1. Increased Accuracy
AI enhances the accuracy of speech recognition systems by better understanding diverse accents, dialects, and speech nuances, even in noisy environments.
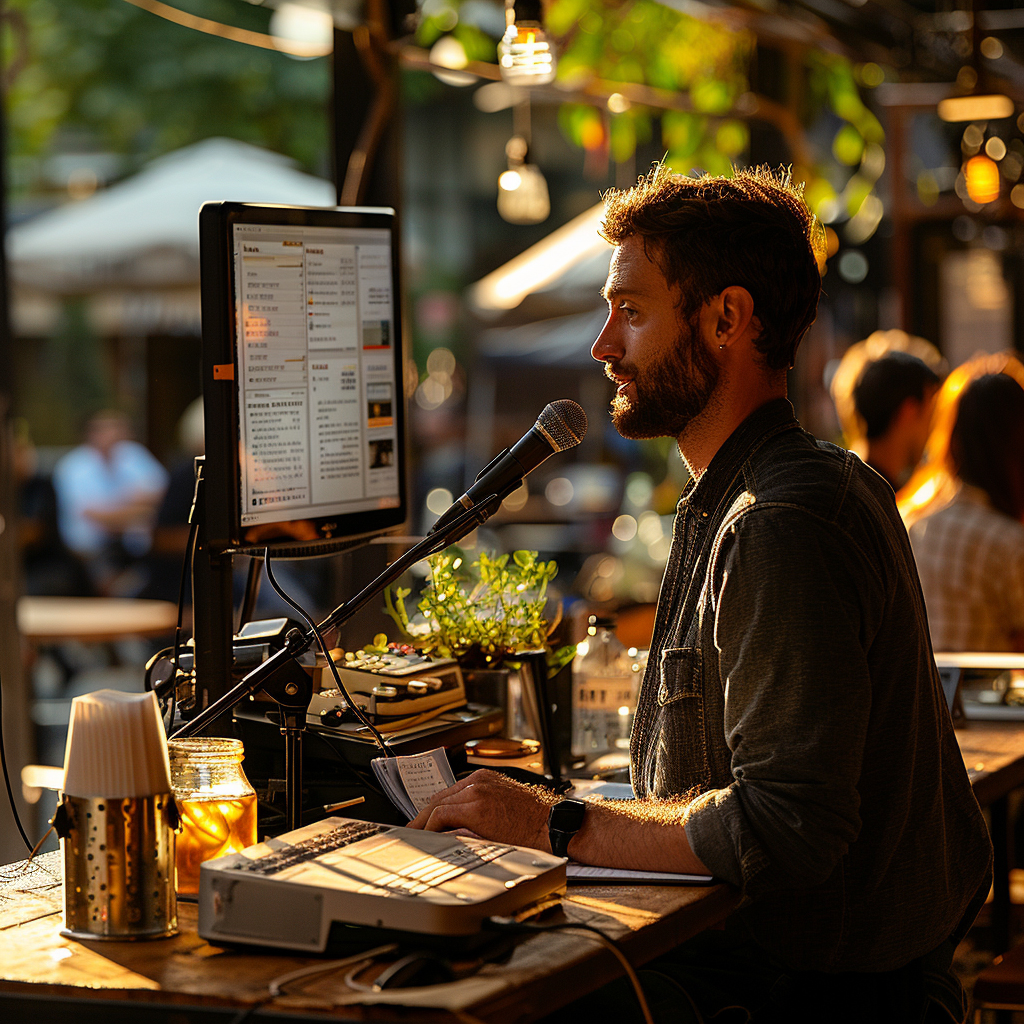
AI significantly improves the accuracy of speech recognition systems by using sophisticated machine learning models that better understand variations in speech such as accents, dialects, and individual speech idiosyncrasies. This is crucial for applications where precision is vital, such as voice-activated systems and transcription services. AI algorithms are trained on diverse datasets, which allow them to recognize and accurately transcribe speech from a wide range of speakers under various conditions.
2. Real-Time Processing
AI enables speech recognition systems to process and convert spoken language into text instantaneously, facilitating real-time communication and transcription.
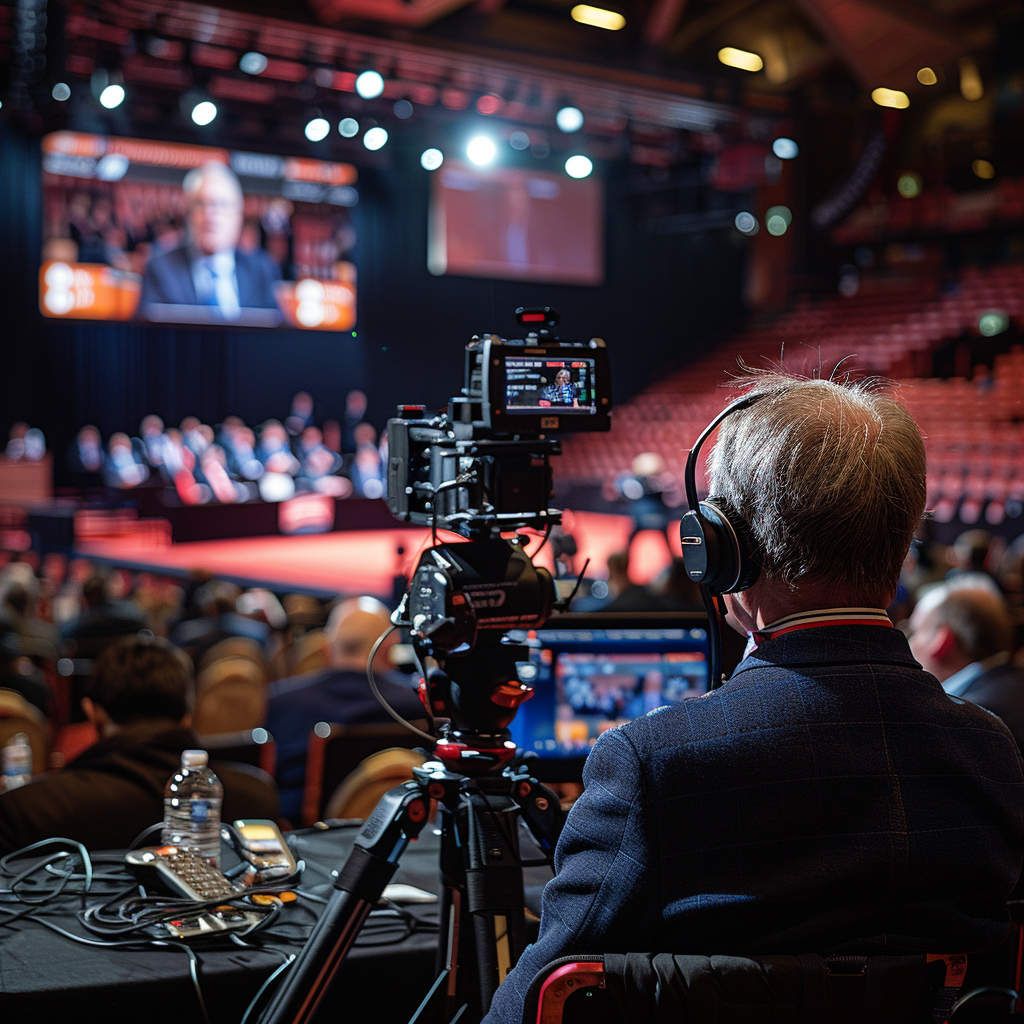
AI enables speech recognition systems to convert spoken language into written text instantly. This real-time processing is essential for applications such as live subtitling and real-time communication tools for the hearing impaired. By minimizing latency, AI enhances the usability and effectiveness of voice-activated assistants and other interactive systems that rely on immediate feedback.
3. Contextual Understanding
I algorithms improve the ability to grasp the context in which words are spoken, helping to distinguish between homophones based on sentence context, thereby reducing errors.
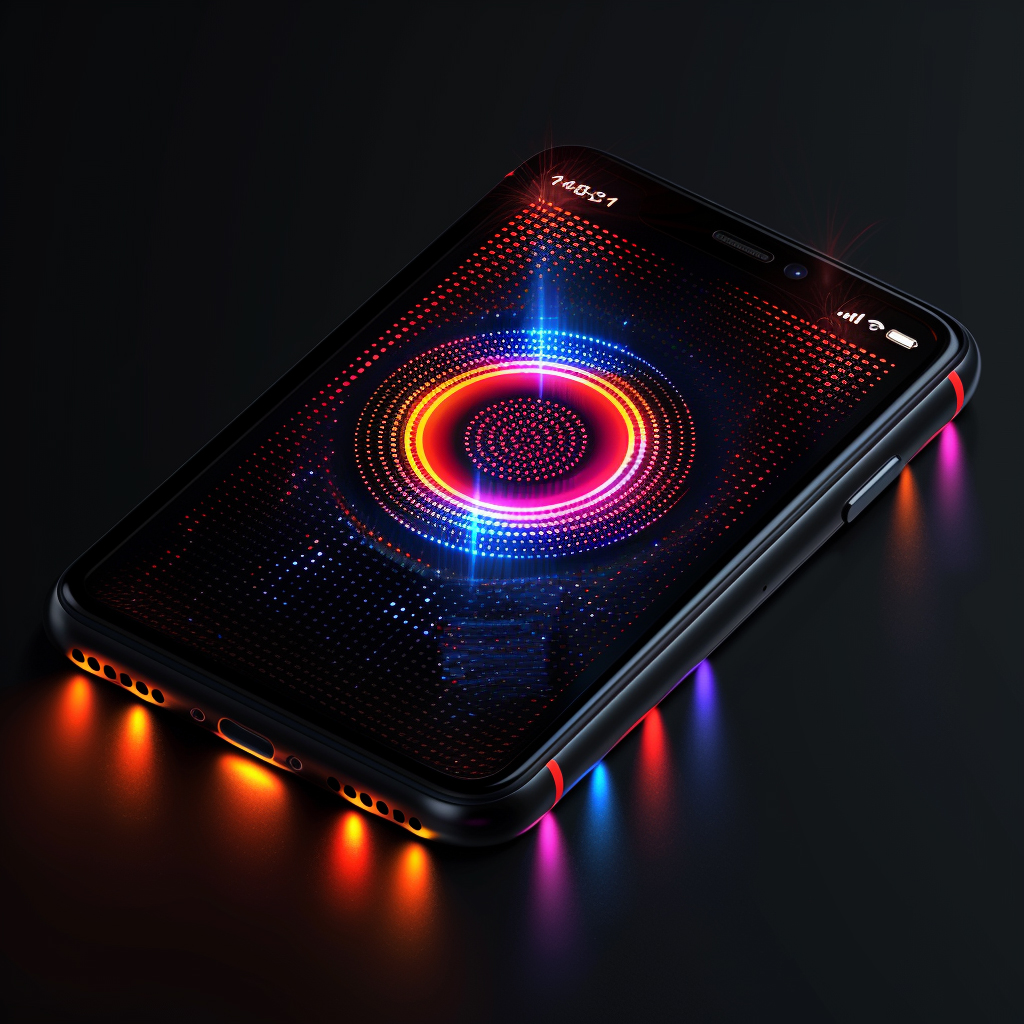
Through advancements in natural language processing (NLP), AI enhances speech recognition systems’ ability to understand the context in which words are spoken. This contextual awareness helps differentiate homophones (words that sound the same but have different meanings) based on the surrounding content, reducing misunderstandings and errors in transcription or voice commands.
4. Language and Dialect Adaptability
AI-driven systems can learn and adapt to a wide range of languages and regional dialects, broadening their usability globally.
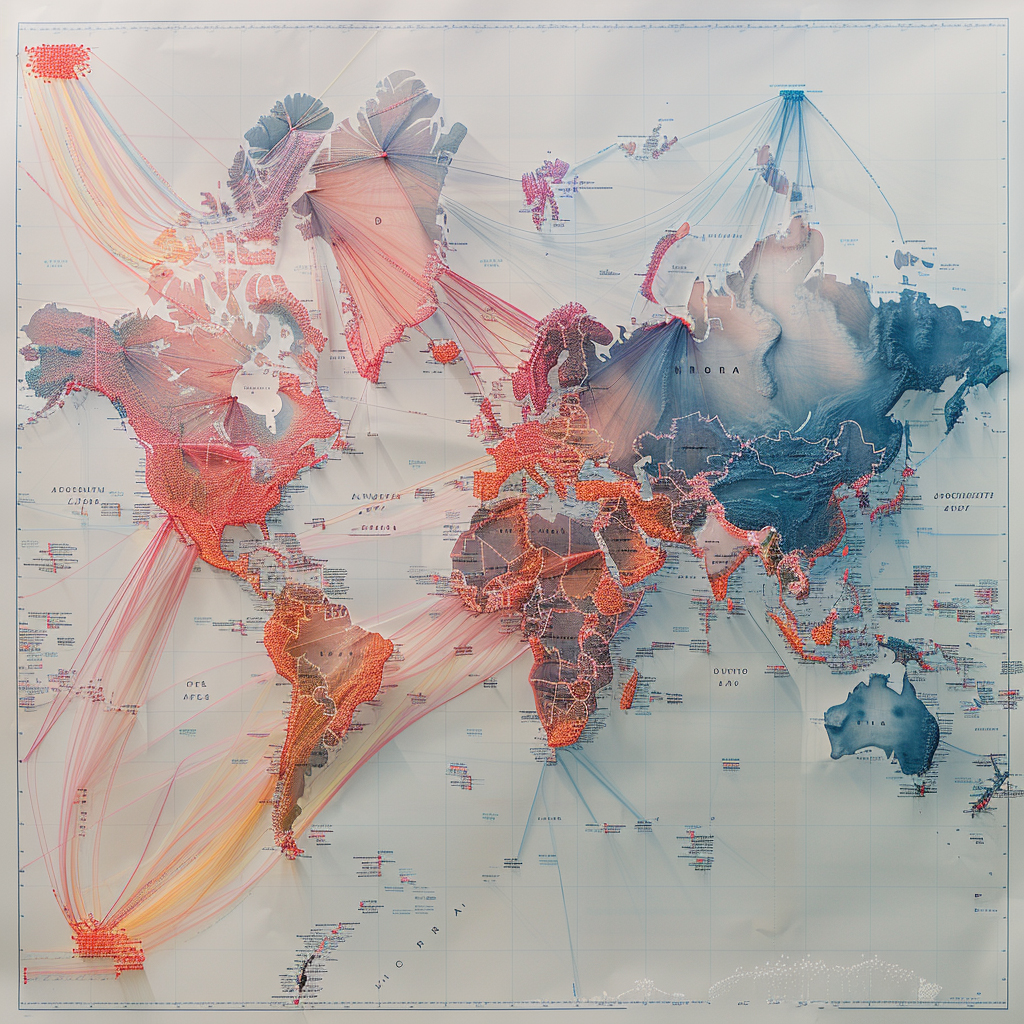
AI-driven speech recognition systems are equipped to learn and adapt to a variety of languages and dialects, making them more versatile and accessible on a global scale. This adaptability is achieved by training the AI on extensive datasets that include a range of linguistic variations, thereby enhancing the system's ability to serve users from different linguistic backgrounds.
5. Noise Cancellation
AI enhances speech recognition by effectively filtering out background noises and focusing on the speaker's voice, which is crucial for applications in public or chaotic environments.
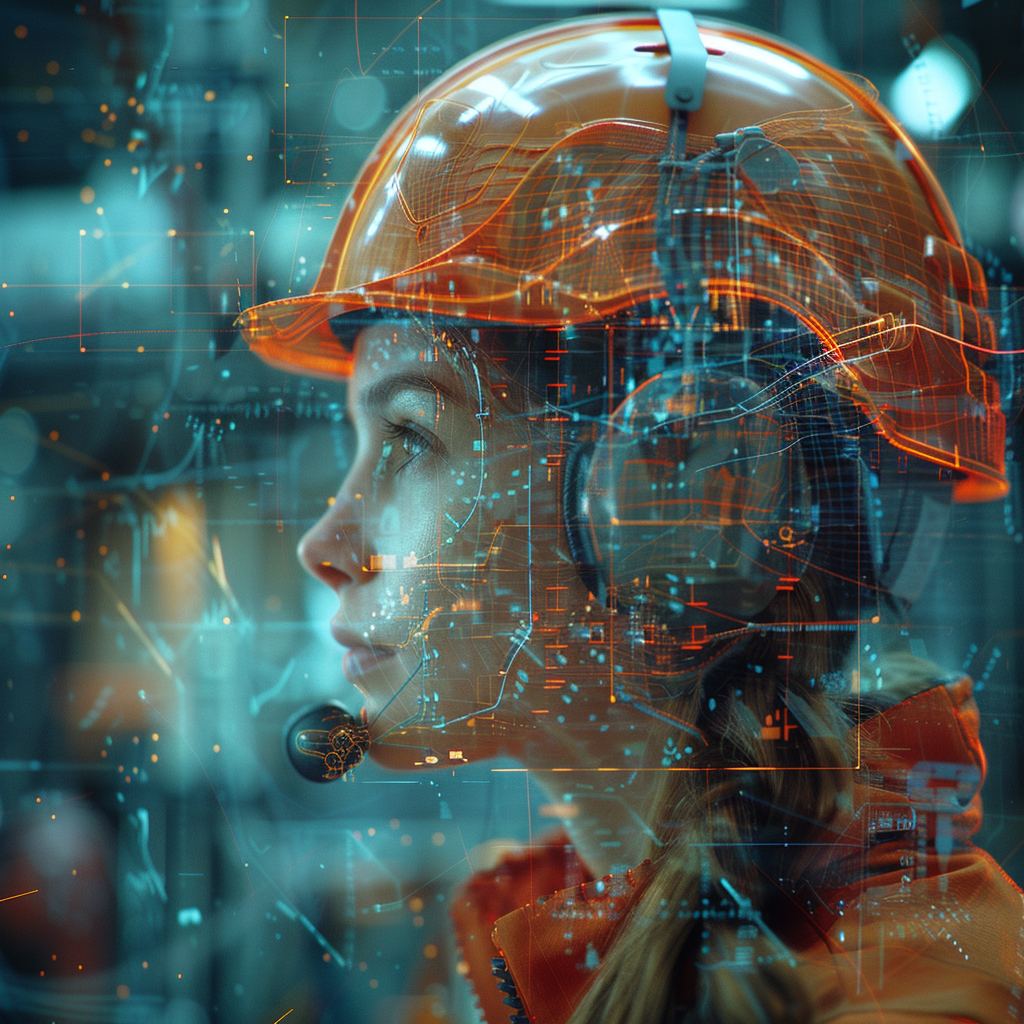
AI improves the capability of speech recognition systems to filter out background noise and focus on the primary speaker's voice. This is particularly important in environments with significant ambient noise, such as busy streets or crowded places. By employing advanced algorithms that isolate speech from noise, AI enables more accurate voice recognition in less-than-ideal acoustic conditions.
6. Integration with IoT Devices
AI facilitates the integration of speech recognition with IoT devices, enabling users to control various smart devices through voice commands.
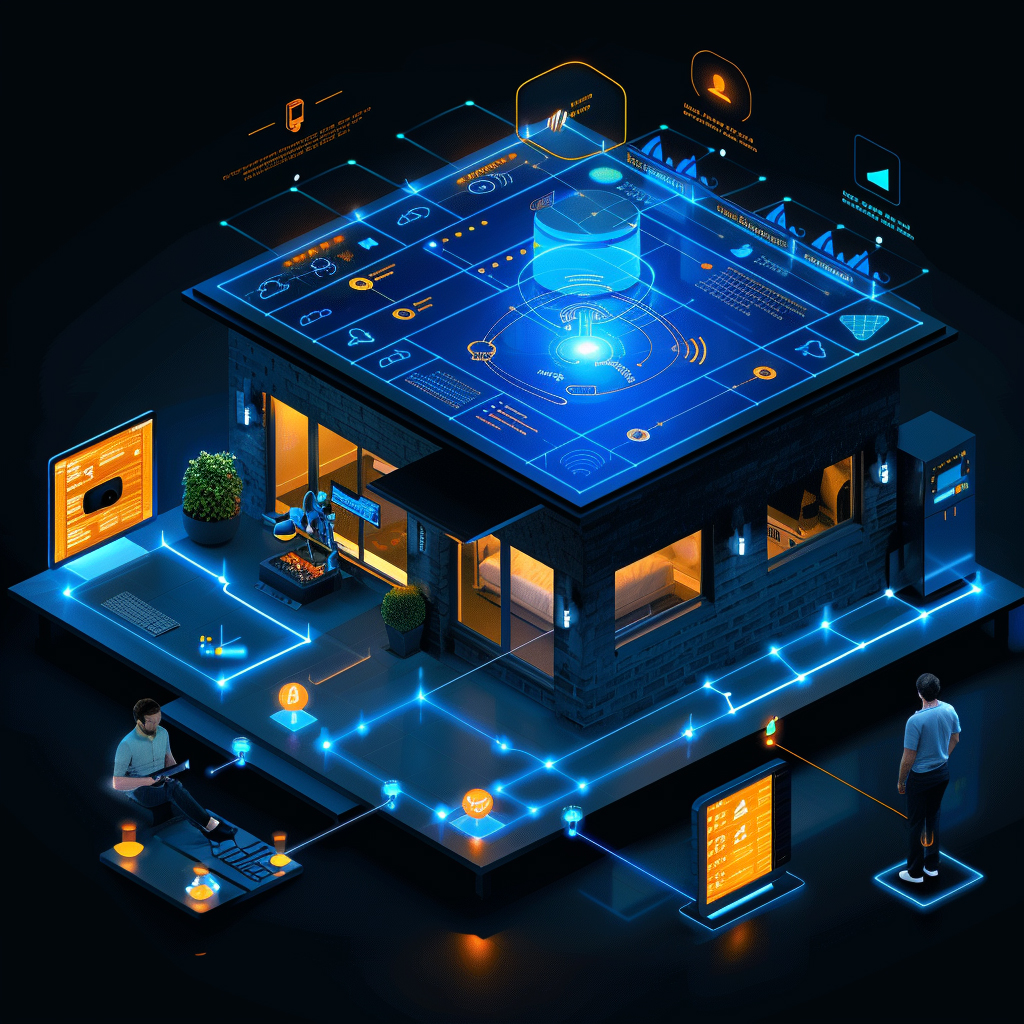
AI facilitates the integration of speech recognition with Internet of Things (IoT) devices, allowing users to control smart home devices, vehicles, and other connected systems purely through voice commands. This integration relies on AI's ability to process and interpret spoken commands accurately and execute actions seamlessly across a network of devices.
7. Voice Biometrics
AI uses speech recognition for secure user authentication by analyzing voice patterns, offering a convenient and secure biometric verification method.
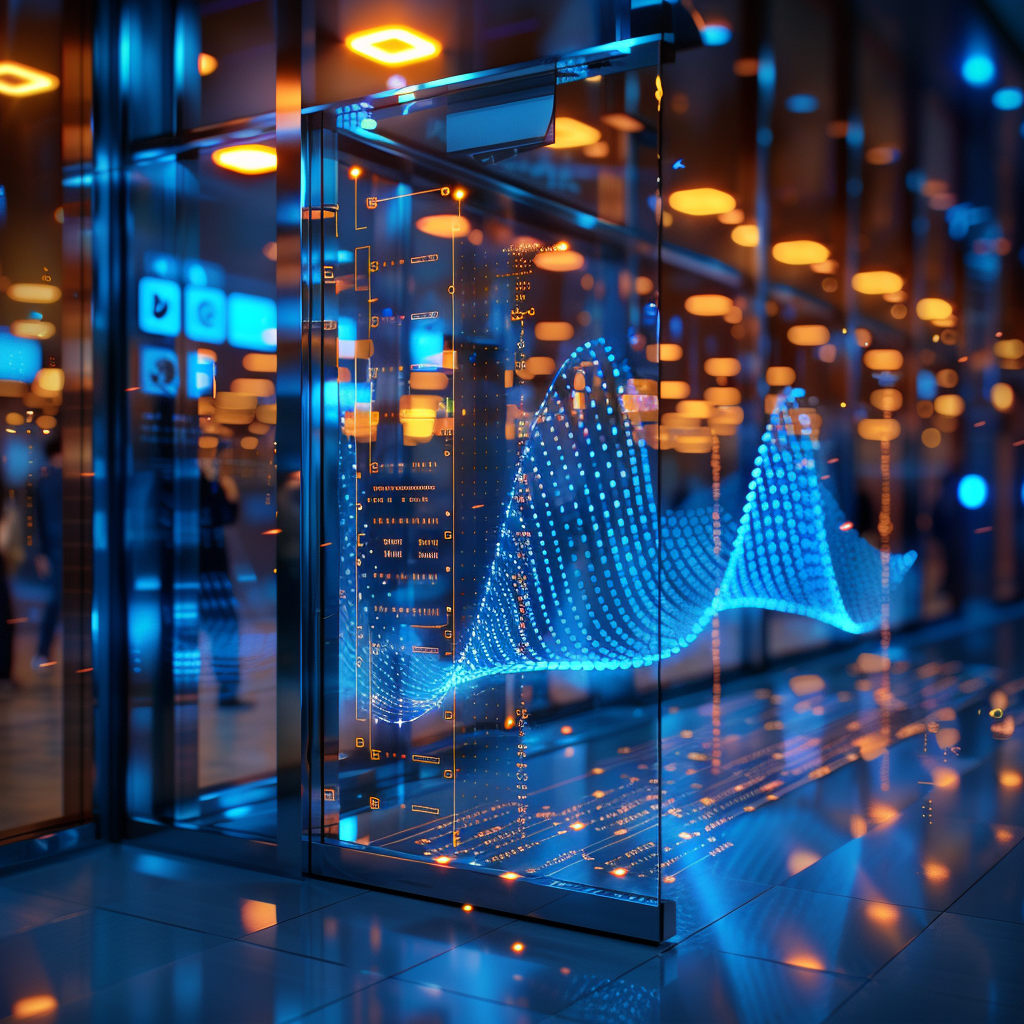
AI uses unique voice patterns for secure and convenient user authentication, leveraging speech recognition for biometric verification. This application is increasingly used in security-sensitive environments, offering a hands-free method of authentication that can be more secure and user-friendly than traditional passwords or physical biometrics.
8. Emotion Recognition
AI can detect nuances in tone and pitch to determine the speaker's emotional state, adding a layer of emotional intelligence to interactions.
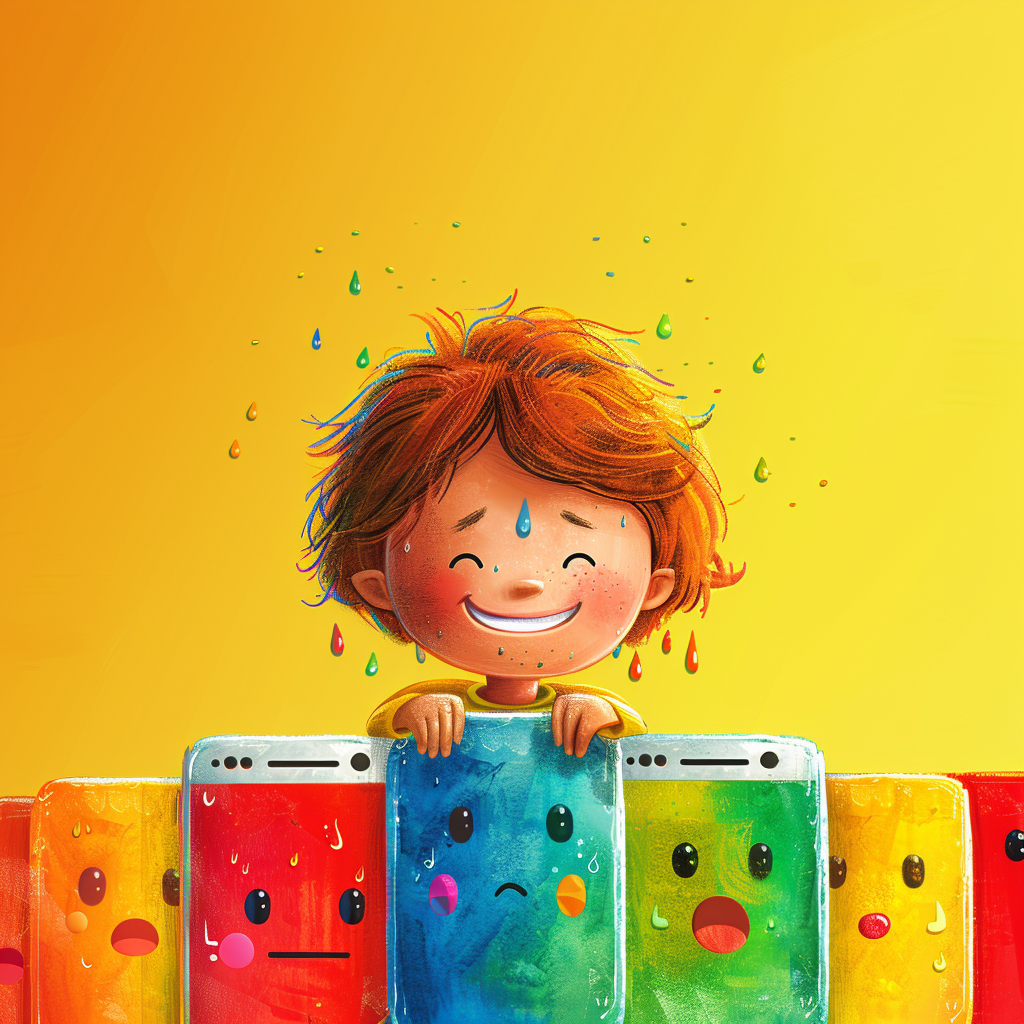
AI enhances speech recognition systems with the ability to detect subtle cues in the speaker’s tone and pitch, which can indicate their emotional state. This capability adds a layer of emotional intelligence to interactions, making AI systems more sensitive and responsive to the user's mood and potentially improving customer service interactions or therapy applications.
9. Multitasking Capabilities
AI enables speech recognition systems to handle multiple speakers simultaneously, distinguishing between different voices and attributing text accurately in conversations or meetings.
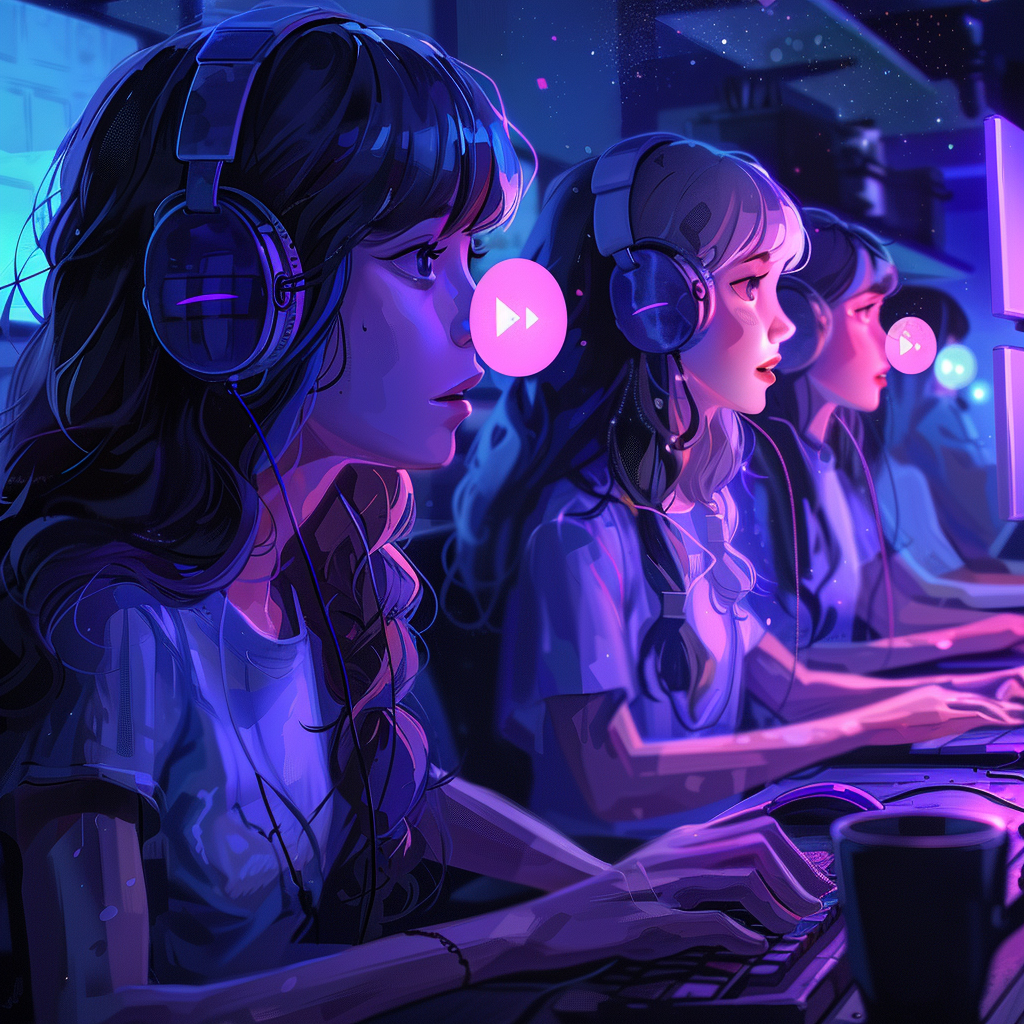
AI enables speech recognition systems to handle inputs from multiple speakers simultaneously, which is essential in scenarios like meetings or group discussions. These systems can distinguish between different voices and accurately attribute spoken text to the correct speaker, enhancing the functionality of transcription services and voice-driven systems in collaborative environments.
10. Continuous Learning and Adaptation
AI systems continuously learn from interactions, improving their accuracy and functionality over time by adapting to users’ speech patterns and preferences.
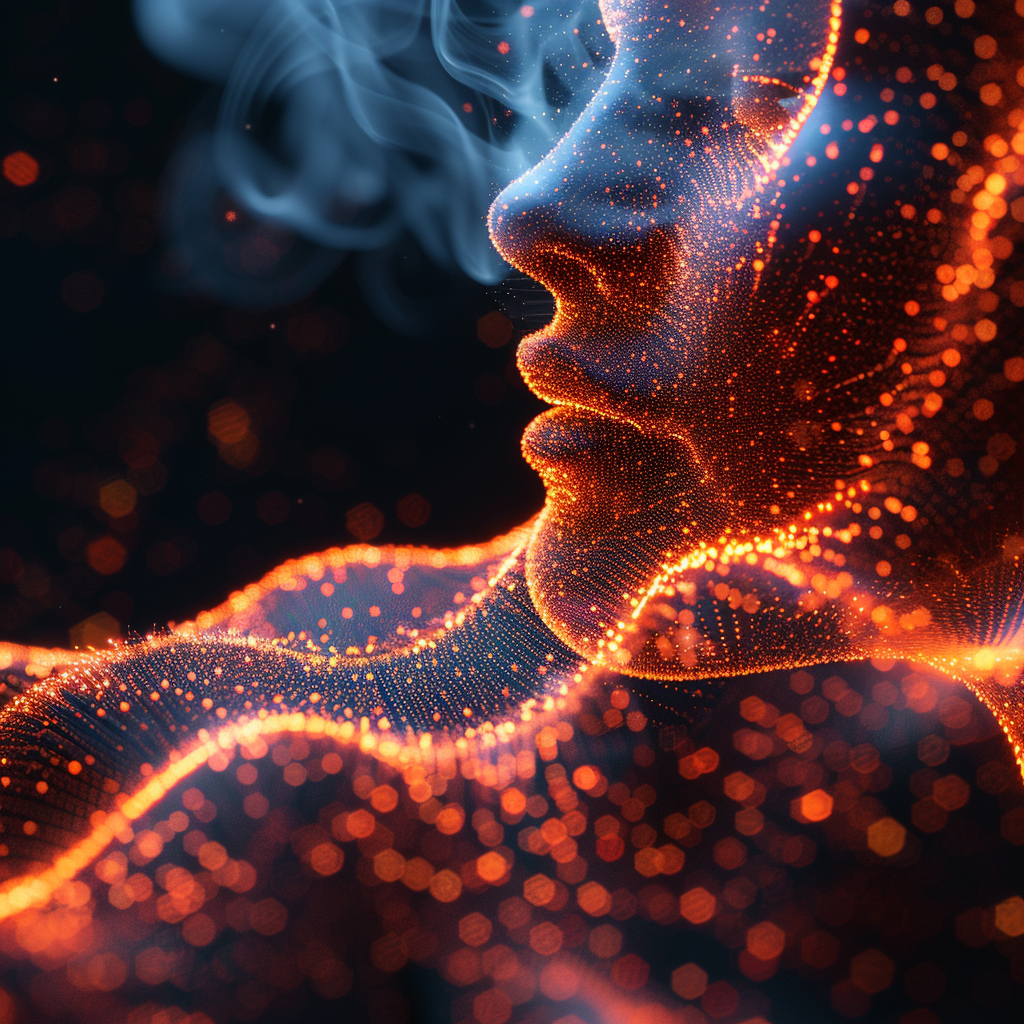
AI systems continually learn and improve from every interaction. By analyzing vast amounts of speech data and user feedback, AI models refine their ability to understand and process speech. This continuous learning allows speech recognition systems to adapt over time to new accents, slang, and evolving language use, ensuring they remain effective as they are used.