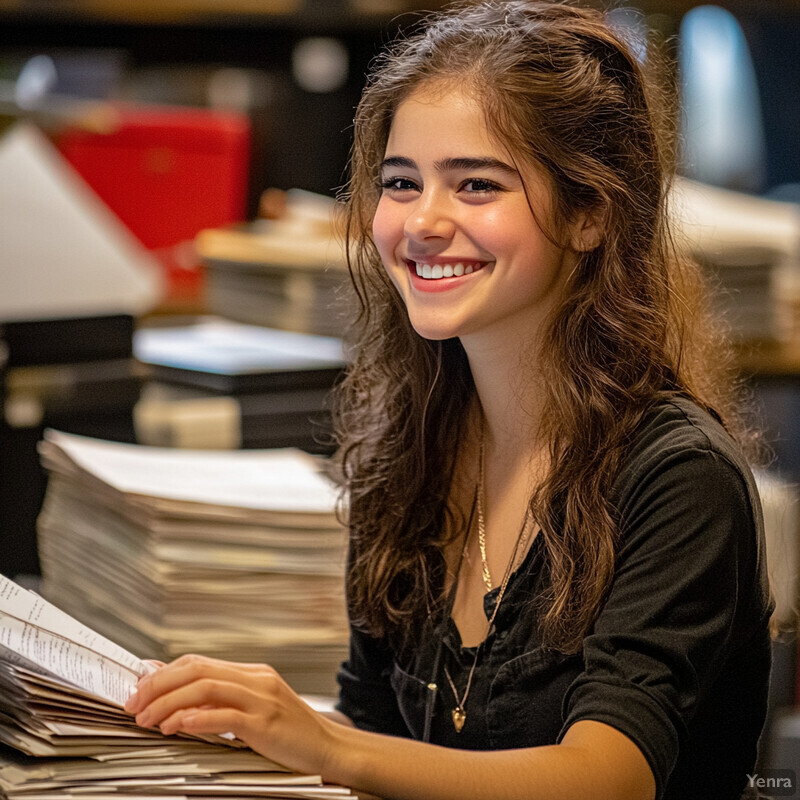
Manual interpretation of acoustic data is time-consuming, error-prone, and subject to human biases and fatigue. AI-powered automation relieves much of this burden, allowing researchers to focus on interpreting results and developing hypotheses rather than performing endless routine tasks. This shift enhances productivity, consistency, and scientific rigor. With repetitive classification and filtering offloaded to AI, ecologists and conservationists can channel their efforts into creative problem-solving, data-driven decision-making, and advancing the broader field of bioacoustics.