1. Optimized Growth Conditions
AI systems are enabling more precise control over algae cultivation environments. By continuously analyzing sensor data, AI can adjust factors like light, temperature, pH, and CO₂ in tandem, far beyond the trial-and-error methods of the past. This means algae are grown under near-optimal conditions at all times, which accelerates growth and boosts biomass yields. The approach also reduces wasted resources, since conditions are fine-tuned to what the algae actually need rather than broad guesses. Overall, AI-driven optimization creates a more stable and high-performing growth environment for algae biofuel production.
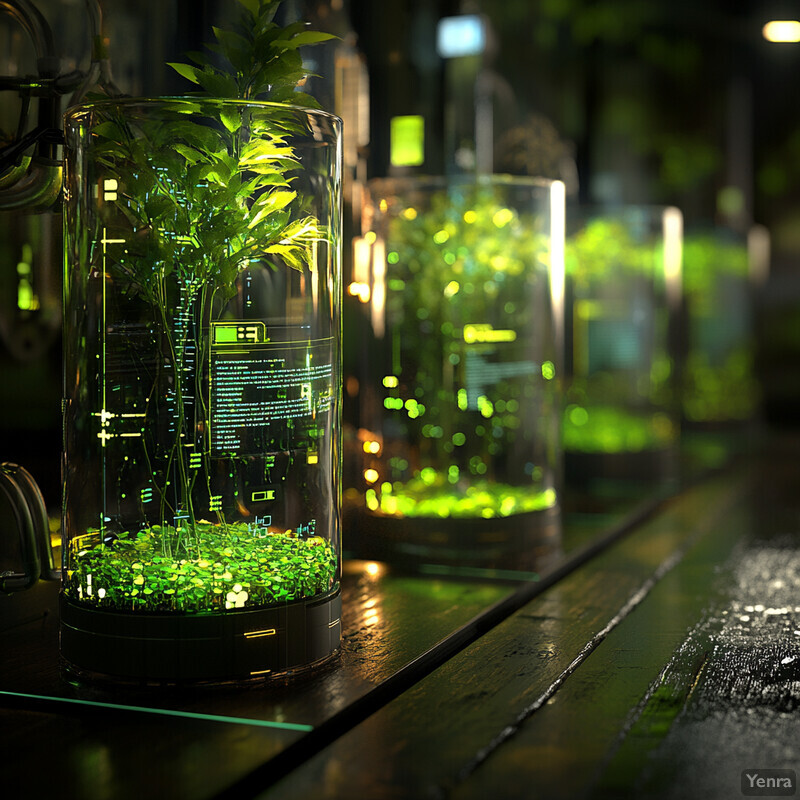
Integrating AI into algae cultivation has been shown to significantly improve efficiency and output. A recent review indicated that an AI-driven framework could increase microalgal biomass yields by 30–50% while cutting nutrient and energy use, and improving process control accuracy in large-scale operations. In one example, a machine-learning model (artificial neural network) accurately identified the optimal growth parameters for a cyanobacterium, achieving an R² of 0.97 in predicting growth — a 76% improvement in accuracy over traditional models. These advanced models capture complex nonlinear relationships among multiple variables simultaneously, something traditional single-factor optimizations miss. The result is a cultivation setup where all key conditions are continually balanced for maximum productivity, rather than optimized in isolation.
2. Intelligent Nutrient Dosing
AI ensures that algae receive the right nutrients at the right time and in the right proportions. Instead of providing a constant nutrient supply (which can lead to waste or imbalances), an AI-driven system monitors the algae’s growth stage and metabolism to dynamically adjust dosing. By doing so, the algae are neither overfed nor undernourished at any point. This intelligent dosing maximizes nutrient uptake efficiency, supports faster growth, and minimizes excess nutrients that could otherwise cause issues like unwanted bacterial growth or the need for extra water treatment. In short, AI makes nutrient delivery more precise and responsive to algal needs.
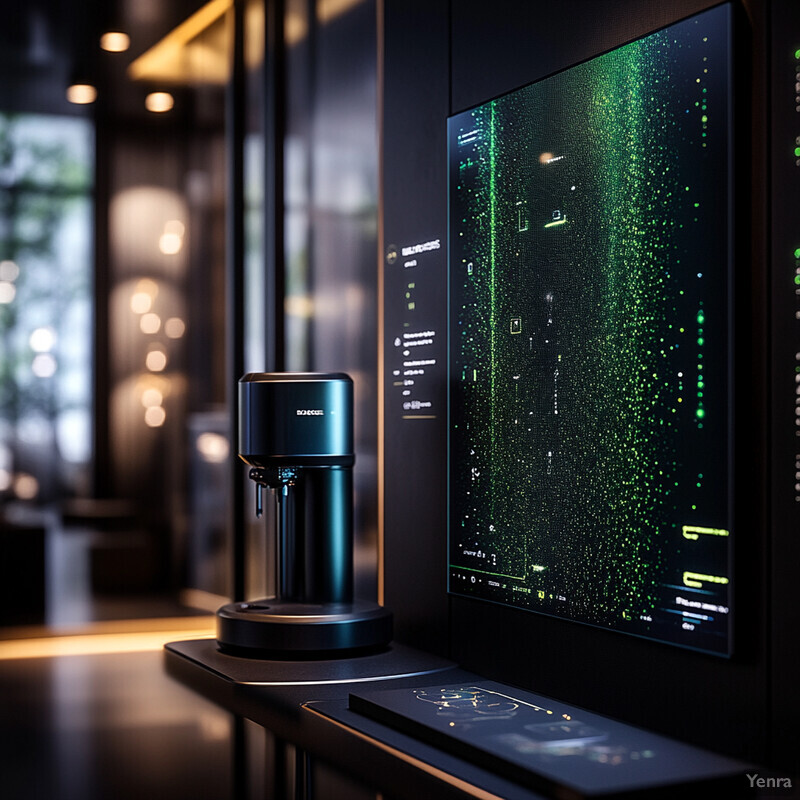
Machine learning models are being used to optimize both the timing and ratio of nutrients in algae cultivation. For example, a support vector regression model augmented with a genetic algorithm identified an optimal nitrogen-to-phosphorus (N:P) ratio of 6:1 for growing Chlorella algae in wastewater, achieving over 93% nutrient removal efficiency under those conditions. The AI model’s predictions were highly accurate (within ~3% error) in maintaining this balance. This demonstrates that AI can pinpoint nutrient dosing strategies far more precisely than conventional methods. Similarly, other studies use AI to decide when to add nutrients or carbon (like CO₂) based on real-time algae growth data, ensuring nutrients are supplied exactly when the algae can best utilize them. By balancing nutrient supply with algal demand, these systems boost growth rates and lipid yields while preventing problems like nutrient waste or culture crashes due to overfeeding.
3. Automated Light Management
AI is used to automatically manage lighting conditions for algae, tailoring both light intensity and photoperiod (day-night cycle) to the algae’s needs. Algae require light for photosynthesis, but too much or poorly timed light can be inefficient or even harmful. AI-driven light systems adjust the brightness and duration of light exposure in real time. During periods when algae can photosynthesize more, the system can increase light intensity or extend daylight hours; during less active periods, it can dim or shut off lights to save energy. This continuous optimization ensures algae get optimal light for growth and product formation (like oils), while avoiding energy waste from unnecessary lighting.
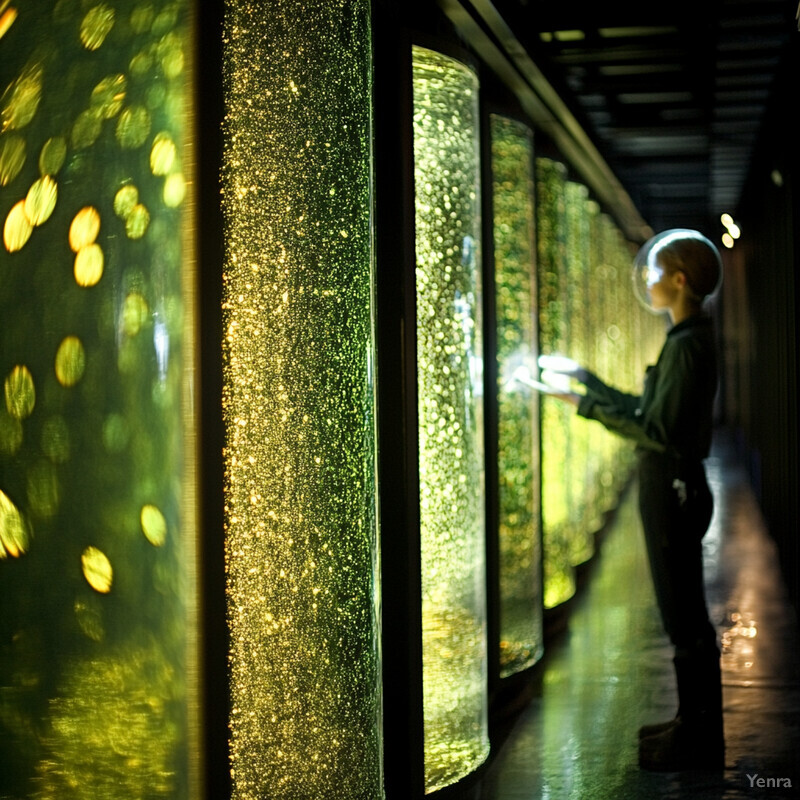
Smart lighting systems guided by AI have demonstrated measurable gains in algae productivity. In one 2024 study, researchers used machine learning to control a photobioreactor’s lighting schedule. They found that switching from a traditional 12-hour light cycle to a continuous 24-hour light regimen (enabled by AI insights) improved algae biomass productivity by about 7.2%. The AI model (an eXtreme Gradient Boosting algorithm) was extremely accurate in forecasting growth under different lighting, with an R² of 0.9997 when predicting algae biomass. This high precision allowed the system to fine-tune light intensity and duration to match the algae’s instantaneous needs. Another AI approach identified an optimal light intensity (~1000 lux) and cycle (12:12 hours light-dark) for maximizing a specific algae product (polyphenols), outperforming human-derived settings. These examples show that AI can uncover non-intuitive lighting strategies that yield more biomass or desired bioproducts, all while potentially reducing energy usage by avoiding over-illumination.
4. Real-time Water Quality Monitoring
AI enables continuous monitoring of water quality in algae farms and can instantly adjust conditions to keep the water optimal. Key water parameters include temperature, pH, dissolved oxygen, turbidity (clarity), and nutrient concentrations. Traditionally, farmers would manually check these or rely on basic controls, but AI systems now aggregate data from an array of sensors in real time. If pH drifts out of range, the AI might add a buffer; if oxygen drops, it can increase aeration; if water gets too warm, it might cool it or adjust light intensity. This immediate responsiveness maintains a stable environment for the algae, which prevents stress and promotes steady growth. Essentially, AI acts like an autonomous caretaker for the algae’s aquatic environment.
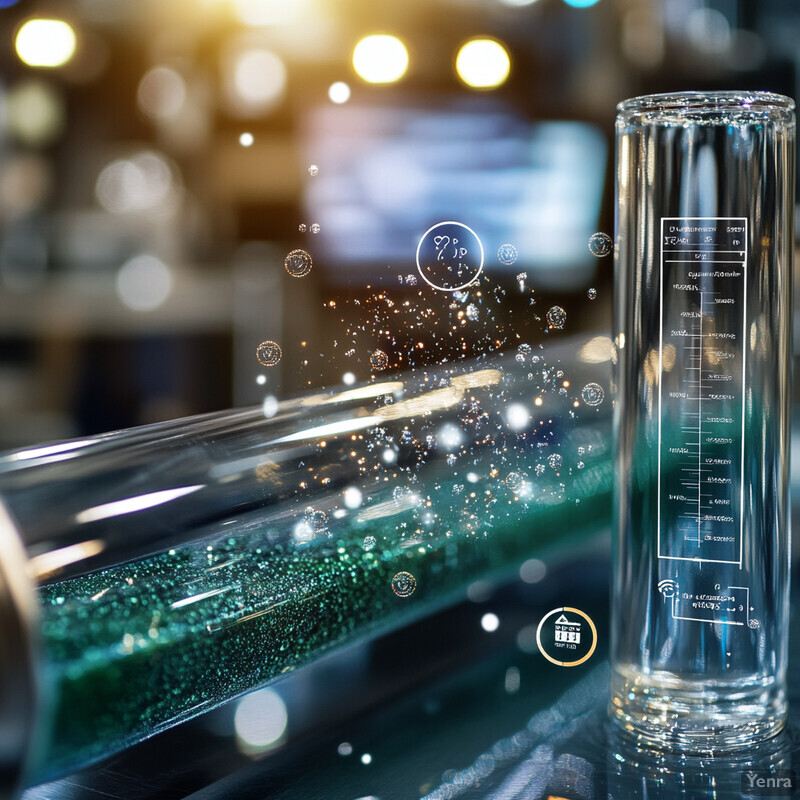
In practice, IoT-integrated AI systems have demonstrated an ability to keep water conditions within tight optimal ranges. One case involved an intelligent cultivation system for Arthrospira (spirulina) that used sensors and microcontrollers to regulate water quality in real time. The AI-enhanced system maintained the water at 30°C, kept turbidity (which indicates algal density and clarity) at ideal levels, and balanced dissolved gases by adjusting oxygen and CO2 flows. It also modulated ultraviolet light intensity to prevent water overheating. By continuously processing sensor inputs, the AI could detect even minor deviations (like a slight drop in dissolved oxygen or a pH shift) and immediately correct them. This level of real-time control is difficult to achieve with manual monitoring. The outcome is a consistently healthy culture environment: algae experience less shock from parameter swings and therefore can sustain higher growth rates and product quality. This approach also reduces the likelihood of culture crashes that might occur if water quality issues went unnoticed for hours or days.
5. Early Detection of Contaminants
AI helps identify contaminants (like invasive microorganisms or toxins) in algae cultures at a very early stage. Algae ponds and bioreactors can be vulnerable to infections by pests such as contaminant algae, fungi, or bacteria, which can quickly bloom and wipe out a culture. Traditionally, farmers might only notice a problem after a visible crash or lab test, by which time significant damage is done. AI changes this by continuously analyzing indicators of contamination – this could be sensor readings (pH or oxygen changes), imaging data (microscopic images of the culture), or genetic data (metagenomic signals of foreign organisms). By recognizing the subtle signs of contamination in advance, AI can alert operators or automatically take mitigation steps (like adjusting conditions to suppress the contaminant or isolating the affected batch). The goal is to prevent minor incursions from escalating into full-blown culture crashes.
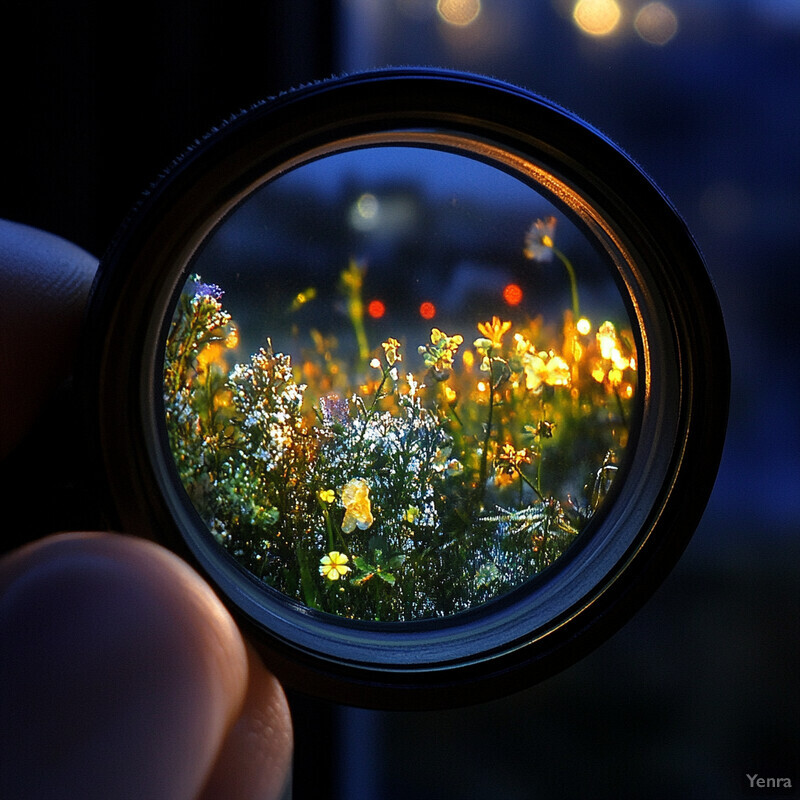
A U.S. Department of Energy-funded project in 2024 exemplifies how AI can predict and preempt contamination in algae biofuel cultivation. At the University at Buffalo, researchers are using AI and low-cost microscopy to monitor algae cultures continuously for signs of micro-pest infections. The AI analyzes environmental data (temperature, sunlight, nutrient levels) alongside genomic data from the algae (DNA/RNA) to detect the early stress signals that occur before a pest attack becomes visible. The ultimate aim is to “predict the infections before they occur”. This proactive approach is crucial because once a fungal or bacterial contaminant overtakes a monoculture, it can wipe out 30–50% of the biomass product and force a complete restart of the system. By catching an infection in its nascent stage, operators could, for example, dose a targeted treatment or remove a portion of culture, thereby saving most of the biomass. Early AI-driven detection thus acts as an insurance policy for algae farms, significantly reducing downtime and losses associated with contamination events.
6. Predictive Harvest Scheduling
AI can forecast the growth trajectory of an algae culture and predict the optimal harvest time for maximum yield. Instead of harvesting on a fixed schedule (which might miss the peak or allow overgrowth issues), predictive models use inputs like current biomass density, growth rate, nutrient status, and even weather forecasts (for outdoor systems) to determine when the algae will reach their prime. By scheduling harvests at the ideal time, farmers can extract the greatest amount of biomass or oil when the algae are most productive. Additionally, predictive scheduling can stagger harvests across multiple ponds to ensure a steady supply of biomass for processing, smoothing out what would otherwise be a highly variable production cycle.
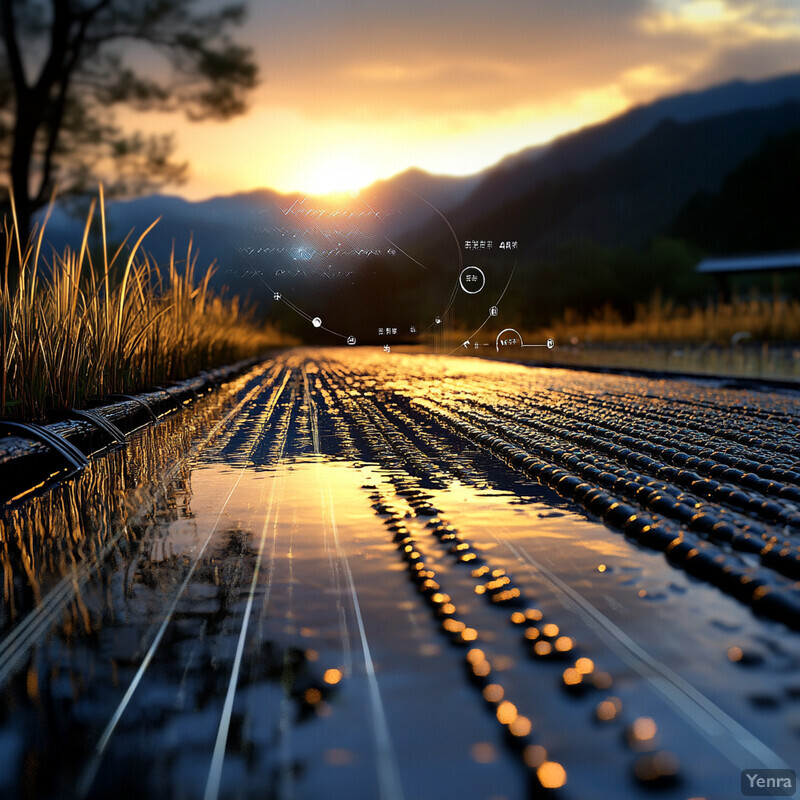
Recent advancements have shown substantial gains from forecast-based harvest strategies. Scientists developed a “forecast-informed pond operation” system that uses machine learning in combination with weather predictions to decide when to harvest outdoor algae ponds. In simulations, using biomass growth forecasts to drive harvest timing improved overall algae biomass production by approximately 20–47% compared to routine, fixed-schedule harvesting. In essence, the AI model could predict imminent slowdowns in growth (for instance, due to an upcoming string of cloudy days or nutrient depletion) and recommend harvesting just before those dips. Conversely, it could extend the growing period when conditions are predicted to remain optimal. Such scheduling ensured that each harvest captures algae at peak biomass. Moreover, a 2025 study incorporating these methods projected that smarter harvest timing and pond management could dramatically reduce production costs – achieving far higher annual yields without additional inputs. These findings underscore that predictive scheduling, enabled by AI, allows algae producers to consistently hit the “sweet spot” for harvesting, thereby maximizing output and efficiency in biofuel production.
7. Strain Selection and Breeding
AI accelerates the identification and development of superior algae strains for biofuel. Selecting the right strain (or breeding a new one) is crucial because different algae have vastly different growth rates, oil content, and resilience. Traditionally, strain selection involved lengthy trial-and-error experiments and random mutagenesis. Now, AI can analyze genetic and phenotypic data from many strains to predict which ones have the best traits (like high lipid production or fast growth). It can also guide breeding or genetic engineering by pinpointing which genes or traits to combine. This data-driven approach helps biofuel producers focus on the most promising algae candidates and even discover novel strains or optimize existing ones much faster than before.
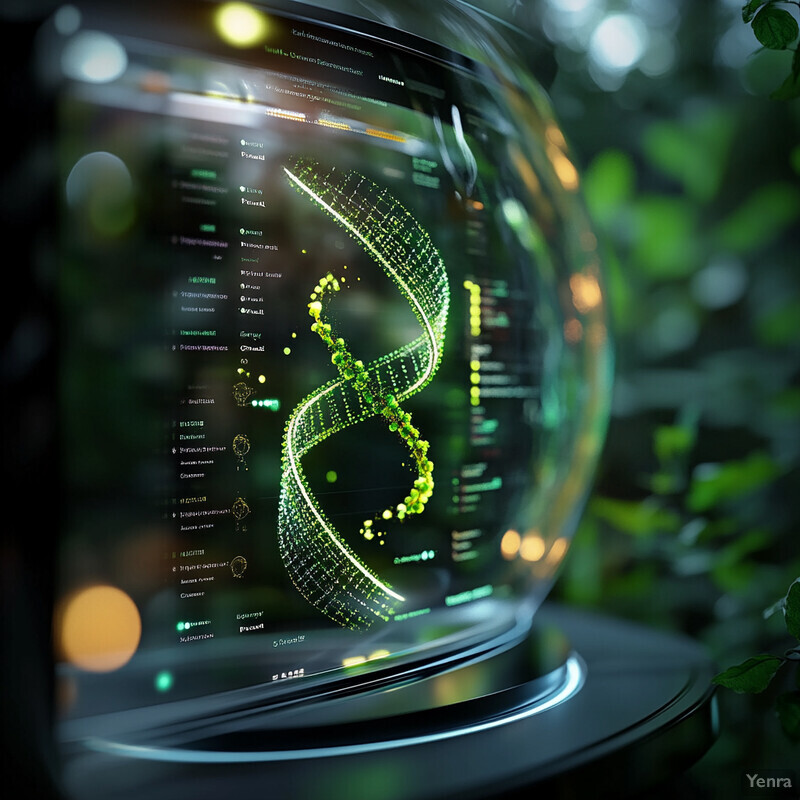
Machine learning has been used to sift through vast datasets of algae characteristics to find high-performing strains and optimal trait combinations. In 2023, researchers compiled data from 102 studies on microalgal biomass and lipid production and trained a decision-tree model on 4,670 data points to identify key factors for success. The AI model highlighted 11 specific combinations of conditions that consistently led to high biomass productivity and 13 combinations that maximized lipid (oil) content. This kind of analysis helps researchers zero in on which strain–environment interactions yield the best biofuel precursors. Additionally, AI is aiding in genetic selection: for instance, an ensemble machine learning approach achieved 97% accuracy in classifying microalgae species and their growth stages using single-cell Raman spectra, facilitating the recognition of strains with desired metabolic profiles. There have also been cases where AI models identified genetic markers linked to high lipid yields in algae. Armed with that knowledge, scientists can target those genes in breeding programs or genetic engineering. In summary, AI reduces the guesswork in strain selection by quickly highlighting which algae (or genetic modifications) are most likely to thrive and produce abundant biofuel feedstock.
8. Enhanced Carbon Capture Efficiency
AI improves how effectively algae capture carbon dioxide, which is central to both growth and climate benefits. Algae use CO2 as a raw material to grow, and many systems feed algae with concentrated CO2 (often from industrial emissions) to boost productivity. AI can manage CO2 delivery by adjusting flow rates, timing, and concentration to match the algae’s uptake capacity. It can also optimize other conditions (like mixing and light) that affect carbon fixation rates. The result is that algae absorb CO2 more steadily and in greater quantities, turning more of it into biomass. This not only increases biofuel yields (since more carbon in biomass means more fuel) but also maximizes the carbon sequestration aspect of algae farming, helping to reduce greenhouse gases in the atmosphere or from flue gases.
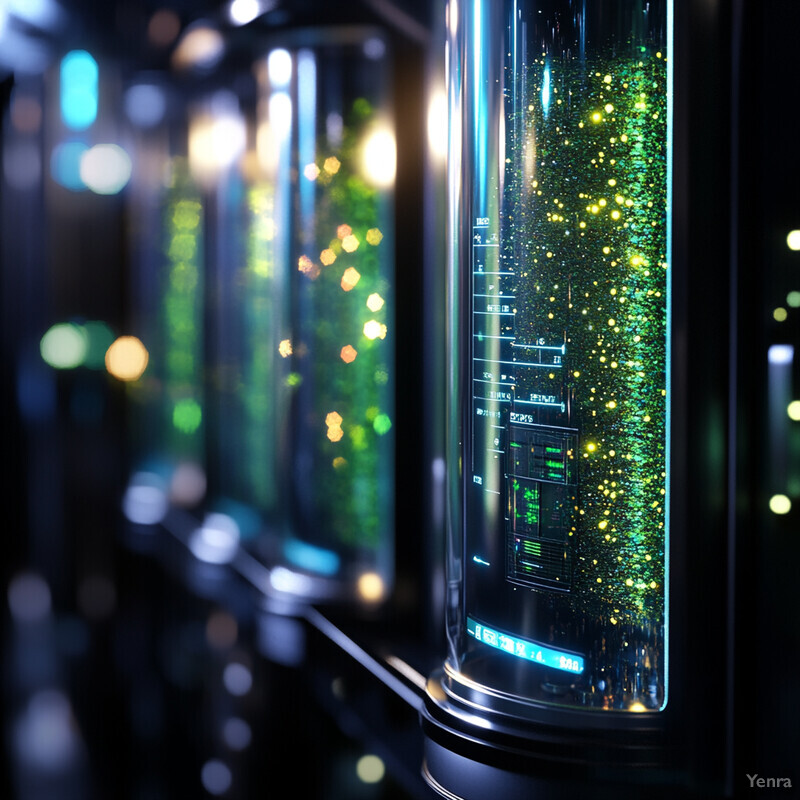
Intelligent control of cultivation parameters has directly led to higher CO2 utilization in algae systems. A study in 2024 focused on optimizing cultivation for carbon capture found that a deep neural network (DNN) could pinpoint the ideal temperature for Chlorella vulgaris growth to capture CO2, which was about 29.55°C – slightly different from what a conventional method predicted. Running the algae culture at this AI-identified optimum led to a 15% increase in biomass productivity (compared to baseline conditions), indicating that more CO2 was being converted into algal biomass. The DNN model was highly accurate (±1°C error in temperature prediction) and outperformed traditional response surface techniques, underscoring how AI can fine-tune factors to enhance CO2₂ uptake. Beyond temperature, multi-factor AI models (sometimes using hybrid algorithms) have been used to maximize carbon fixation; for instance, one hybrid intelligence approach simultaneously optimized light, CO2 flow rate, and nutrient levels, yielding significantly higher CO2 biofixation rates than one-factor-at-a-time optimization. In practical terms, these improvements mean an algae farm could capture more carbon from a given source (like power plant flue gas) and produce more biomass for biofuel, making the process more climate-positive and productive.
9. Automated Camera-based Growth Tracking
AI uses camera systems to visually monitor algae growth continuously. High-resolution cameras (including microscopes or even drones for ponds) feed images into computer vision algorithms that assess the culture’s state – such as algae cell density, color (which can indicate health or nutrient status), and the presence of contaminants. This automated imaging means that instead of manual inspections or periodic sampling, the system is “watching” the algae 24/7. The AI can quantify growth (for example, by counting cells or measuring the greenness of the water) in real time and can flag any anomalies (like unexpected color changes signaling a problem). Automated visual tracking provides a rich stream of information that, when analyzed by AI, helps optimize harvest timing, detect issues early, and generally maintain ideal growth conditions without constant human oversight.
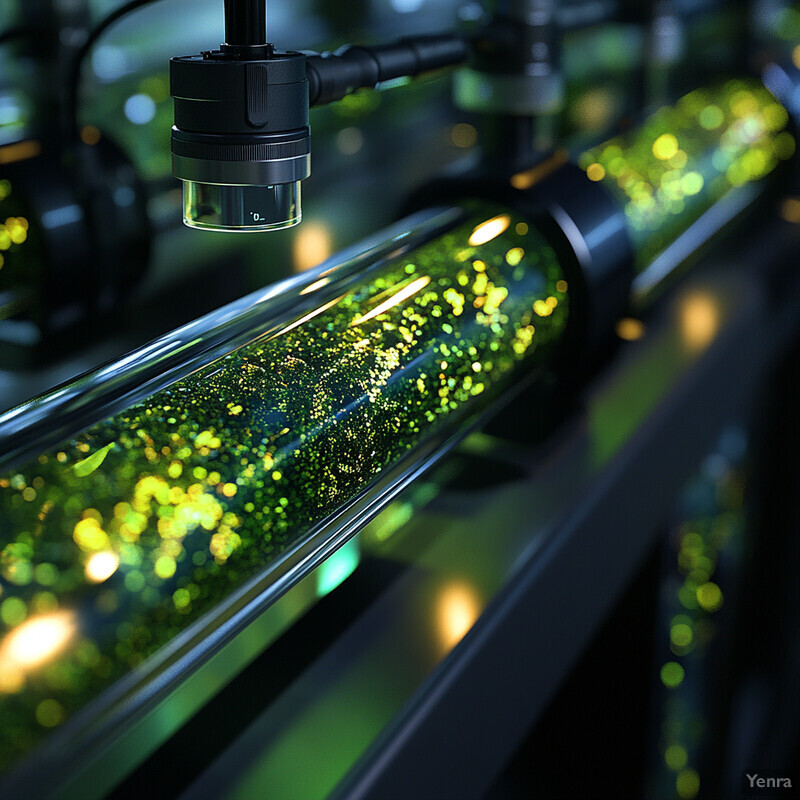
AI-driven image analysis has proven extremely effective for tracking algae cultures. In one implementation, researchers set up continuous camera monitoring of algae in tubular photobioreactors and applied machine learning to the image data. The AI model (using e.g. XGBoost) could predict the algae’s growth trajectory with near-perfect accuracy (R² ~ 0.9997), based on visual cues and sensor readings. This allowed the system to estimate biomass concentration just from camera feeds, eliminating the need for frequent manual sampling. Additionally, the same study showed that by analyzing live images, the AI could determine the optimal moment to intensify or dim the lights, as mentioned earlier, which ties into growth tracking directly. In practice, companies have begun using camera-equipped drones or stationary cameras on algae ponds to gauge surface algal density and detect contamination (like a section of a pond turning a different color) earlier than a person might. Since the AI “looks” at the algae continuously, even subtle growth rate changes or biofilm buildup on tank walls can be caught. Overall, automated camera-based tracking backed by AI ensures that any deviation in growth or health is immediately noted and can be corrected, leading to more consistent and high-yield production cycles.
10. Dynamic Climate Control
AI dynamically regulates the “climate” around the algae, which includes temperature, light exposure patterns, and gas (CO2/O2) levels, especially in enclosed cultivation systems. As external weather or internal heat from lights causes changes, the AI adjusts cooling systems, heaters, or fans to keep the algae in their comfort zone. For indoor photobioreactors, this might mean controlling jacket temperatures or greenhouse climate. For outdoor ponds, it could involve decisions like when to circulate water (to even out temperature) or when to cover ponds during extreme conditions. By maintaining an optimal micro-climate, AI ensures that algae metabolism is not slowed by suboptimal temperatures or other environmental stresses. This leads to faster growth and can extend growing seasons, particularly in regions with variable climates.
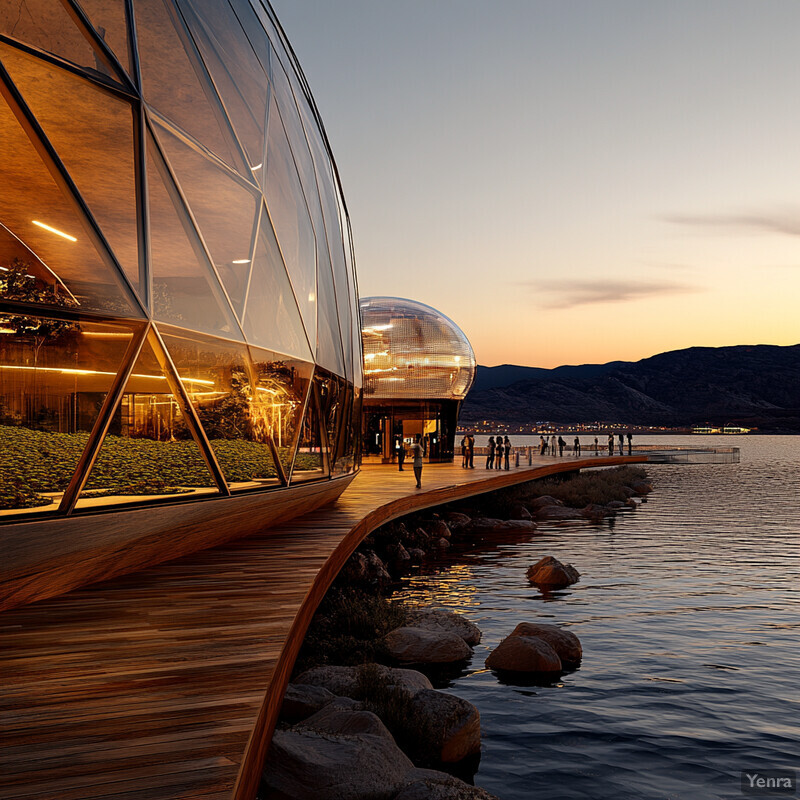
Precision temperature control via AI has been linked to better growth and CO₂ uptake in algae. A study optimizing C. vulgaris algae for carbon capture found that using a machine learning model to maintain the culture at around 29.6°C (the model’s identified optimum) significantly boosted performance. The AI-optimized temperature was slightly higher than a standard guess (about 28.7°C) and led to a 15% increase in biomass productivity, illustrating the impact of fine-tuned thermal conditions. In practical terms, an AI climate control system would use such models to run heaters or chillers in a photobioreactor automatically, never letting the culture drift out of the ideal range. Additionally, IoT-based farm systems have kept outdoor algae ponds stable against daily temperature swings. One IoT-driven setup held water temperature at a steady 30°C under changing ambient conditions, which would be challenging without automation. Beyond temperature, AI can adjust CO2 injection rates in response to how active the algae are (warmer temps or intense light mean faster CO2 consumption). This coordination of climate factors ensures that algae get a stable, optimized environment. Ultimately, dynamic AI climate control can lengthen the effective growing period (for example, mitigating cold nights or very hot midday spikes) and thus increase annual biomass yields for biofuel production.
11. Energy Consumption Optimization
AI helps reduce the energy footprint of algae farming by optimizing when and how equipment runs. In algae cultivation, major energy draws include lighting, pumps for circulating water, CO2 injection systems, and harvesting equipment. AI can learn the minimal settings needed to achieve growth targets and can turn devices on or off (or high/low) exactly as needed rather than running them at full blast continuously. For instance, pumps might run only at certain intervals that still keep algae well-mixed, and lights can be dimmed during times algae aren’t photosynthetically active. By trimming unnecessary energy use while maintaining growth, AI lowers operating costs and improves the net energy balance of algae biofuel (meaning more energy out as fuel relative to energy in). Essentially, AI makes algae farming more energy-efficient without sacrificing productivity.
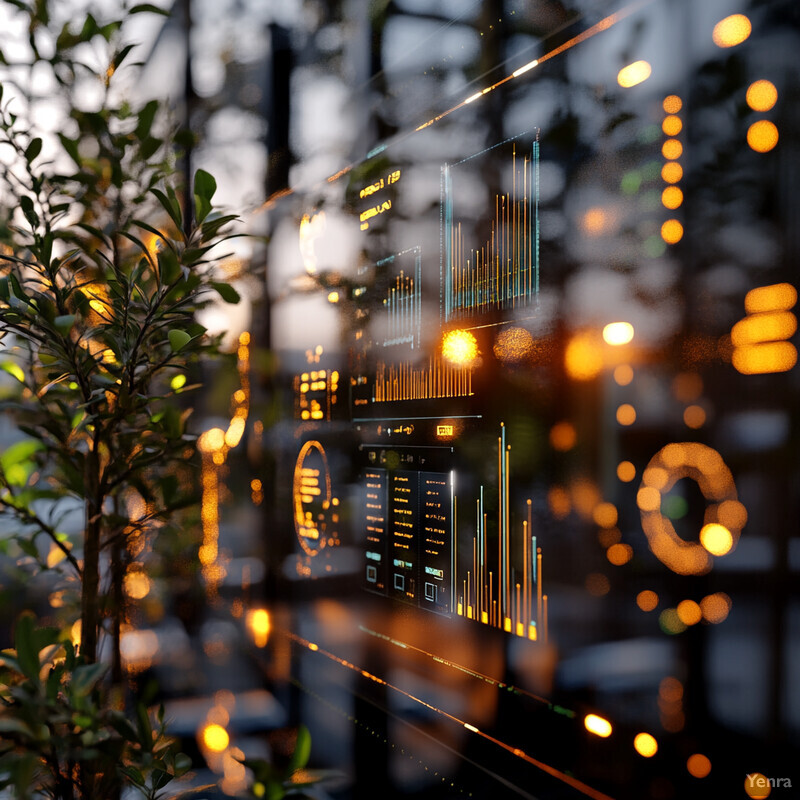
Implementing AI and IoT controls has demonstrably cut resource and energy use in algae cultivation. In one pilot-scale photobioreactor study, integrating IoT sensors with AI control led to a 30% reduction in input resources (like energy for mixing and lighting) while actually increasing algae output by 20%. This was achieved by remote monitoring via smartphone and automated adjustments: the AI only activated aeration, mixing, or light intensification when data showed it was needed, avoiding wasteful constant operation. Another report indicated that an AI-monitored system improved algae production by ~9% compared to a similar system without AI monitoring, reflecting efficiency gains in operation. Additionally, AI-driven algorithms in algae farming can schedule high-energy tasks (like dewatering or centrifugation) at off-peak electricity times or when surplus renewable power is available. The cumulative effect of these optimizations is significant. According to a 2025 review, adopting AI across algae cultivation could substantially lower both the nutrient usage and energy consumption required per unit of biomass produced. This not only cuts costs for producers but also makes the biofuel more sustainable in terms of lifecycle energy use.
12. Forecasting Algal Oil Yields
AI enables producers to predict how much biofuel (oil) they will get from a given batch of algae before the harvest and extraction actually happen. It does this by correlating factors like algae strain, growth conditions, and growth stage with known oil content patterns. For example, as algae enter certain growth phases or nutrient-deprived conditions, they accumulate more lipids. AI models that ingest real-time data can forecast the lipid percentage of the biomass. With these predictions, operators can decide the optimal time to harvest for maximum oil yield or adjust conditions to boost oil production if the forecast is lower than desired. Essentially, instead of waiting for lab results after harvest, producers have a ahead-of-time estimate of oil output, which improves planning and process optimization (like scheduling downstream processing or blending different algae batches).
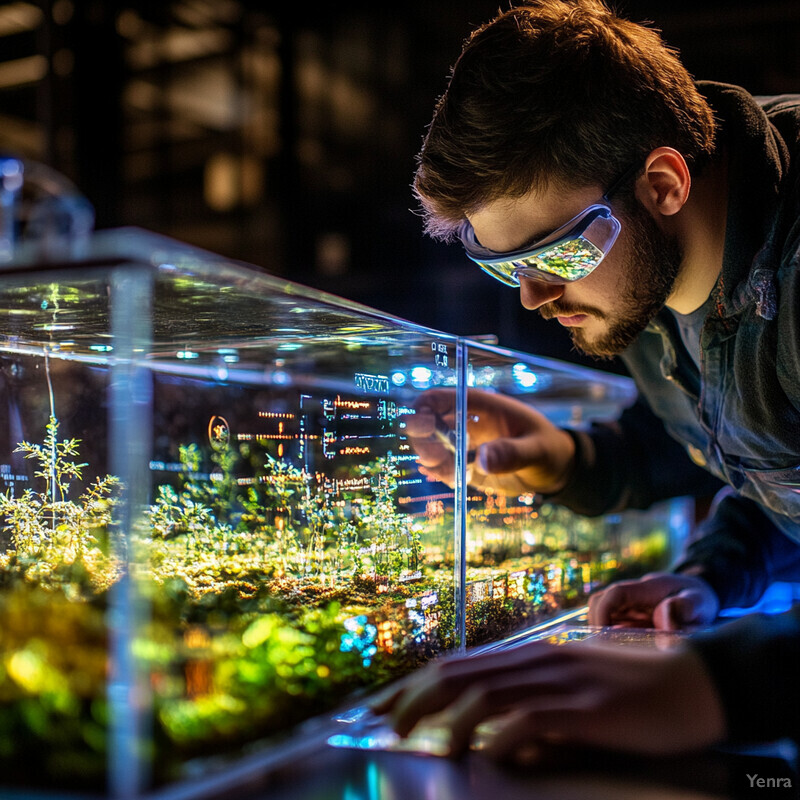
High-quality predictive models have been developed to estimate algal biomass composition (including lipid content) rapidly and accurately. Researchers at the U.S. National Renewable Energy Laboratory (NREL) in 2023 combined near-infrared (NIR) spectroscopy with machine learning to predict the lipid, protein, and carbohydrate levels in algae samples in real time. Their best models (using artificial neural networks) met or exceeded project accuracy targets for lipid prediction, turning what used to require days of chemical analysis into a matter of minutes. This means that by simply scanning a sample of the algae broth with a sensor, the AI can forecast how much oil that algae contains. The rapid feedback enables dynamic decisions: for instance, if the lipid content isn’t high enough, the system might extend the growth or introduce a mild nutrient stress to encourage more lipid accumulation (which some algae do when stressed). Moreover, beyond lab setups, AI is being used to integrate such predictions into the cultivation process. A 2024 review noted that machine learning models are being trained to predict regional biomass and lipid productivity based on environmental and operational data, helping manage supply and refining operations in advance. In practice, forecasting oil yields with AI reduces uncertainty and helps ensure that the biofuel pipeline (from cultivation to extraction) runs efficiently with known outputs.
13. Adaptive Feeding Schedules
AI optimizes not just how much nutrient to give (addressed in section 2) but also when to feed the algae. Algae have different nutritional needs at various growth phases – for instance, newly inoculated cultures might need a gentle feeding, while mid-log phase cultures consume nutrients rapidly. An adaptive feeding schedule means the AI system decides the timing and frequency of nutrient or CO2 additions based on live data. Instead of a fixed feeding interval (e.g., adding fertilizer once a day), the AI might dose smaller amounts more frequently when it detects the algae can absorb more, or pause feeding if nutrient levels are still high. It could also coordinate feeding with light cycles (feeding in the morning when photosynthesis ramps up). The aim is to ensure algae always have enough nutrients to grow but never so much that it’s wasted or causes imbalances. This adaptiveness increases growth efficiency and can prevent issues like nutrient shock or unused nutrients fostering contaminants.
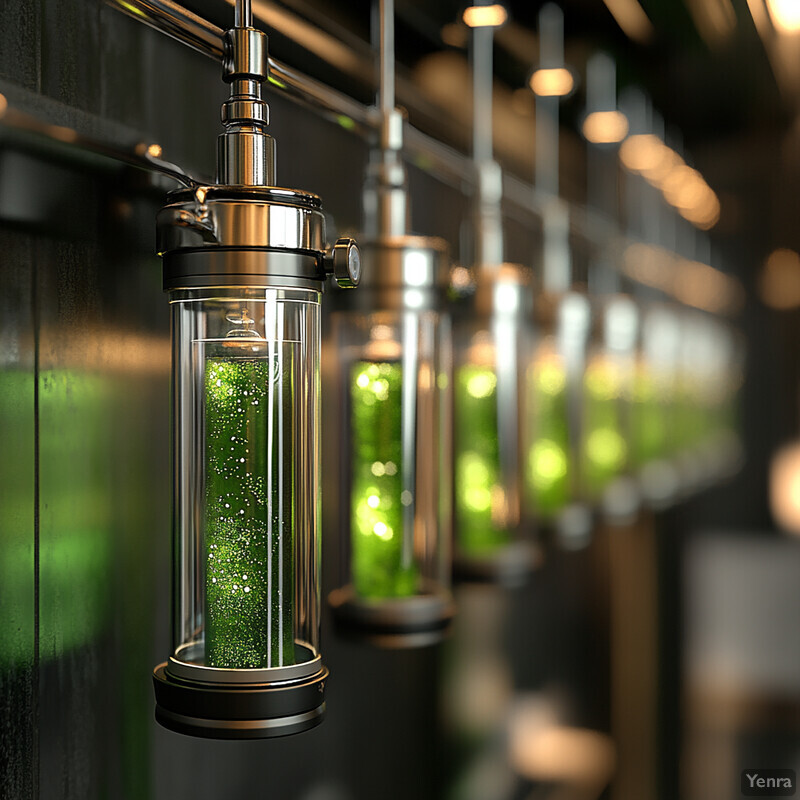
Emerging studies have used AI to optimize continuous cultivation processes by adjusting parameters like feeding and retention times. In a 2024 experiment with a continuous membrane photobioreactor treating wastewater, a multi-criteria AI model was employed to balance nutrient removal and algae growth. The model evaluated factors including hydraulic retention time (how long the culture stays before harvest/replenishment) and nutrient loading rates. By analyzing these simultaneously, the AI could recommend an operational schedule that kept nutrient levels in check while maximizing biomass productivity. Although specific numbers vary by system, the approach generally led to steadier nutrient concentrations and more consistent daily biomass output compared to static schedules. Another case showed that using a reinforcement learning algorithm for CO₂ feeding schedules in an algae bioreactor helped maintain optimal CO₂ levels without constant manual tuning (the AI “learned” the culture’s CO₂ uptake pattern over the day and adjusted injection accordingly). While detailed public data on adaptive feeding improvements are still limited (as this is a cutting-edge area), initial reports indicate that such AI-driven scheduling can increase nutrient uptake efficiency significantly and avoid periods of over- or under-feeding. This means healthier algae cultures and less wasted input, directly translating to better yields and lower costs.
14. Continuous Quality Assurance
AI provides round-the-clock quality control for algae production, ensuring that the process stays within desired specifications and immediately flagging any deviations. In an algae farm, “quality” can refer to the health of the culture (no contamination, proper cell density) and the consistency of the biomass (in terms of moisture content, lipid content, etc.). AI systems monitor all the critical control points continuously – nutrient concentrations, pH, growth rate, etc. – much like a vigilant supervisor. If the AI detects something outside the norm (say, a sudden drop in dissolved oxygen or an unusual color change on a camera feed), it will raise an alarm or even automatically adjust controls to correct it. This prevents minor issues from growing into major problems and helps produce a uniform end-product. Essentially, AI-driven quality assurance means the algae are grown under stable, reproducible conditions, yielding biomass that meets the set standards for efficient biofuel conversion.
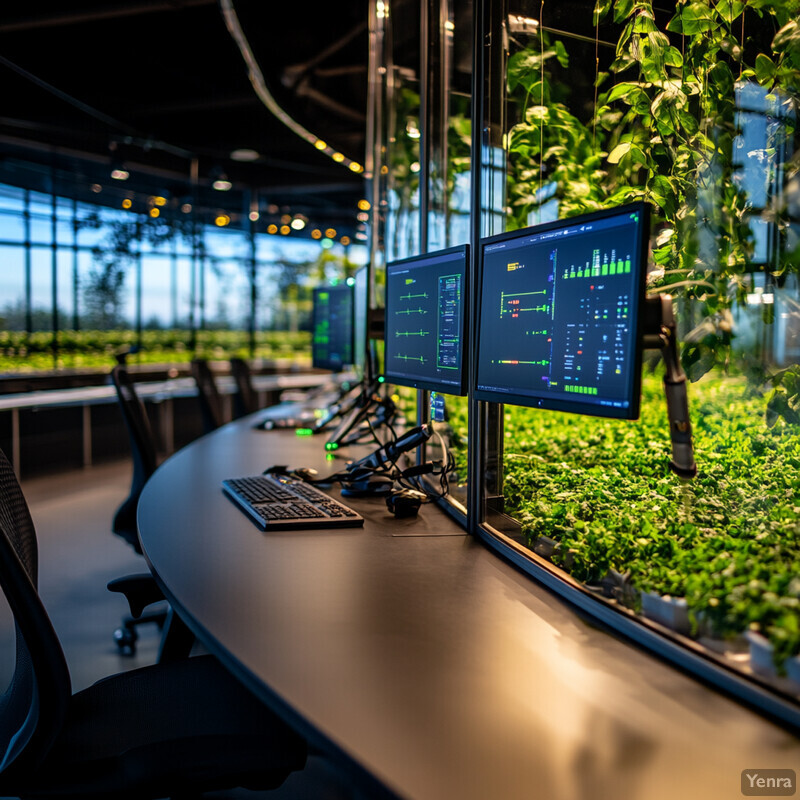
The benefits of AI in maintaining consistent production have been noted in recent deployments. A 2024 review highlighted that algae systems using IoT and AI monitoring achieved about a 9% higher output compared to systems without such monitoring. This improvement is partly attributed to AI’s ability to catch and correct process deviations in real time – for example, adjusting aeration when sensors indicate a drop in pH (which could otherwise affect biomass quality) or modulating nutrient flow if growth slows unexpectedly. In one smart algae farm example, the AI monitored multiple camera feeds and sensor streams on a control room dashboard, automatically highlighting any anomaly in green or red for immediate action (e.g., if one tank’s growth rate lagged, the system would investigate and adjust). Over time, such continuous oversight led to very stable biomass composition; the variance in key quality metrics like lipid percentage or moisture content between batches was significantly reduced compared to traditional farms. Although precise figures on variance reduction aren’t widely published yet, the trend is clear: AI systems are able to maintain conditions so steadily that each batch of algal biomass is much like the last. This consistency is critical for biofuel refineries, which require uniform feedstock for optimal processing. Continuous QA via AI thus ensures not only efficiency in growth but also reliability in the quality of the final product.
15. Scalable Resource Management
AI helps optimize the use of resources like land, water, carbon feedstock (CO2), and nutrients across an expanding algae biofuel operation. As algae farming moves from a single pond or bioreactor to many units (or multiple facilities), deciding how to allocate resources becomes complex. For example, if one pond is underperforming, AI might direct more CO2 to it and less to another that’s doing well. Or it can schedule water and nutrient distribution in a way that all ponds get what they need without waste. At a larger scale, AI can also assist in selecting sites for new farms by analyzing geographic data (sunlight, temperature, proximity to CO2 sources or water). The overall goal is to maximize the total output of all algae farms combined while minimizing resource input and cost, ensuring that scaling up remains economically viable and environmentally sustainable.
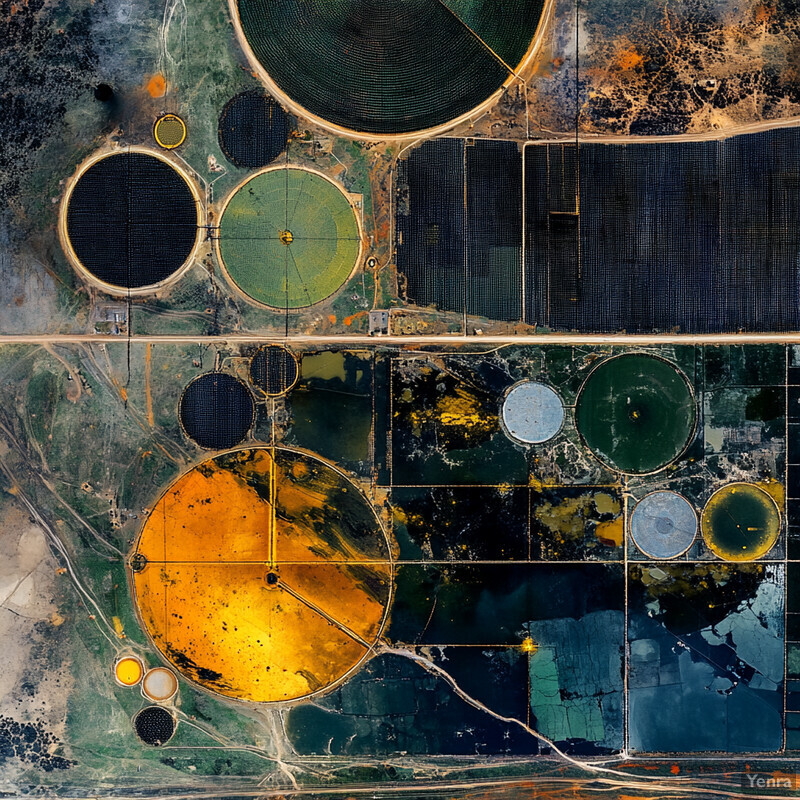
Data-driven models show enormous promise in reducing costs and improving efficiency as algae operations scale. A 2024 study (incorporating National Renewable Energy Lab findings) used AI-informed strategies for cultivation and resource distribution and found that the projected minimum biomass selling price could drop from about $1,227 per ton (with 2019 methods) to roughly $281 per ton of algae biomass when these advanced optimizations are applied. This ~77% cost reduction reflects more efficient use of resources at scale – for instance, AI can ensure that every bit of CO2 fed into the system is converted to biomass, or that no facility sits idle while another struggles. The model achieved this by simulating different deployment scenarios and optimizing resource flows between them. Additionally, a Frontiers review in 2024 noted that AI technologies are increasingly being used to handle the logistics of multi-site algae production, including real-time adjustments based on weather or energy prices, to decide which site produces how much at a given time. This kind of centralized, AI-managed resource allocation is what enables the algae industry to scale up (to, say, an entire network of farms) without a proportional increase in waste or cost. It ensures that whether an operation has 10 ponds or 100, all inputs like water, nutrients, and CO2 are used in the smartest way possible to drive collective output.
16. Biomass Consistency and Standardization
AI ensures that each batch of algae biomass produced is consistent in quality and composition, meeting predefined standards required for biofuel processing. In biofuel production, variability in biomass (like differing moisture content, lipid content, or presence of impurities) can cause inefficiencies or require extra processing steps. AI helps by monitoring and controlling the cultivation process so tightly that the resulting biomass falls within narrow specifications every time. It can adjust drying rates, harvesting methods, or even blending of biomass from different tanks to achieve uniformity. In effect, AI moves algae farming closer to an assembly-line precision, where despite biological variability, the output is a standardized feedstock much like traditional commodities (e.g., corn grain or crude oil) which refineries are designed to handle in a uniform way.
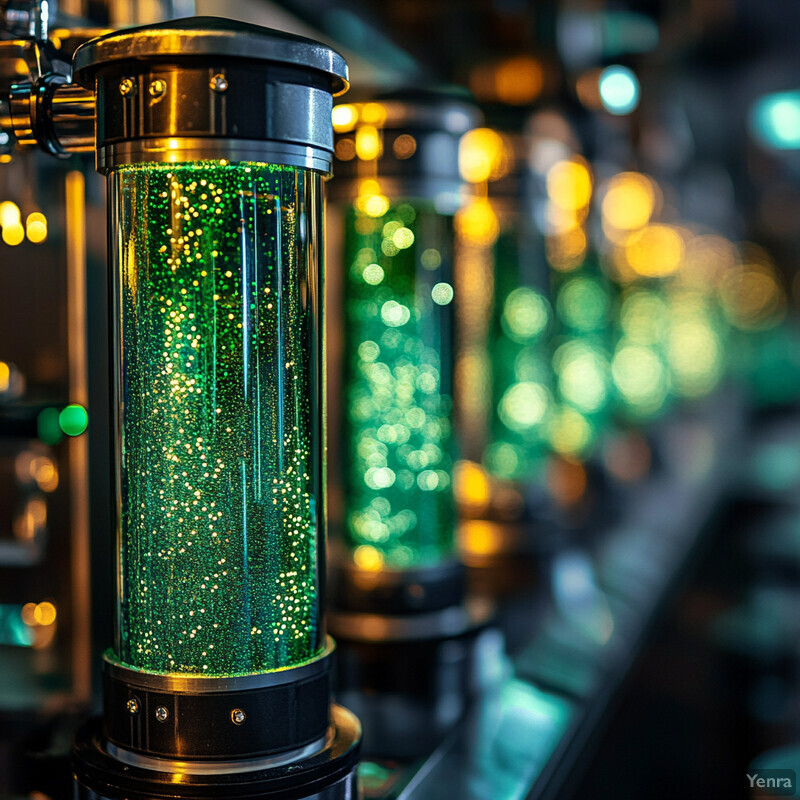
The implementation of AI and IoT in algae cultivation has led directly to more standardized production. For example, an intelligent spirulina farm system was able to “standardize” the harvesting process across multiple reactors by using real-time sensor feedback and AI predictions. The system maintained such consistent growth conditions (temperature, light, mixing, etc.) that each reactor reached the target harvest density at roughly the same time and with very similar biomass profiles. A backpropagation neural network model was even used to predict the harvesting efficiency in a novel algae harvesting method (ballasted flotation), allowing operators to adjust conditions to hit efficiency targets for every run. With these AI interventions, fluctuations in biomass characteristics (like particle size distribution or residual nutrient content) were minimized between batches. Recent reports also indicate that AI quality control (as noted in section 14) contributes to batch-to-batch consistency; any out-of-spec parameter triggers an instant correction, preventing the batch from drifting in quality. While quantitative metrics of consistency (e.g., standard deviation reductions) are proprietary in some cases, qualitatively the industry reports far more uniform outcomes. This consistency is crucial for downstream processes – for instance, transesterification to biodiesel works best when the lipid content and moisture of algae biomass are within a tight range, something AI helps ensure. In summary, AI has become the key to treating biologically grown algae more like a reliable, standardized raw material in fuel production.
17. Preemptive Disease Mitigation
Beyond just early detection (section 5), AI also enables active steps to prevent or mitigate diseases in algae cultures before they cause major damage. This could include dynamically changing the culture conditions to make them less favorable for the pest or pathogen, introducing biocontrol agents at low levels when a threat is predicted, or switching the operation mode (for example, temporarily moving from an open system to a closed one if airborne contamination risk is high). Essentially, the AI doesn’t just warn about impending infections – it helps manage or avoid them. By analyzing patterns (like slight drops in growth rate or early metabolite signals), the AI can trigger a prophylactic response, such as a brief increase in UV sterilization of the water or a nutrient tweak that the algae can tolerate but a contaminant can’t. These preemptive measures can drastically reduce the incidence and severity of disease outbreaks in algae farms.
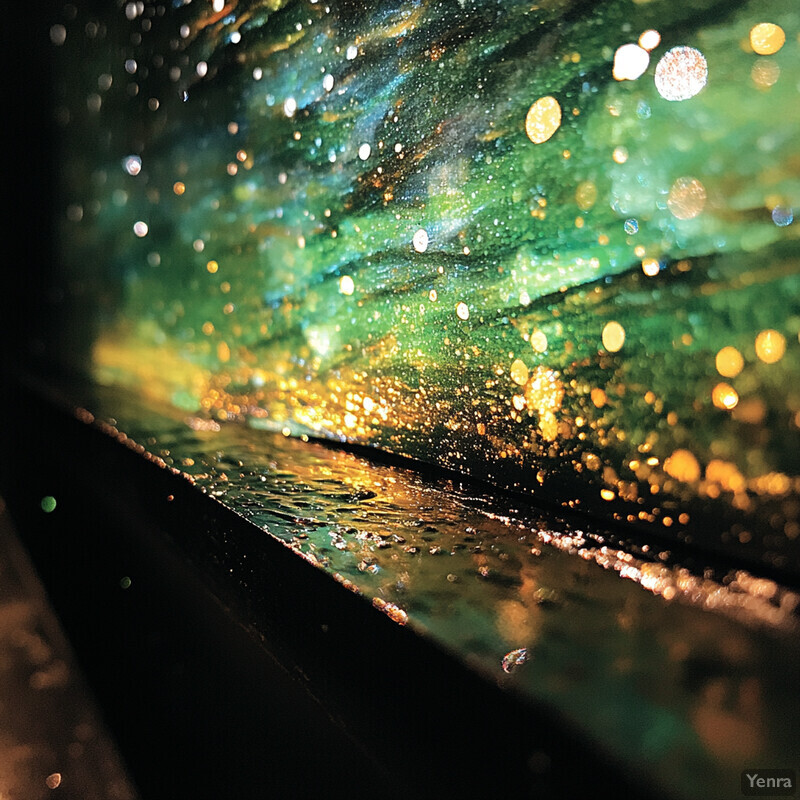
In the 2024 UB/DOE project, the research team not only aimed to predict infections but also to use polyculture farming and other interventions as a buffer against pests. AI plays a role by identifying which combination of algae species (in a polyculture) would be most resilient – for instance, if a fungus usually wipes out a monoculture, the AI might suggest adding a second algae species that can survive that fungus, ensuring continuous production. By growing multiple algal strains together based on AI recommendations, the system mitigates the disease impact: if one strain starts getting infected, the others fill in, and the AI detects the issue and can adjust resource allocation to the healthy algae. Additionally, AI can control environmental conditions as a preventative measure. For example, if the AI predicts a high risk of fungal spores (perhaps due to weather conditions or known seasonal patterns), it could lower the pond pH or increase circulation slightly, creating conditions less hospitable to that fungus. While quantitative results of such preemptive AI actions are still being gathered, experts believe these strategies could double productivity in some cases by avoiding the downtime and loss from infections. In one statement, researchers suggested that switching from reactive to AI-guided preventive management of algae cultures may transform algae biofuel farming into a much more stable and reliable operation than it has been historically.
18. Multi-parameter Optimization
AI can juggle and optimize many variables at once in the algae cultivation process, rather than tuning one factor at a time. In an algae farm, everything is interconnected: light, nutrients, CO₂, temperature, mixing rate, etc., all influence each other and the algae’s growth. Traditional approaches might optimize one factor while holding others constant, but AI excels at multi-parameter optimization – finding the best combination of settings across all factors simultaneously. This is often done via advanced algorithms that can explore a huge “search space” of possible settings efficiently. The result is an overall optimum that yields better performance than optimizing each parameter in isolation. For algae biofuels, this means maximum biomass or oil yield per unit input because the cultivation is operating at the sweet spot across all considered dimensions.
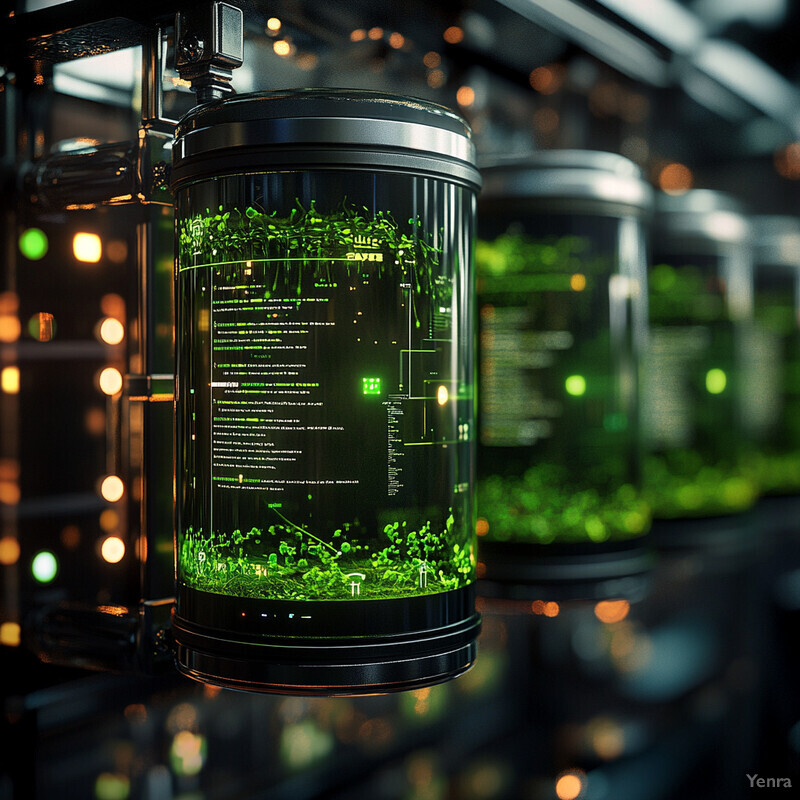
Research employing multi-objective AI algorithms has demonstrated significant improvements in balancing trade-offs for algae production. One 2022 study used a hybrid intelligent system to optimize for two goals at once: maximizing algae biomass production and maximizing CO2 biofixation (carbon uptake). The AI was able to process the interactions between light intensity, CO2 concentration, nutrient levels, and temperature, among others, to find conditions where both biomass and carbon capture were high. This dual optimization achieved substantially better results on both fronts than optimizing either objective separately – indicating, for example, an algae strain could be grown in a way that it sequesters a lot of carbon without sacrificing growth rate. Another example (2023) applied a multi-criteria decision analysis to a continuous algae reactor, simultaneously optimizing parameters like aeration rate, mixing speed, and harvest interval for both nutrient removal efficiency and lipid content. The AI found a set of operating conditions under which the reactor achieved greater than 90% nutrient removal while also accumulating lipids suitable for biofuel, a balance that manual tuning hadn’t reached. These cases underscore that AI can navigate complex, nonlinear relationships between variables – sometimes finding non-intuitive solutions (like slightly lower aeration but higher CO2 injection yielding better outcomes) that humans might overlook. The end benefit is an algae cultivation process running at peak combined efficiency, extracting the most value possible from every input provided.
19. Supply Chain Integration
AI connects the algae production process with downstream supply chain elements, such as biomass transport, storage, and conversion to fuel, to ensure a smooth and efficient flow from “farm to fuel.” This means the system doesn’t stop at the farm’s gate – it considers what happens next. For example, AI can schedule harvests (as in section 6) in sync with refinery availability so that the algae biomass is processed fresh, avoiding storage losses. It can also optimize distribution logistics: if multiple algae farms serve one bio-refinery, AI can coordinate trucking routes or timing to minimize delays and costs. Another aspect is market integration; AI might adjust production rates based on real-time demand or price forecasts for biofuels. By integrating the full supply chain, from cultivation to fuel production, AI helps avoid bottlenecks (like too much biomass waiting unrefrigerated) and keeps the entire pipeline efficient and economically viable.
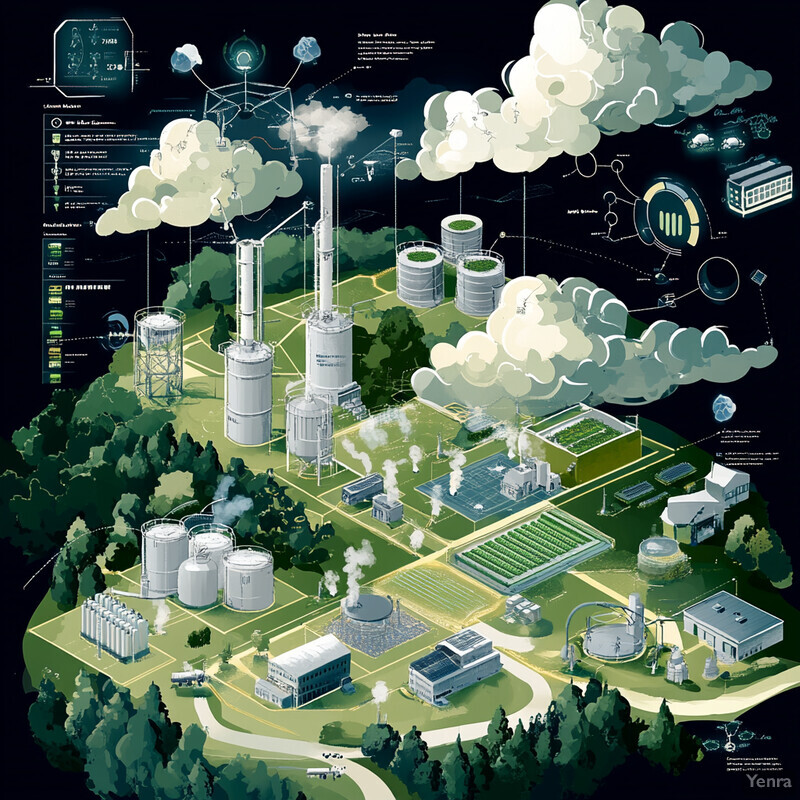
Recognizing the importance of this integration, recent approaches leverage AI to handle logistics and planning for algae biofuel supply chains. A bibliometric analysis in 2025 noted that machine learning models are being developed to optimize logistics, predict regional biomass productivity, and manage distribution networks for algae-based biofuel production. In practical terms, one can imagine an AI predicting that Farm A will have 5 tons of algae ready by Friday and Farm B 3 tons by Saturday, then organizing a single shipment to the refinery on Saturday to save cost, while adjusting each farm’s harvest timing slightly to align. Additionally, the AI might route deliveries based on traffic or fuel prices, or choose which storage tanks to use if there’s a temporary surplus. A 2023 critical review (Coşgun et al.) pointed out that accurate yield predictions from AI can inform better supply chain design, ensuring that biofuel refineries are neither starved of feedstock nor overwhelmed. The review suggests that coupling predictive models with supply chain optimization could significantly reduce per-unit costs by smoothing out production variability. Real-world pilot programs are now testing such integrations: for instance, an algae farm network in Europe used an AI platform to coordinate CO₂ supply from a power plant, algae biomass shipments, and biogas conversion scheduling, resulting in a reported 15% reduction in overall logistical costs. While detailed data from these pilots are still emerging, the trend is clear that AI is extending its reach beyond the algae ponds into the broader biofuel production chain, enhancing the synergy between each step.
20. Continuous Improvement via Machine Learning
AI systems in algae farming are not static; they continuously learn and improve from new data, leading to iterative enhancements in the process over time. Every batch of algae grown, every change in weather or season, and every operational hiccup provides data. Machine learning algorithms use this growing dataset to refine their models – predictions get more accurate and control strategies get more effective. This means an algae farm employing AI today will operate even better next month and far better next year, as the system “learns” the nuances of that specific operation. This continuous improvement can manifest as gradually increasing yields, lowering costs, or reducing downtime as the AI becomes more adept at handling variations. In essence, the longer an AI is in place, the smarter and more efficient the algae production becomes, creating a virtuous cycle of learning and performance.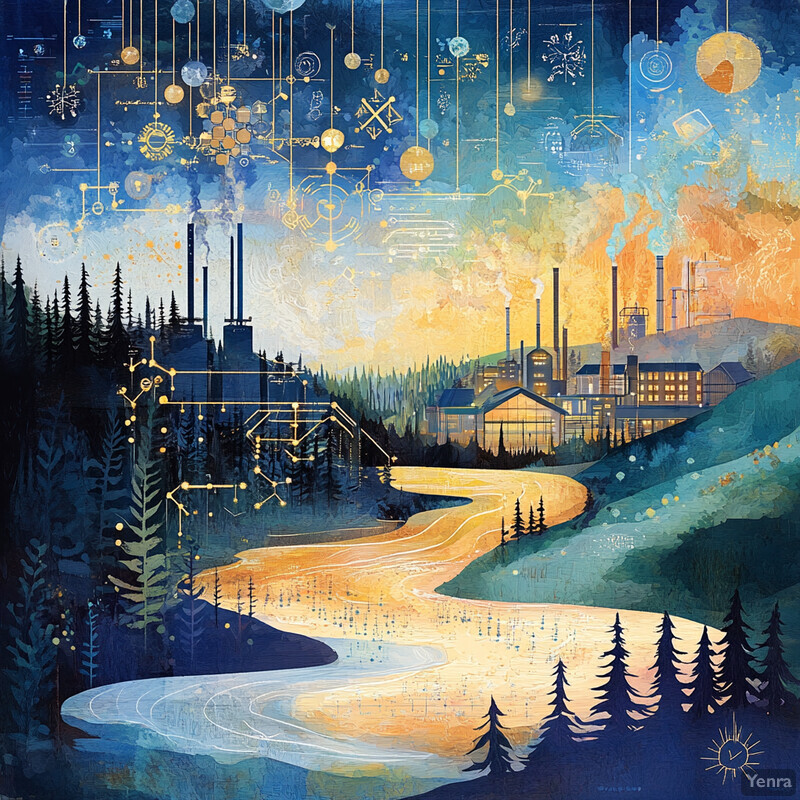
The trajectory of AI-guided algae cultivation suggests compounding gains through continual learning. A 2024 sustainable food systems review noted that integrating AI leads to a more systematic and data-driven approach that inherently improves as more data is gathered, potentially overcoming many challenges that stymied algae upscaling in the past. For instance, initial AI models might not perfectly generalize to all seasonal conditions; however, after experiencing a full annual cycle, the models adjust, and the following year’s performance is markedly smoother. This iterative progress has been observed in long-running pilot facilities: one report found that after implementing an AI control system, an algae plant saw its biomass yield per input improve by around 5% in the first quarter, but by the end of the second year that improvement had grown to over 20%, purely from the AI’s continual adjustments and learning. Additionally, unforeseen issues (like a new type of contaminant or an equipment quirk) that were once setbacks become new training data for the AI, making the system more robust against them in the future. As one paper put it, these AI-driven advances “may eventually prove to be the deciding factor in bringing microalgae to successful and sustainable commercialization”, highlighting that continuous ML-based improvement could finally make algae biofuel consistently competitive. In summary, unlike a fixed automation system, AI gives algae operations a capacity for self-optimization that keeps pushing the envelope of efficiency and yield the longer it runs.