Song: Agricultural Pest and Disease Prediction
1. Remote Sensing and Imaging Analysis
AI models are applied to high-resolution satellite, drone, and aerial imagery to detect subtle changes in crop color, texture, and shape that can indicate early pest infestations or disease onset.
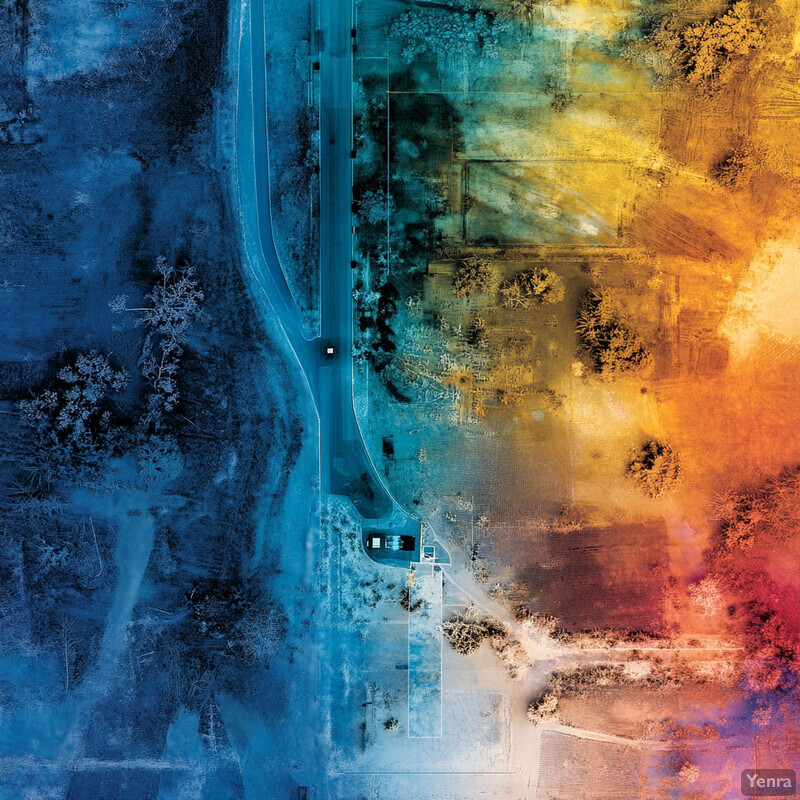
With the growing availability of high-resolution satellite imagery and advanced drone cameras, AI has become instrumental in analyzing visual data to detect the early signs of pests and diseases in crops. By processing multispectral and hyperspectral imaging data, machine learning models can identify subtle shifts in leaf coloration, canopy density, and crop morphology that are invisible to the naked eye. These early indicators can signal the onset of an infestation or infection well before it becomes severe. As a result, farmers gain time to implement targeted interventions, reducing both crop losses and the environmental impact of excessive pesticide use.
2. Machine Vision for In-Field Monitoring
Advanced computer vision algorithms can analyze real-time imagery from cameras mounted on farm equipment or robots, identifying pests, infected leaves, or fungal growth as soon as they appear.
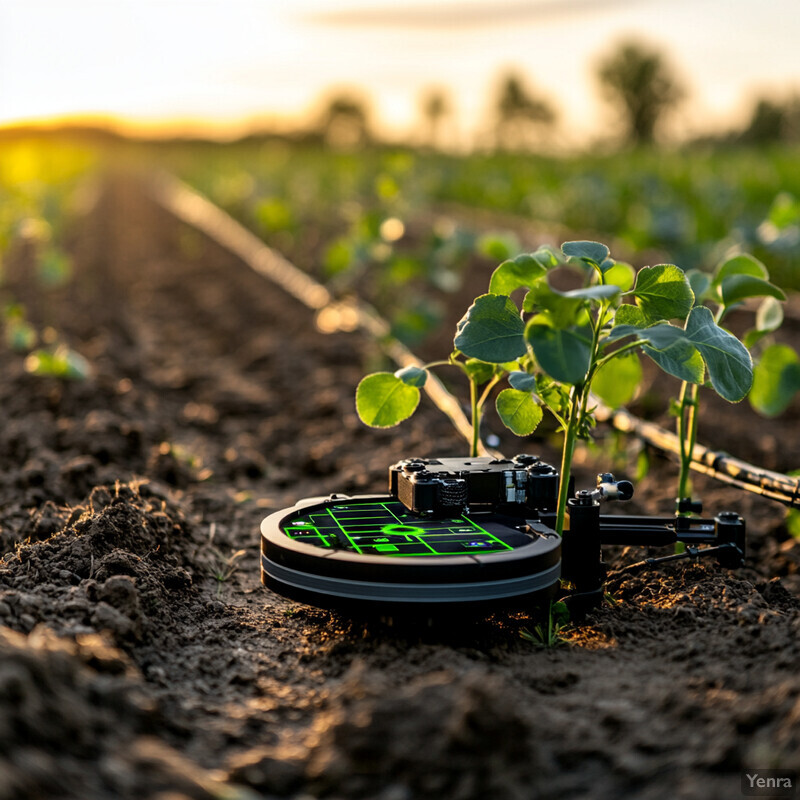
Beyond satellite imagery, AI-driven machine vision systems deployed at ground level can continuously monitor crops in real time. Cameras mounted on tractors, robotic scouts, or unmanned ground vehicles can scan individual plants for signs of pest damage, fungal spores, or bacterial lesions. Deep learning algorithms interpret patterns, detect anomalies, and differentiate between common crop stressors and actual disease symptoms. By offering this fine-grained analysis, farmers can concentrate their field visits and spraying routines on specific zones, saving time, labor, and resources while also enhancing the precision and timeliness of pest management measures.
3. Predictive Modeling Using Weather Data
AI integrates historical and current meteorological data with crop growth patterns, enabling predictive models to forecast the likelihood of specific pest outbreaks or disease occurrences based on weather conditions (e.g., temperature, humidity, rainfall).
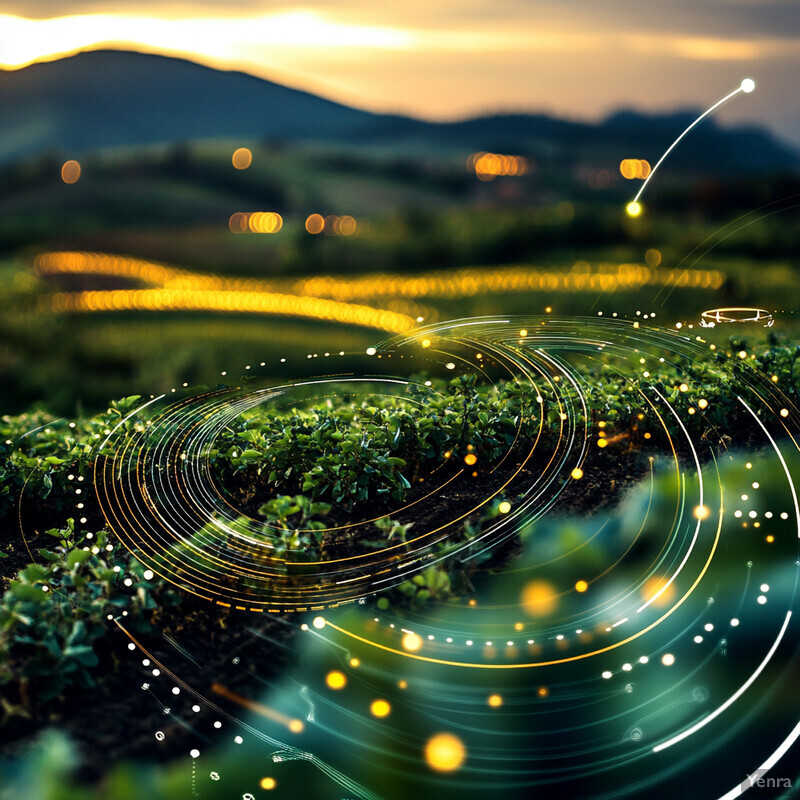
Weather conditions—temperature, humidity, rainfall, and sunlight—are core factors influencing pest life cycles and disease propagation. AI can integrate decades of historical climate data with current forecasts and on-the-ground conditions to predict the likelihood of pest and pathogen outbreaks. Advanced statistical methods and machine learning models identify correlations, revealing how certain weather patterns drive the emergence or spread of particular pests. Armed with these predictions, growers can anticipate periods of heightened vulnerability, plan preemptive treatments, adjust planting and harvesting schedules, and better safeguard their yields against environmental uncertainties.
4. Soil Health Analysis
Machine learning algorithms use soil testing data—nutrient composition, pH, moisture levels—to predict conditions that favor certain pathogens or pests, helping farmers proactively implement preventive measures.
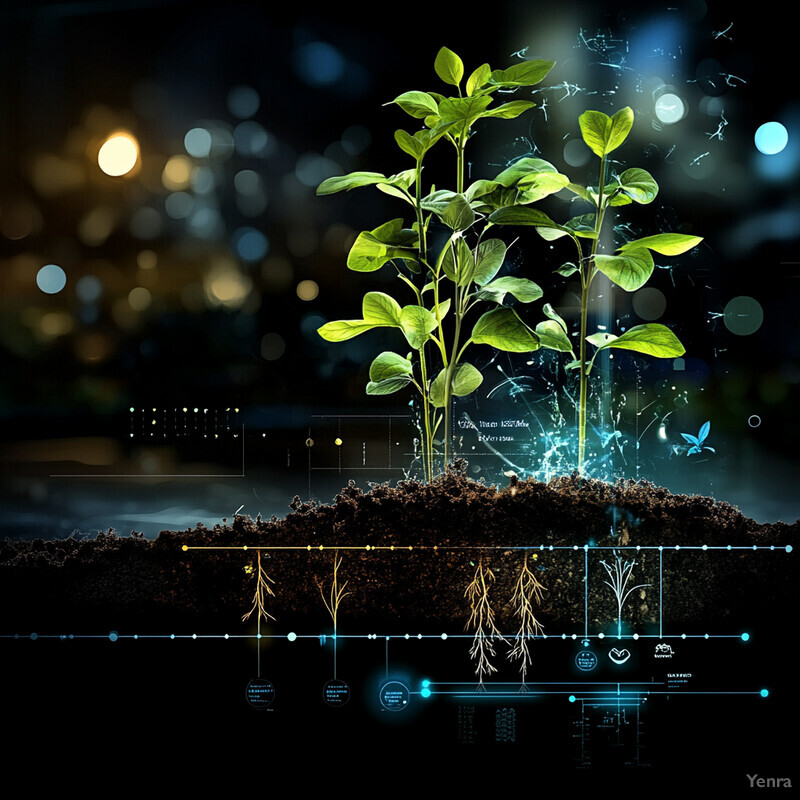
Soil properties such as nutrient balance, pH, and moisture directly affect plant health and resilience. AI algorithms leverage big data from soil sensors, field tests, and laboratory analyses to understand how specific soil conditions predispose crops to certain pests or diseases. Machine learning models can highlight relationships between nutrient deficiencies, soil compaction, or microbial imbalances and the subsequent outbreak of pathogens. Farmers can use these insights to amend soil quality through targeted fertilization, organic matter addition, or tillage practices that improve the soil ecosystem, thereby reducing disease occurrence and enhancing long-term crop vigor.
5. Early Warning Systems with Sensor Fusion
AI-driven systems combine data from multiple sensor types (moisture, temperature, acoustic, pheromone traps) to provide early alerts about pest presence and disease spread, improving timeliness of interventions.
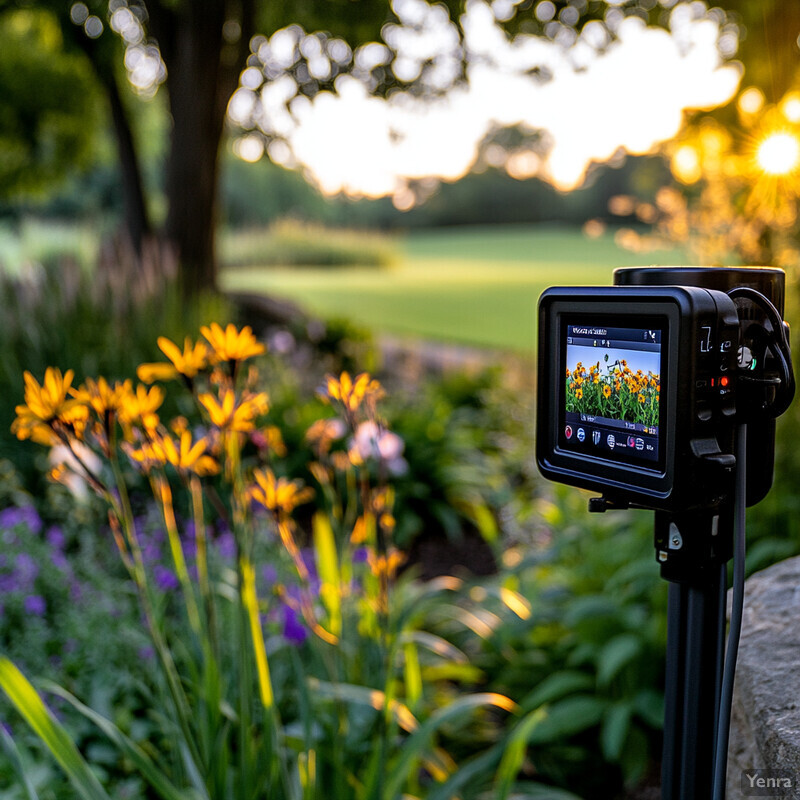
Modern farms deploy a range of Internet of Things (IoT) devices—from humidity sensors and pheromone traps to acoustic and temperature sensors. AI-based sensor fusion integrates these multiple streams of data, detecting complex patterns that may signal an impending pest population boom or disease onset. For example, an unusual spike in temperature coupled with higher moisture and pheromone trap counts might trigger an early-warning alert. This proactive approach ensures that farmers receive actionable information early, enabling them to respond with timely interventions rather than dealing with a full-blown outbreak later.
6. Phenotyping and Plant Trait Recognition
By analyzing images of plant leaves, stems, and overall structure, deep learning models can recognize phenotypic traits that suggest vulnerability to certain pests or diseases, guiding breeding for more resistant varieties.
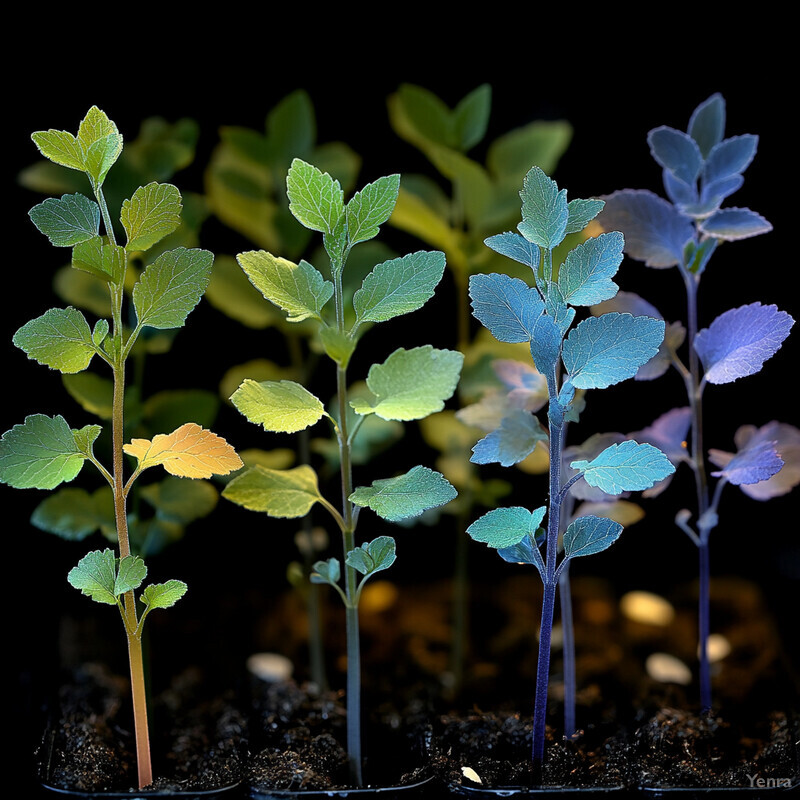
Some plants possess genetic traits that make them more susceptible or resistant to certain pests and pathogens. AI-based phenotyping tools analyze imagery and genetic data to classify these traits, enabling breeders and farmers to select cultivars that naturally resist or tolerate common threats. By comparing a plant’s leaf structure, coloration, and growth patterns against established disease-resistant profiles, AI can guide selective breeding efforts and seed selection. Over time, this leads to improved crop varieties that withstand pests and diseases more effectively, reducing the need for chemical treatments and stabilizing yields.
7. Automated Identification of Pest Species
AI-powered species recognition can classify pests at the egg or larval stage, helping farmers take action before populations explode and spread through the field.
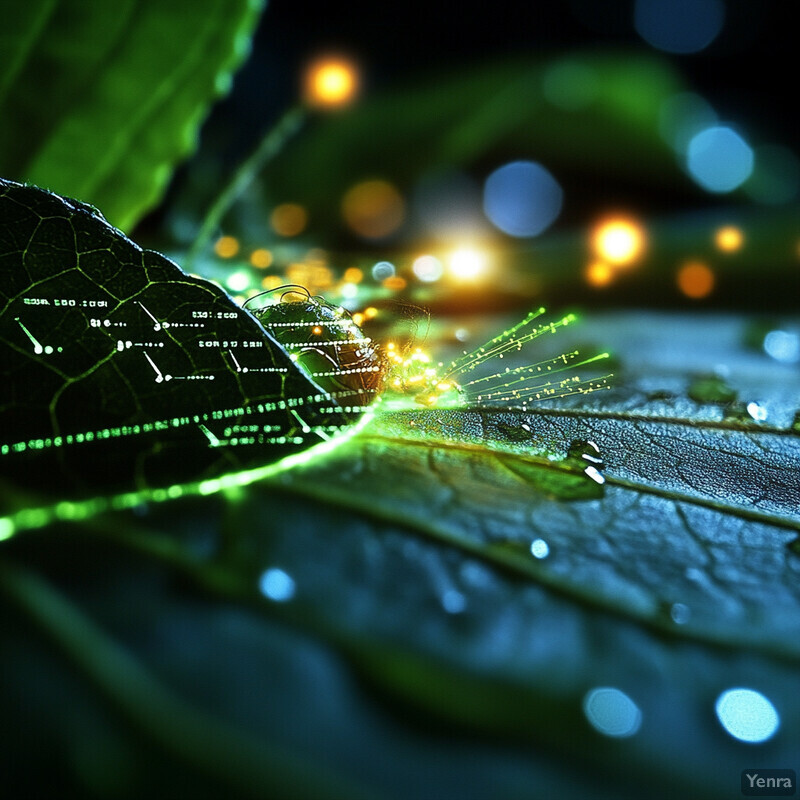
Identifying pests at an early stage is crucial for effective control. AI-driven image classification systems can differentiate between benign insects and harmful pests based on subtle morphological differences. Utilizing convolutional neural networks and large training datasets, these models recognize early-stage larvae, eggs, or adult insects even when they’re tiny or hidden under foliage. By pinpointing the exact species, farmers can select appropriate control strategies—be it biological, mechanical, or chemical—ensuring that interventions are effective and specifically targeted to the threat at hand.
8. Precision Spraying and Variable-Rate Treatments
Predictive AI models help determine exactly where and when to apply pesticides or fungicides, reducing chemical use, cost, and environmental impact while ensuring better pest and disease control.
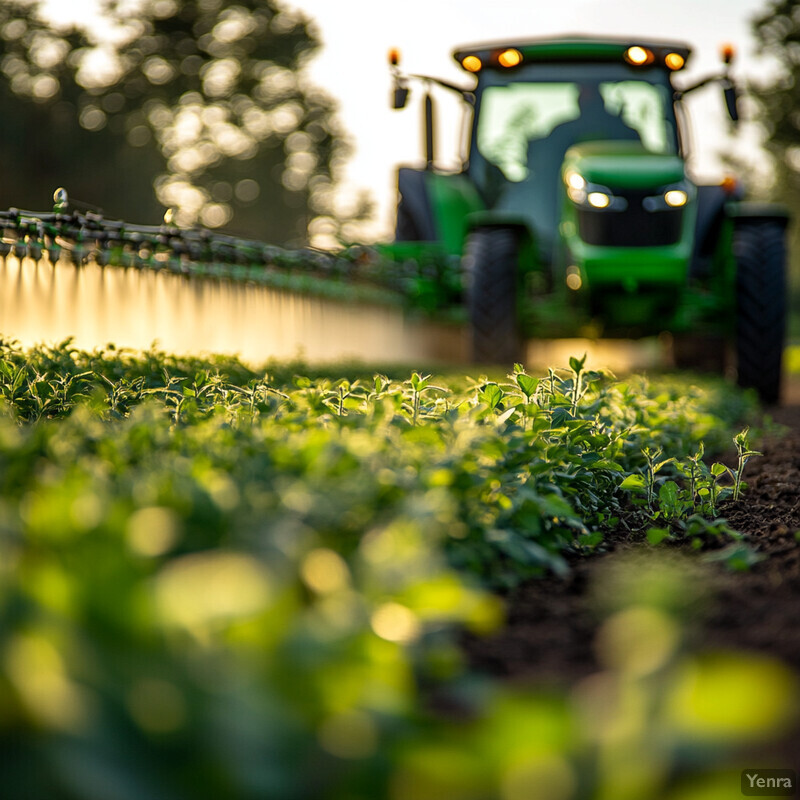
Traditionally, pesticide application often involves spraying entire fields uniformly, wasting chemicals and harming beneficial organisms. AI-guided variable-rate spraying systems integrate pest detection data with GPS coordinates and crop health indices to apply treatments only where and when needed. By using real-time maps and predictive analytics, farmers can fine-tune their pesticide use, cutting costs, reducing environmental impact, and improving overall crop quality. This level of precision not only protects the ecosystem but also prolongs the effectiveness of pesticides by decreasing the chance of pests developing resistance.
9. Geospatial Risk Mapping
Machine learning integrates geographic information systems (GIS) data to generate detailed risk maps, showing where pest populations are likely to be highest and allowing farmers to prioritize monitoring in hotspot areas.
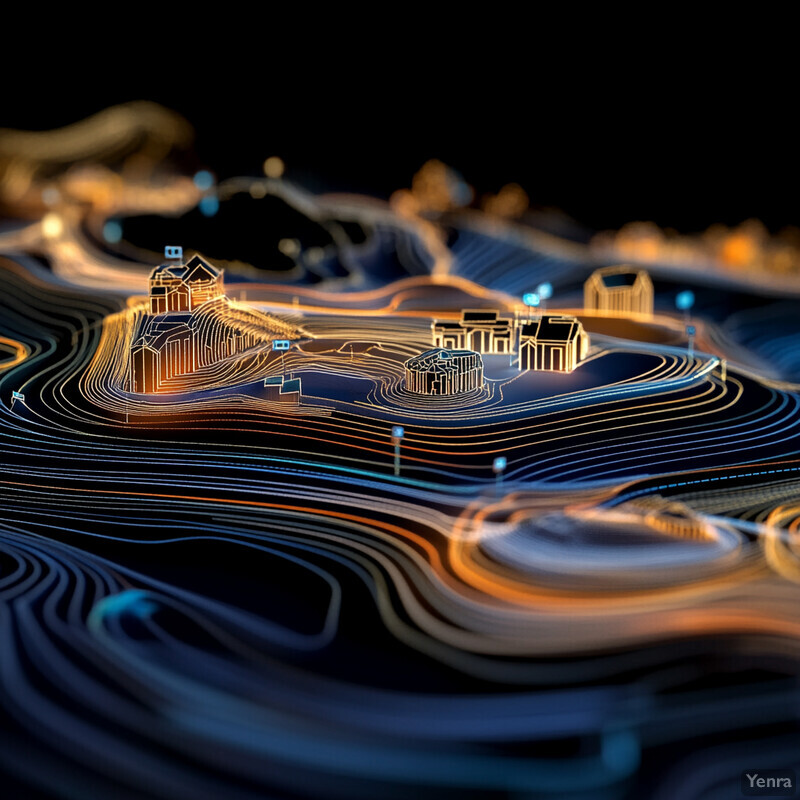
Geospatial analytics augmented by AI can produce detailed risk maps showing hotspots of pest pressure and disease likelihood. By combining spatial data—such as elevation, proximity to water bodies, crop distribution, and historical infection patterns—with predictive modeling, AI pinpoints fields or regions at greatest risk. Farmers and extension workers can use these maps to focus scouting efforts, implement buffer zones, or adopt preventive measures in high-risk areas. Over time, these geospatial insights enable a more informed and strategic approach to pest and disease management at both farm and regional levels.
10. Long-Term Trend Analysis
Through data aggregation over multiple seasons, AI models can detect patterns and emerging trends, providing long-term guidance on how changing climate conditions and farming practices influence pest and disease patterns.
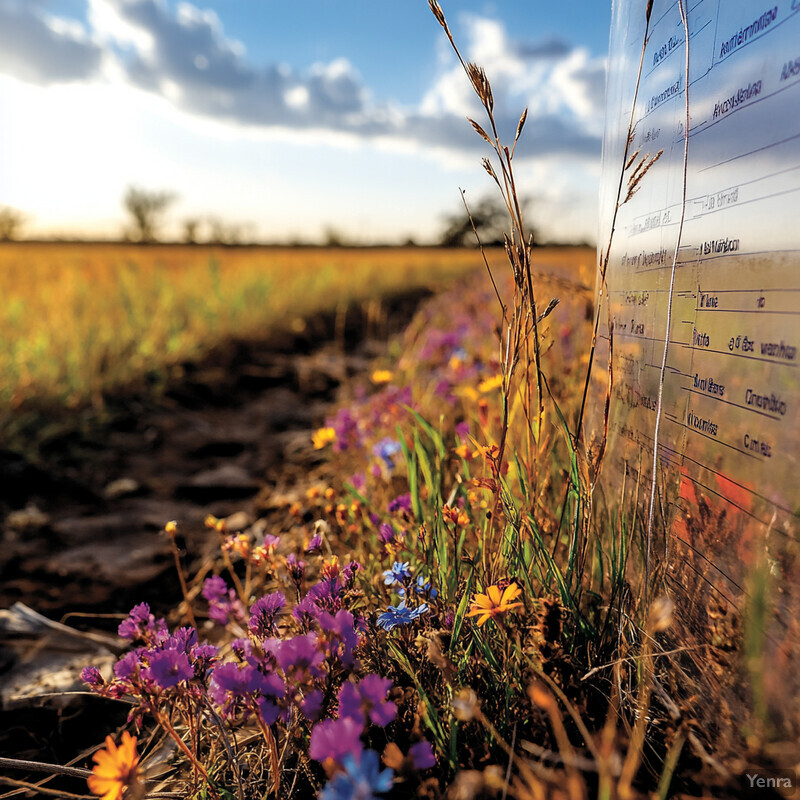
Agricultural production occurs against a backdrop of climatic shifts, evolving pest populations, and changing land use patterns. AI-powered long-term trend analysis examines multi-year datasets to uncover how pests and diseases respond to variables like global warming, invasive species introductions, or altered cropping sequences. These insights help researchers and policymakers predict future challenges, adjust integrated pest management (IPM) strategies, and even guide farmers toward more sustainable rotations and cultivar choices. The long view afforded by AI ensures resilience planning that can handle an uncertain agricultural future.
11. Natural Language Processing for Research Integration
NLP-based AI tools can scan scholarly articles, extension bulletins, and regulatory documents, extracting insights and new findings about pest behaviors and disease management strategies to improve prediction models.
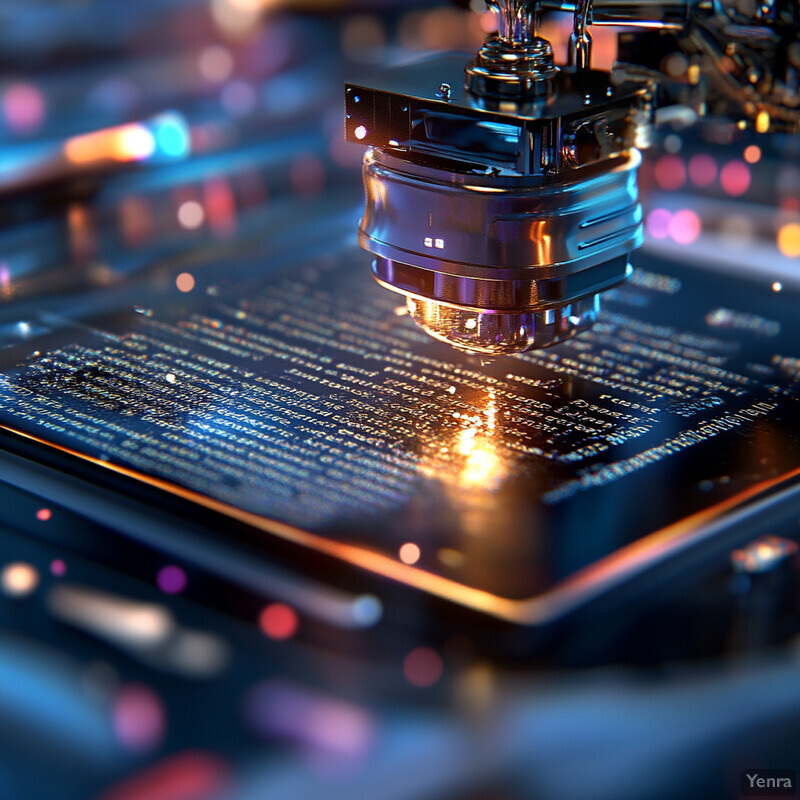
The body of scientific literature and extension publications on pests and diseases is vast. Using NLP, AI systems can quickly sift through thousands of papers, agronomic reports, and regulatory guidelines, extracting relevant knowledge and summarizing findings into actionable insights. By synthesizing the latest academic discoveries, historical best practices, and emerging regulatory frameworks, AI-driven NLP ensures that farmers, agronomists, and decision-makers stay informed of cutting-edge solutions. This continuous research integration supports evidence-based improvements in prediction models and management techniques.
12. Real-Time Alerts and Dashboards
AI-powered dashboards provide farmers with up-to-date alerts on pest and disease risks, integrating multiple data streams and offering clear recommendations for immediate action.
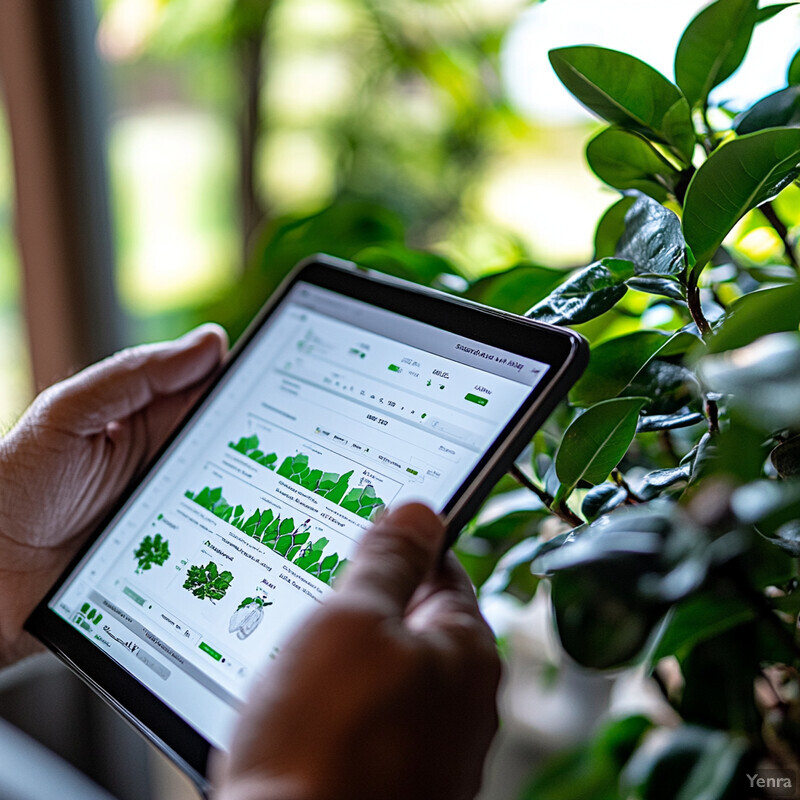
AI-powered platforms present complex data in user-friendly dashboards accessible via desktops, tablets, or smartphones. These interfaces deliver immediate alerts when sensor thresholds are crossed, or when predictive models foresee a pest outbreak in the coming days. By integrating data visualization and user-friendly analytics, farmers can grasp their field’s current status at a glance and receive clear recommendations for action. Such platforms facilitate better on-the-spot decision-making, reduce guesswork, and enhance the overall efficiency of farm operations.
13. Adaptive Learning Systems
Continual learning algorithms refine their models as they acquire new data, becoming progressively more accurate in predicting outbreaks and adjusting recommendations as conditions evolve.
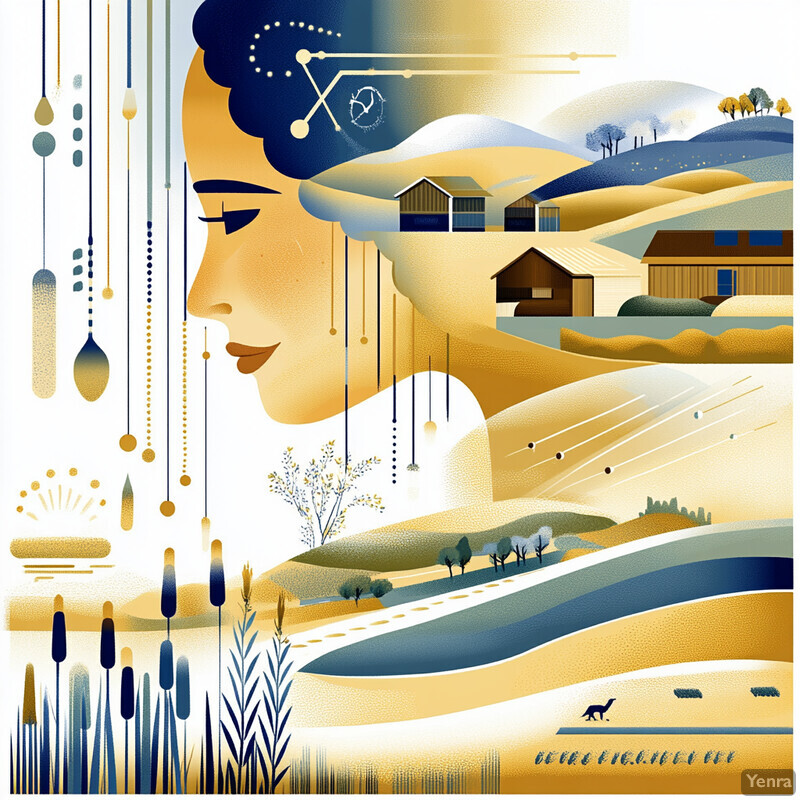
The agricultural landscape is dynamic—new pest species emerge, climates shift, and agricultural practices evolve. Adaptive learning AI models continuously refine themselves as they encounter fresh data. When predictions fail or unexpected outbreaks occur, these systems learn from the discrepancies and update their patterns. Over time, this adaptability leads to more accurate forecasts, tailored to each farm’s unique conditions. As the model learns from every season’s successes and failures, it grows more reliable, ensuring that farmers can count on increasingly precise predictions and recommendations.
14. Farmer-Contributed Data and Crowdsourcing
AI systems can incorporate data shared by farmers—such as observations or smartphone images of affected crops—improving prediction accuracy and enabling community-based early detection networks.
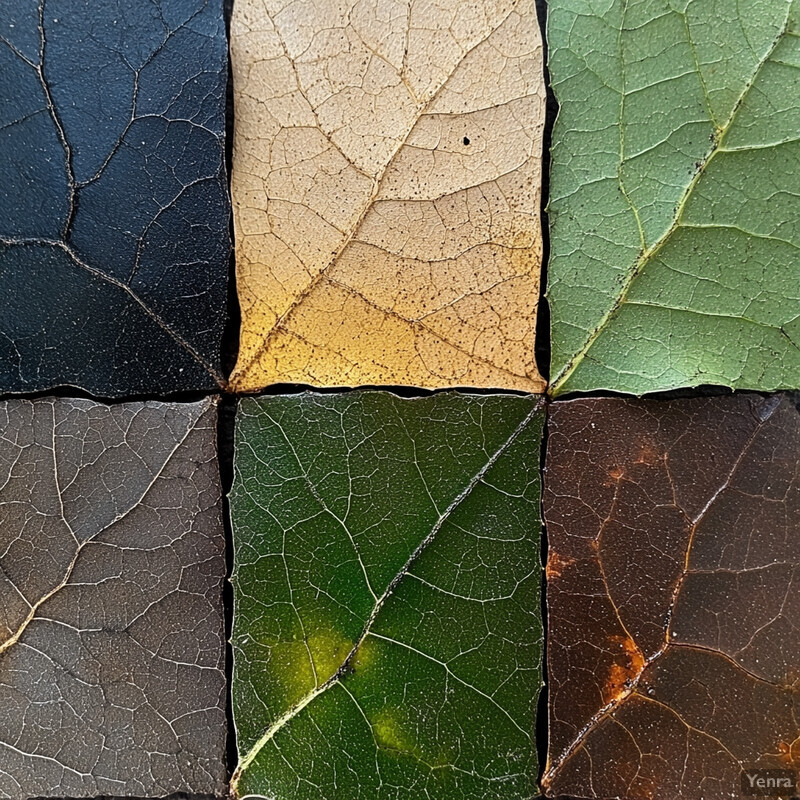
Farmers are often the first to spot abnormal symptoms or pest presence. AI platforms that invite farmers to upload smartphone images, notes, and observations crowdsource valuable, localized data. Machine learning models that incorporate this user-generated information gain access to real-time, ground-truth inputs across vast geographies. By validating and cross-referencing farmer data, these models improve their detection and prediction accuracy. This collaborative approach democratizes agricultural intelligence, making it accessible and beneficial to all participants in the value chain.
15. Integration with IoT Devices
Smart traps, drones, and field sensors connected to AI systems deliver continuous, automated pest population monitoring, triggering alerts as soon as certain thresholds are reached.
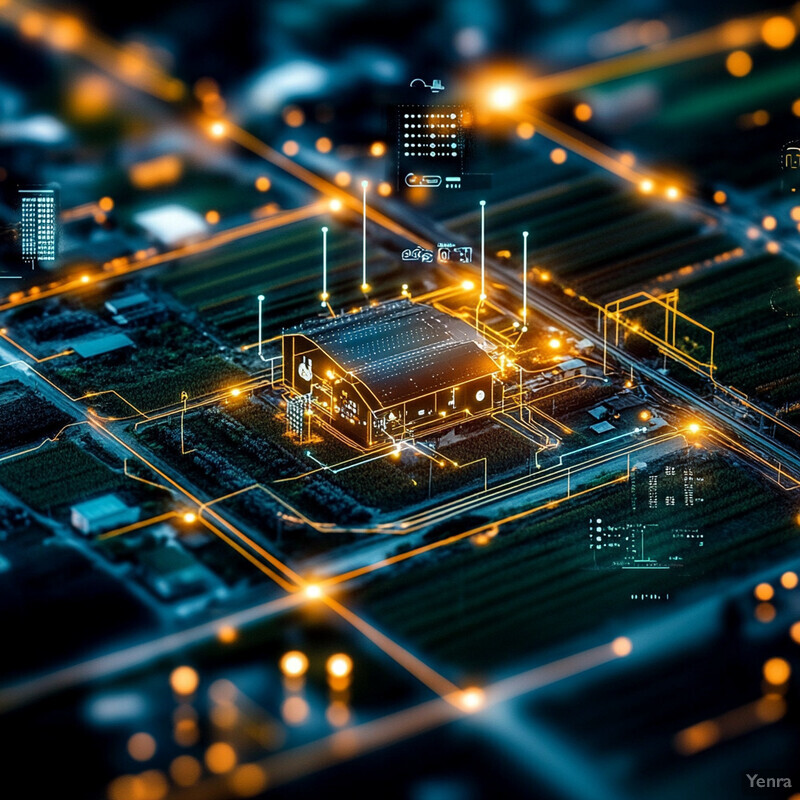
Smart farms equipped with IoT devices continuously generate data on soil moisture, plant health, insect trap counts, and even microscopic spore detection. AI unifies these heterogeneous data streams into a coherent picture of pest and disease status. By correlating sensor readings with historical patterns, AI can infer subtle signals—like a slight uptick in humidity plus an increased pheromone trap count—as precursors to a pest outbreak. This integrated approach fosters a proactive, data-driven pest management strategy that reduces reliance on calendar-based treatments.
16. Modeling Pathogen Life Cycles
AI can simulate how pathogens survive, spread, and thrive under different conditions, predicting disease cycles and helping farmers disrupt key phases through targeted intervention.
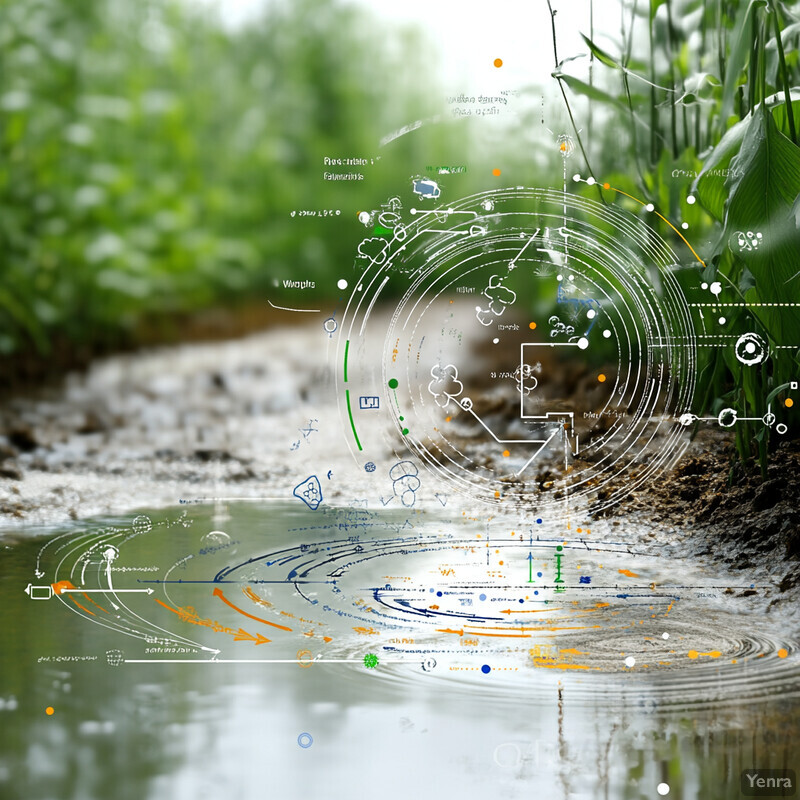
Understanding a pathogen’s life cycle is key to disrupting its spread. AI simulates the complex interactions that drive disease progression, incorporating factors such as vector populations, spore dispersal patterns, and host plant susceptibility stages. Advanced simulation models can predict when a pathogen is most vulnerable, informing strategic interventions that target those critical windows. By timing treatments precisely—spraying just before spore release or interrupting a pest’s breeding cycle—farmers can maximize the efficacy of their interventions while minimizing overall chemical use.
17. Multi-Crop and Intercropping Analysis
Complex AI models handle diverse cropping systems, identifying how interactions between different crops influence pest and disease dynamics, guiding crop rotation or intercropping strategies to reduce risk.
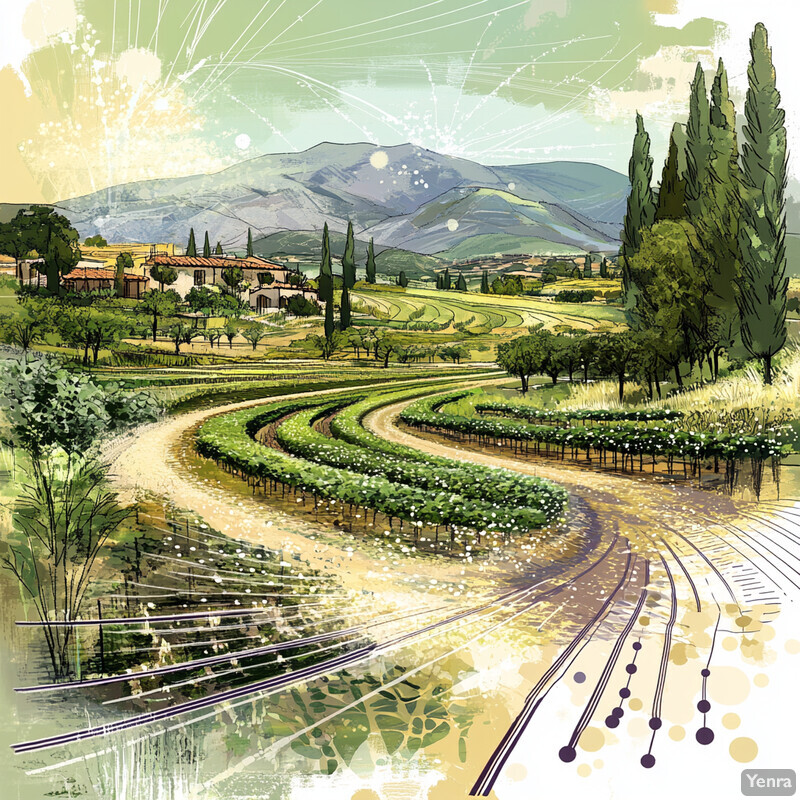
Diverse cropping systems, where multiple species are grown together or in rotation, can disrupt pest and disease cycles. AI analyzes these complex scenarios by identifying how certain crop combinations deter pests or foster beneficial predators. By processing data on intercropping patterns, companion planting benefits, and rotational sequences, AI guides growers toward practices that naturally reduce pest pressure. This systems-level approach enhances biodiversity, improves soil health, and contributes to sustainable agriculture that is more resilient to pest and disease challenges.
18. Remote Diagnosis via Smartphone Apps
AI-powered diagnostic apps allow farmers to capture and upload images of symptomatic plants, receiving rapid diagnoses and treatment recommendations even without on-site experts.
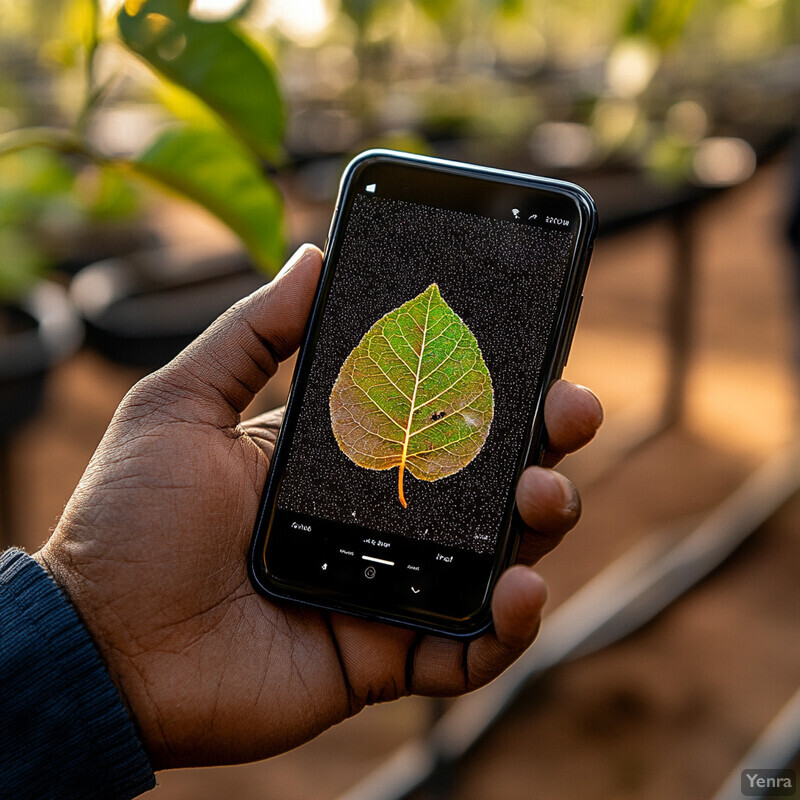
Even in regions without advanced field sensors, a smartphone camera and an AI-powered diagnostic app can bring expert-level pest and disease identification to farmers’ fingertips. By comparing an uploaded photo with vast image libraries, machine learning algorithms can diagnose problems in seconds. The app’s recommendations might include immediate measures like removing infected leaves, adjusting irrigation, or applying a particular biopesticide. This approach democratizes access to precision agriculture, empowering farmers with limited resources to make informed, timely decisions.
19. Risk Indexing and Forecast Models
Using a combination of weather, soil, and historical outbreak data, AI assigns a dynamic risk score for different pests and diseases, enabling farmers and agronomists to allocate resources more efficiently.
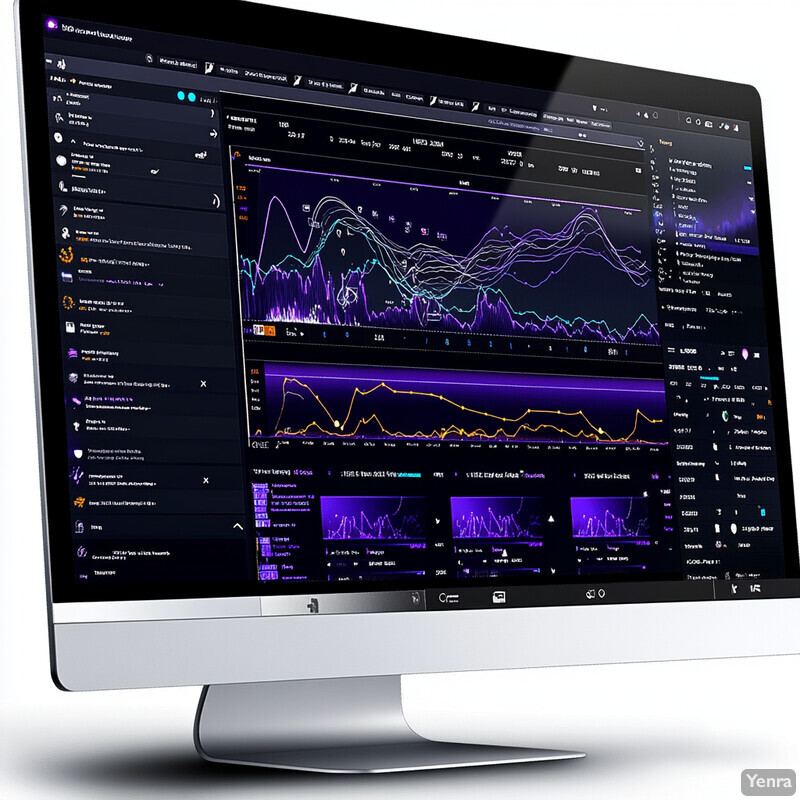
A single numerical “risk score” that synthesizes multiple variables—weather forecasts, soil conditions, historical outbreak data, and current pest scouting results—can guide resource allocation and management priorities. AI models produce such indices to quantify pest and disease risk, making complex data comprehensible at a glance. Farmers can use these risk scores to plan pesticide budgets, schedule crop rotations, or invest in protective infrastructure. By converting raw data into actionable metrics, AI turns uncertainty into strategic opportunity.
20. Integration with Supply Chain Forecasting
By forecasting pest and disease outbreaks, AI helps predict potential crop yield impacts. This integration informs broader decision-making, from procurement and market predictions to ensuring stable supply and food security.
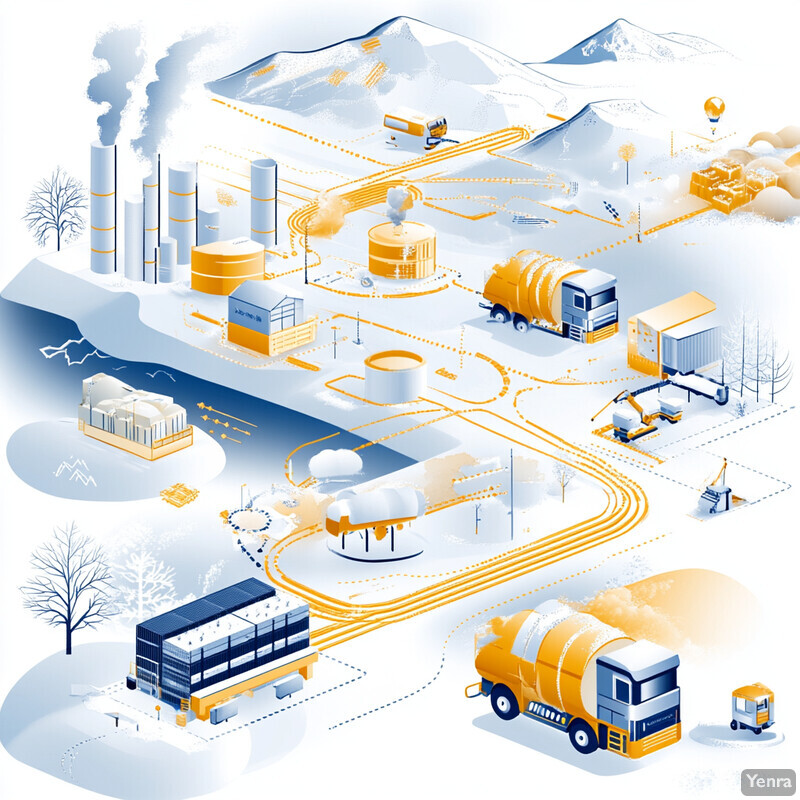
The impacts of pest and disease outbreaks extend beyond the farm gate, influencing yield availability, market prices, and food security. AI-driven supply chain forecasting models incorporate pest and disease predictions to estimate changes in production volumes and quality. By understanding how a potential outbreak might affect crop output, traders, distributors, and policymakers can adjust their procurement strategies, stabilize markets, and minimize waste. This holistic view ensures that improved pest and disease forecasting not only benefits farmers but also supports the entire agricultural supply chain and ultimately consumers.