1. Predictive Traffic Flow Management
AI-driven analytics can forecast future air traffic volumes and patterns, enabling controllers to anticipate congestion and adjust flight paths or schedules proactively.
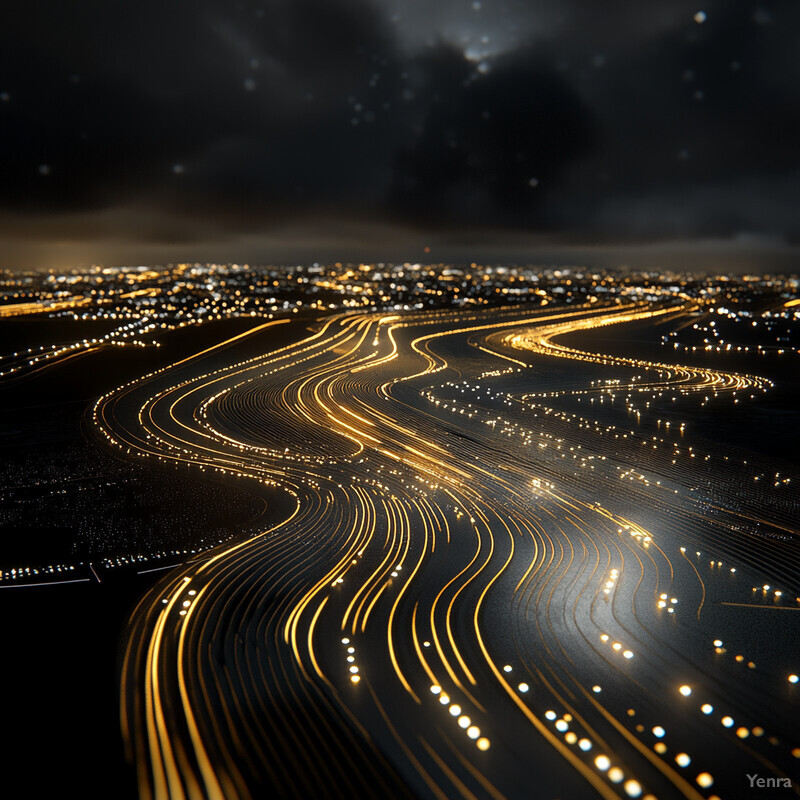
Air traffic demand can be notoriously difficult to predict due to fluctuating airline schedules, variable weather patterns, and unexpected events. AI-driven analytics leverage historical flight data, current airspace usage, seasonal patterns, and meteorological inputs to forecast short- and long-term traffic volumes with greater accuracy than traditional models. By anticipating where and when congestion is likely to occur, air traffic controllers and flow managers can take proactive measures such as adjusting route assignments, sequencing takeoffs and landings more strategically, or temporarily restricting certain sectors. This improved foresight helps ensure that flights operate more smoothly, reducing delays and enhancing the overall efficiency of the air transportation system.
2. Dynamic Airspace Configuration
Machine learning algorithms can assess current conditions, such as weather and traffic density, to dynamically reconfigure airspace sectors, reducing controller workload and improving efficiency.
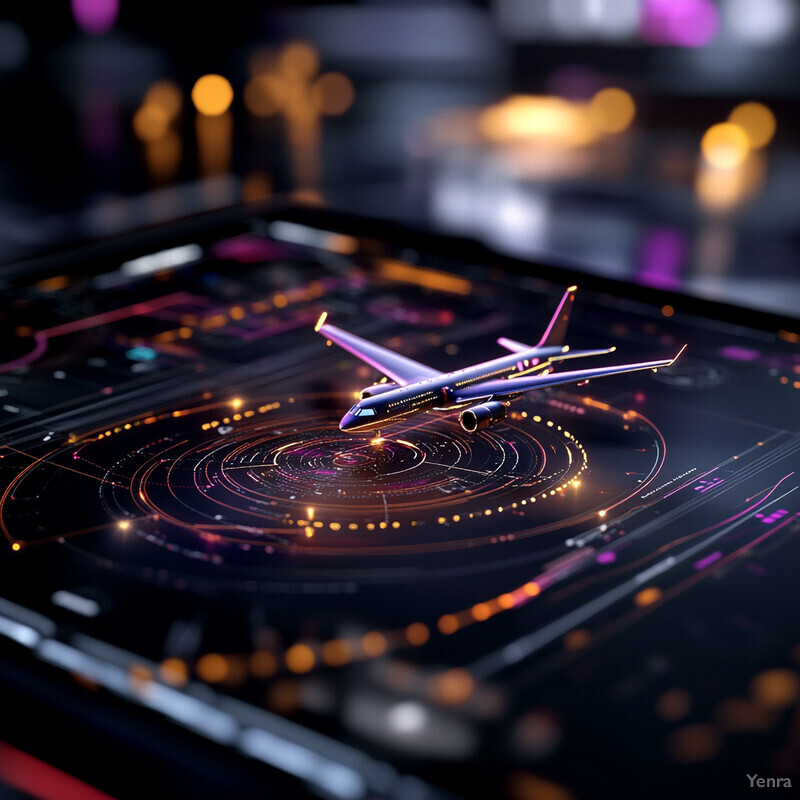
Historically, airspace sectors are relatively fixed, each managed by specific control teams and divided according to predefined geographical boundaries. AI now enables flexible, dynamic segmentation of these sectors based on real-time conditions. Machine learning algorithms factor in prevailing weather, current traffic density, complexity levels, and controller workload to reconfigure airspace boundaries as needed. This agility allows traffic managers to balance demand more effectively, assigning additional resources where congestion is intensifying and reducing fragmentation where traffic is light. As a result, controllers can handle traffic more fluidly, minimizing bottlenecks, improving safety margins, and enhancing overall operational capacity.
3. Automated Conflict Detection and Resolution
Advanced AI tools can rapidly identify potential aircraft conflicts and propose deconfliction maneuvers, increasing safety while minimizing the need for manual intervention.
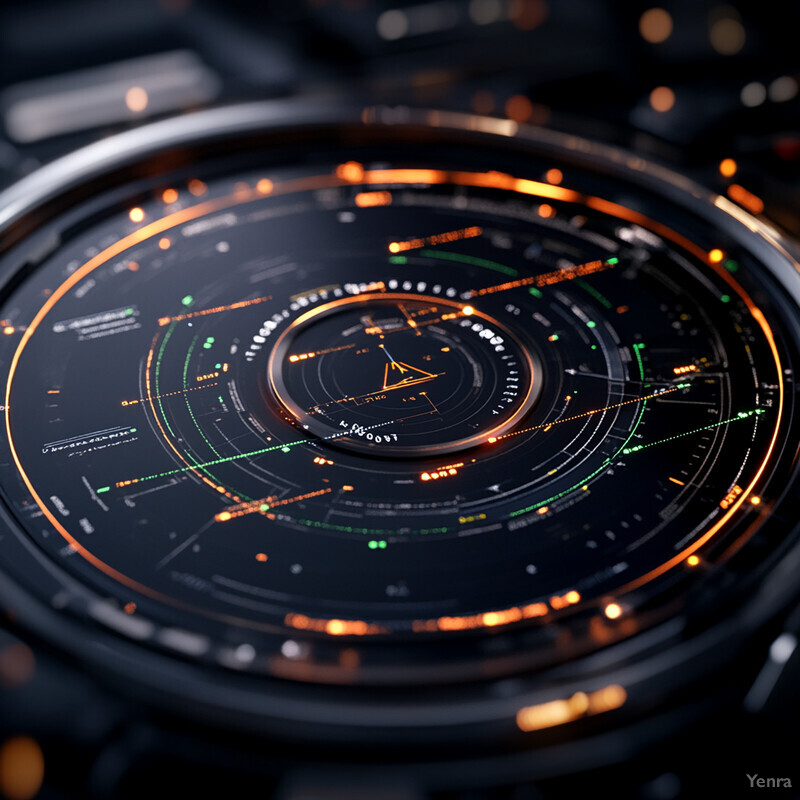
One of the most critical tasks in air traffic control is ensuring that aircraft maintain safe separation distances and do not come into conflict. AI-powered tools, built on advanced collision avoidance algorithms, process massive quantities of radar data and flight trajectories in real-time. These systems instantly identify potential convergence points between aircraft and recommend subtle heading, speed, or altitude adjustments to prevent conflicts long before controllers might have spotted them manually. This automation not only eases the pressure on human controllers but also significantly improves safety and reduces the risk of human error. Moreover, by suggesting minimal route deviations, these solutions help maintain optimal efficiency and minimize fuel consumption.
4. Optimized Arrival and Departure Sequencing
AI-powered scheduling tools consider multiple variables—weather, aircraft performance, runway capacity—to produce optimized arrival and departure sequences, reducing delays and runway occupancy times.
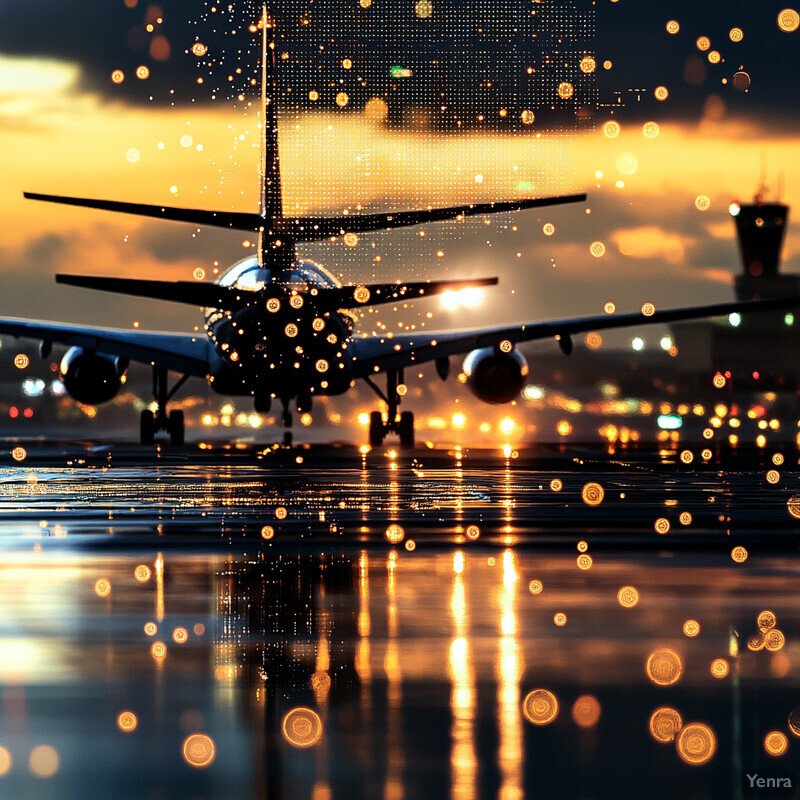
The sequence in which aircraft arrive and depart at busy airports can greatly influence delays, gate usage, and terminal congestion. AI-driven scheduling engines consider a multitude of factors, including weather forecasts, runway capacity, wake turbulence separation requirements, and individual aircraft performance profiles. By crunching these variables simultaneously, AI can generate an optimal arrival and departure schedule that streamlines runway usage. Pilots experience reduced holding times in the air, while passengers enjoy shorter overall travel times and fewer delays. Ground operations can also run more smoothly, as the airport’s ecosystem adapts to more predictable flows of arriving and departing aircraft.
5. Surface Movement Optimization
Intelligent algorithms can manage ground operations, guiding aircraft to optimal taxi routes and preventing bottlenecks at terminals, gates, and taxiways.
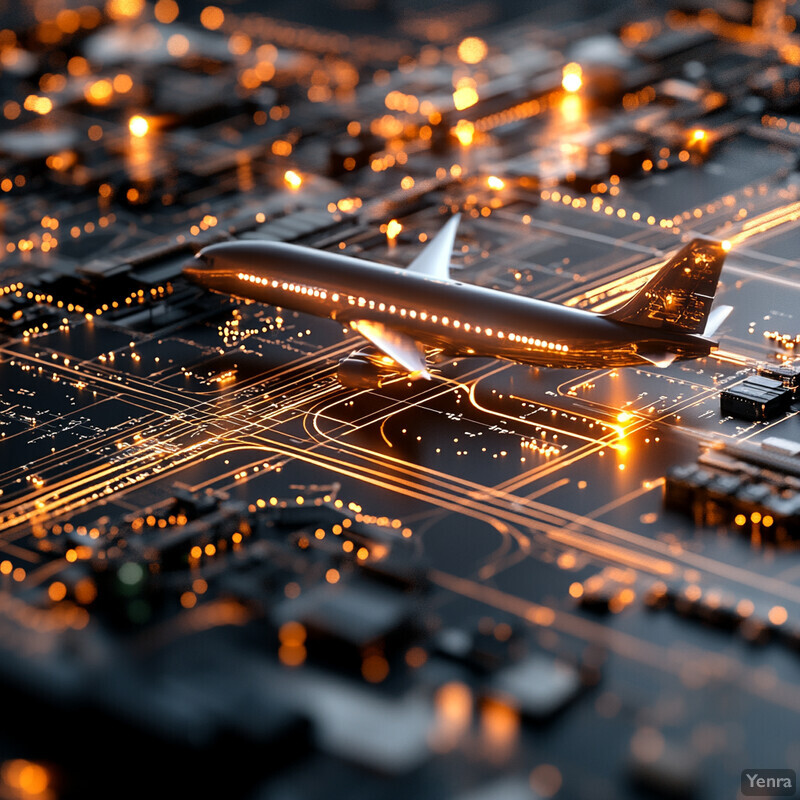
On the ground, traffic jams on taxiways, apron congestion, and inefficient gate-to-runway routing can cause considerable delays and increase fuel burn. AI algorithms that optimize surface movement process inputs such as gate assignments, taxiway closures, pushback timings, and runway availability. By determining the most efficient taxi routes for each aircraft and scheduling movements to prevent standstills, these systems minimize taxi times and reduce the environmental footprint. This seamless integration between air and ground operations ensures that flights spend more time in the air and less time idling, leading to more punctual operations and improved passenger satisfaction.
6. Enhanced Weather Forecast Integration
Machine learning models can incorporate micro-forecasting of turbulence, thunderstorms, and wind patterns into traffic planning, improving both safety and operational efficiency.
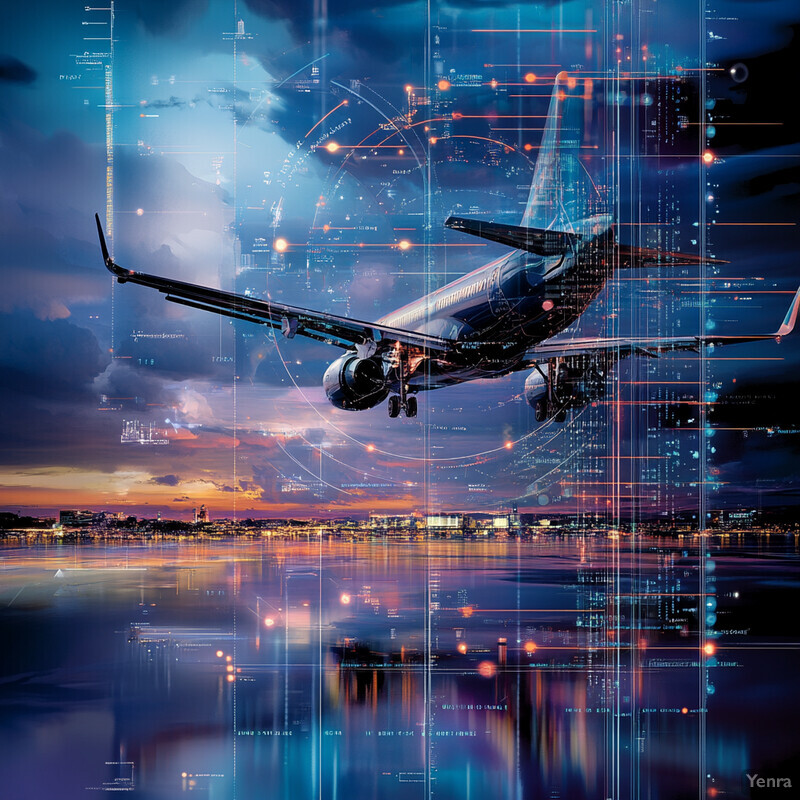
Weather is one of the biggest uncertainties in air traffic management. With AI-driven micro-forecasting, air traffic controllers can now receive highly localized and accurate weather predictions that span minute-to-minute changes. Machine learning models use historical weather data combined with current atmospheric readings and satellite imagery to pinpoint where turbulence, thunderstorms, or strong headwinds are likely to occur. Armed with this information, controllers and dispatchers can reroute flights to avoid hazards, strategically select optimal cruising altitudes, and anticipate when runways may be closed or opened. This leads to safer and more fuel-efficient operations, as aircraft can be guided around problematic areas well in advance.
7. Trajectory-Based Operations (TBO) Support
AI can facilitate precise four-dimensional trajectory management, allowing aircraft to follow optimized routes that minimize fuel burn and emissions while ensuring punctual operations.
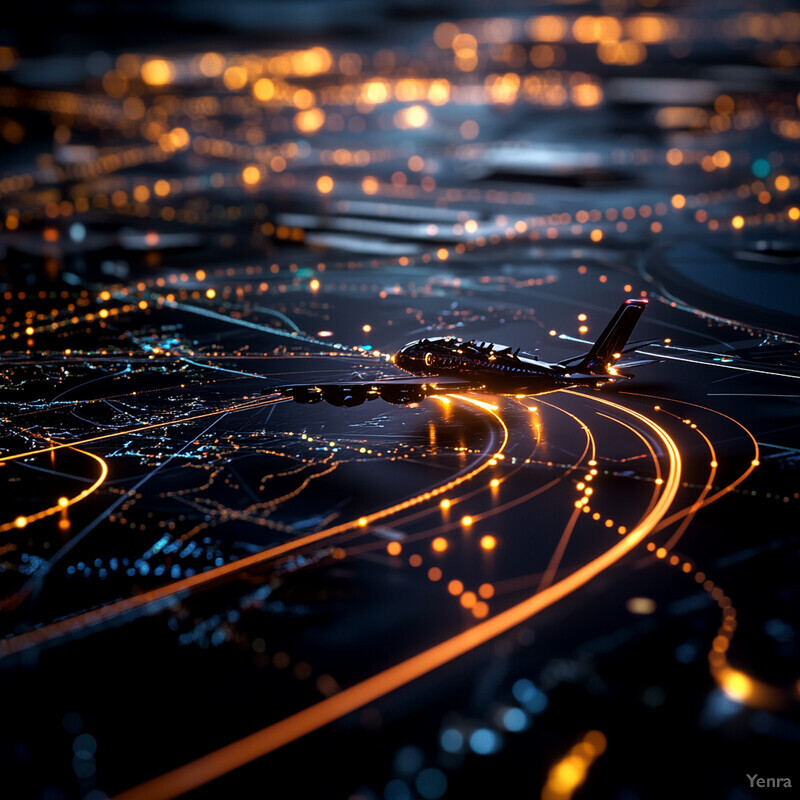
In trajectory-based operations, each aircraft follows a four-dimensional path (latitude, longitude, altitude, and time) that is carefully planned to optimize efficiency and punctuality. AI enhances TBO by predicting the best possible trajectory for each flight, considering factors like airspace constraints, aircraft performance, air traffic density, and weather. Machine learning models iterate through thousands of scenarios to pinpoint the route that yields minimal fuel consumption and emissions, all while adhering to safety and capacity requirements. The result is a finely tuned flight plan that reduces the overall environmental impact, improves on-time performance, and makes the entire system more predictable.
8. Adaptive Sector Complexity Management
AI systems can predict when a sector may become overly complex due to increased traffic or weather changes and proactively redistribute workloads or suggest sector boundary adjustments.
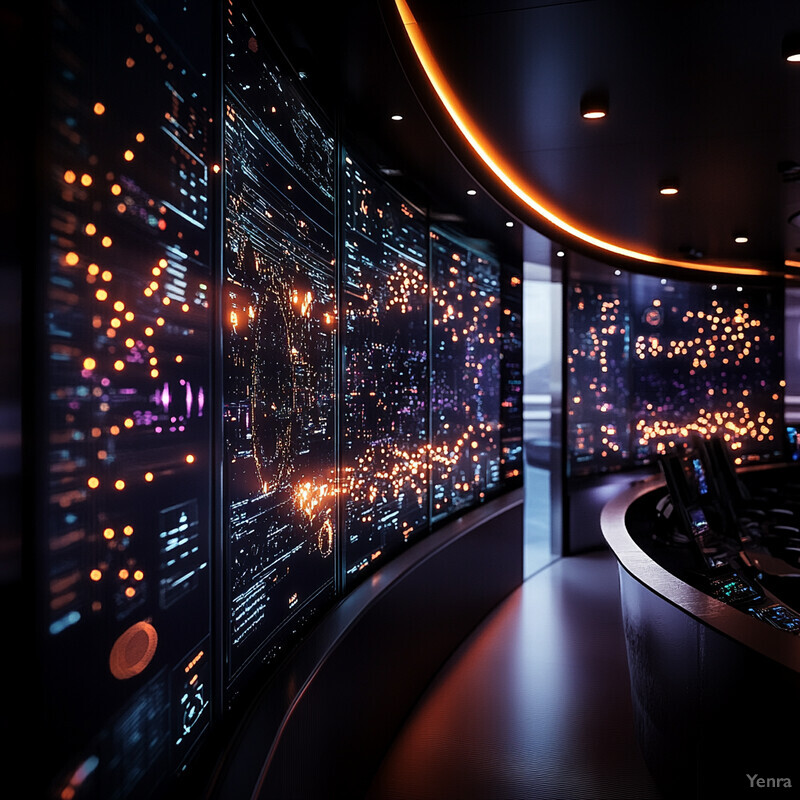
Not all airspace sectors are created equal—some regions can quickly become more complex due to increased traffic density, weather changes, or military exercises. AI tools continuously monitor air traffic patterns and apply complexity metrics to identify when a sector’s cognitive load on controllers might become unsustainable. If a sector is approaching overload, the system can recommend redistributing traffic among adjacent sectors, adjusting routes slightly, or temporarily assigning additional control resources. By making these adjustments before controllers reach their workload limits, the system preserves safety margins, reduces fatigue-induced errors, and keeps traffic flowing smoothly.
9. Controller Decision Support Tools
AI-driven decision-support systems can provide real-time recommendations to air traffic controllers, allowing them to focus on strategic oversight instead of routine manual tasks.
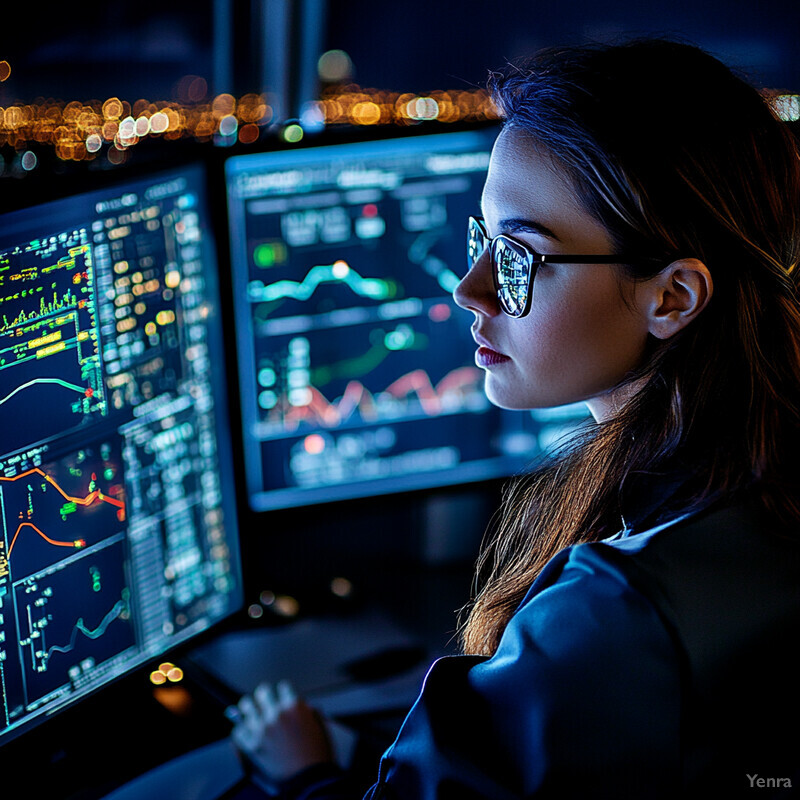
Air traffic controllers already manage highly intricate tasks, and adding new technologies should lighten their workload rather than complicate it. AI-driven decision support systems serve as a trusted assistant, analyzing massive datasets in real-time to provide actionable insights. For instance, these tools can highlight the top few conflict-resolution options or suggest optimal headings and speeds for incoming aircraft. By offloading routine or data-intensive tasks, controllers can dedicate more attention to strategic decision-making, unusual scenarios, and maintaining a high level of safety. Ultimately, the human remains at the center, guided by intelligent software that enhances situational awareness and operational efficiency.
10. Speech Recognition and Natural Language Processing
Advanced language models can transcribe and interpret pilot-controller communications in real-time, reducing misunderstanding, workload, and response times.
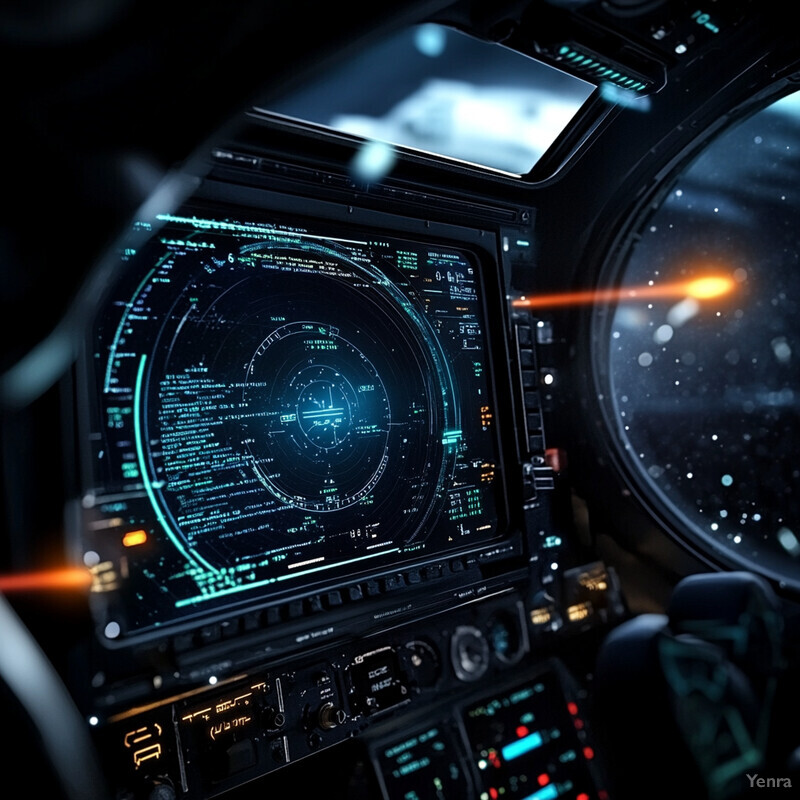
Radio communication between pilots and controllers is rapid, specialized, and prone to misunderstandings or mishearings. Advanced AI models trained in speech recognition and domain-specific aviation language can transcribe and interpret these communications in real-time. They can verify that read-backs from pilots match the instructions given, flag potential misunderstandings immediately, and even translate between different languages or accents more reliably. This reduces the cognitive burden on controllers, ensures that critical instructions are followed correctly, and can prevent minor misunderstandings from escalating into safety incidents.
11. Noise and Emission Reduction
By analyzing historical data and current conditions, AI can suggest routings and altitude adjustments that minimize noise footprints over populated areas and reduce fuel consumption and emissions.
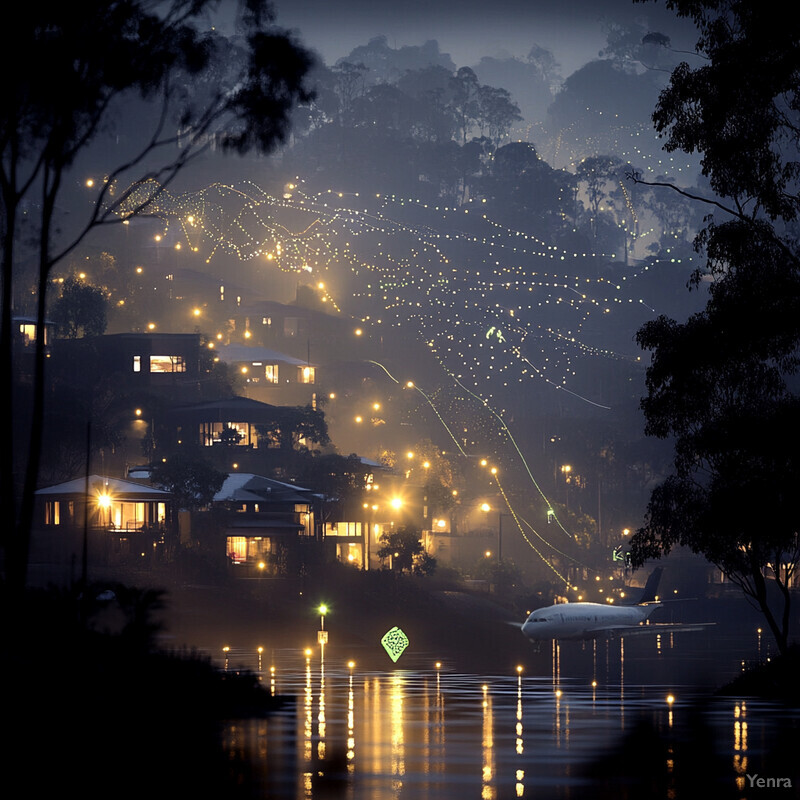
Communities around airports and along heavily trafficked flight corridors are increasingly concerned about noise pollution and environmental impact. AI solutions can help minimize these concerns by suggesting alternative flight paths or altitudes that reduce noise footprints. Concurrently, machine learning algorithms that factor in aircraft efficiency and atmospheric conditions can guide routes that reduce fuel consumption and decrease CO2 emissions. Over time, these incremental changes foster better relationships with local communities, adhere to environmental regulations, and support the aviation industry’s long-term sustainability goals.
12. Predictive Maintenance Scheduling Integration
AI can forecast when navigational aids or radar systems will need maintenance. Adjusting traffic flows to accommodate maintenance windows ensures minimal disruption.
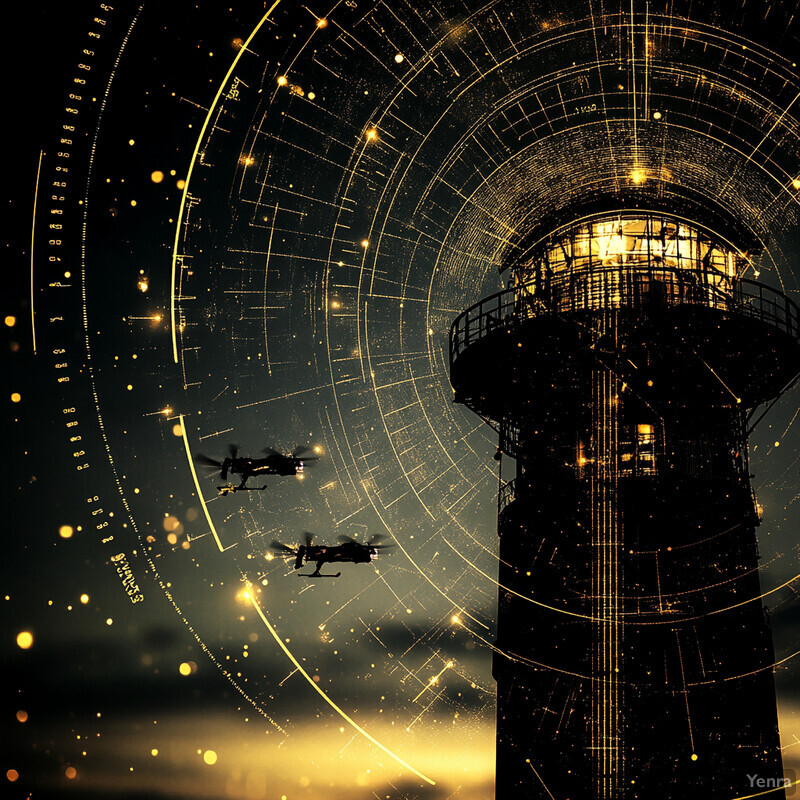
Air traffic optimization doesn’t only concern the moving aircraft—navigational aids, communication systems, radars, and other infrastructure components must remain reliable. AI-driven predictive maintenance models analyze historical performance data and sensor readings to predict when equipment may fail or require servicing. By forecasting maintenance windows, air traffic managers can preemptively reroute traffic to maintain safety without causing unexpected disruptions. This leads to fewer abrupt system outages, more predictable airspace availability, and sustained operational integrity of critical systems.
13. Safety Incident Prediction and Prevention
Machine learning can analyze historical safety reports, identifying patterns that might lead to incidents. Controllers can then be alerted to take preventive measures before issues arise.
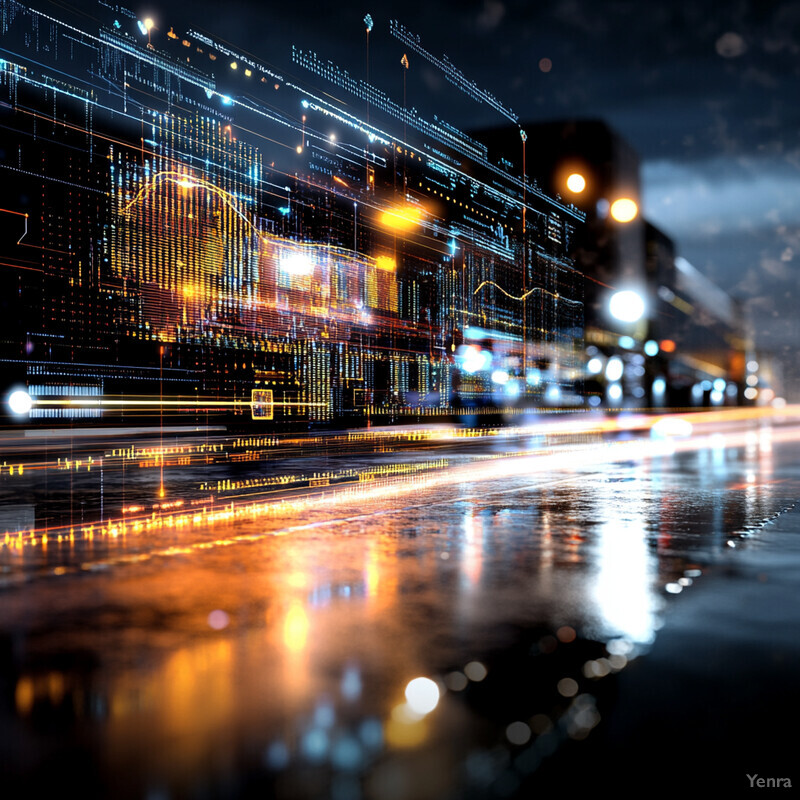
Safety stands at the forefront of aviation priorities. AI can identify subtle trends and patterns in historical safety reports, near-miss incidents, and maintenance logs to predict where future issues may arise. Perhaps certain runway configurations correlate with miscommunications, or a particular type of weather condition frequently precedes navigation errors. By uncovering these latent relationships, AI alerts controllers and safety managers to potential trouble spots before they materialize. Taking early action—through adjusted procedures, additional training, or updated protocols—helps prevent incidents and maintains the impeccable safety record demanded in the aviation sector.
14. Unmanned Aerial Vehicles (UAV) Integration
AI helps incorporate drones and UAVs into controlled airspace by predicting their movements, preventing conflicts, and designing procedures for mixed-traffic environments.
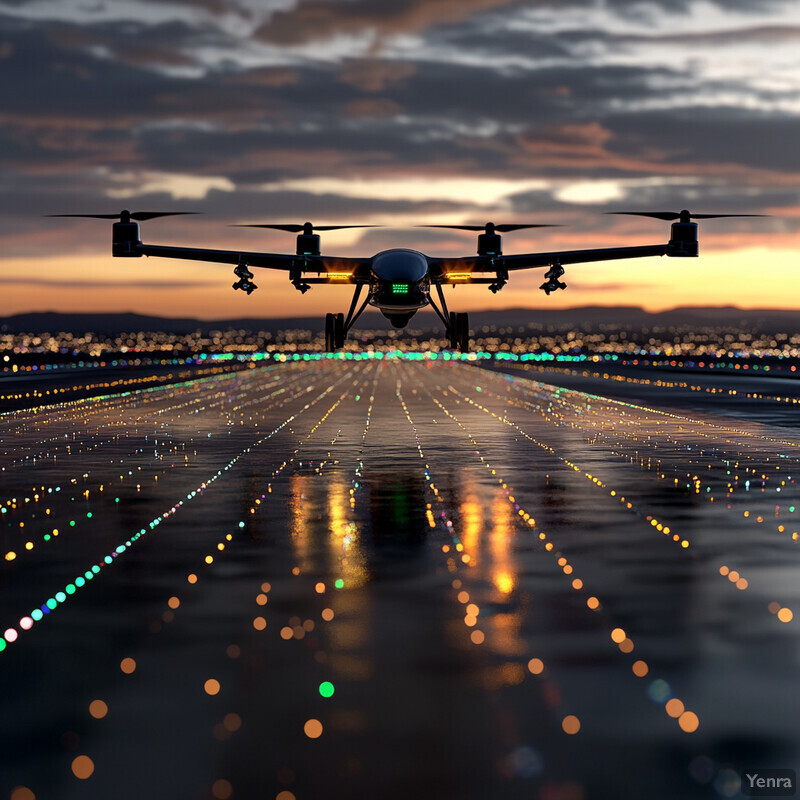
As drones and other UAVs begin to share the sky with traditional aircraft, ensuring they coexist safely and efficiently poses new challenges. AI-driven platforms can track UAV trajectories, predict their future positions, and integrate them into controlled airspace by aligning them with standard traffic flows. By continuously adjusting UAV routing and using autonomous conflict resolution, the system ensures that drones do not interfere with commercial or private manned flights. This careful harmonization paves the way for a future where air cargo delivery drones, air taxis, and other novel aerial vehicles operate smoothly alongside traditional aviation.
15. Real-time Capacity Assessment
By continuously evaluating airspace utilization and runway occupancy in real-time, AI can inform controllers of capacity constraints and optimal management strategies.
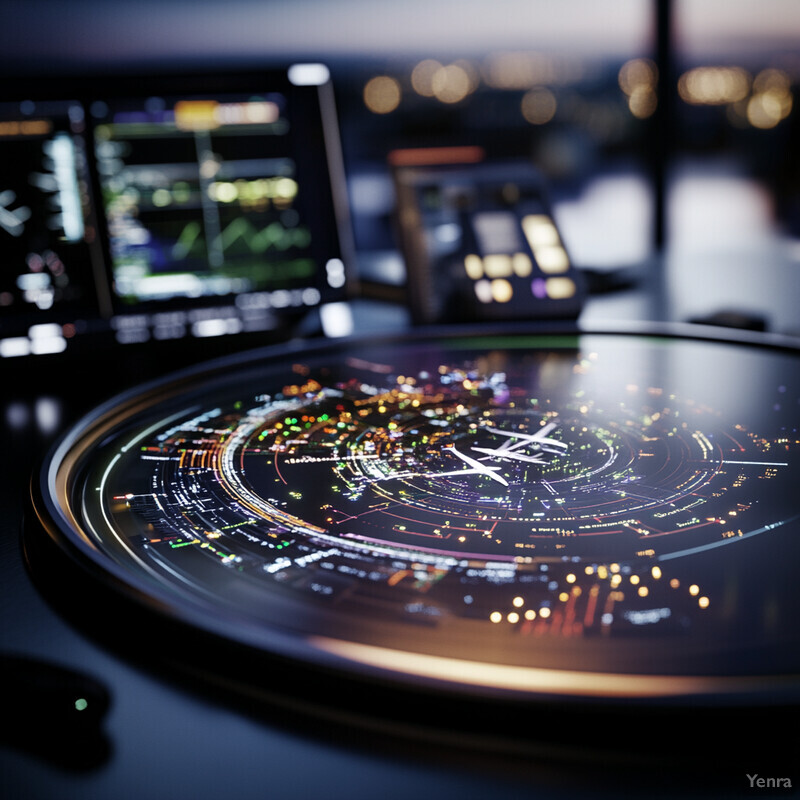
Airspace capacity and runway throughput are dynamic variables influenced by factors like weather fronts, controller workload, and equipment availability. AI systems can run continuous assessments of real-time conditions, providing air traffic managers with ongoing, data-driven insights into how many aircraft the system can safely and efficiently handle. By displaying early warnings when nearing capacity limits, AI tools allow managers to implement measures to mitigate overload—such as metering arrivals, adjusting departure release rates, or offering alternative routes. The result is more consistent and reliable service levels, even under changing conditions.
16. Automation of Routine Tasks
AI systems can handle routine clearances, data entry, and monitoring tasks, allowing human controllers to focus on decision-making and anomaly handling.
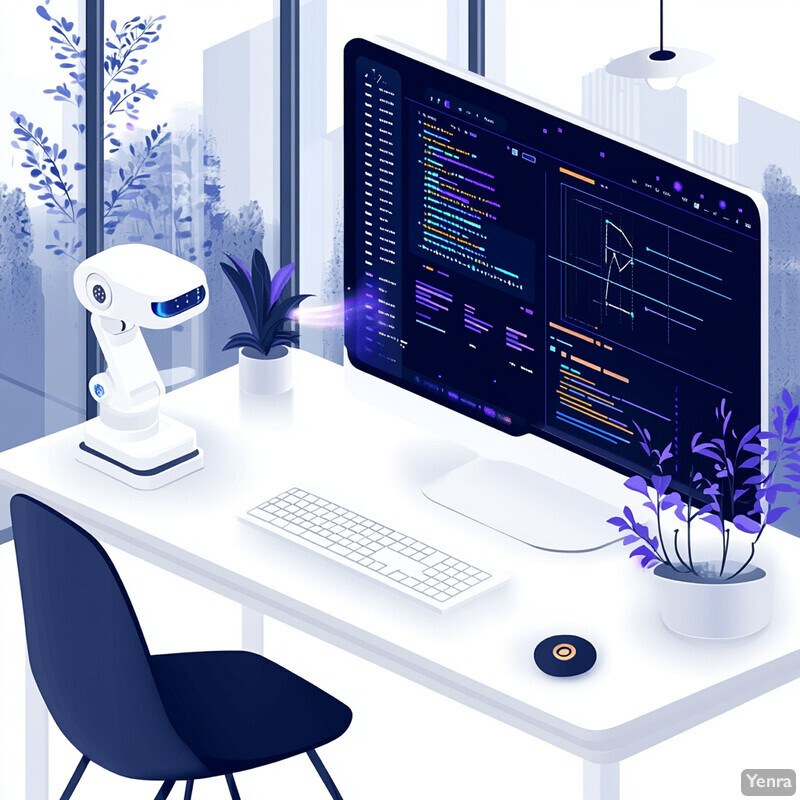
Controllers perform countless routine tasks, from reading flight plans to entering data into management systems and granting standard clearances. AI and automation tools can shoulder these repetitive activities, ensuring consistent adherence to standard procedures and reducing administrative burdens. By eliminating human error from routine data entry and freeing up mental bandwidth, controllers can maintain a higher level of concentration for higher-order decision-making, safety-critical issues, and supervisory oversight. This human-machine partnership increases overall efficiency and reduces the likelihood of fatigue-related mistakes.
17. Enhanced Arrival/Departure Rate Adjustments
AI models factor in current demand, weather, and operational constraints, suggesting precise adjustments to arrival and departure rates to maintain optimal throughput.
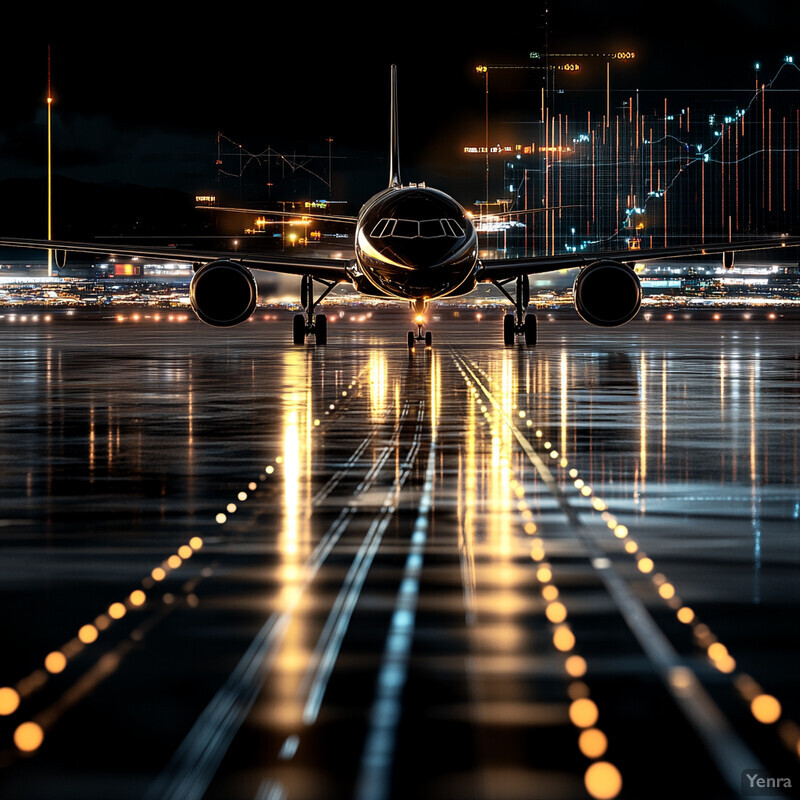
Adjusting the rate at which aircraft arrive and depart is a delicate balancing act influenced by runway availability, prevailing weather, and overall demand. AI can refine these adjustments by processing real-time data streams and predictive analytics. For example, if a weather system is predicted to slow arrival times, the system might incrementally reduce departure rates to prevent runway gridlock. Conversely, if traffic thins, the system can increase the arrival rate to accommodate more flights promptly. This fine-tuning, driven by intelligent modeling, helps airports run closer to their optimal capacity while maintaining safety and minimizing passenger delays.
18. Intelligent Gate and Ramp Management
By predicting gate availability and turnaround times, AI enables more efficient gate assignments, reducing waiting times and streamlining passenger transfers.
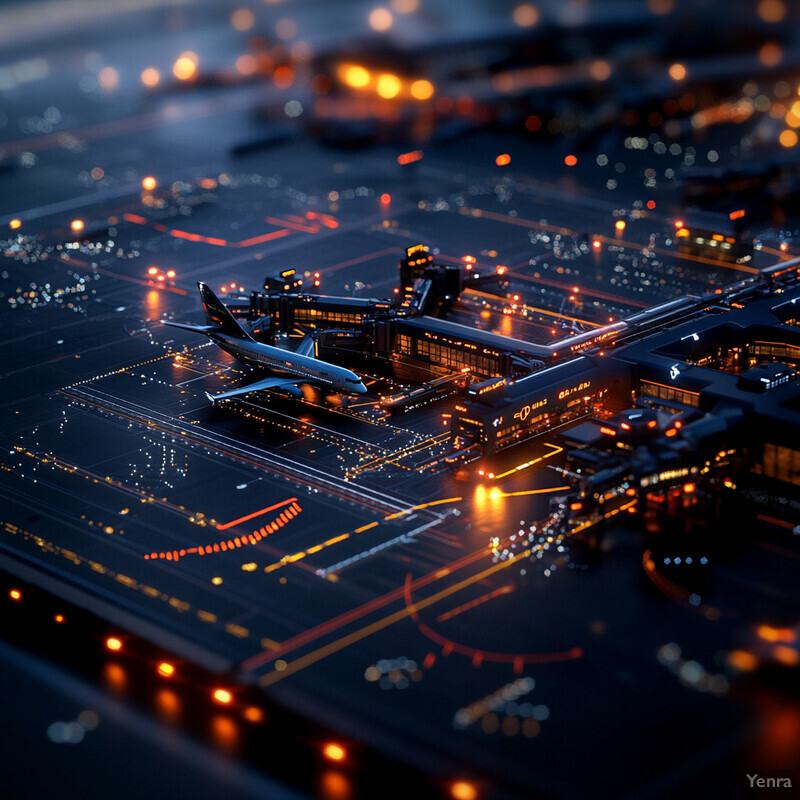
The gate and ramp area is a complex ballet of aircraft movement, ground vehicles, baggage handlers, passenger boarding, and maintenance crews. AI solutions observe patterns and constraints to optimize gate assignments and turnaround times. Predictive models anticipate when a flight will be ready for departure or when the next arriving aircraft can dock, minimizing gate occupancy downtime. By using historical performance and live updates, these intelligent systems prevent gate conflicts and reduce waiting times. Over the long term, this leads to more predictable airport operations, smoother passenger connections, and more efficient use of limited ground resources.
19. Air Traffic Flow Management Collaboration
AI tools can share predicted traffic flows and capacity data with different stakeholders—airlines, airports, and meteorological agencies—to ensure cohesive, well-informed decisions.
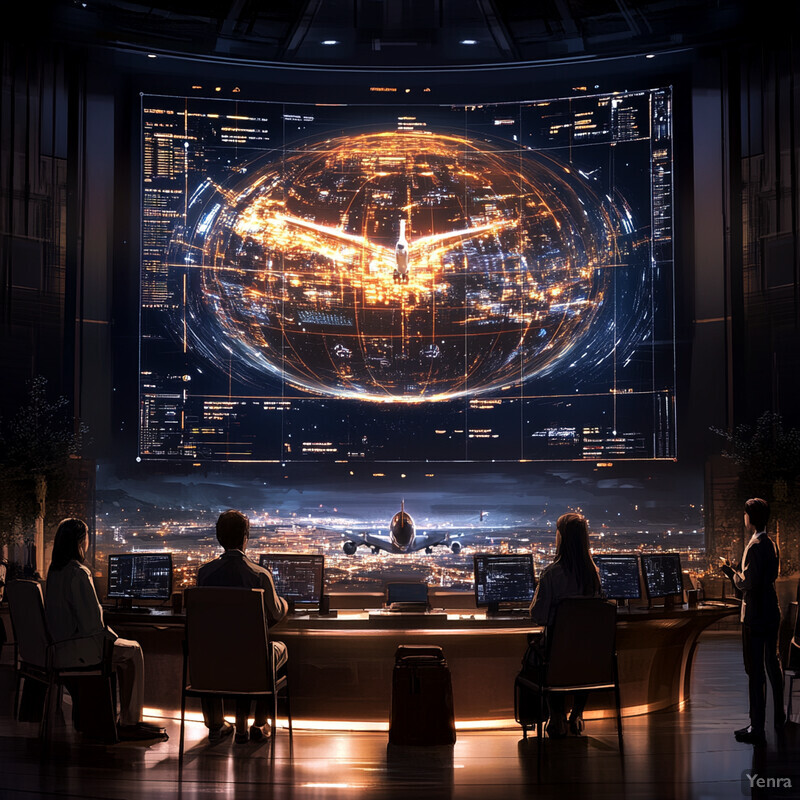
Managing air traffic flow requires input and cooperation among multiple stakeholders—airlines, airports, air navigation service providers, meteorological agencies, and regulators. AI platforms can serve as a common interface, aggregating diverse data feeds and sharing insights that are accessible and understandable to all parties. With clearer transparency into predicted traffic levels, weather forecasts, and capacity constraints, stakeholders can better coordinate their decisions. This cohesive planning environment allows for unified strategies, reducing surprises, improving punctuality, and enhancing the overall resilience of the air transportation network.
20. Continuous Learning and Adaptation
As AI models consume more data and improve over time, they can adapt to changing traffic patterns, emerging technologies (like electric VTOL vehicles), and evolving controller techniques, ensuring ongoing optimization of air traffic operations.
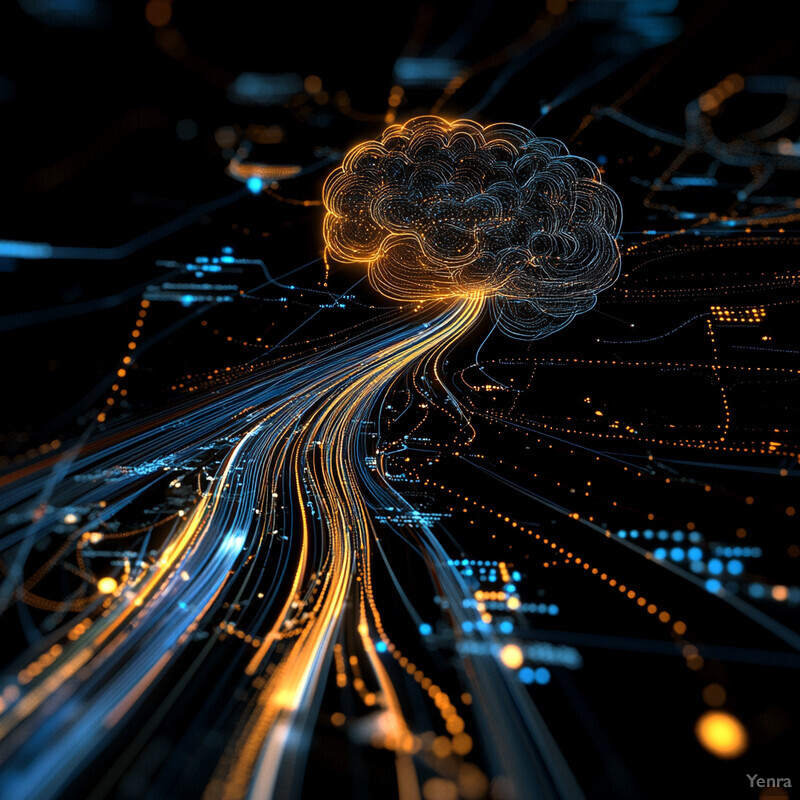
Perhaps the most transformative aspect of AI in air traffic control is its ability to learn and improve over time. Advanced machine learning models not only adapt to evolving traffic patterns and changing technologies but also incorporate lessons learned from operational feedback. As more data is collected and analyzed—from seasonal shifts in demand to the increasing presence of new types of aircraft—the AI systems become better at forecasting, optimizing, and making reliable recommendations. This continuous improvement ensures that the air traffic control environment remains agile, forward-looking, and capable of integrating future innovations and concepts like electric vertical takeoff and landing vehicles (eVTOLs) and space launch coordination into the national airspace system.