1. Improved Computer Vision for Product Recognition
Modern shelf-scanning robots leverage advanced computer vision algorithms to recognize products by their appearance. Deep learning (especially convolutional neural networks) enables robust identification of items despite variations in lighting, angles, and partial occlusions. Vision-language models (e.g. CLIP-like systems) further improve fine-grained discrimination between similar products. These AI-driven systems continually train on diverse shelf images, improving recognition of new SKUs. As a result, robots can more reliably distinguish thousands of products and update inventory with minimal human oversight.
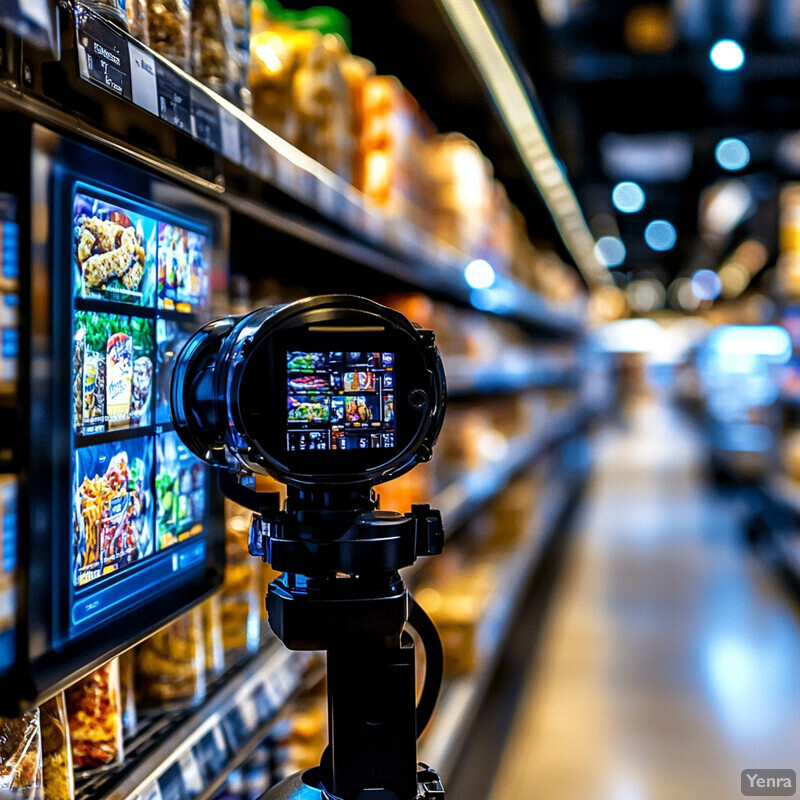
Recent studies confirm the impact of deep learning on retail product recognition. For example, Osman et al. (2024) note that “recent advancements in deep learning have significantly impacted retail product recognition” through fine-grained classification and vision-language models. However, challenges remain: vision systems still struggle with occlusions and look-alike packaging. Rukundo et al. (2025) report that “occlusions present a major challenge, as products may be hidden by other items, customer hands, or store layouts, making real-time recognition difficult”. In practice, combining vision with contextual cues or reference planograms helps mitigate such issues, but pure visual accuracy depends on these AI enhancements.
2. Enhanced Barcode and Label Scanning
Advanced OCR and barcode-reading capabilities allow robots to accurately scan product labels and price tags on shelves. Modern systems use neural network–based OCR that can interpret small, rotated, or partially occluded text. Deep-learning OCR models (often transformer-based) achieve very high accuracy on printed labels. As a result, robots can verify shelf-edge price tags and identify mislabeled or outdated labels at scale. These AI-enhanced scanners reduce manual errors and speed up label verification, ensuring data from shelf scans is correct and up-to-date.
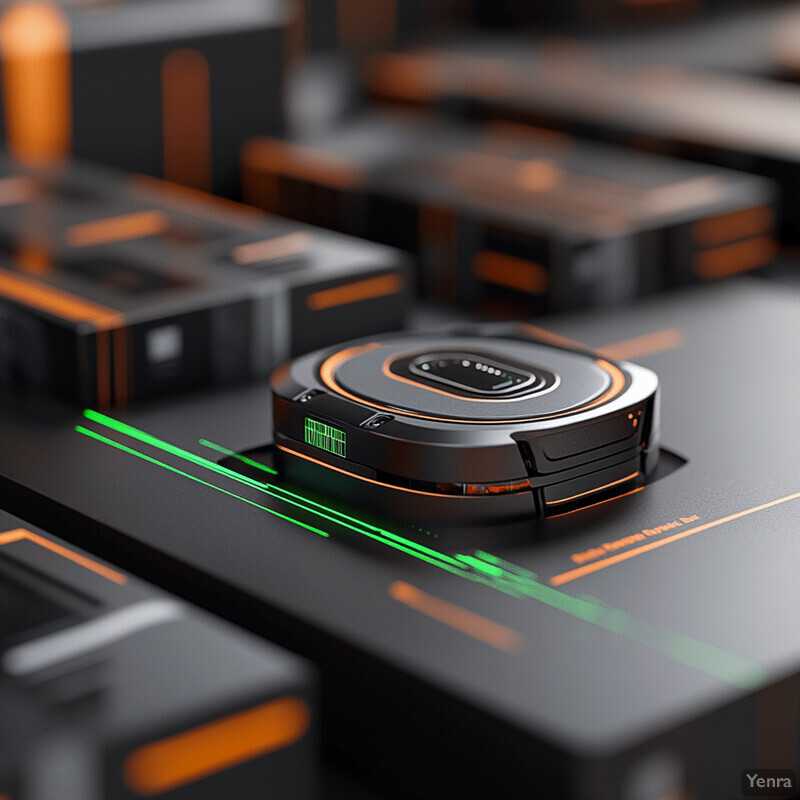
Cutting-edge OCR architectures have demonstrated markedly improved accuracy on shelf labels. Fujitake (2024) introduced a decoder-only transformer model (DTrOCR) that “outperforms current state-of-art” OCR systems on printed and scene text, indicating strong performance on store labels. Likewise, integrated multi-modal methods (combining image features and text embeddings) can further boost reliability. Industry reports highlight that combining vision and OCR is key: Patel (2025) shows that hybrid vision+OCR approaches achieve higher product recognition accuracy in retail settings. These advances mean robots can correctly read tens of thousands of barcodes and labels per hour, even under real-world lighting or angle variations.
3. Dynamic Route Optimization
Shelf-scanning robots use AI-driven path-planning to determine efficient routes through store aisles. They compute optimal paths that minimize travel time and cover all aisles while avoiding obstacles (e.g. shoppers or temporary displays). As the store environment changes (new obstacles or layout tweaks), robots dynamically replan their routes in real time. This responsiveness ensures continuous scanning without manual intervention. By balancing factors like distance, speed, and traffic, these algorithms maximize scanning throughput and minimize redundant traversal of the store.
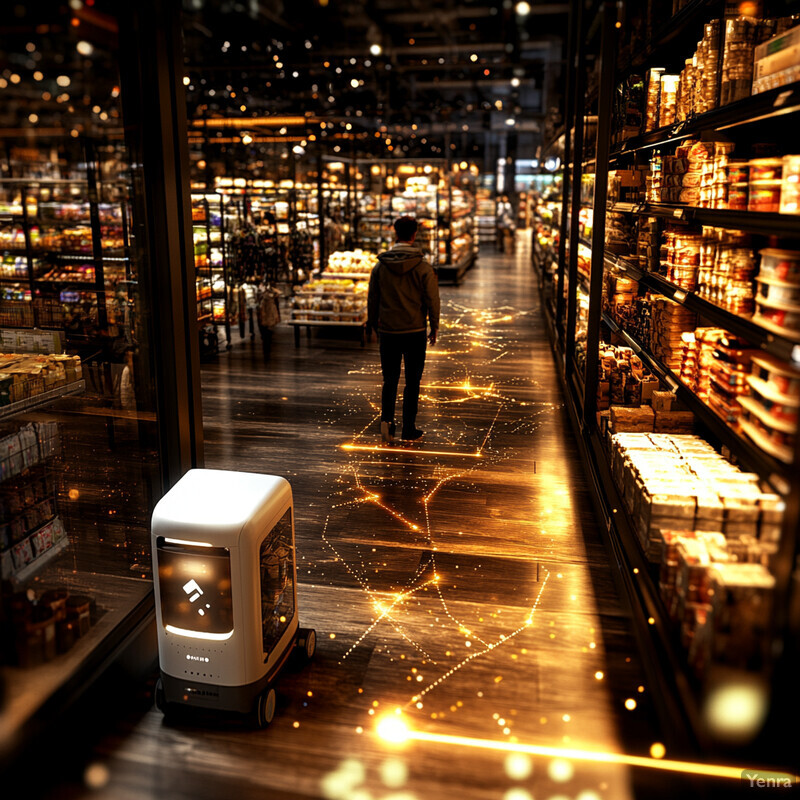
Research on dynamic path planning highlights several key methods. Surveys note that planners must optimize metrics such as path length, travel time, and computation time. Classic grid-based algorithms (e.g. A*) use heuristics for efficiency, while incremental planners like D* Lite can quickly replan when the environment changes. AbuJabal and Baziyad (2024) explain that D* Lite efficiently adapts to new obstacles in real time, enabling robots to reroute without re-planning from scratch. In practice, these dynamic algorithms allow robots to reduce travel distance and avoid traffic: studies indicate that enabling real-time rerouting significantly improves task completion rates and coverage efficiency.
4. Real-Time Inventory Accuracy
By scanning shelves continuously, robots provide up-to-the-minute inventory data. This real-time monitoring ensures the digital inventory matches physical stock, catching out-of-stocks or overstocks immediately. The result is vastly improved inventory accuracy: stores quickly identify missing items or misplaced stock. Automation also frees staff from periodic manual audits. In practice, companies report that robot-led scanning dramatically raises on-shelf accuracy and reduces the time lag between an item selling out and the system knowing about it.
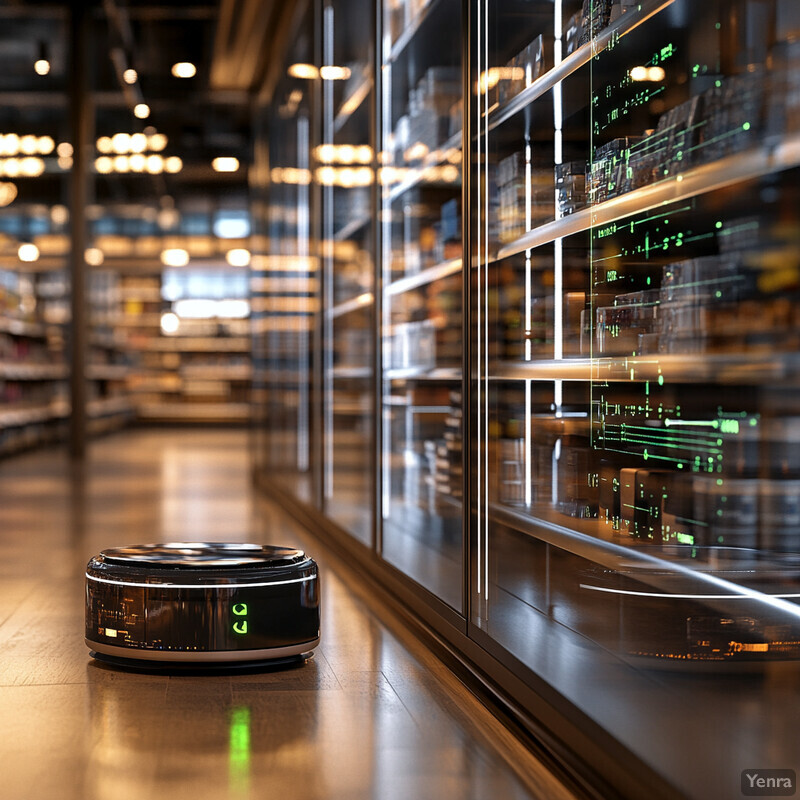
Accurate inventory tracking is known to be critical yet challenging. Rukundo et al. (2025) emphasize that real-time monitoring “directly impacts operational efficiency, customer satisfaction, and revenue”. By continuously updating counts, robots help maintain this accuracy. Indeed, industry surveys reflect broad trust in these tools: for example, Jabil reports that 94% of retail decision-makers are implementing or considering AI/data analytics to improve store inventory management. This overwhelming adoption underscores that near-real-time shelf data from robots is seen as a solution to chronic inventory errors and stockouts.
5. Product Misplacement Detection
AI-enabled robots automatically spot items that are shelved in the wrong location. By comparing live shelf images to the planned planogram layout, the system can flag misplacements or odd product combinations. This capability reduces human errors where an item drifts from its correct slot. As a result, stores maintain planogram compliance and ensure products are available in their designated locations without manual patrols.
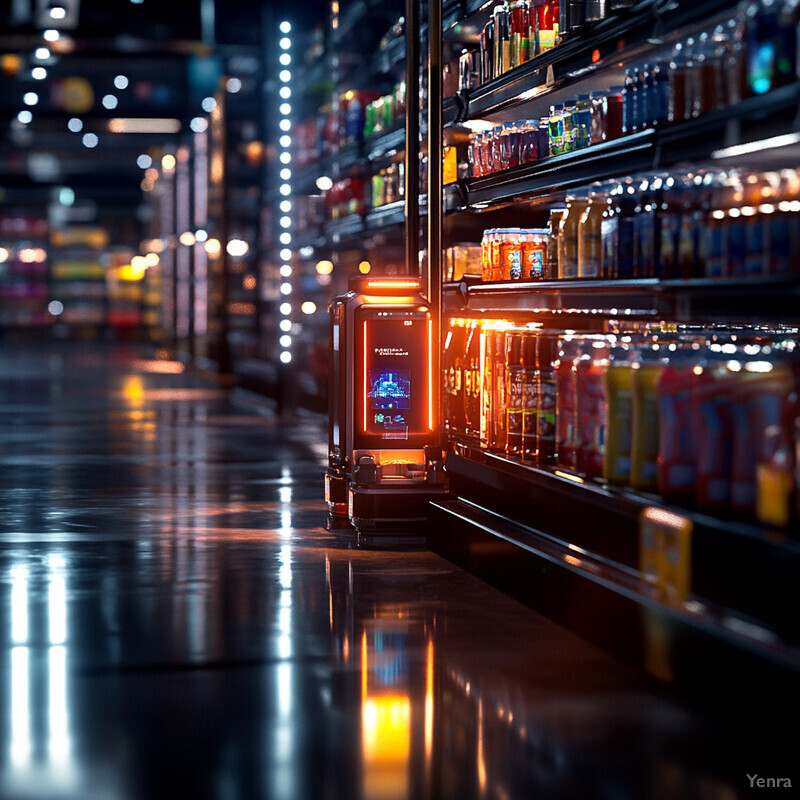
Modern vision systems can automate planogram audits and misplacement checks. For instance, V7 Labs demonstrates that object-detection AI can “automate product placement and planogram auditing”. In practice, this means robots can scan a shelf, identify each product, and compare their positions to the expected layout. Any deviation (a product in the wrong spot) is immediately flagged for correction. This automation greatly reduces the labor of manual shelf checks and helps keep planograms accurate, directly addressing common store compliance gaps.
6. Planogram Compliance Verification
Shelf-scanning robots verify that each product matches the store’s planogram (the specified shelf layout) and alerts staff to violations. They check not only that items are present, but that they are in the correct locations, right facing, and correctly priced per shelf tag. This continual verification ensures promotional and merchandising plans are implemented as intended. By automating this audit, stores maintain consistent merchandising and avoid customer confusion from misplaced or incorrectly displayed products.
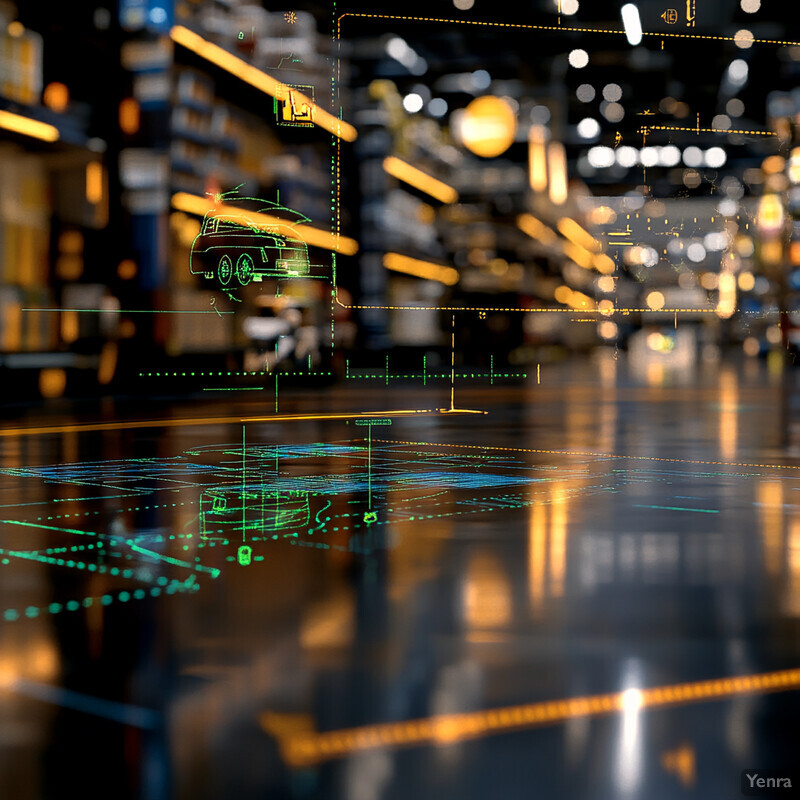
Research shows AI vision can achieve near-perfect planogram checks. Yücel et al. (2024) built an embedded vision system using YOLOv5 that achieved an F1 score of 1.0 (perfect) on planogram compliance tests. In practice, this means the system almost never misses a planogram violation. Commercial systems report similar capabilities: RFID Journal notes that Tally robots “provide inventory management to ensure goods are stocked on proper shelves with correct price labels”. Thus, automated scanning can catch planogram errors and incorrect labels at scale, enabling rapid correction.
7. Price and Label Validation
Robots scan shelf-edge price tags and labels to confirm pricing data and promotions. Their high-resolution cameras and OCR verify that the displayed price matches the store database and that promotional labels are correct. This process catches pricing errors or outdated tags faster than manual spot checks. Automated label validation ensures customers see accurate prices at all times, supporting dynamic pricing strategies and compliance with shelf tag changes.
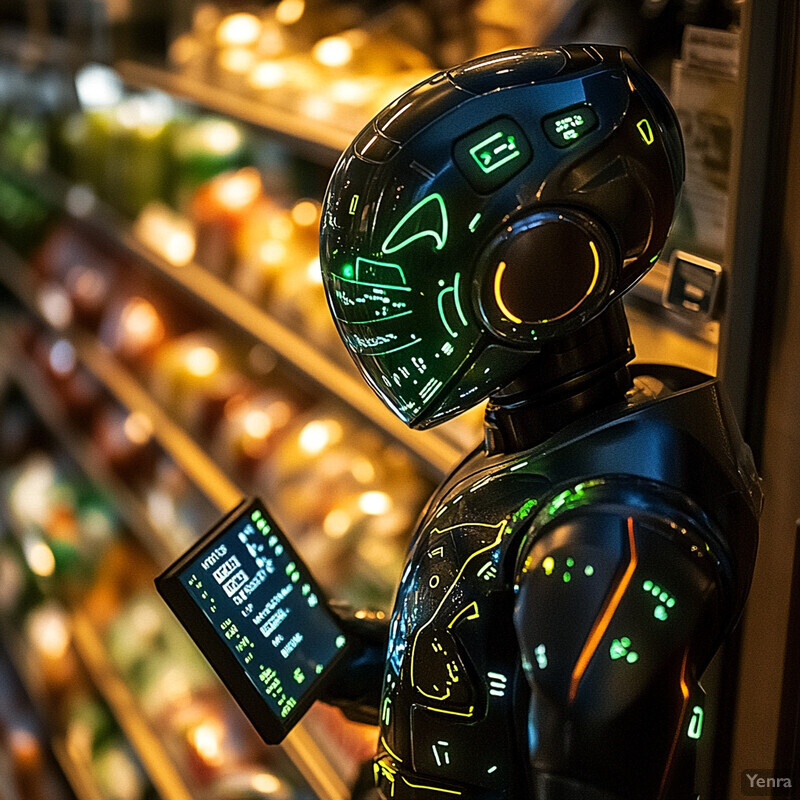
Shelf robots can read thousands of items per hour and identify pricing discrepancies. For example, Simbe’s Tally robot reads 15,000–30,000 products per hour, automatically flagging “mispriced” items and incorrect labels. It “reads price tags to automate the audit of pricing and promotional information”. Field deployments report dramatic drops in pricing errors: studies note that powered scans help drop labeling mistakes by 90%. Such high-throughput, automated price verification is far beyond human capability and greatly enhances shelf accuracy.
8. Pattern Recognition and Trend Analysis
The vast image and inventory data from shelf scans are analyzed for trends and patterns. Machine learning identifies which products sell best, customer browsing habits, and seasonal shifts. These insights help optimize stock levels and shelving layouts. For example, recognizing patterns of frequent stockouts on certain aisles can guide restocking strategies. In essence, robots provide raw data that algorithms turn into actionable business intelligence about demand and product trends.
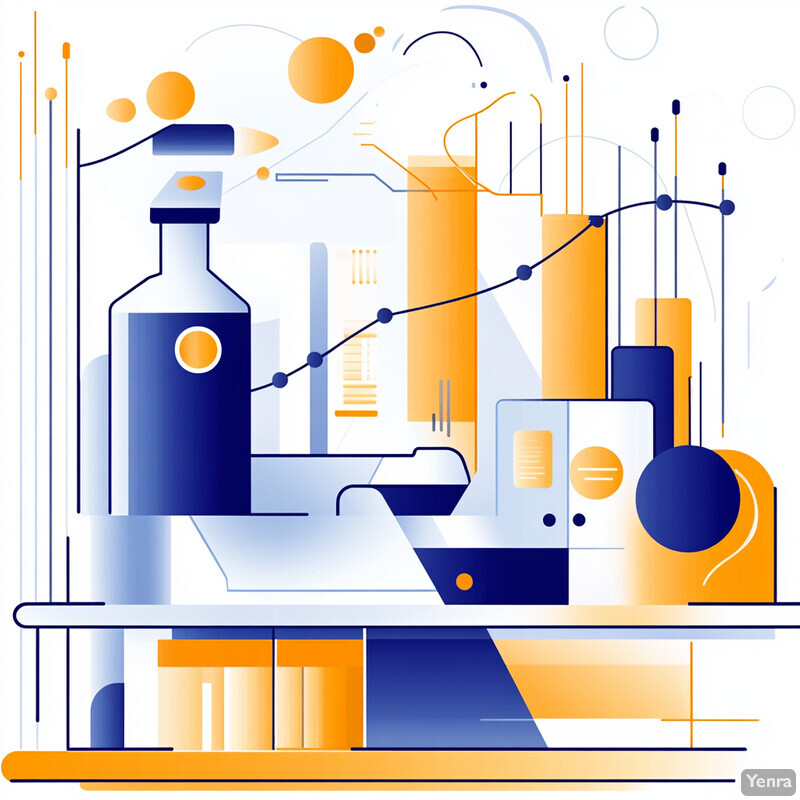
AI trend analysis has been shown to significantly reduce waste and out-of-stocks. Industry reports citing McKinsey data reveal that retailers using AI-driven analytics have “reduced inventory waste by 30–50% and saw a 65% decrease in out-of-stock situations”. This implies that analyzing shelf data for trends (such as rapidly selling SKUs) directly improves operations. In practice, shelf-scanning robots feed timely sales and stocking data into these analytics platforms, enabling such dramatic improvements in forecast accuracy and trend spotting.
9. Predictive Inventory Forecasting
Robots’ continuous data feed enables AI to forecast future demand. Machine learning models (time-series, regression, LSTM, etc.) use scan data to predict when items will run out. This predictive insight allows managers to reorder stock just-in-time and optimize inventory levels. Compared to traditional forecasts, AI models better capture local demand fluctuations and seasonal effects. Over time, forecasts become more accurate as the system learns from real sales vs. predictions.
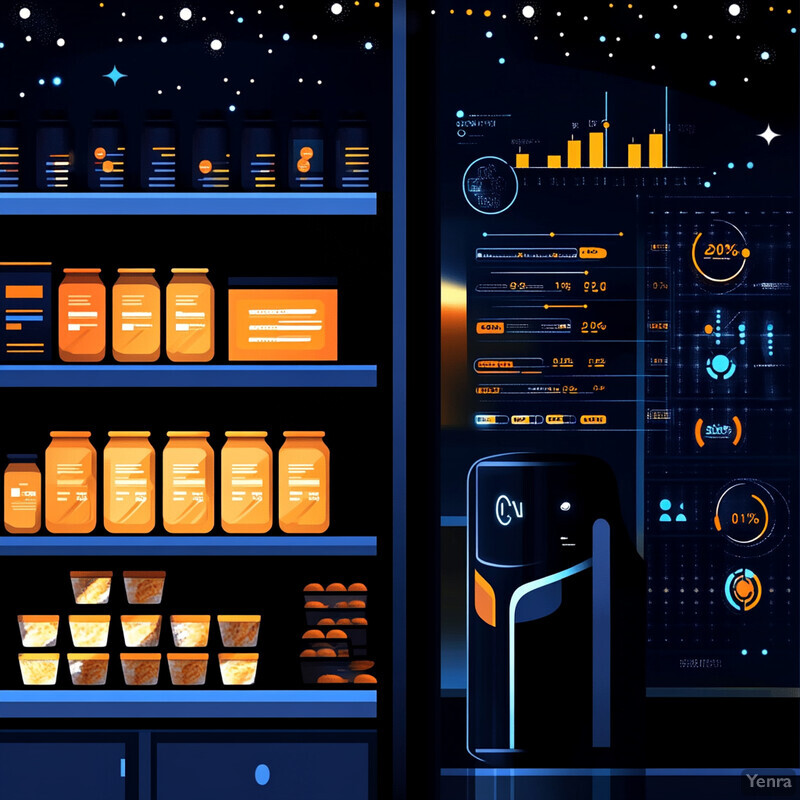
Academic work shows AI forecasting outperforms classical methods. Singh et al. (2024) compared various ML algorithms on retail inventory data and “demonstrate[d] the superior performance of ML-based methods compared to traditional inventory management systems,” achieving markedly higher accuracy and fewer stockouts. Their study highlights substantial improvements in “inventory accuracy” and “enhanced operational efficiency” when using ML forecasting. These findings confirm that embedding robot-captured data in AI forecasting systems can greatly improve inventory outcomes.
10. Multi-Robot Collaboration
In large stores, multiple robots work together, coordinating their routes and tasks. They share mapped floor plans and inventory data, avoiding collisions and redundant scanning. For example, when one robot scans a row, another focuses elsewhere. This teamwork divides the workload and covers more area quickly. Collaboration protocols ensure robots hand off information (like discovered out-of-stocks) and schedule scanning so that staffing needs are minimized and full coverage is maintained.
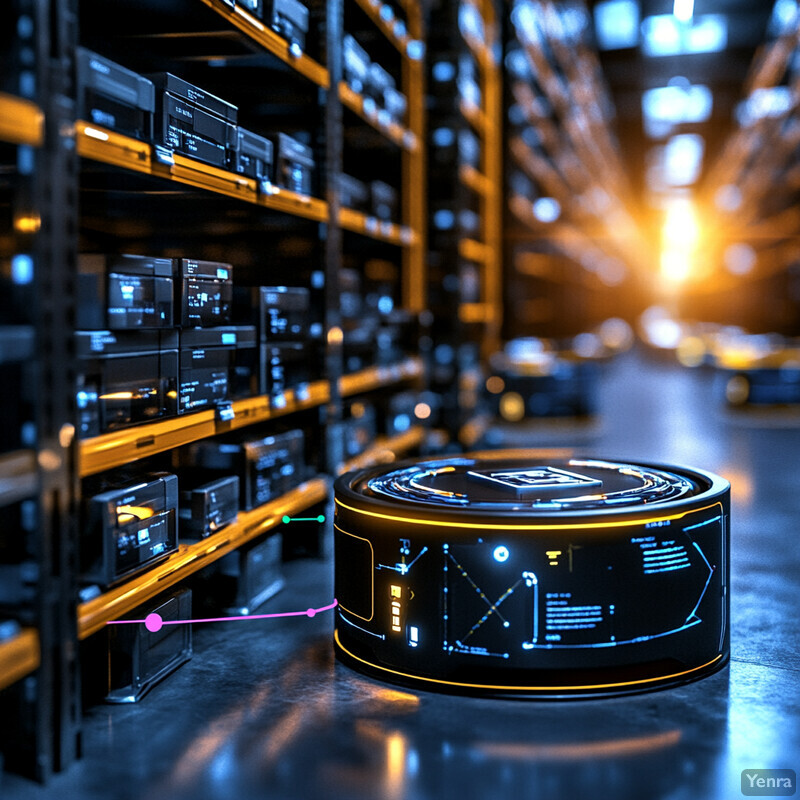
Research confirms that coordinated multi-robot systems greatly improve efficiency. Wang and Liu (2024) developed a multi-robot framework where robots use a YOLOv5 detection model and reinforcement learning (PPO) to coordinate. They report that their system can “achieve multi-robot collaborative operation, significantly improve task completion efficiency, and maintain…high accuracy” in various scenarios. In other words, compared to independent operation, collaborative routing and task allocation enabled faster coverage and higher throughput while preserving detection accuracy. This demonstrates the benefit of AI-enabled teamwork among shelf-scan robots.
11. Contextual Understanding of Store Layouts
Shelf-scanning robots build and use a map of the store layout to contextualize their scans. They recognize which aisle and shelf a product belongs to and use this to interpret scan data correctly. For instance, knowing the physical location helps robots confirm items are in the correct category area. Some systems also use knowledge of store “zones” or customer traffic patterns. This spatial understanding allows robots to plan their routes intelligently and correlate shelf images with the intended placement.
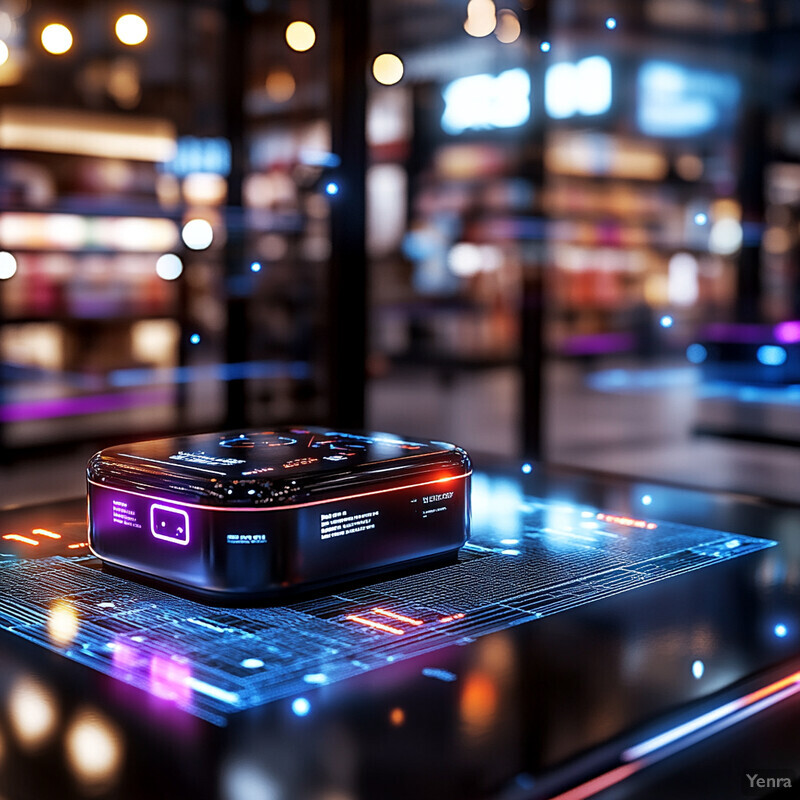
Robots tag each scan with precise location information. For example, Brain Corp explains that as a robot moves “up and down the aisles, it takes high-resolution photos…so that the location of the scan is known”. In this way, each product scan is linked to a shelf coordinate, enabling automatic checking of “products are located where they should be”. On a broader level, retailers use computer vision to analyze store layouts; Tchoe (2025) notes that companies create heat maps of customer traffic (“retail heat maps”) so staff can optimize shelf placement and product arrangement. Together, these contextual tools (robot mapping and analytical models) ensure that shelf data is interpreted with spatial awareness.
12. Robust Object Detection in Crowded Aisles
Robots must detect products in busy environments—aisles with many overlapping items or moving shoppers. Robust detectors are trained to handle occlusions (when a product is partially hidden) and clutter. For example, the vision system may use multiple viewpoints or combine 2D vision with depth sensors to recognize items even when shelves are crowded. Improving robustness allows the robot to correctly identify most products on a densely stocked shelf, maintaining inventory accuracy despite visual complexity.
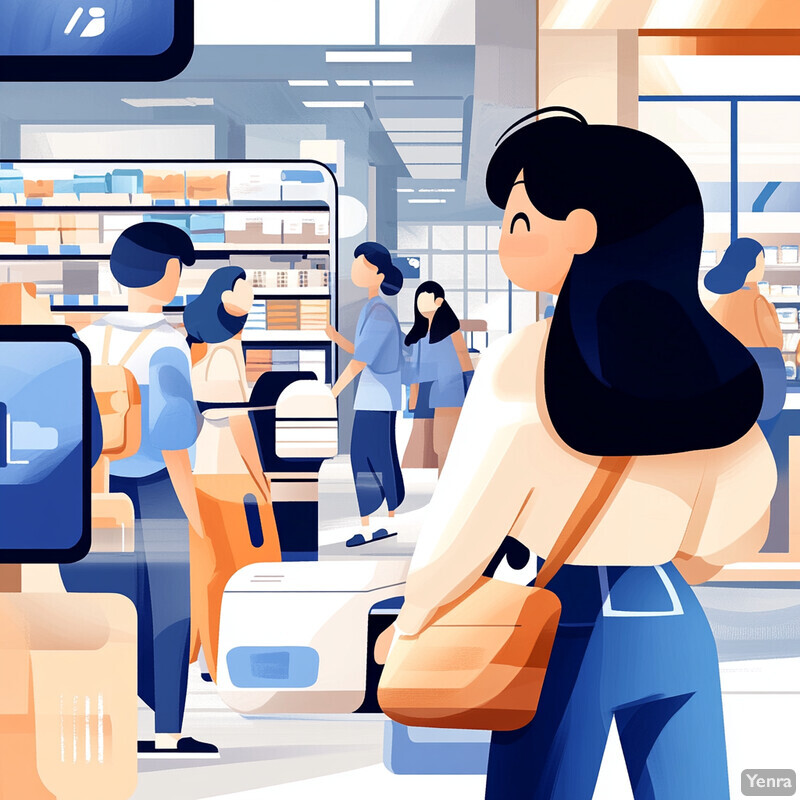
Analysis of retail vision systems highlights occlusion as a key challenge. Rukundo et al. (2025) point out that when “products may be hidden by other items, customer hands, or store layouts,” real-time recognition becomes difficult. To counter this, some systems use multiple sensor modalities or ensemble models. In practice, successful implementations might combine RGB images with depth data or deploy cameras at staggered angles, thus improving detection under crowding. These strategies, informed by the noted limitations, help robots reliably scan even congested aisles.
13. Integration with Backend Systems
For shelf-scan data to be actionable, robots interface with the store’s backend (WMS, ERP, inventory database). Scan results (e.g. product counts, tag data) are uploaded to the retail management system in real time. This integration lets inventory systems update stock levels automatically. It also enables centralized analytics on robot-collected data. Seamless data flow ensures that shelf information (like out-of-stocks or pricing) immediately triggers backend alerts or replenishment orders.
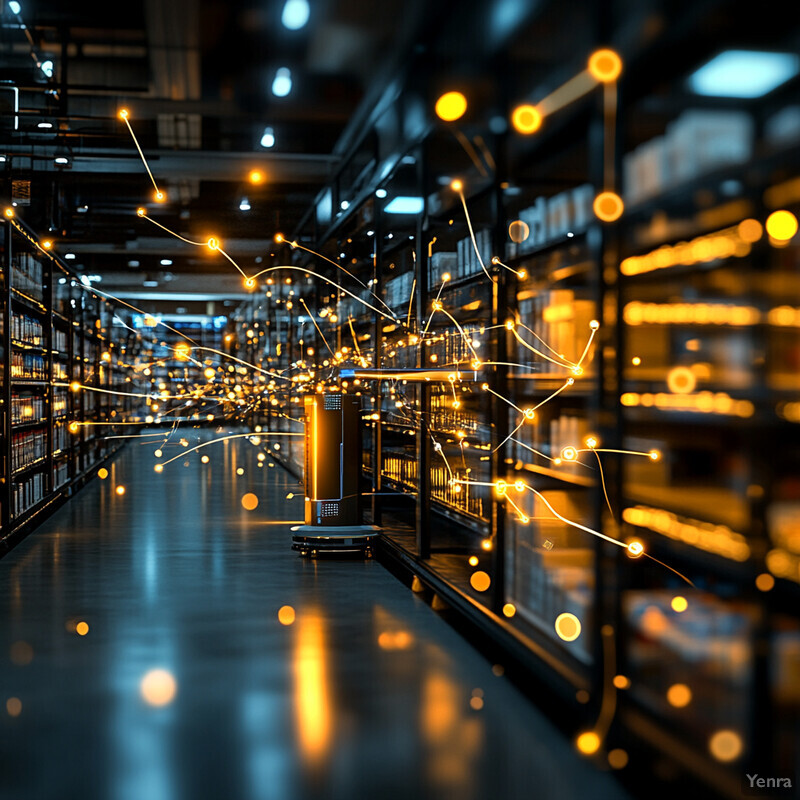
Effective automation requires tight integration with back-end systems. Marvelmind notes that barcode/RFID scanning “only exhibits (its) true potential when… wirelessly connected to WMS or ERP systems”. In other words, raw scans must feed into the store’s data systems to update inventory and product information. They also point out that retail deployments often have more complex back-end needs than warehouses, since the retail environment is less structured. This underscores that shelf-scanning robots are built on a cloud/ERP infrastructure, ensuring scan data directly updates store management software.
14. Anomaly Detection and Alerts
Robots use AI to recognize unusual events or patterns. This includes detecting spilled products, damaged packaging, or suspicious customer behavior (potential theft). For example, vision models can flag when stock levels suddenly drop or when a customer stays too long in one aisle. When an anomaly is detected, the system generates an alert to staff. These real-time alerts help managers quickly address issues like safety hazards or shrinkage, rather than discovering them later through manual checks.
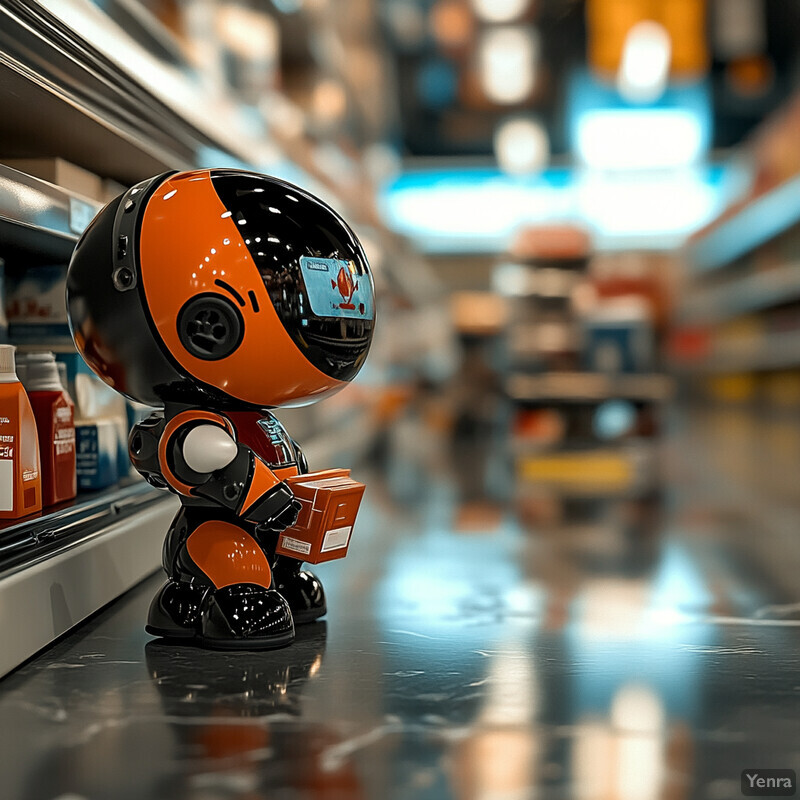
Treating anomalies as deviations from normal patterns is an emerging approach. Ghazal et al. (2025) formulated shoplifting detection as an anomaly-detection task on retail video, using human pose data. Their “pose-based” models achieved high accuracy in identifying theft behaviors. The need is clear: retail theft cost an estimated $121.1 billion in the U.S. in 2023. By analogy, shelf-scanning robots (often equipped with cameras) can flag anomalies like unexpected movements or missing items. This application of computer vision to anomaly detection promises to reduce losses by catching unusual events as they happen.
15. Advanced Sensor Fusion
Robots often use multiple sensors to boost reliability. For example, combining camera vision with weight sensors or vibration sensors on shelves can verify if an item was removed. This sensor fusion reduces false negatives (like an item hidden from view) by cross-checking signals. Fusion may also include LiDAR to avoid collisions or thermal sensors in refrigerated aisles. By intelligently merging data from different sources, robots achieve more accurate detection and inventory estimates than with vision alone.
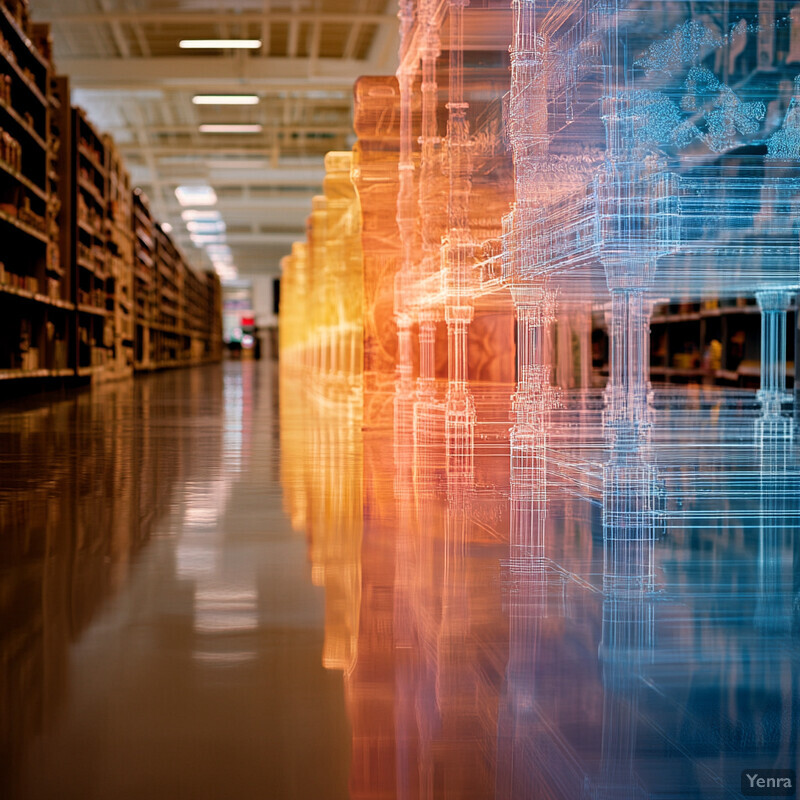
Multi-modal sensing greatly enhances inventory detection. Rukundo et al. (2025) describe systems that “combine weight, vision, and vibration-based sensing for more accurate inventory monitoring”. Such fused systems have been shown to improve recall when vision alone would miss occluded items (because the weight sensor detects an unremoved product). The tradeoff is increased computation, but studies indicate that despite this, multi-sensor robots achieve higher overall accuracy in practice. Thus, sensor fusion is a promising path for robust shelf monitoring.
16. Voice-Assisted Management Interfaces
Robots increasingly feature voice interfaces for staff. Managers can ask the robot questions (e.g. “Which aisle is out of milk?”) or receive spoken alerts. These natural-language interfaces use speech recognition and dialogue models to interpret queries. This hands-free interaction helps workers get information (like inventory status or robot status) without a screen. It also simplifies robot control: e.g. staff can tell a robot to “scan aisle 5,” and the robot understands the command.
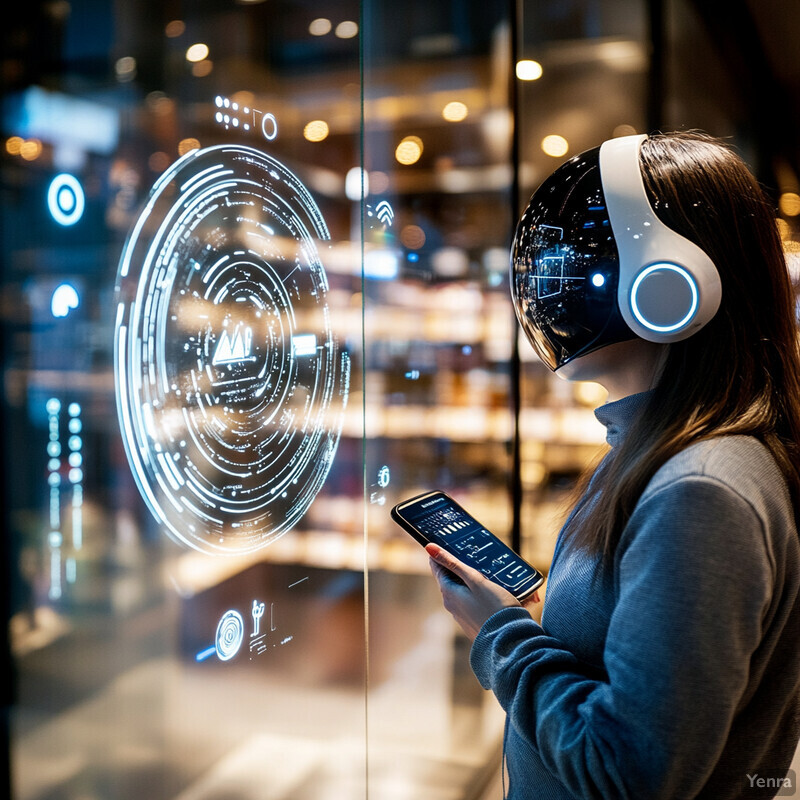
Voice-robot interfaces in retail are under development. Nandkumar & Peternel (2025) created a voice-based conversational agent for supermarket robots. They evaluated multiple speech-recognition technologies and found OpenAI’s Whisper achieved the best accuracy across genders and languages. Their multi-model chatbot (using LLMs) outperformed a baseline GPT-4 system in user satisfaction and efficiency. Importantly, the system maps user queries to shelf locations, so spoken instructions lead the robot to the correct aisle (“mapping the final chatbot response to the correct shelf numbers”). This demonstrates that advanced voice interfaces can effectively guide shelf-scanning robots and help staff use them naturally.
17. Augmented Reality (AR) Insights for Staff
Augmented reality gives store staff enhanced visibility into shelf conditions. For example, staff can use a tablet or AR glasses to see live overlays of inventory data on top of actual shelves. They might point a device at a shelf and see icons indicating stock levels or expiration dates. Alternatively, robots enable remote monitoring: staff can view robot-captured images in a mobile app as if taking a virtual tour of the store. These AR-like tools turn the robot’s scans into actionable insights that employees can use on the spot.

Vendors are delivering mobile viewing of robot scans. Grocery Dive reports that Simbe Robotics has launched an application allowing store employees to “remotely monitor…inventory levels, product layouts and other aspects” on smartphones and desktops. The system provides high-definition snapshots and time-lapse footage from the robot’s cameras. In other words, it uses robot-collected imagery as a form of AR dashboard: staff get a realistic view of shelf states in real time. These virtual insights help employees make data-driven decisions without physically trailing the robot.
18. Energy and Maintenance Optimization
AI can optimize a robot’s energy use and maintenance schedule. For example, robots may learn the best times and paths to recharge their batteries with minimal downtime (e.g. returning to dock during low-traffic periods). Predictive maintenance uses sensor data (motor currents, temperature) to forecast parts wear and schedule service before failures occur. Overall, this reduces unplanned downtime and extends battery lifespan, ensuring robots remain operational longer between charges and repairs.
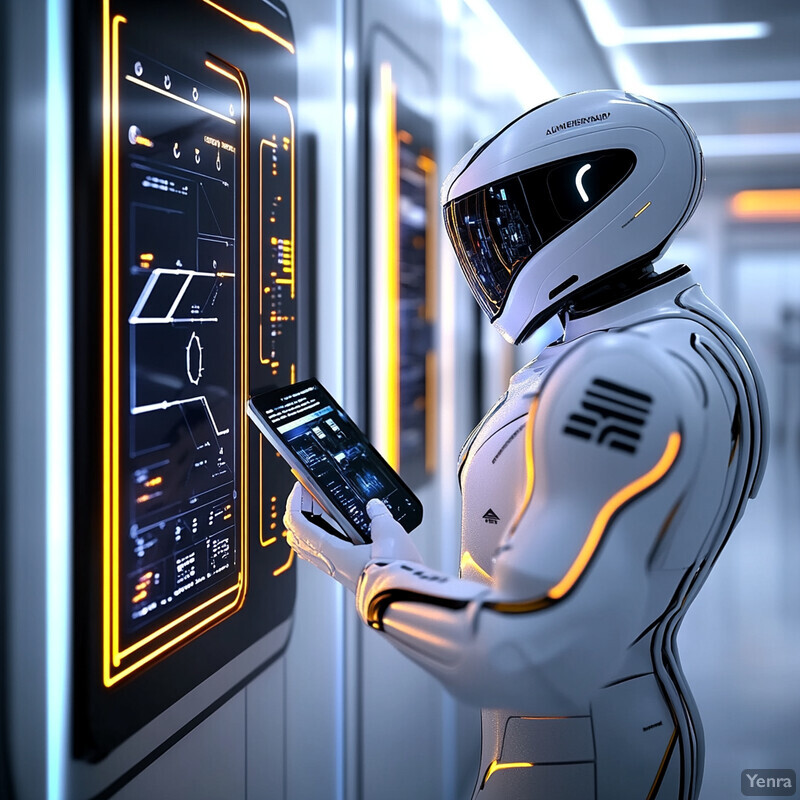
Predictive maintenance is crucial for reliable operation. Gromov (2024) explains that data-driven maintenance planning is a “game-changer” for robotics: by forecasting failures, it allows strategic scheduling of repairs rather than reactive fixes. This approach means robots incur far less unplanned downtime. In practice, shelf robots transmit health and usage data to the cloud, where AI models predict when components like wheels or batteries need service. This proactive maintenance (often termed “fail-safe” operation) has been shown to significantly reduce maintenance costs and increase robot availability.
19. Continuous Improvement through Cloud-Based Analytics
Shelf-scanning robots connect to cloud platforms where data is aggregated and analyzed over time. This enables continuous learning: manufacturers update AI models with new data (e.g. new product images) and deploy improvements to all robots remotely. Retailers benefit from longitudinal analytics (e.g. monthly reports on stock trends). Essentially, cloud connectivity means insights from one store can improve robots in another, and the system perpetually refines its scanning algorithms and business analytics as more data is collected.
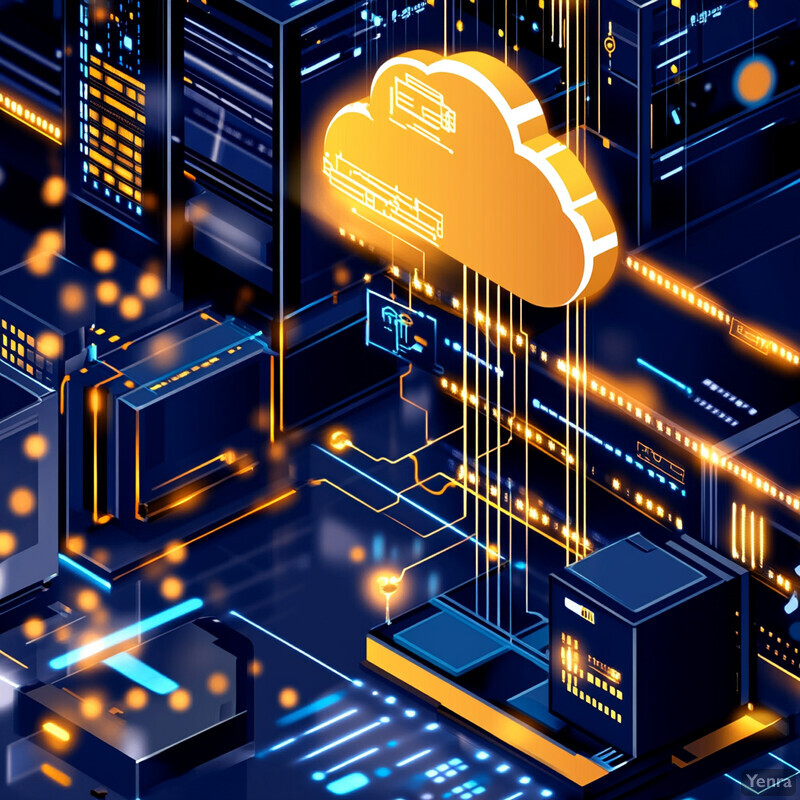
Cloud analytics are integral to modern retail robotics. Badger Technologies reports that its cloud-based robotic platform “produces analytics and reports to improve floor and shelf conditions”. For instance, a press release notes that cloud connectivity lets busy Beaver stores use aggregated scan data to monitor on-shelf availability and price accuracy in real time. By continuously uploading scan results to the cloud, these systems enable data scientists to spot long-term trends and retrain recognition models. Over months of operation, the algorithms are iteratively improved, leading to better accuracy and more actionable insights with each software update.