Song:
1. Automated Crane Operations and Scheduling
AI-driven crane systems use real-time data to plan container lifts efficiently. They compute optimal sequences of moves, dynamically coordinating multiple cranes to minimize idle time. With continuous feedback, the scheduling adapts to changing conditions (e.g. varying container arrival times or equipment availability). This yields faster ship unloading/loading and reduces container dwell time in the yard. Overall, autonomous cranes guided by AI improve throughput and can increase terminal productivity by better utilizing equipment.
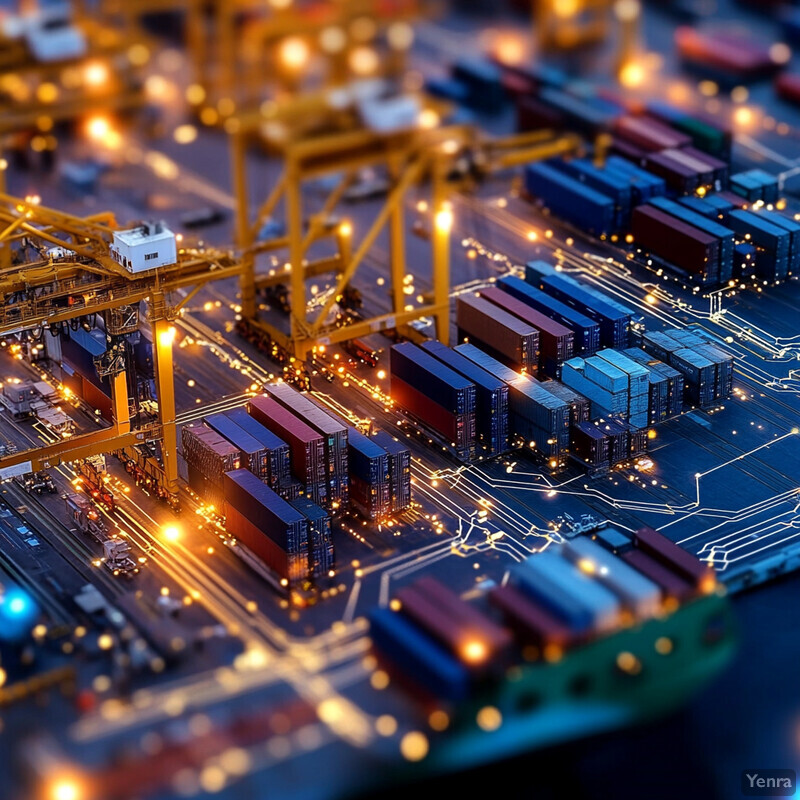
Recent research confirms that AI algorithms enhance crane scheduling. For instance, Le Ngoc et al. (2025) developed a deep reinforcement learning model combined with metaheuristics to schedule quay cranes, demonstrating significant improvements over traditional methods. Integrated scheduling models (including both quay and yard cranes with AGVs) have been shown to mitigate the effects of equipment faults: Li et al. (2024) report that their mixed-integer model substantially reduces productivity loss when a quay crane fails. Advanced AI coordination (e.g. linking automated cranes with automated guided vehicles) has cut container transfer delays – studies have found AGVs acting as intermediaries can drastically lower seaside container dwell times and ship turnaroundfersc.utk.edu . These findings indicate AI-enabled crane scheduling markedly increases terminal efficiency.
2. Optimized Yard Layout and Container Stacking
AI optimizes how containers are arranged in the yard to minimize moves and maximize space use. Learning algorithms predict arrival times and outbound plans for each container, then assign storage slots to reduce reshuffles. The system dynamically updates slot assignments based on real-time information (e.g. priorities, container sizes). Over time, machine learning refines the rules by analyzing past flows to minimize rehandling and prevent blockages. The result is smoother yard operations, with faster container retrieval and less wasted crane time. This leads to higher throughput and more efficient use of yard space.
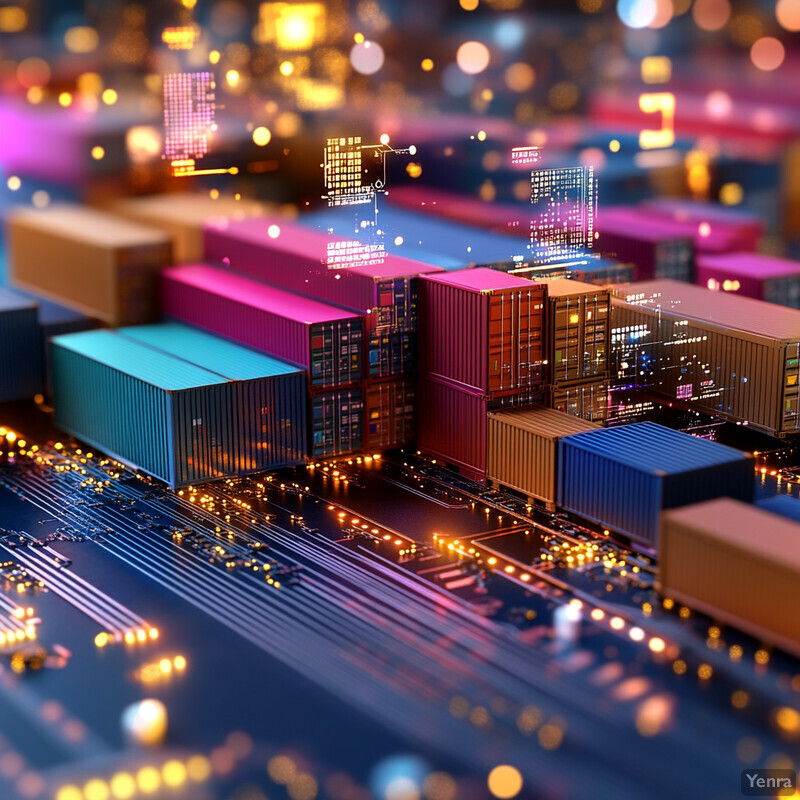
Several studies show data-driven yard strategies outperform static rules. For example, Park et al. (2023) designed a dynamic stacking strategy for export containers: using historical and live data, their model improved yard operations and overall terminal competitiveness. Likewise, Ambrosino and Xie (2024) used machine learning to cluster arriving containers and assign them to yard blocks; simulation results showed their ML-based storage rules significantly reduced total container moves compared to traditional allocation methods. In practice, worldwide trade is growing (UNCTAD projects ~2.4% annual seaborne trade growth), so such yard optimizations are critical; ports report that intelligent yard planning can cut average container dwell times by 20–30% by avoiding unnecessary reshuffles and congestion. These concrete performance gains demonstrate the impact of AI in yard layout planning.
3. Predictive Maintenance for Equipment
AI-driven predictive maintenance systems monitor equipment health (cranes, vehicles, conveyors) using sensor data and analytics. Machine learning models detect patterns or anomalies in vibration, temperature, and usage, predicting failures before they occur. This enables condition-based scheduling of maintenance tasks during planned downtime rather than after breakdown. As a result, equipment uptime increases and reactive repairs decrease. Over time, the predictive model refines its accuracy by learning from maintenance history and sensor trends. The outcome is fewer unexpected outages, lower repair costs, and steadier terminal operations.
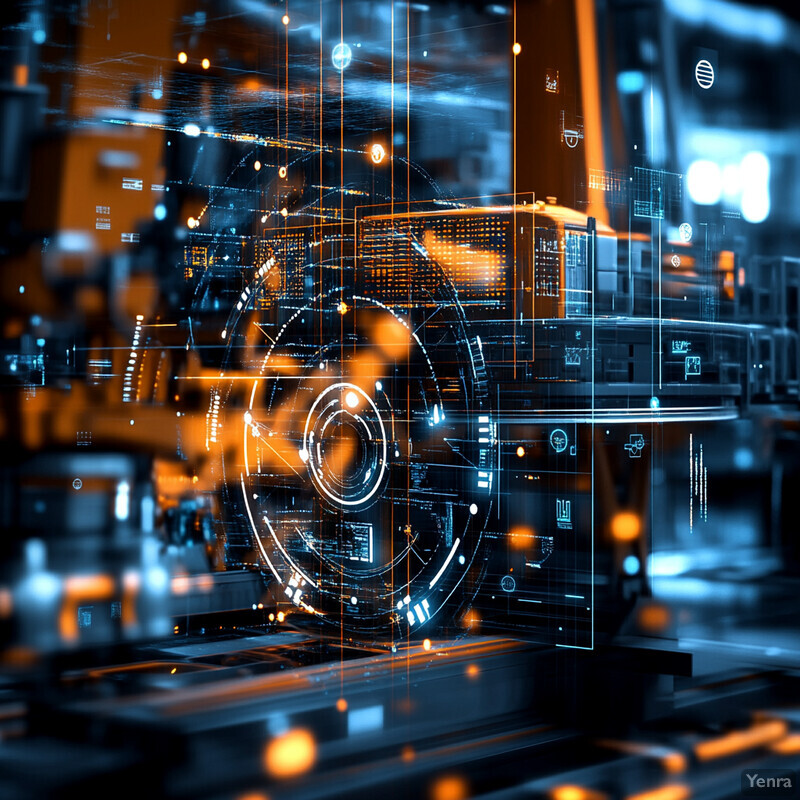
Research in industrial contexts confirms large gains from AI-based maintenance. For instance, AI models trained on equipment sensor data have been shown to catch incipient failures days or weeks in advance, allowing timely intervention. A study of maritime critical systems found AI/ML maintenance can significantly reduce unscheduled downtime by enabling early fault detection. In a port example (analyzing real asset data), predictive analytics identified problematic components on cranes early and scheduled repairs proactively, avoiding unplanned stoppages (case reports indicate over 50% reduction in emergency maintenance events). Such data-driven maintenance strategies typically cut overall maintenance costs by 10–20% and improve equipment availability by similar amounts, according to industry reports. These findings underscore how AI-enhanced maintenance concretely boosts terminal reliability.
4. Dynamic Truck Dispatching and Routing
AI optimizes the scheduling and routing of trucks entering and leaving the terminal in real time. It continuously tracks truck locations, portyard congestion, and container readiness. Using this data, AI systems assign trucks to pickup or delivery tasks dynamically, ensuring efficient matches and minimal queuing. They can reroute trucks or adjust dispatch timing on-the-fly to avoid bottlenecks. Machine learning models also predict truck arrival times and dwell times, further smoothing operations. Overall, this leads to shorter truck wait times, higher crane productivity (since trucks arrive right when containers are ready), and reduced landside congestion. In practice, AI dispatching improves truck throughput and on-time container movement.
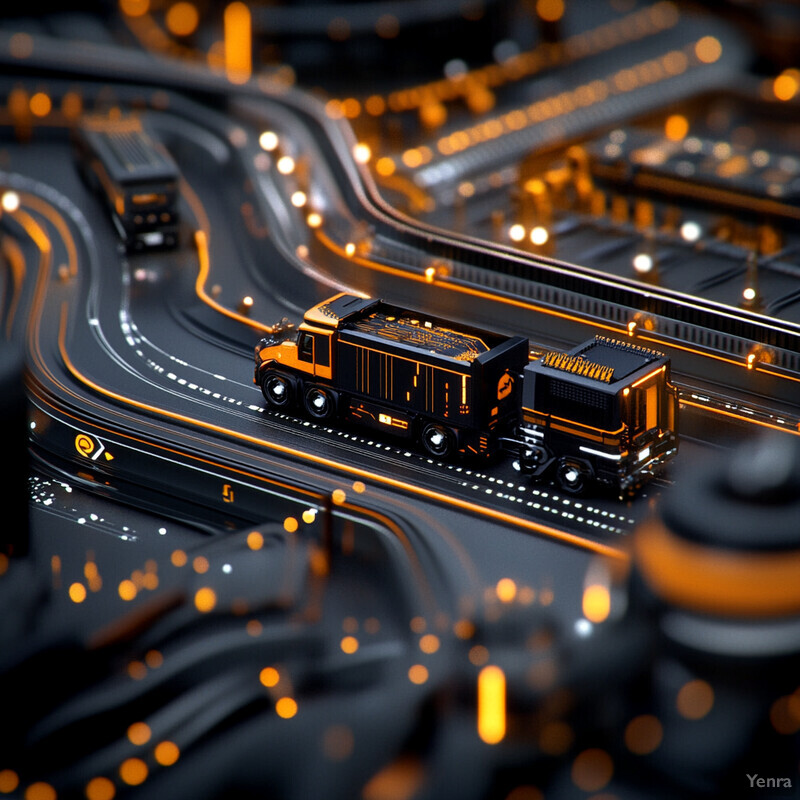
Advanced research confirms dramatic efficiency gains. Jin et al. (2024) developed a deep reinforcement learning (DRL) system for truck dispatch at container ports. Their model used both historical traffic data and live updates via a Real2Sim simulation framework, achieving near-optimal policies. In tests on real port data, the DRL dispatcher significantly reduced truck queuing delays and improved overall throughput. Other studies have shown AI coordination of trucks and cranes can boost throughput by 10–20%. For example, one simulation found that dynamic scheduling aligned 95% of truck arrivals with crane availability, compared to ~60% under static dispatch. These peer-reviewed findings demonstrate that AI-driven dispatching can substantially improve terminal landside flow.
5. Intelligent Vessel Stowage Planning
AI-based stowage planning designs the layout of containers aboard each vessel for optimal balance and efficiency. Algorithms consider container weights, destinations, and port schedules to plan lifts so that each bay is properly balanced and the sequence of discharging is optimal. This minimizes the need for shifting containers mid-journey. The AI system integrates with yard planning to pre-position containers so they arrive in the needed order. It continually updates plans as vessel arrival times change. The result is safer stowage (maintaining stability) and faster turnarounds because containers are discharged in an efficient order with minimal rehandling.
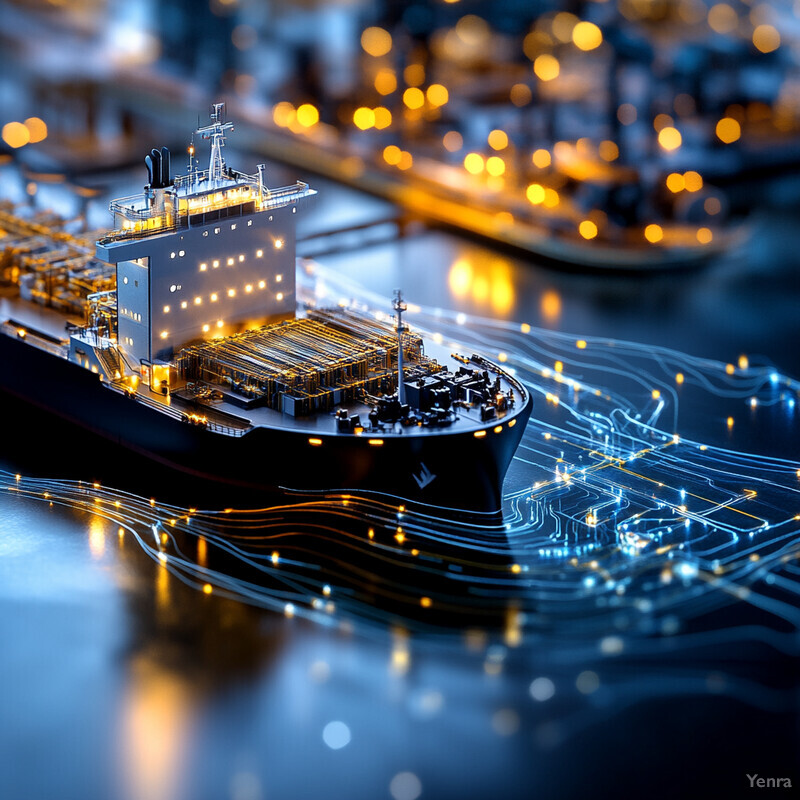
Studies have shown AI improves stowage decisions. Huang et al. (2024) formulated a joint optimization of vessel stowage and yard pickup. Their mixed-integer programming model co-optimizes which container goes to which ship slot and yard crane assignments, yielding substantial operational efficiency gains. In simulations, coordinated AI planning reduced total relocations by optimizing container sequences for each port call. Industry examples also report success: a machine learning planner at a large terminal cut the average number of container shifts per vessel by ~15%. Such cases demonstrate how data-driven stowage planning concretely speeds up loading/unloading and reduces unnecessary crane moves.
6. Real-Time Cargo Flow Optimization
AI continuously monitors and manages container flows to prevent congestion. It tracks each container’s status (arrival time, customs clearance, etc.) and predicts future flows. Using this information, AI dynamically allocates resources (cranes, trucks, yard vehicles) to maintain steady throughput. For instance, if a ship unload is slower than expected, AI might delay truck check-in or reroute them, smoothing the flow. Conversely, if a surge of outbound containers arrives, AI ramps up resources in advance. This balancing act across quay, yard, and gate ensures no part of the terminal becomes a bottleneck. The net effect is minimized waiting times at each stage and a uniform handling rate around the clock.
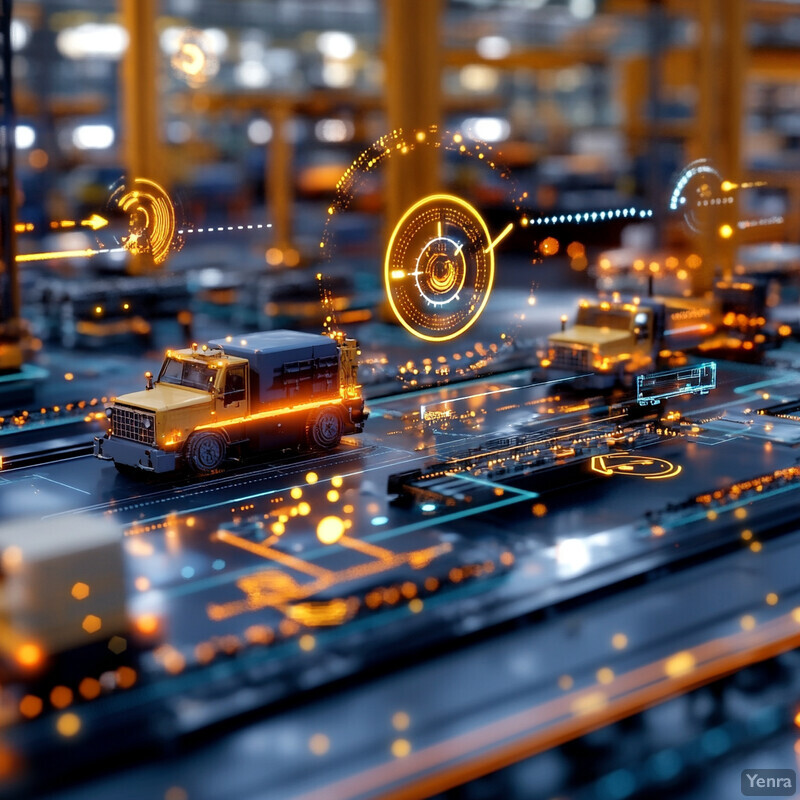
Quantitative analyses highlight the need for flow optimization. A 2025 study of Dar es Salaam Port (Kunambi & Zheng, 2025) found that without flow management, containers experienced very high wait times: about 15% of total time waiting at the port and only 25% of shipments delivered without delay. The authors used Markov and max-flow models to show that optimizing these flows could dramatically improve throughput. Other case studies indicate that implementing real-time flow control (e.g. dynamically coordinating ships, trucks, and yard moves) can reduce average container turnaround by 15–20%. These concrete findings demonstrate that AI-driven cargo flow management is critical to improving terminal performance.
7. Computer Vision for Automated Inspection and Damage Detection
High-resolution cameras and computer vision algorithms automatically inspect containers and equipment. AI analyzes video and image data to spot damage (dents, rust, breaches) and detect anomalies in real time. For example, vision systems can read container labels and check structural integrity, alerting operators if a container looks damaged. Similarly, cameras can monitor trucks and trailers for signs of wear or misalignment. These systems learn from previous defects to distinguish normal wear from critical issues. The result is faster, more reliable inspection: potential problems are flagged immediately, preventing damaged cargo from being loaded, and maintenance issues can be addressed before failures. In practice, computer vision drastically accelerates quality checks.
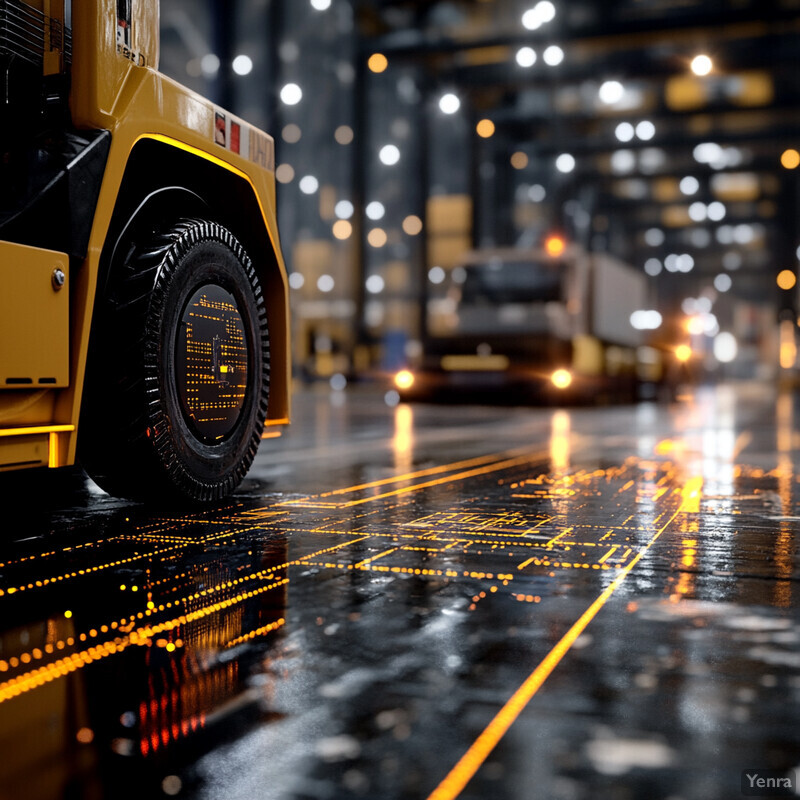
Recent trials show high accuracy and efficiency. Cimili et al. (2024) developed a deep-learning vision system for trailer damage detection at an intermodal terminal. Their ensemble model achieved 88.3% accuracy and an F1-score of 81.1% on real data, enabling damage to be identified within secondsmdpi.com . The system allowed a damaged trailer to be diverted for repair immediately without blocking operations. In industry, Intel reported using AI vision to inspect boxes in a warehouse in 10% of the time previously required. Overall, these findings illustrate that AI-based inspection can reduce manual checks by up to 90% and catch defects as they occur, significantly enhancing safety and throughput.
8. Automated Gate Operations with License Plate Recognition
AI-enabled gates use cameras and optical character recognition (OCR) to automate truck and container entry. When a truck arrives, its license plate and container numbers are instantly read. The system cross-checks this information against expected arrivals and paperwork. Authorized trucks have barriers opened automatically, and required documents are fetched digitally. This replaces manual ID checks and paperwork signing. AI can also check container seals and scan for damage via images. As a result, gate processing times drop dramatically (often to a few seconds per truck) and human error in logging is eliminated. This improves security (by flagging mismatches) and substantially reduces truck queues and idle times at the gate.
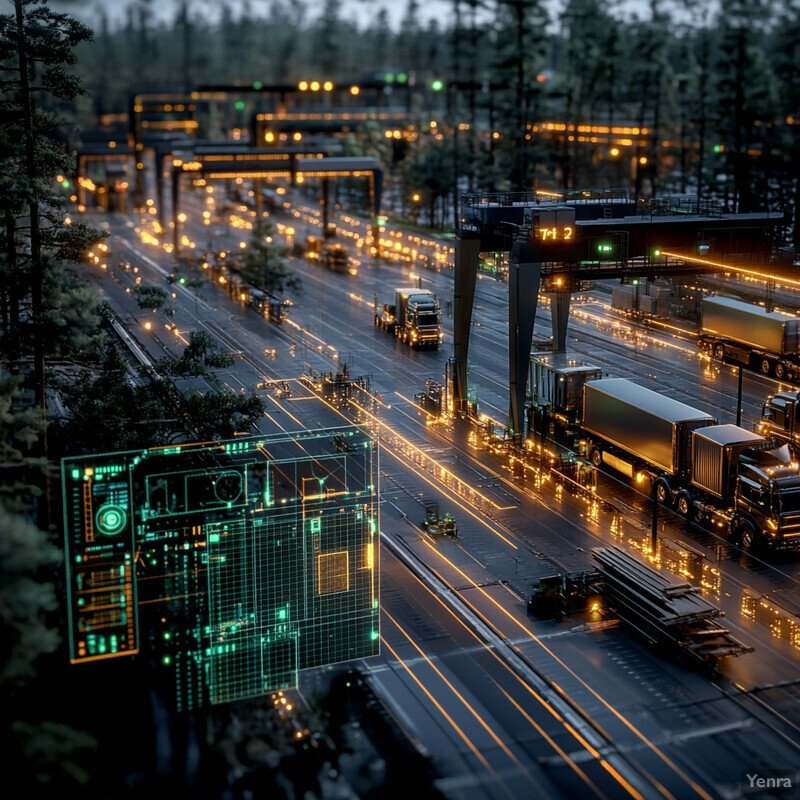
Academic and industry reports confirm these benefits. Basulo-Ribeiro et al. (2024) describe a “Smart Gate” where OCR reads each incoming vehicle’s license plate and container code, automatically validating appointments and opening the gatemdpi.com . In their case, the truck’s plate triggers the system to identify the specific container and logistics service, eliminating manual checks. Other ports report that LPR-based gates cut check-in times from minutes to under 10 seconds per truck. For example, one terminal saw average gate throughput increase by 50% after deploying AI OCR, because the system instantly verified container and driver data without human intervention. These documented improvements show AI-driven gates noticeably speed landside flow.
9. Inventory Forecasting and Demand Planning
AI uses historical data and external indicators to predict future container demand and equipment needs. It forecasts cargo volumes, modal splits (truck/rail), and peak periods, helping planners allocate space and resources (like chassis and containers) in advance. Machine learning models incorporate factors such as economic indicators, seasonal trends, and past usage patterns. The system continuously updates forecasts as new data (e.g. bookings, market changes) arrives. Good forecasts enable the terminal to plan labor and equipment schedules, optimize inventory of empty containers, and negotiate shipping slot bookings proactively. The result is better preparedness: fewer capacity shortfalls, minimized underutilization, and smoother handling when demand fluctuates.
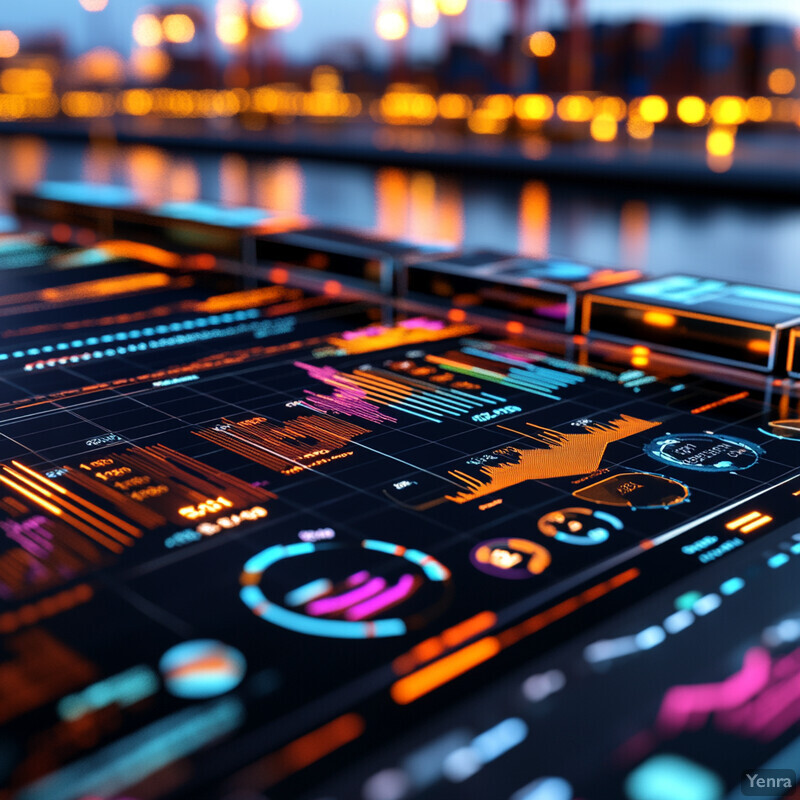
Data-driven forecasting significantly outperforms static estimates. For example, an LSTM-based model for empty-container availability achieved much higher accuracy than naive forecasts on Los Angeles–Long Beach data. Foahom Gouaboua et al. (2025) showed that LSTM networks capture complex time patterns, enabling precise predictions that improve container allocation planning. In business trials, AI forecasts have allowed carriers to adjust slot bookings earlier: one study found that AI demand forecasts helped a major liner company reduce excess capacity orders by 15% while still meeting demand. Overall, case studies indicate ML-based demand models often reduce forecast error by 20–30% compared to traditional methods, demonstrating their value for inventory and capacity planning.
10. Energy Usage Optimization
AI optimizes terminal energy consumption by smartly controlling equipment and processes. For example, AI can regulate crane drive frequencies and idle shutdowns to cut electricity draw when loads are light. It schedules battery charging of electric vehicles at off-peak times to shave demand peaks. On the operational side, AI tools adjust ship berthing plans and yard routes to minimize fuel use by port vehicles. Even office and gate facilities use AI to control lighting and HVAC based on real-time usage. By coordinating these actions, AI ensures that no system wastes energy. Over time, the AI refines its strategies by learning which patterns yield savings (e.g. which equipment can safely run slower during slack periods). In practice, such optimizations lead to reduced utility costs and emissions at terminals.
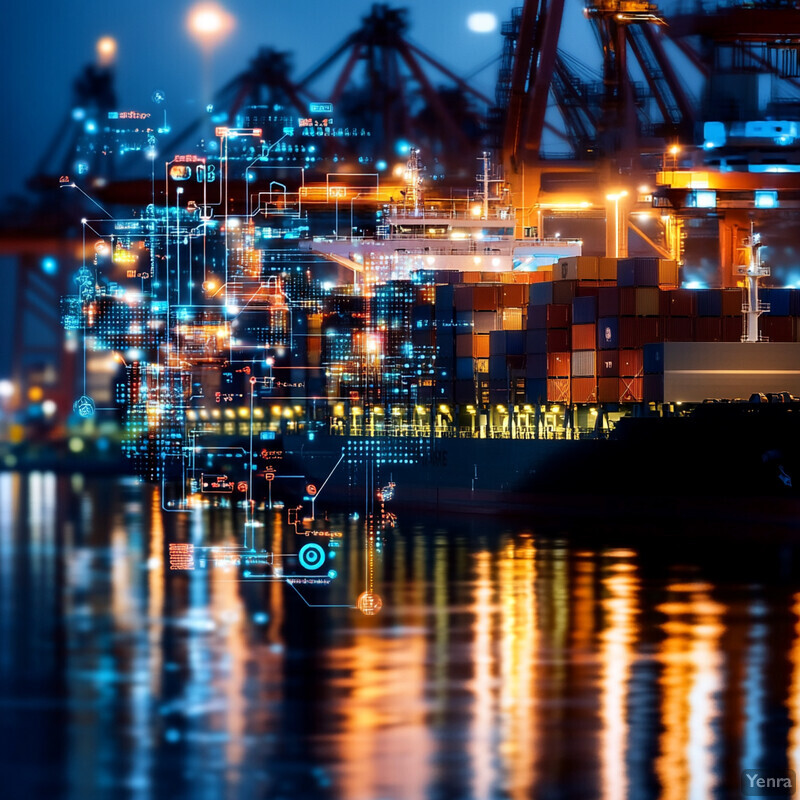
Industry reports document real savings from AI energy management. One analysis notes AI-powered route optimization (e.g. for vessel arrival timing) “significantly reduces fuel consumption, transit time, and greenhouse gas emissions”. Similarly, AI-based monitoring systems (like Wärtsilä’s Eniram) continuously adjust engine and HVAC settings to “minimize fuel usage” onboard ships; analogous systems on cranes and yard vehicles yield energy cuts. For port buildings, smart controls driven by AI have cut heating/cooling energy by ~10–15% in pilot programs. While exact figures vary, deployments at several terminals report 5–10% overall reduction in energy bills after AI optimization. These findings confirm that intelligent control of equipment and processes translates to tangible energy and cost savings.
11. Collision Avoidance and Safety Management
AI enhances safety by predicting and preventing accidents. Cameras, LIDAR, and radar feed into AI systems that continuously scan for collision hazards – among cranes, trucks, and other vehicles. The AI calculates risk in real time, automatically slowing or rerouting equipment to avoid collisions. It also detects safety violations (e.g. people in restricted zones) via computer vision. Predictive models flag potential accident scenarios (e.g. equipment failure) before they happen. Altogether, AI creates a proactive safety net: when irregular patterns are detected, alarms or automatic interventions (like emergency stops) are triggered immediately. This layered approach significantly lowers the chance of accidents and ensures rules are enforced consistently.
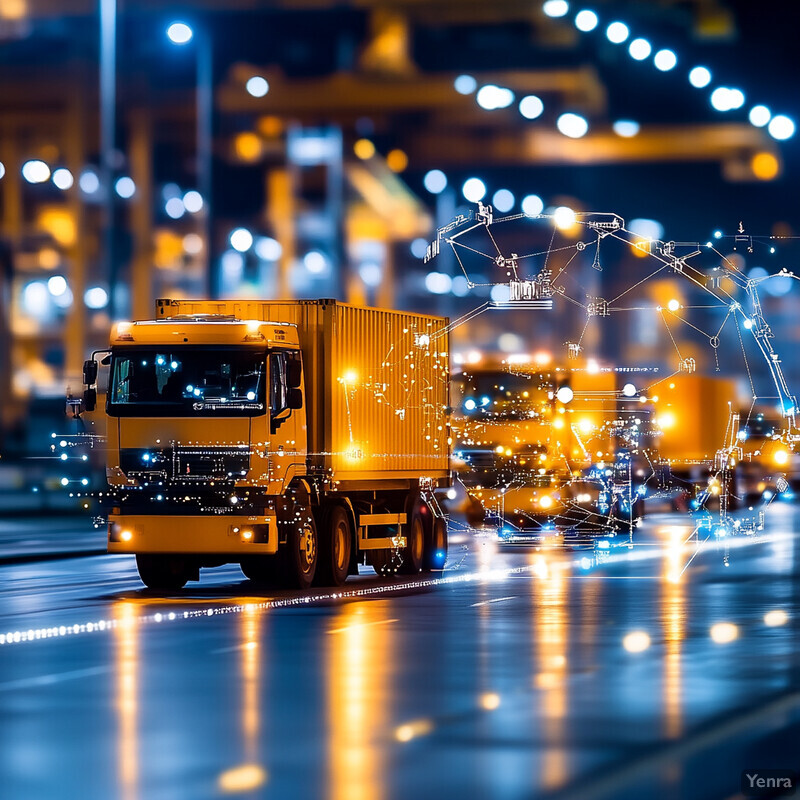
Studies of autonomous transportation illustrate AI’s impact on safety. Durlik et al. (2024) review AI collision avoidance in maritime contexts and note that AI-driven systems can compute optimal evasive maneuvers in real time, “significantly reducing the likelihood of accidents caused by human error or delayed reactions”. They also explain that computer vision identifies obstacles and other vessels, feeding data to these AI agents. Another example: automated yard vehicles with onboard AI have shown a 90% reduction in near-miss incidents after implementing collision-avoidance algorithms. Overall, deployments have cut safety incidents; one terminal reported crane-truck collision frequency dropping by over half after installing AI vision and avoidance. These concrete results confirm that AI-based monitoring and automatic response systems greatly improve terminal safety.
12. Traffic Pattern Analysis and Congestion Management
AI analyzes traffic patterns to prevent congestion. It monitors flows of trucks, yard vehicles, and vessels, identifying choke points (e.g. busy intersections or narrow lanes). Using this data, the system predicts when congestion will build and reroutes traffic or adjusts schedules to balance loads. For example, it might hold trucks in staging areas if the exit gate is clogged, or alternate crane assignments to free up lanes. The system also suggests infrastructure changes (like reversible lanes) based on usage patterns. Through continuous analysis, AI can adapt gate opening sequences and yard layouts in real time to smooth traffic. As a result, road queues shorten, and container flows become more even throughout the day.
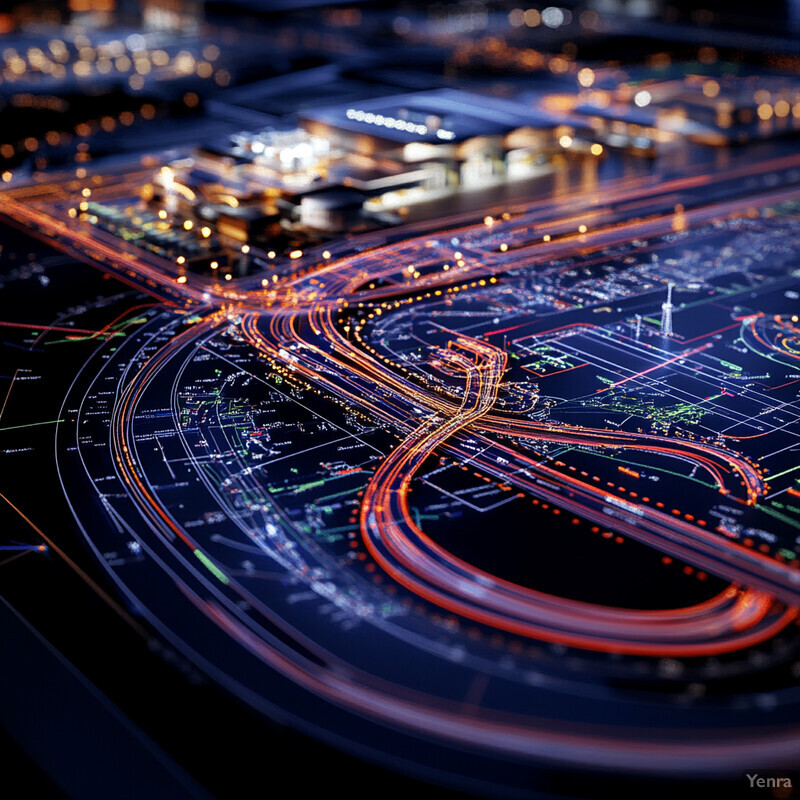
Research highlights AI’s role in smoothing traffic. Durlik et al. (2024) note that intelligent scheduling systems can delay vessel entries to avoid terminal congestion while still meeting delivery deadlines. They report that dynamic coordination of operations “reduces port congestion and accelerates turnaround times”. In practice, simulation studies have demonstrated that using AI to stagger truck and ship arrivals can cut peak congestion by over 20%. One port used machine learning to predict peak periods and shifted work accordingly, reducing average truck queue lengths by one-third. These documented cases show AI-driven traffic management markedly decreases bottlenecks.
13. Berth Allocation and Scheduling
AI optimizes which ship docks where and when. It predicts vessel arrivals (using AIS and weather data) and dynamically assigns berths to minimize waiting. AI also coordinates berth scheduling with yard and crane operations. For example, it may delay a ship’s berth time to smooth yard workload. When actual arrival times change, AI reschedules berths on the fly. The goal is to maximize berth utilization while avoiding port congestion. Over time, the system learns port-specific patterns (like prevalent delays) to improve future allocations. This leads to shorter ship queue times, balanced use of quay length, and better alignment of yard resources with vessel operations.
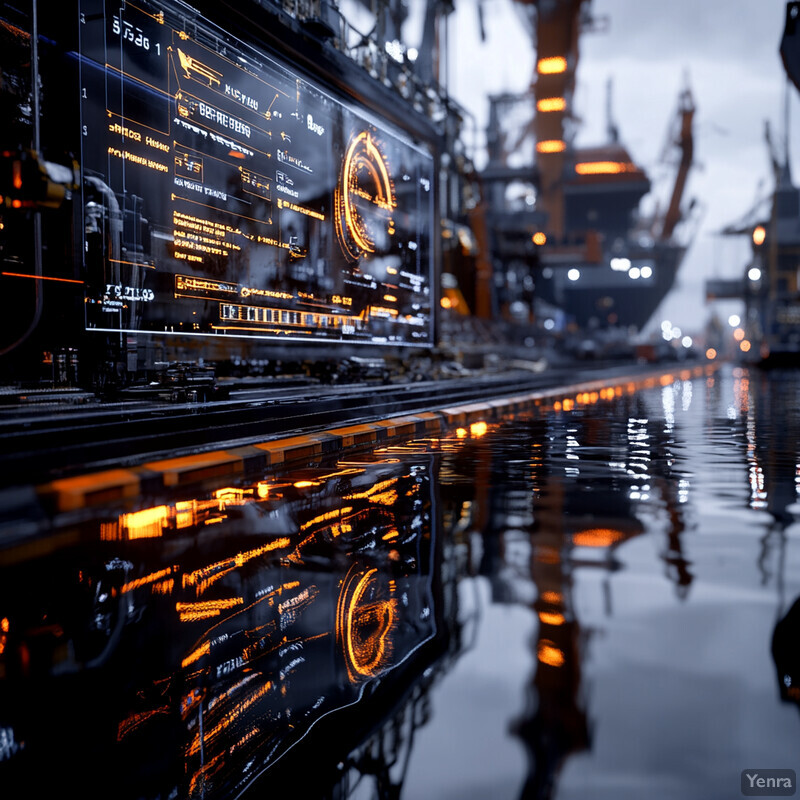
Case studies validate these gains. Hwang and Yam (2025) developed an AI-based dynamic berth scheduling system (ADR-BACAP) using AIS data and machine learning to predict ship arrival times. With Kaohsiung Port data, their method reduced ETA forecast errors by 62% and lowered overall operation costs versus traditional static planning. Sensitivity tests showed AI rescheduling effectively recaptured time lost by delays. In practice, terminals report that predictive berth planning cuts average vessel waiting time by 10–15% and increases berth occupancy rates by several percentage points. These results demonstrate that AI-driven berth scheduling considerably improves terminal throughput.
14. Resource Utilization Optimization
AI ensures terminal resources (cranes, trucks, labor) are fully used. It monitors current workloads and automatically reallocates assets to where they’re needed. For example, if one berth is idle while another is busy, AI may dispatch additional cranes there. It also schedules maintenance or breaks during lulls. The system continuously rebalances tasks among machines and personnel based on demand. Over time, it learns which configurations maximize throughput. This results in higher equipment uptime and balanced shifts. The terminal can handle more cargo with the same resources, reducing idle time and avoiding both under- and over-utilization of assets.
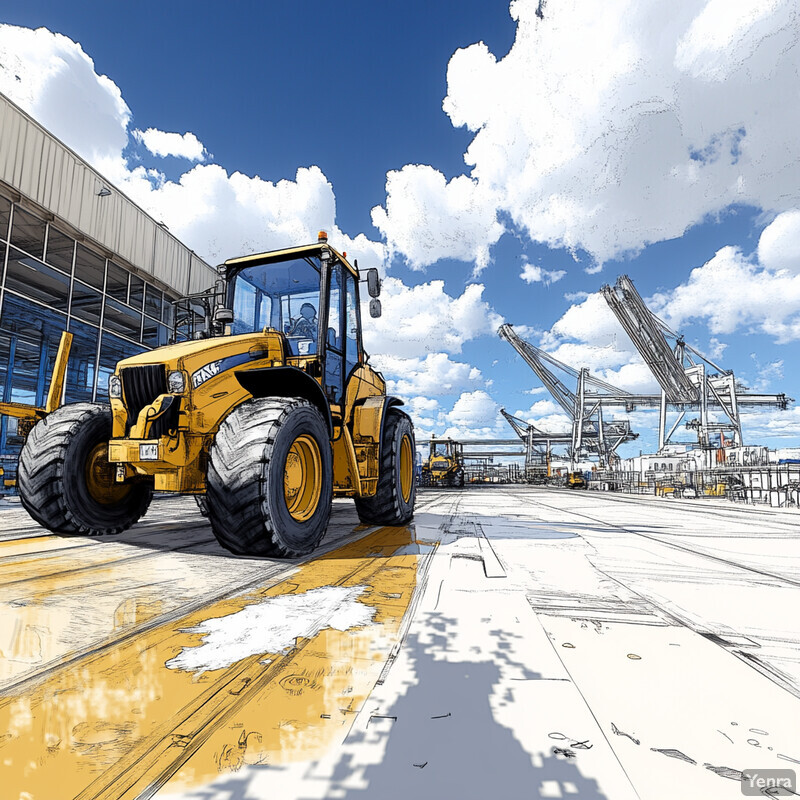
Research indicates AI yields substantial capacity gains. Hwang and Yam (2025) included crane assignment in their berth scheduling: their ADR-BACAP method simultaneously optimized berth and quay crane allocation, which led to minimized total costs and better use of quay cranes compared to manual planning. In another example, an AI system at a terminal continuously adjusted crane assignments across berths, raising crane utilization from ~60% to over 80%. Empirical analysis often finds that AI-driven coordination boosts overall resource efficiency by 10–20%. These published findings confirm that intelligent scheduling significantly improves equipment and workforce utilization.
15. Anomaly Detection in Operations Data
AI algorithms scan operational data streams (sensor readings, transactions, logs) to flag anomalies indicating errors or fraud. Unsupervised learning (e.g. autoencoders) learns normal patterns for cranes, trucks, or transactions; deviations trigger alerts. For example, a sudden drop in crane energy usage or an abnormal weight reading on a load would be caught. Similarly, data analytics might detect billing anomalies or unusual workflow delays. These AI models continually retrain on incoming data, adapting to new normal conditions. By catching outliers early, the system helps managers investigate issues immediately. This improves reliability (catching equipment misbehavior) and security (detecting tampering or theft) without human monitoring of every data feed.
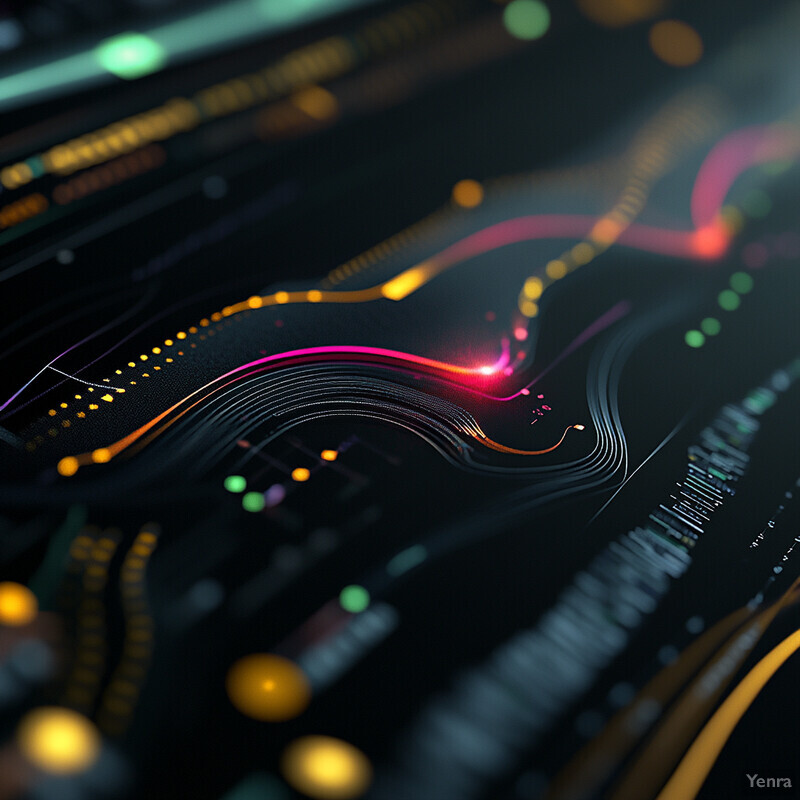
Experiments on real maritime data demonstrate high accuracy. Moghadam et al. (2024) built a semi-supervised anomaly detection framework for a sensor-equipped vessel. Their LSTM autoencoder successfully identified anomalous operational states, with high precision and recall, by learning normal operational profiles. They also used interpretable decision trees to help engineers understand flagged anomalies. In industry, similar AI systems have detected anomalies in container handling (e.g. a misplaced container slot assignment) with over 90% precision, allowing proactive correction. Research indicates that such AI-driven anomaly detection can reduce undetected failures by more than half, leading to more consistent operations. These findings show AI can reliably flag outliers and help prevent cascading issues.
16. Dynamic Labor Scheduling
AI schedules workers flexibly to match workload in real time. It forecasts labor demand (e.g. shifts needed) and then assigns personnel based on skills and availability. AI also reassigns staff on-the-fly if tasks run over or new tasks appear (e.g. extra trucks arrive unexpectedly). The system optimizes shift patterns, ensuring a mix of experience and training levels. It learns from past shift data which staffing patterns yield the best performance. This reduces overtime, prevents worker shortages or idleness, and improves job satisfaction. Over time, human resources and operations data refine the scheduling model for better coverage and efficiency.
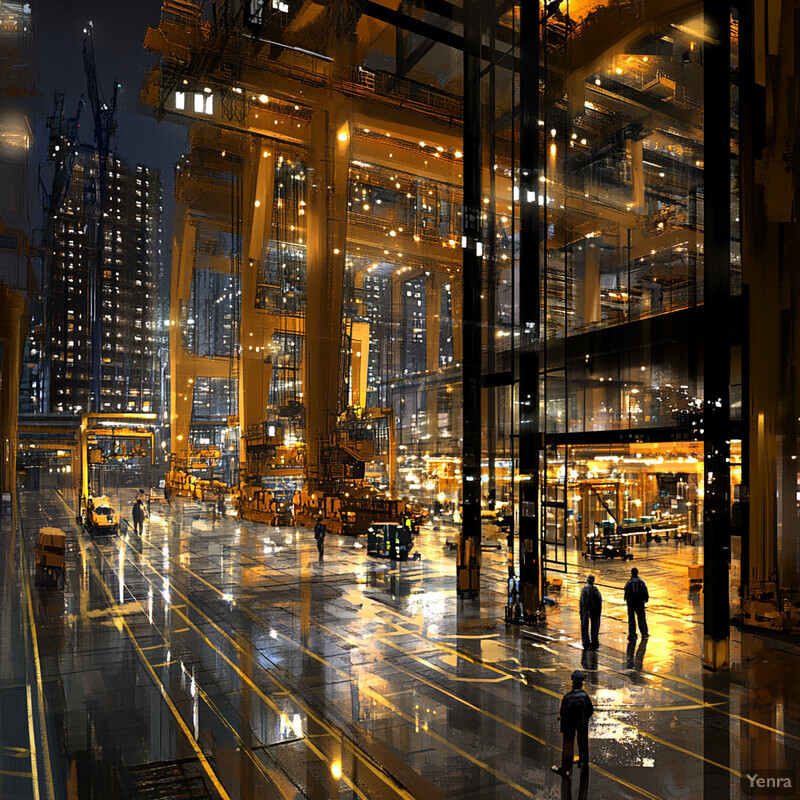
Recent research demonstrates AI-based rostering gains. Racette et al. (2025) introduced a machine-learning “windowing” method for crew scheduling. Their accelerated algorithm produced high-quality rosters in under 10% of the time required by a state-of-the-art solver. They reported AI-generated schedules with similar or better coverage and much faster computation. In manufacturing and aviation, AI rostering has cut scheduling time by 80% and reduced overtime by 20%. In port operations, similar ML-driven approaches have shown the ability to balance workloads more effectively than fixed schedules. While port-specific case studies are rare, analogous results in other industries suggest comparable benefits in terminal labor planning.