1. Demand Forecasting
AI algorithms predict electricity demand in real-time, allowing grid operators to adjust power supply efficiently and ensure reliability without overproducing energy.
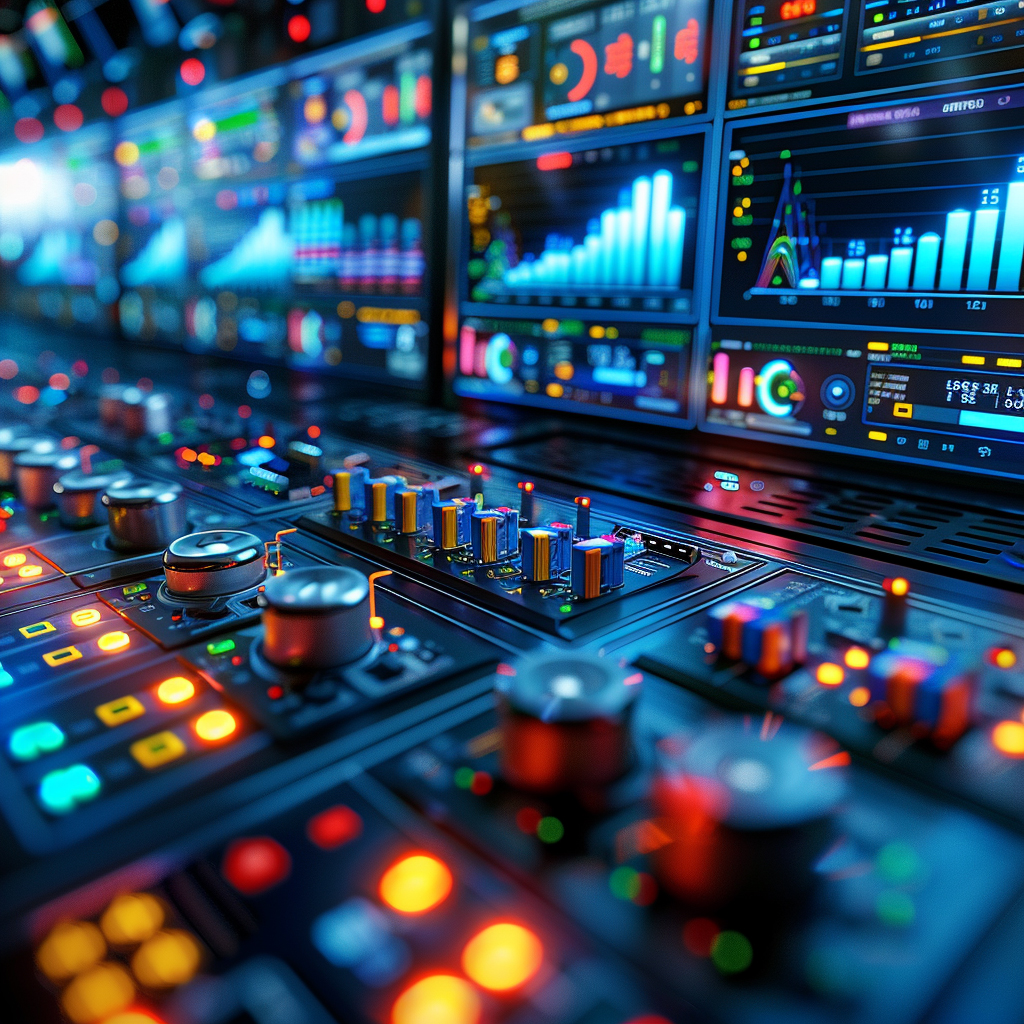
AI algorithms excel at predicting electricity demand by analyzing historical consumption data along with variables like weather conditions, time of day, and economic activity. This precise forecasting allows grid operators to adjust the supply dynamically, ensuring energy is available when needed without excessive production. This capability is critical for optimizing resource allocation and reducing operational costs.
2. Grid Stability and Reliability
AI monitors and analyzes grid performance continuously, detecting and responding to fluctuations, faults, or failures instantly to maintain stability and prevent outages.
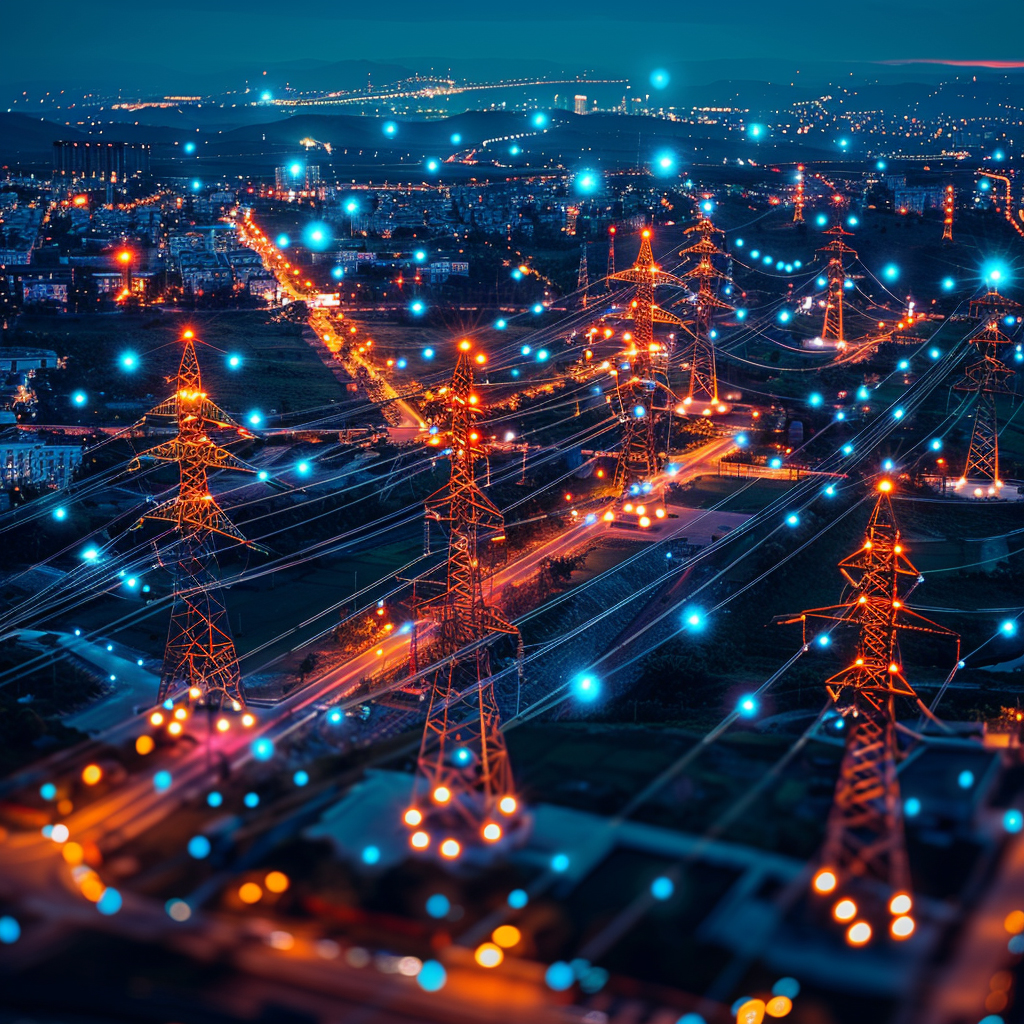
AI continuously monitors the health and performance of the electrical grid by analyzing data from sensors and IoT devices distributed throughout the system. It can detect and respond to anomalies, fluctuations, or potential failures in real time. This immediate responsiveness helps prevent power outages and ensures a stable and reliable supply of electricity, enhancing the resilience of the grid.
3. Renewable Energy Integration
AI optimizes the integration of renewable energy sources like solar and wind into the power grid by predicting variability and adjusting grid operations accordingly to handle intermittent energy flows.
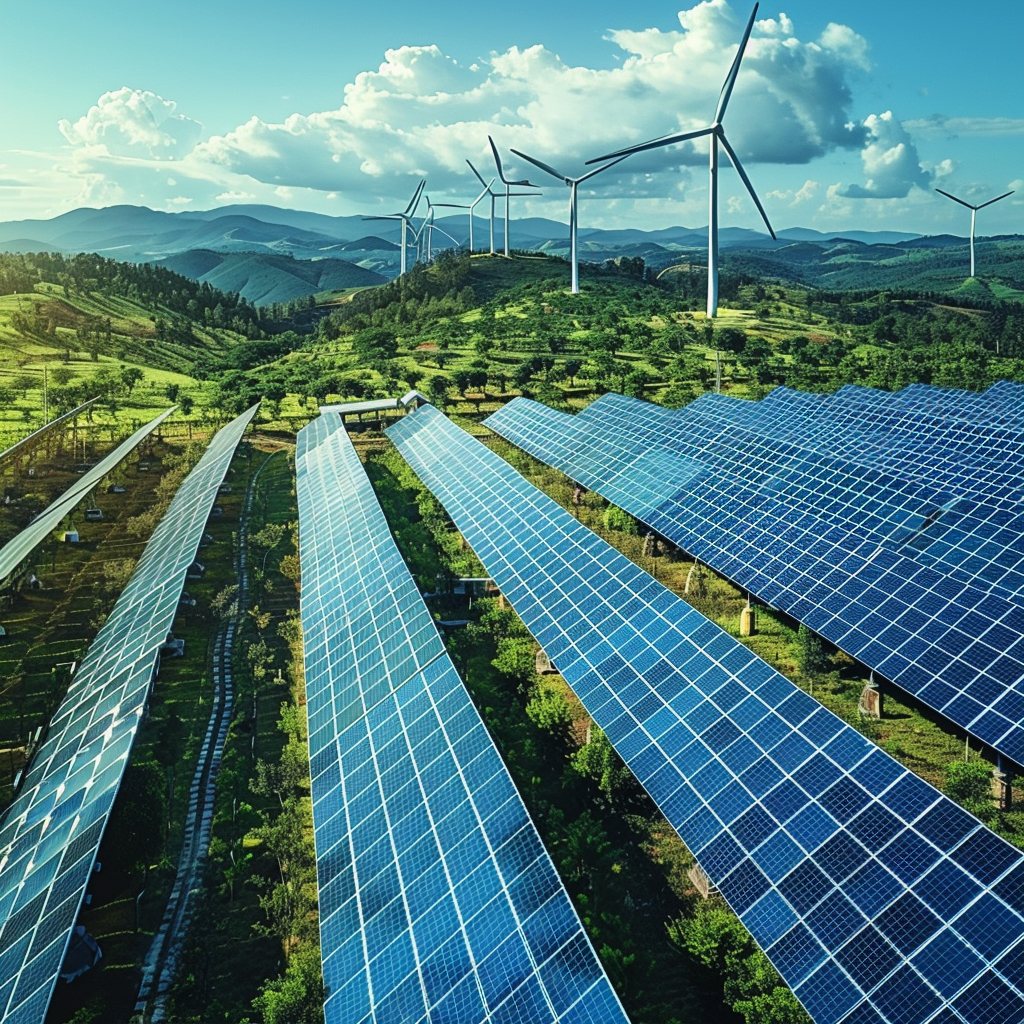
Integrating renewable energy sources into the power grid presents challenges due to their intermittent nature. AI helps manage this by predicting fluctuations in wind and solar energy production and adjusting grid operations to accommodate these changes. This optimization ensures a smooth and efficient integration of renewable sources, maximizing their utility while maintaining grid stability.
4. Predictive Maintenance
AI analyzes data from various sensors across the grid to predict equipment failures before they occur, scheduling maintenance to prevent downtime and extend the life of grid components.
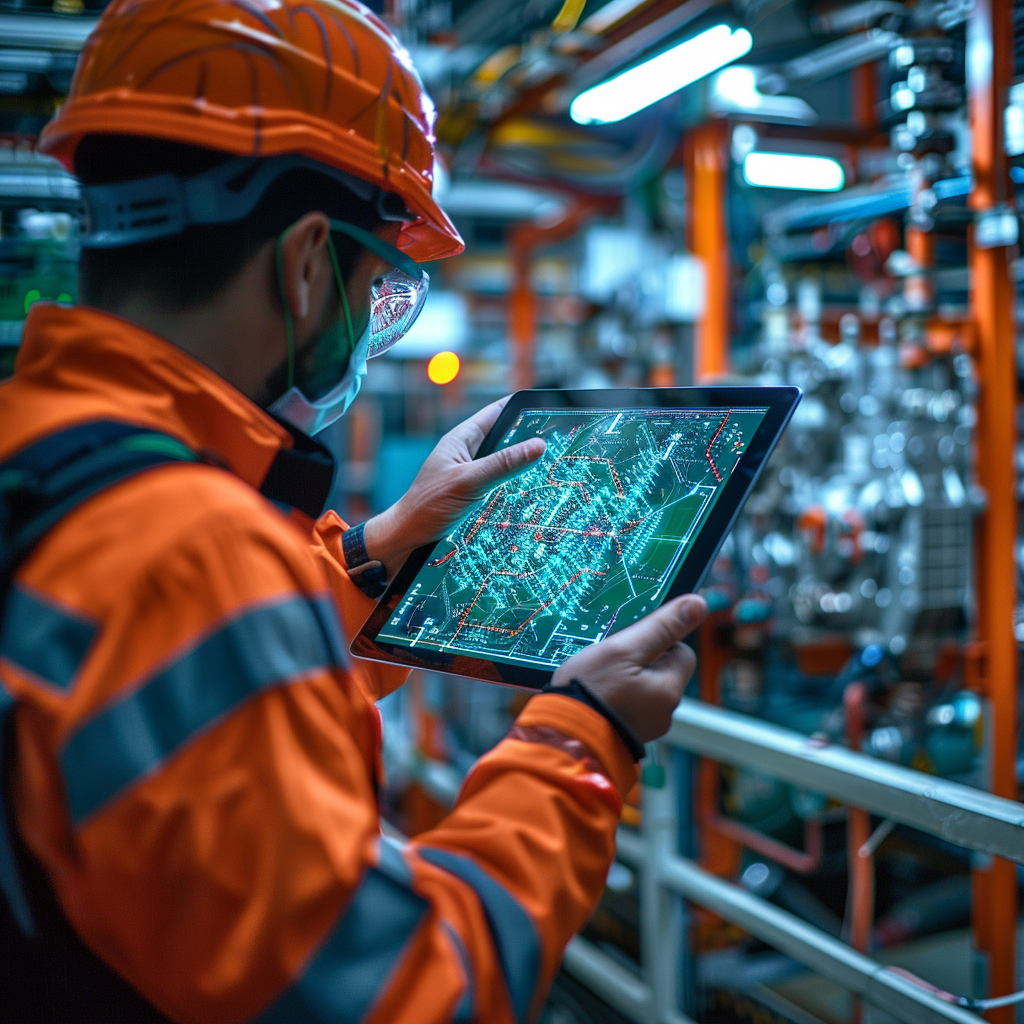
AI analyzes data from grid infrastructure to predict when and where maintenance is needed before failures occur. By identifying potential issues in advance, maintenance can be scheduled proactively during off-peak times, minimizing disruptions and extending the lifespan of grid components. This predictive approach significantly reduces unexpected downtime and maintenance costs.
5. Energy Efficiency Optimization
AI enhances energy efficiency by dynamically adjusting the flow and distribution of power based on real-time usage data, helping to reduce wastage and improve overall energy management.
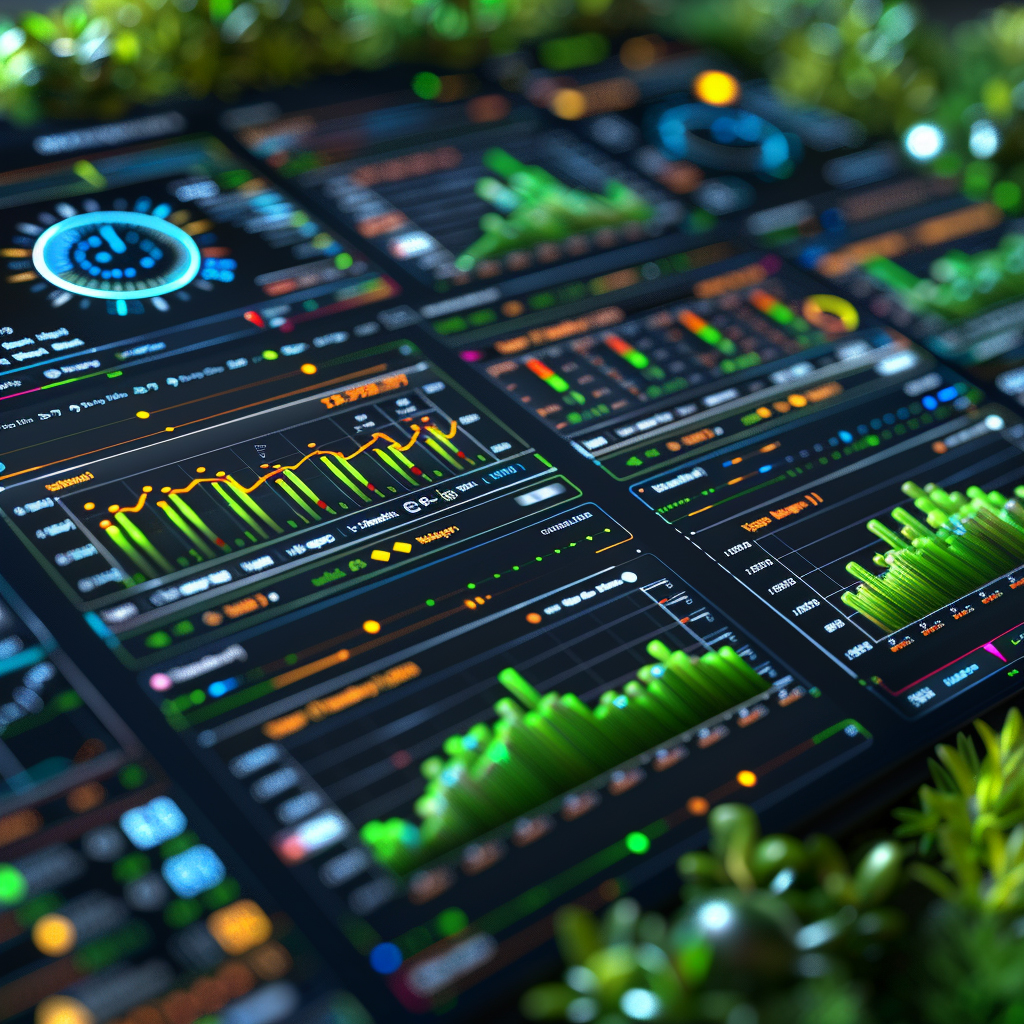
AI optimizes the distribution and flow of electricity based on real-time usage patterns across the grid. By dynamically adjusting energy distribution, AI reduces wastage and improves the overall efficiency of the power grid. This not only conserves energy but also lowers operational costs and reduces environmental impact.
6. Peak Load Management
AI systems manage and alleviate peak load pressures by predicting high usage periods and deploying strategies such as demand response or energy storage solutions to balance the load.
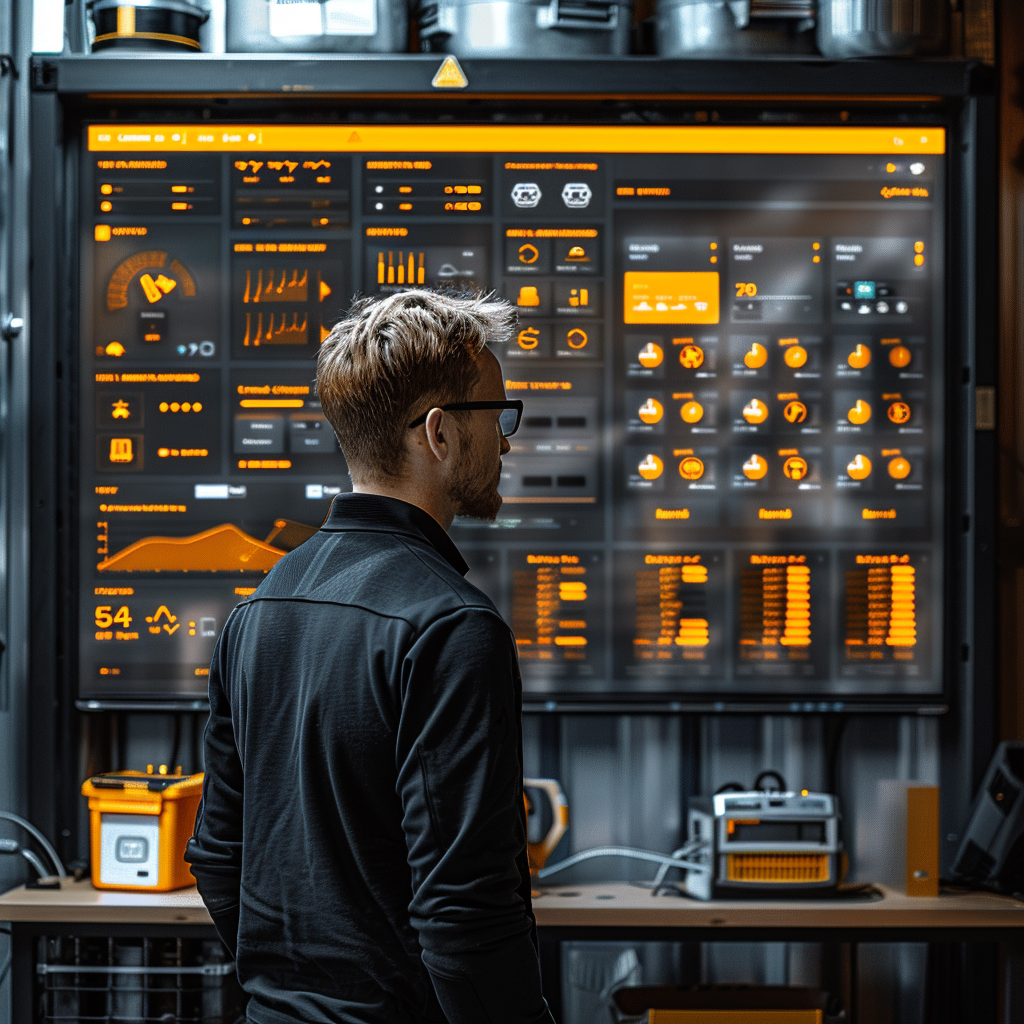
During periods of high electricity demand, AI systems can predict peak loads and implement strategies to manage the pressure on the grid. Techniques such as demand response (adjusting prices or incentivizing reduced consumption) and activating energy storage solutions help balance the load, ensuring the grid operates efficiently without the need for costly infrastructure expansions.
7. Real-Time Pricing
AI enables real-time energy pricing based on supply and demand dynamics, encouraging consumers to use energy during off-peak hours, thus helping balance the grid and reduce energy costs.
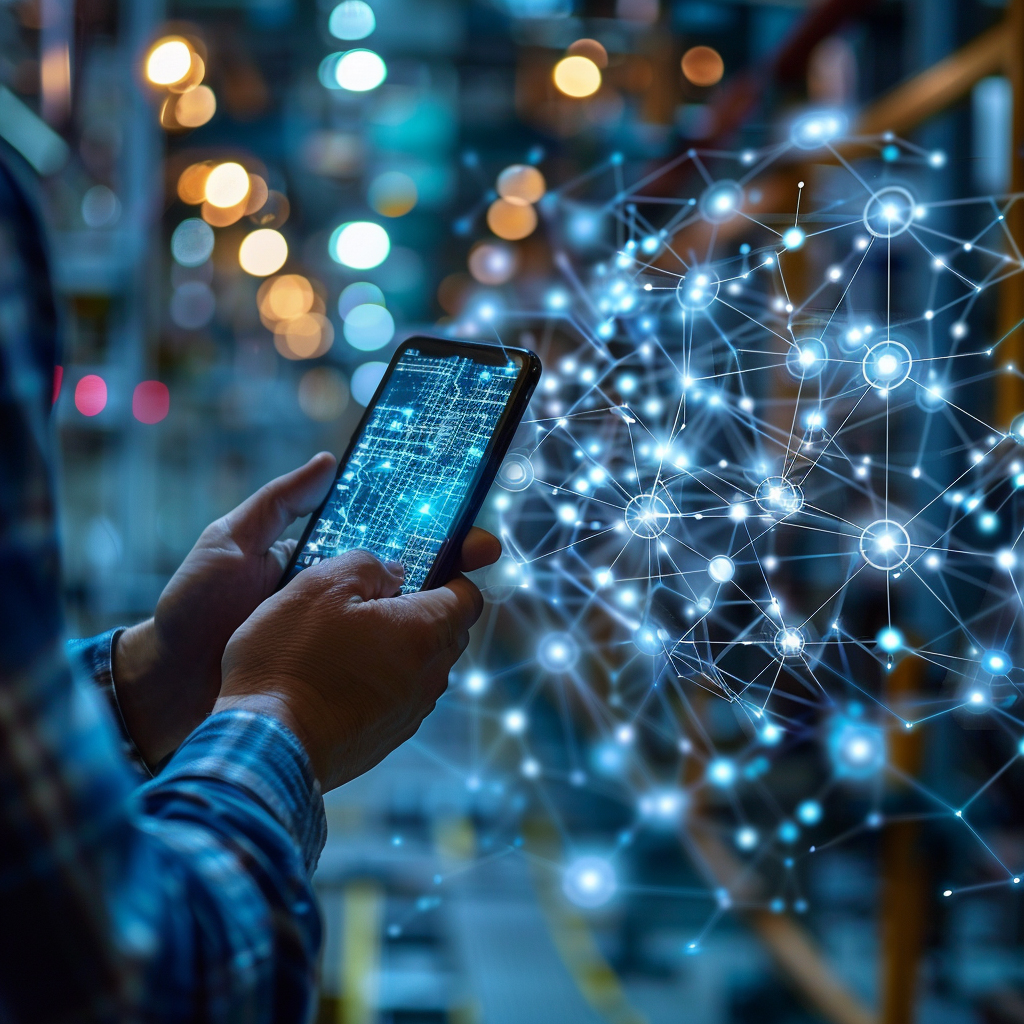
AI enables dynamic pricing models that reflect real-time changes in electricity supply and demand. This pricing strategy encourages consumers to use power during off-peak hours, balancing the load on the grid and potentially lowering energy costs. Consumers benefit from cheaper electricity rates during these times, while utilities can manage the grid more effectively.
8. Cybersecurity
AI enhances the cybersecurity of smart grids by continuously monitoring network activities and detecting anomalies or potential cyber threats quickly, ensuring the protection of critical infrastructure.
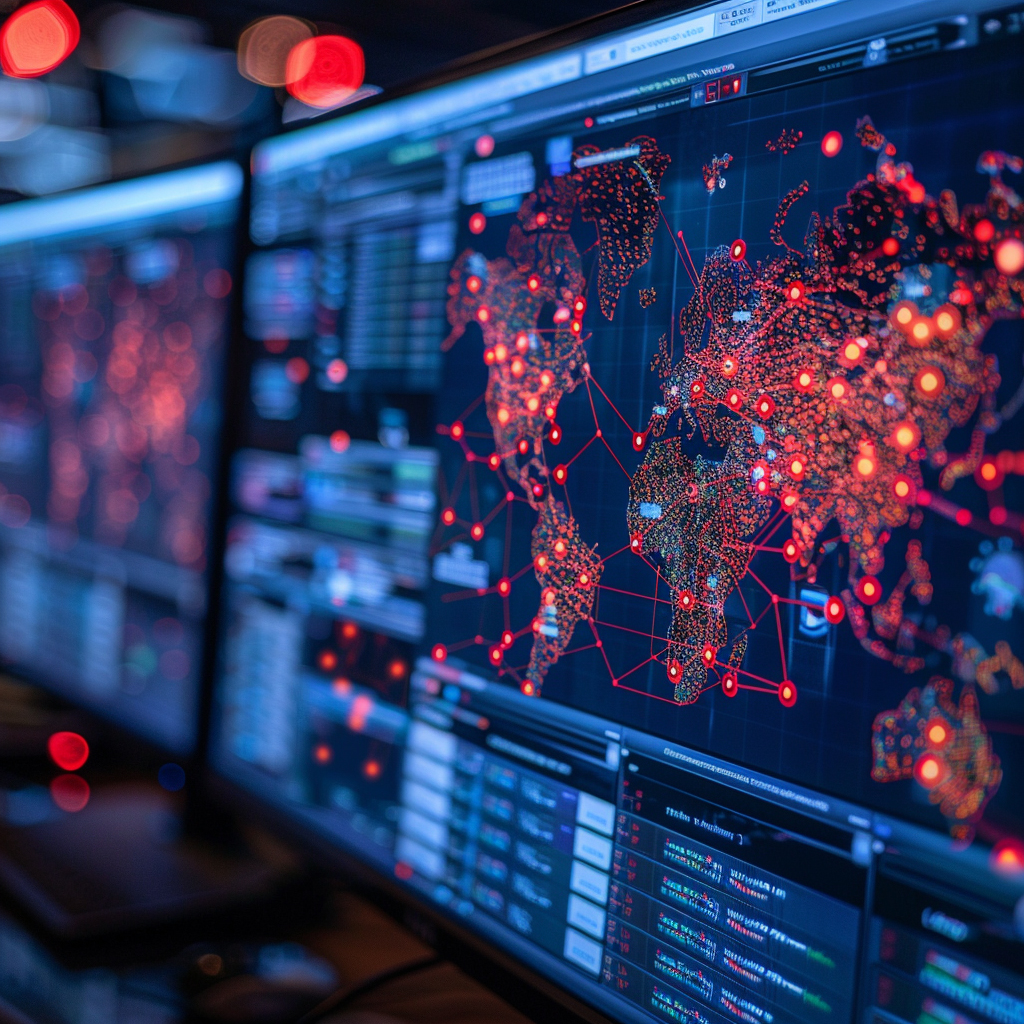
With smart grids becoming increasingly digital and connected, cybersecurity is a major concern. AI enhances the security of these systems by continuously monitoring for unusual network activity that could indicate a cybersecurity threat. Quick detection allows for immediate responses, safeguarding critical infrastructure from potential breaches.
9. Energy Storage Optimization
AI optimizes the charging and discharging cycles of energy storage systems like batteries, improving their efficiency and lifespan while ensuring they are used effectively during peak periods or when renewable generation is low.
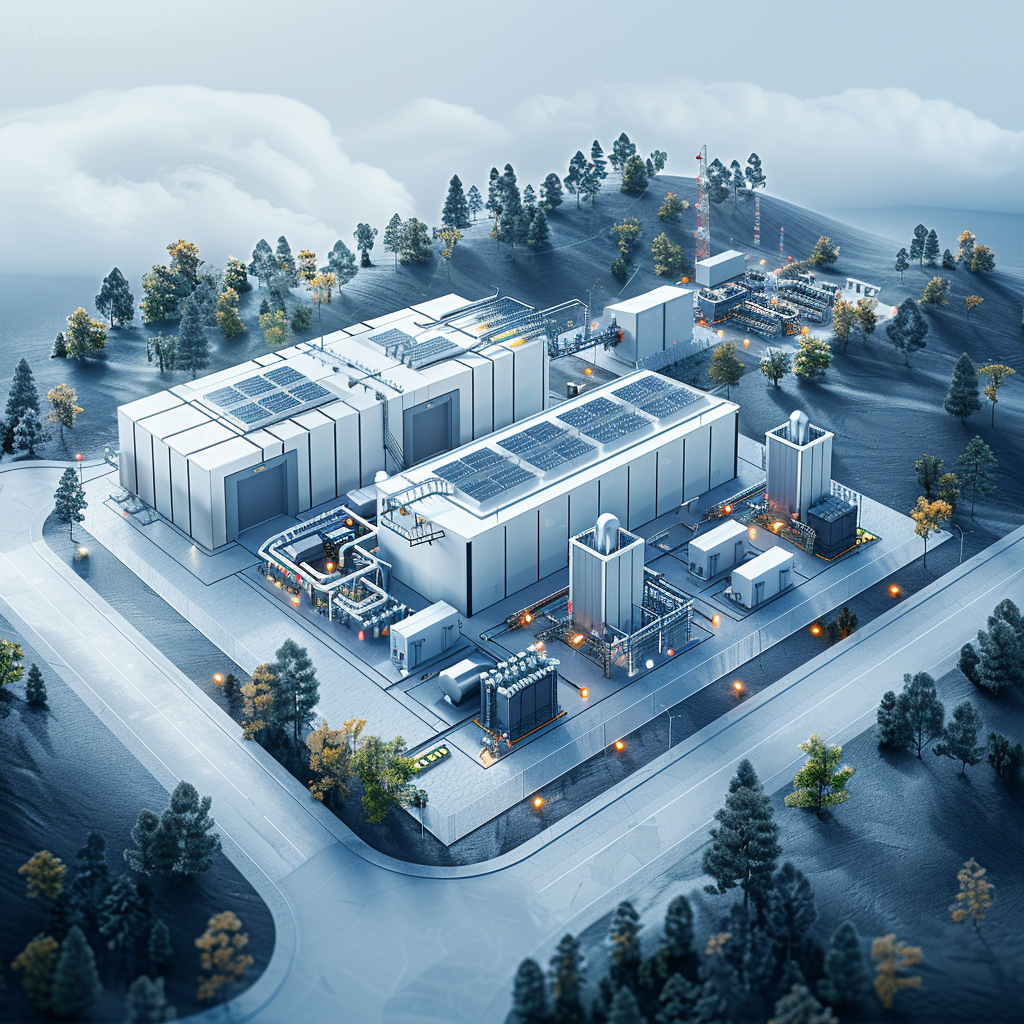
AI optimizes the operation of energy storage systems within the grid, such as battery banks. It manages the charging and discharging processes based on predictions of demand peaks and dips, renewable energy output, and other relevant factors. This ensures that energy storage is used efficiently, enhancing the grid's ability to handle load variations and maintain stability.
10. Customer Engagement and Interaction
AI facilitates better customer interaction with the smart grid through personalized energy consumption feedback and tailored recommendations for energy saving, enhancing user engagement and satisfaction.
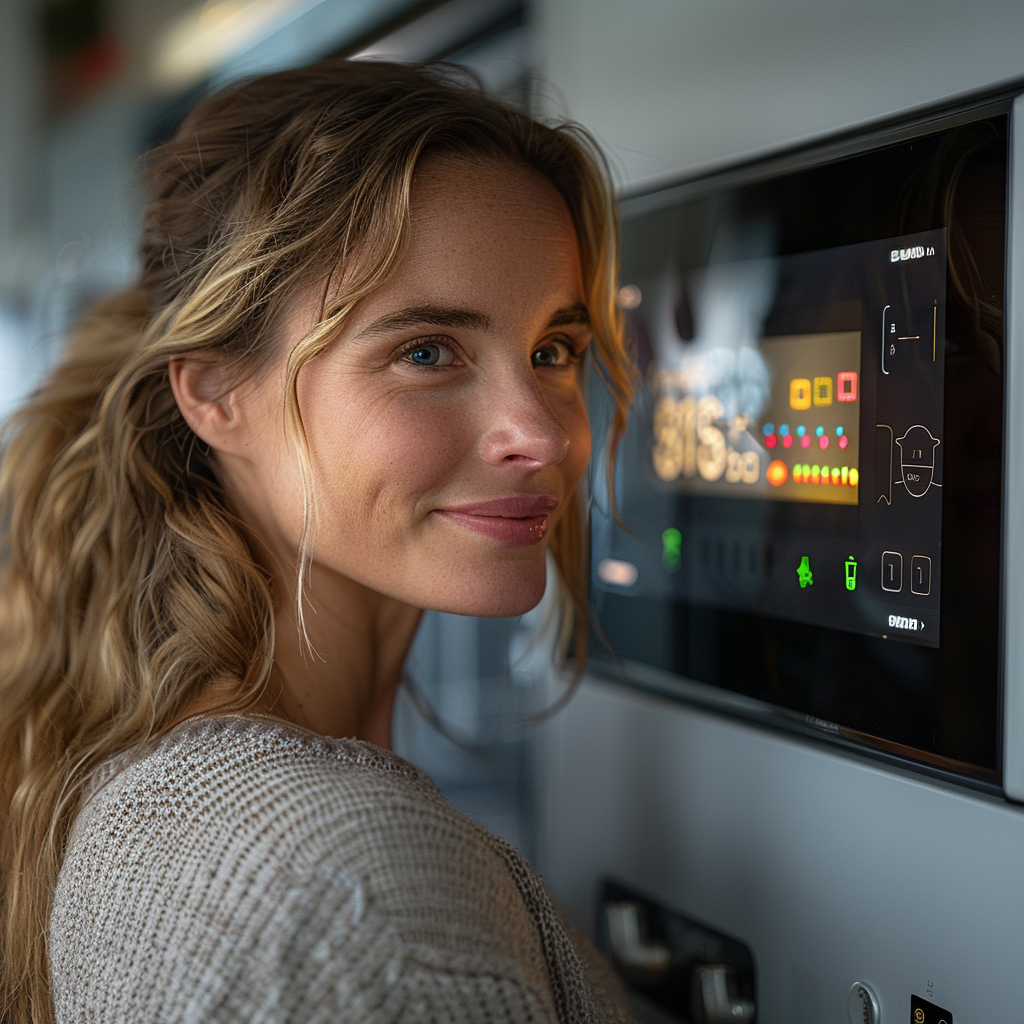
AI transforms how consumers interact with the smart grid through personalized energy usage analytics and recommendations for reducing consumption. This not only helps consumers save money but also promotes energy conservation and efficiency. Enhanced customer engagement also fosters a better understanding and appreciation of energy usage impacts, leading to more sustainable behaviors.