1. Autonomous Spacecraft Navigation
AI enables spacecraft to navigate autonomously through space, analyzing data from their environment to make real-time decisions about course adjustments without human intervention.
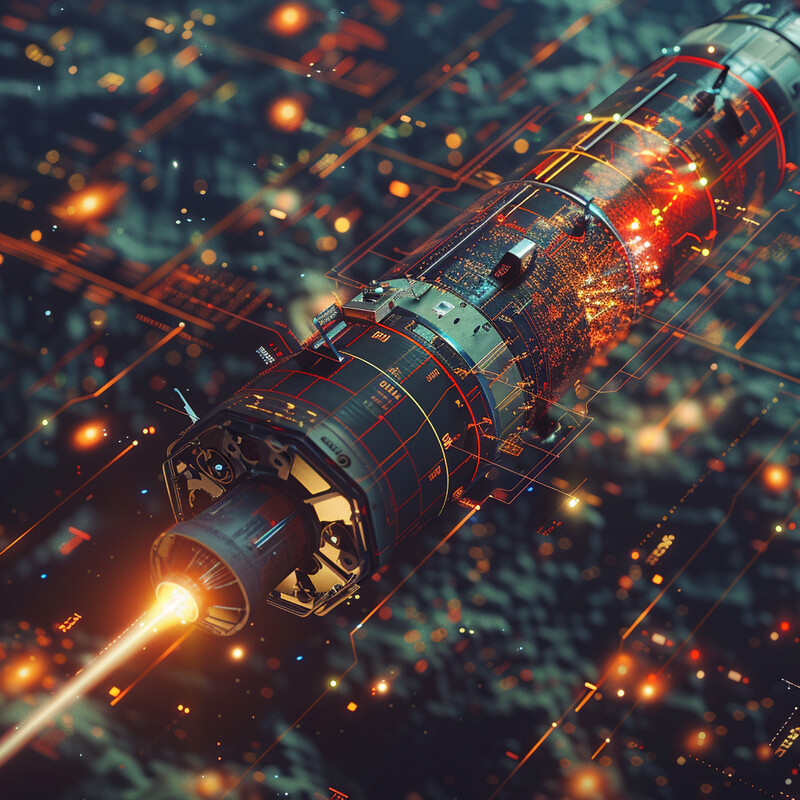
AI empowers spacecraft with advanced autonomous navigation capabilities, allowing them to make critical decisions about trajectory adjustments on the fly. Utilizing data from onboard sensors and instruments, AI algorithms process environmental inputs to dynamically navigate through space, avoiding obstacles and optimizing routes. This autonomy is crucial for missions far beyond Earth, where communication delays make real-time human oversight impractical.
2. Data Analysis from Space Telescopes
AI processes and analyzes vast amounts of data collected by space telescopes, helping to identify and categorize celestial objects like stars, galaxies, and exoplanets more efficiently.
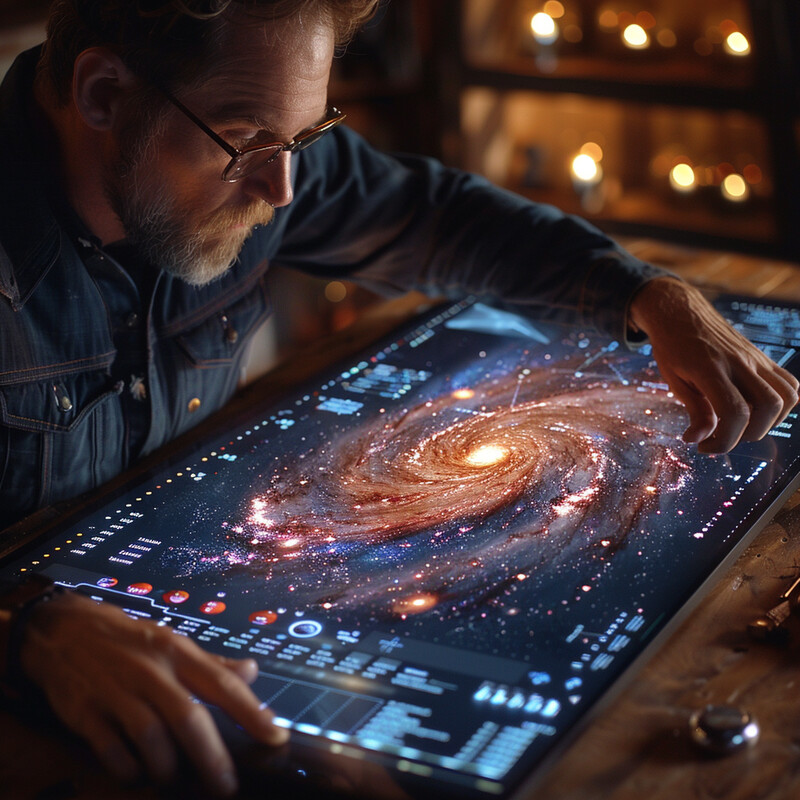
Space telescopes generate enormous volumes of astronomical data. AI helps process and analyze this information more efficiently, identifying and categorizing celestial objects, detecting distant galaxies, and spotting exoplanets. AI algorithms can sift through data to find patterns and signals that may be overlooked by human analysts, speeding up the discovery process and enhancing our understanding of the universe.
3. Rover Autonomy on Planetary Surfaces
AI equips planetary rovers with the capability to navigate and conduct scientific experiments on surfaces like Mars autonomously, optimizing paths and handling complex terrain.
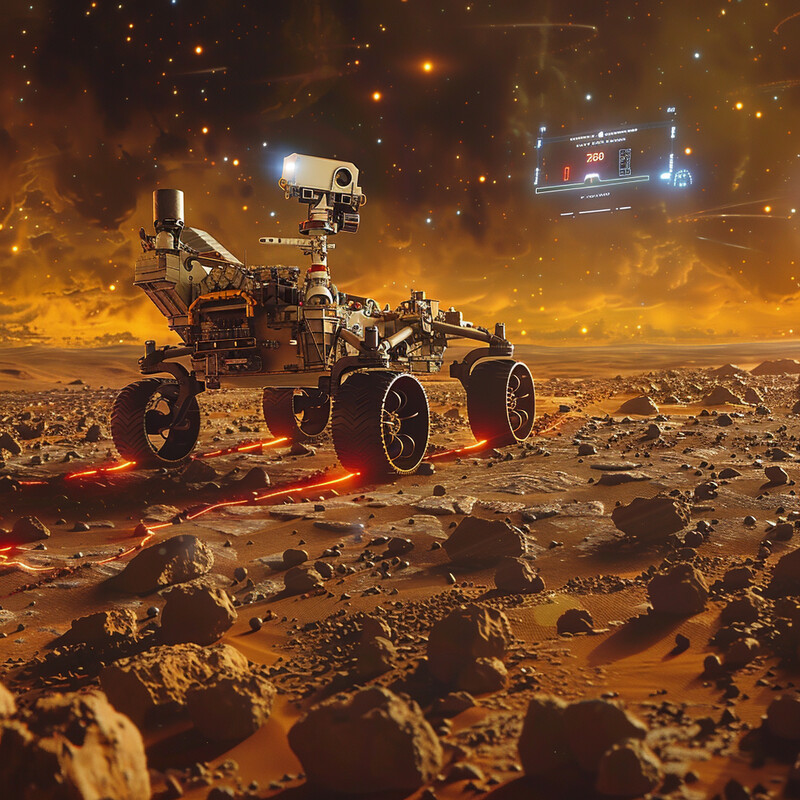
On planets like Mars, AI-equipped rovers navigate complex terrains autonomously, making intelligent path choices to avoid hazards and optimize scientific data collection. These rovers use AI to analyze soil samples, atmospheric conditions, and other environmental factors, making decisions that maximize their mission objectives while ensuring their operational safety.
4. Life Support Systems Management
AI monitors and manages life support systems on manned spacecraft, optimizing resource use such as air and water, and ensuring the systems adapt to changing conditions and crew needs.
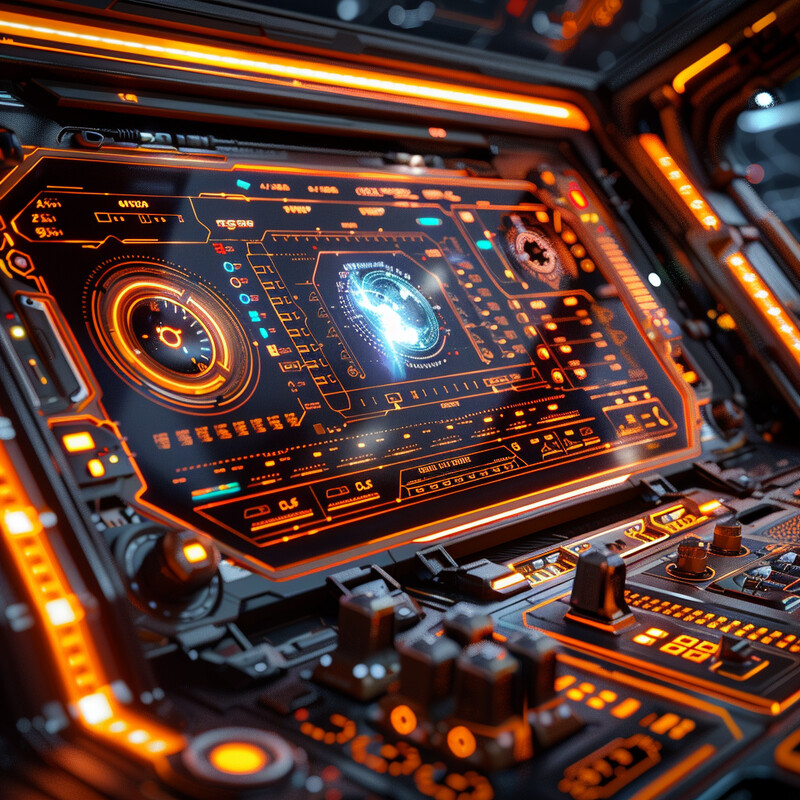
In manned space missions, AI plays a crucial role in managing life support systems, continuously monitoring variables like air quality, temperature, and water supply. By predicting consumption rates and adjusting resource distribution based on the crew's activities and the spacecraft's needs, AI ensures efficient use of vital resources, enhancing crew safety and comfort.
5. Predictive Maintenance for Spacecraft
AI predicts and schedules maintenance for spacecraft systems by analyzing performance data and detecting anomalies that could lead to failures, thus preventing issues before they occur.
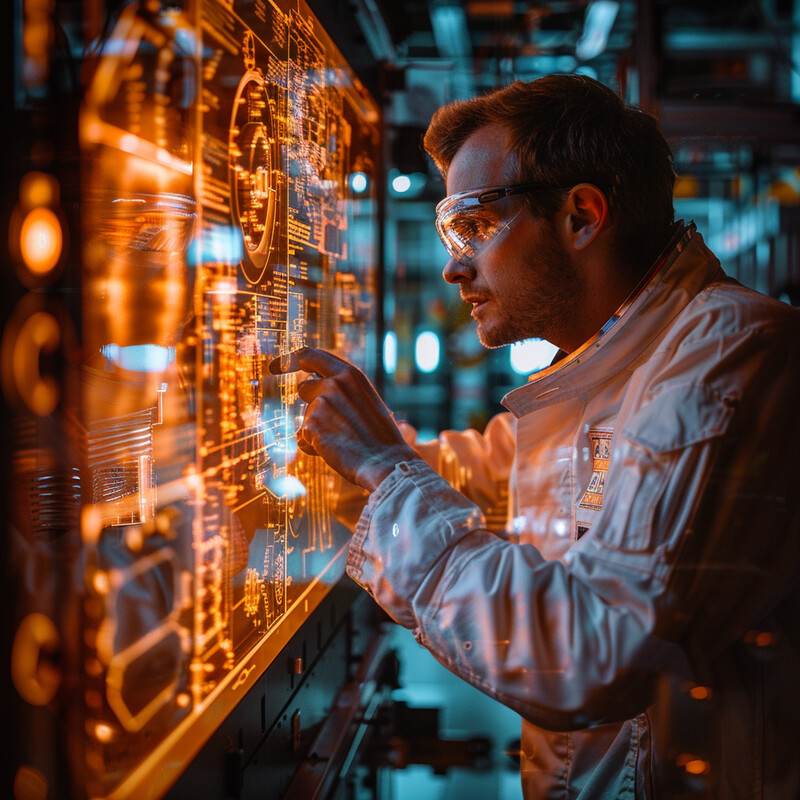
AI enhances spacecraft reliability through predictive maintenance, analyzing operational data to identify potential failures before they occur. This proactive approach allows mission controllers to perform maintenance or adjustments to avoid malfunctions, significantly reducing the risk of mission-compromising incidents.
6. Enhanced Communication Systems
AI improves communication between Earth and spacecraft, reducing delays and enhancing the quality of data transmission across vast distances.
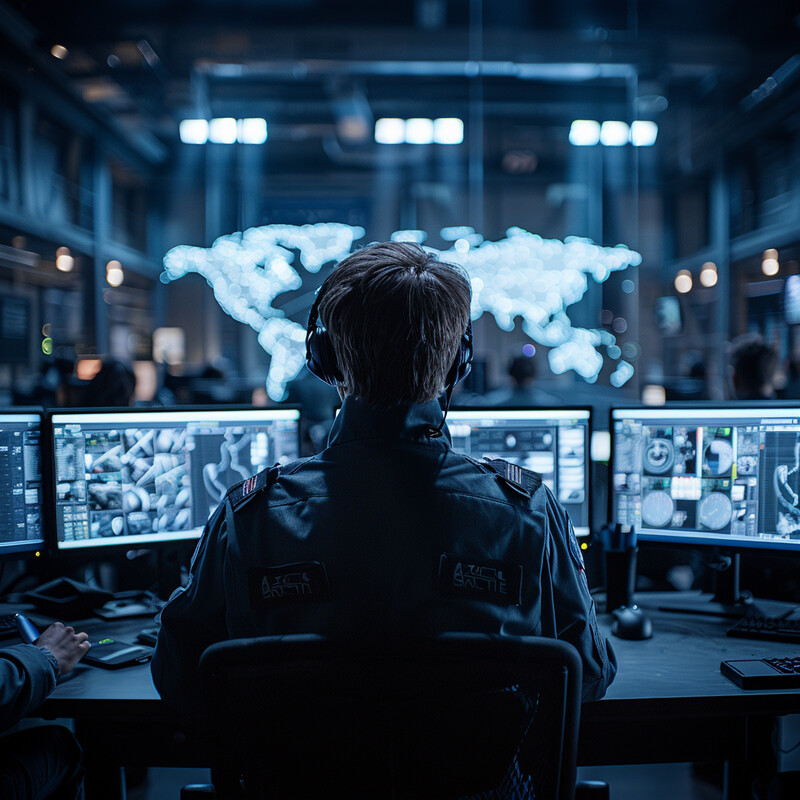
AI improves the efficiency and reliability of communications between spacecraft and Earth. By optimizing data transmission protocols and reducing signal degradation over vast distances, AI ensures that valuable scientific data and mission-critical communications are preserved and transmitted as efficiently as possible.
7. Astronaut Health Monitoring
AI systems monitor the health of astronauts in real-time, analyzing physiological data to provide early warnings of potential health issues and suggesting countermeasures.
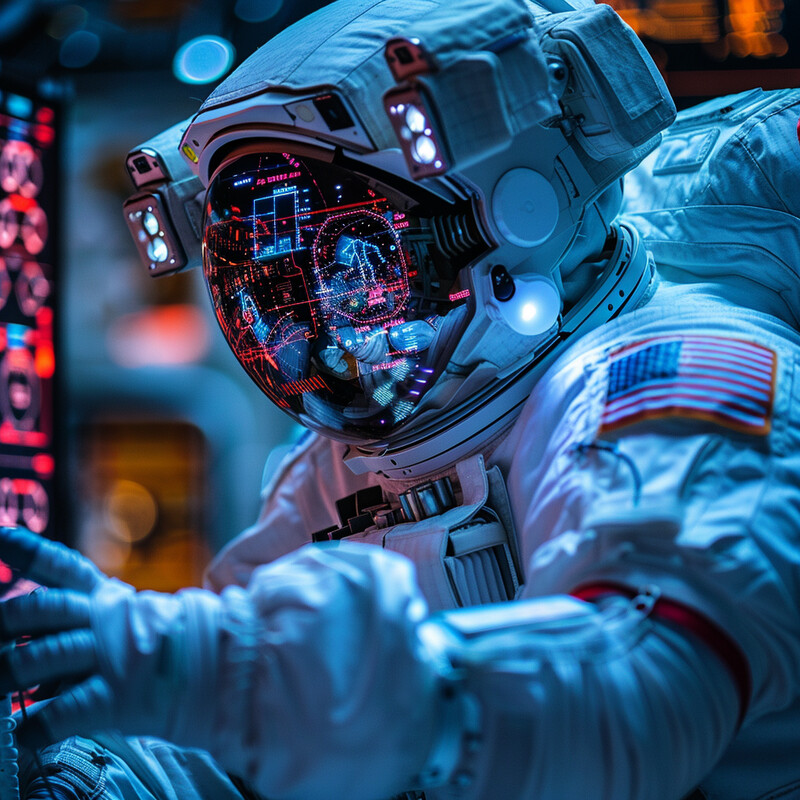
AI systems onboard spacecraft monitor the health of astronauts by analyzing physiological data in real-time. These systems can detect early signs of health issues, from changes in heart rate and blood pressure to more subtle indicators of stress or fatigue, providing early warnings and recommended interventions to maintain astronaut health.
8. Scientific Experimentation
AI assists in designing and conducting complex experiments in space by managing variables and analyzing results in real-time, thus maximizing scientific output.
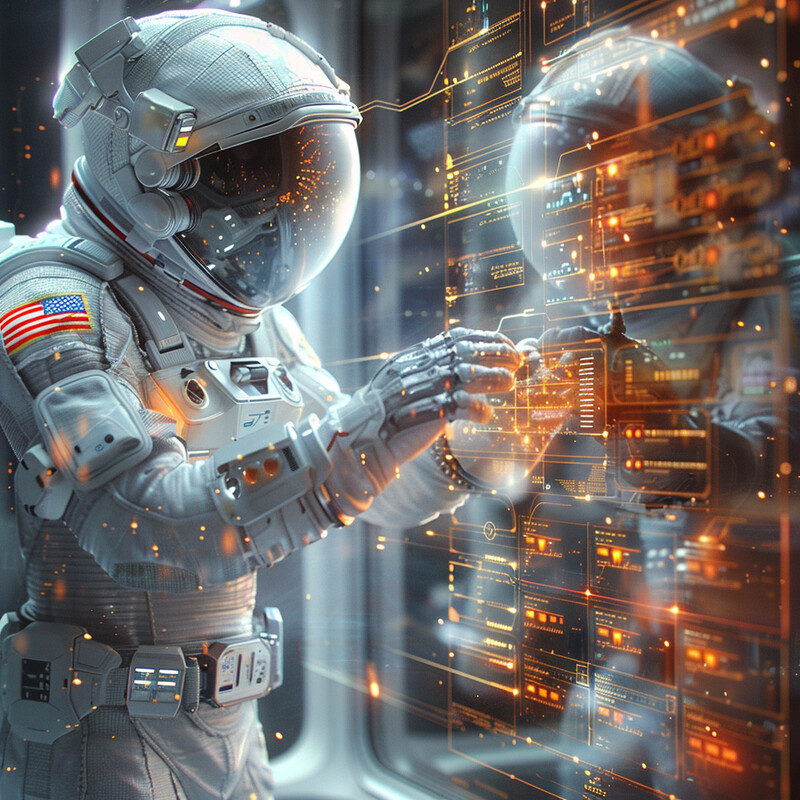
AI assists astronauts and scientists in conducting complex experiments in space. By managing experimental parameters and analyzing results in real time, AI systems help optimize research outcomes and adapt experimental approaches based on immediate data analysis, greatly increasing the scientific yield of space missions.
9. Mission Planning and Simulation
AI aids in mission planning by simulating various scenarios and outcomes, helping to choose optimal mission strategies and reduce potential risks.
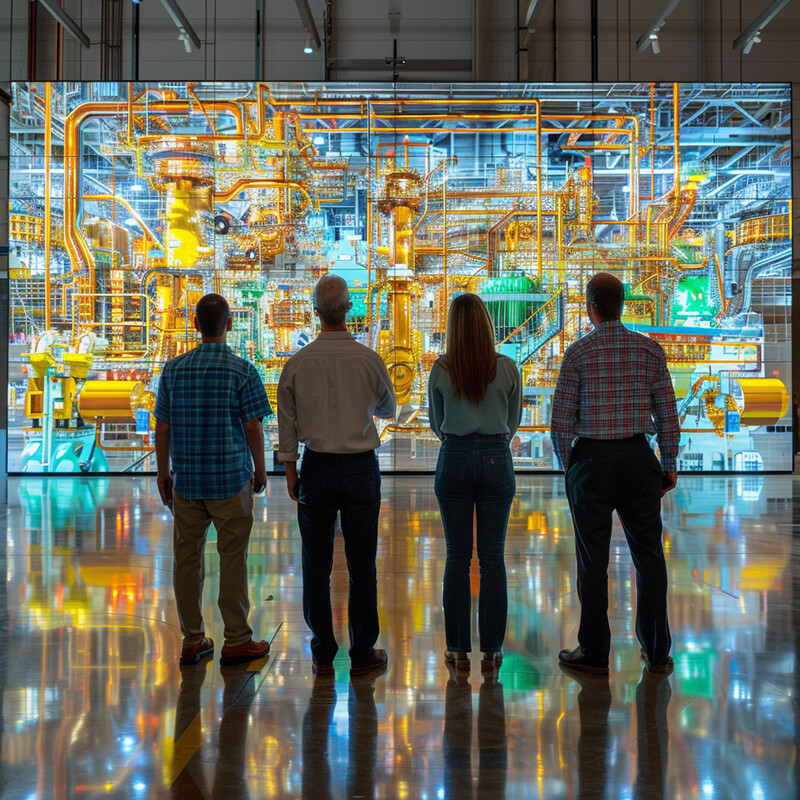
AI tools are used extensively in the planning stages of space missions, simulating various scenarios to predict outcomes and identify potential risks. By evaluating different strategies and mission plans, AI helps planners choose the most effective approaches, ensuring the highest chances of mission success.
10. Space Debris Tracking and Avoidance
AI tracks and predicts the paths of space debris to help spacecraft avoid collisions, ensuring the safety and longevity of space missions.
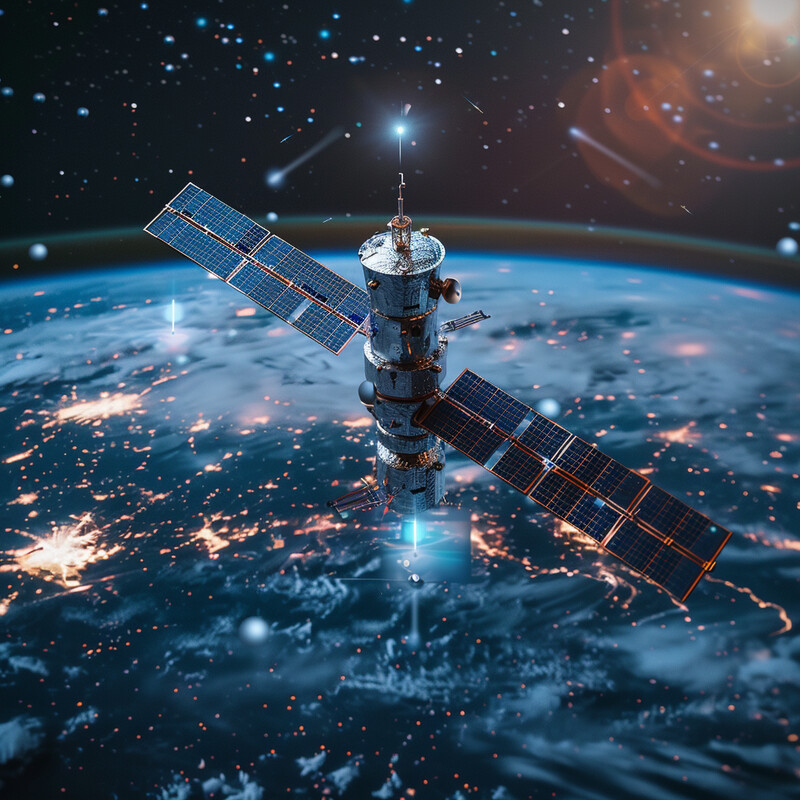
AI is crucial in tracking and predicting the trajectories of space debris. These systems provide real-time updates and avoidance maneuvers to spacecraft, ensuring that satellites and other space assets do not collide with debris, which could be catastrophic. This capability is essential for safeguarding current and future space missions against the growing threat of orbital debris.