1. Automated Design Iterations
AI automates the generation of multiple design iterations quickly, allowing designers to explore a wider array of options and refine their concepts based on feedback and performance metrics.
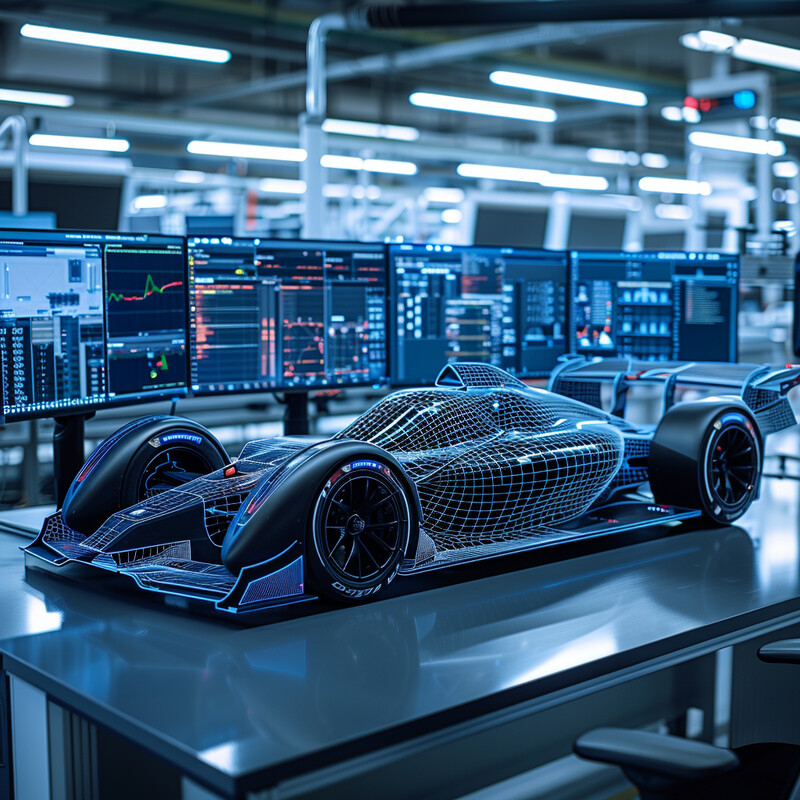
AI enables rapid generation of multiple design iterations by automating the modification and testing of design parameters. This capability allows designers to quickly explore a broad spectrum of design alternatives and optimize based on aesthetic, functional, and compliance criteria. By streamlining the iteration process, AI significantly reduces the time and effort involved in reaching the final design.
2. Enhanced User Experience Design
AI analyzes user behavior data to create more intuitive and user-friendly designs. It helps in understanding how users interact with products, facilitating improvements in user interface and experience.
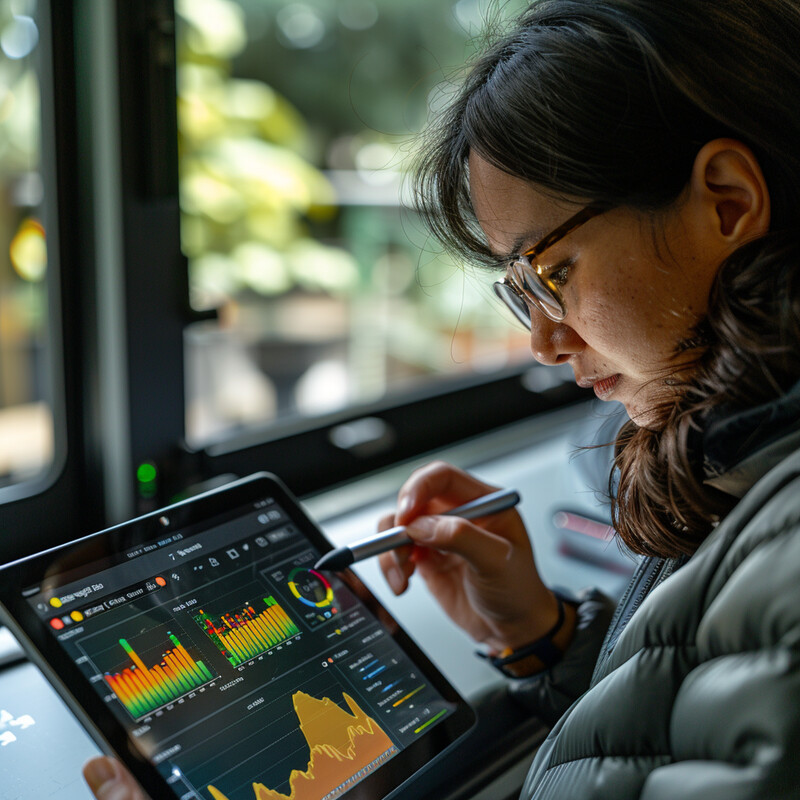
Utilizing user interaction data, AI analyzes how users engage with products and identifies patterns that could inform design improvements. This insight helps in creating more intuitive interfaces and user-friendly designs that cater directly to user preferences and behaviors, ultimately enhancing the overall user experience.
3. Material Optimization
AI assists in selecting optimal materials for different parts of a product by analyzing factors like durability, cost, sustainability, and weight, ensuring the best combination for performance and manufacturability.
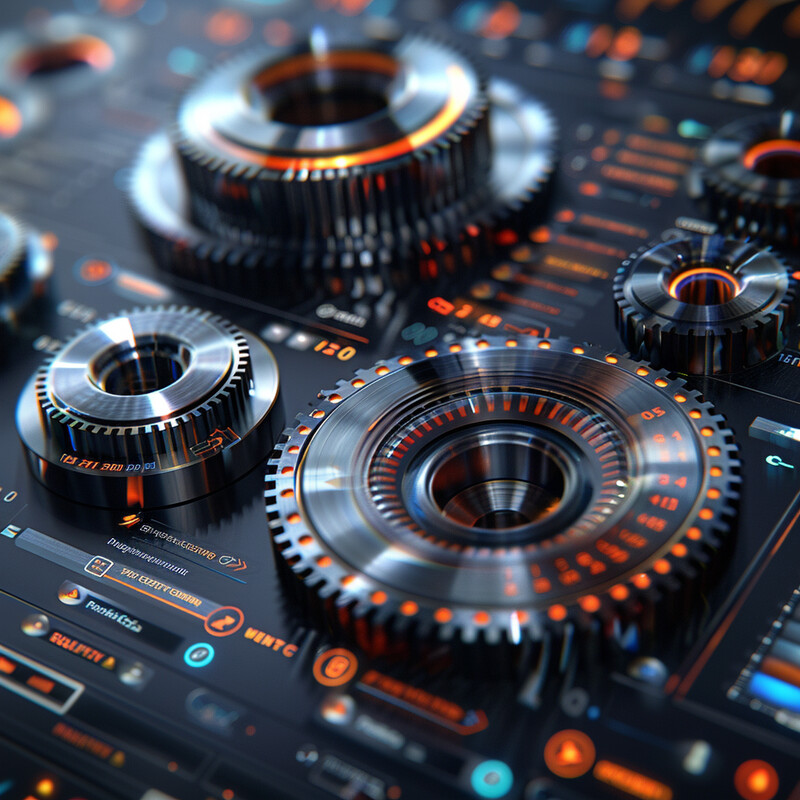
AI tools assess various materials based on properties such as strength, flexibility, cost, and environmental impact to recommend the best options for specific product applications. This optimization ensures that products not only meet the required performance standards but are also cost-effective and sustainable.
4. Predictive Analytics for Market Trends
AI predicts future trends in design and consumer preferences, enabling designers to create products that meet future market demands and stay ahead of competition.
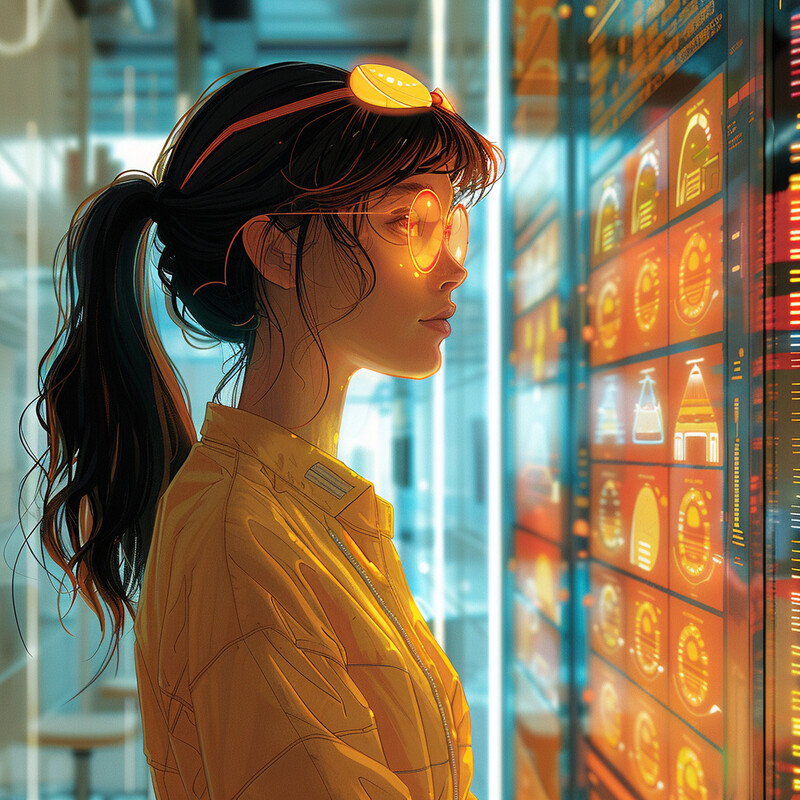
AI predicts future market trends by analyzing current data on consumer preferences, industry innovations, and socio-economic factors. This foresight allows designers to anticipate shifts in consumer demands and integrate emerging trends into product design early, giving companies a competitive edge.
5. Customization and Personalization
AI enables mass customization of products by allowing individual consumers to tailor aspects like color, size, and features to their preferences, all within an automated system that integrates seamlessly with production lines.
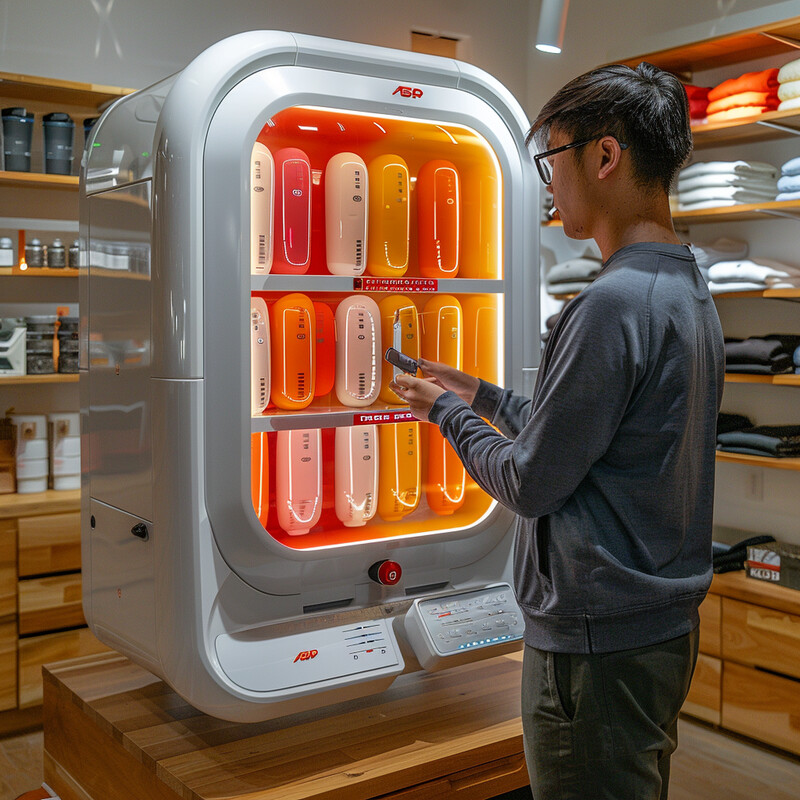
AI facilitates the mass customization of products by allowing consumers to personalize aspects such as design, color, and features through an interactive interface. AI systems process these individual preferences and adjust manufacturing instructions accordingly, enabling personalized production at scale.
6. Integration with Virtual Reality
AI integrates with VR to create immersive design environments where designers can interact with their creations in three-dimensional space, enhancing the understanding of how a product feels and functions before it is built.
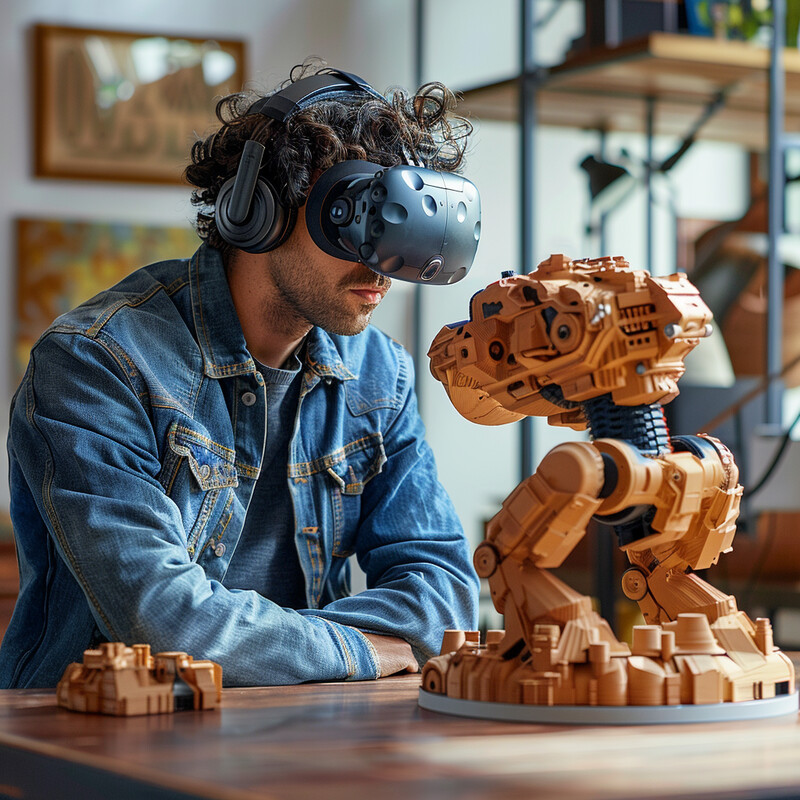
AI enhances virtual reality platforms used in product design by enabling more realistic and interactive simulations. Designers can use VR to visualize and interact with their models in a fully immersive environment, making it easier to evaluate the design's look and feel in a real-world context before proceeding with physical prototypes.
7. Real-Time Collaboration
AI facilitates real-time collaboration among global design teams by synchronizing design changes and communications, ensuring that all team members are updated and can contribute efficiently.
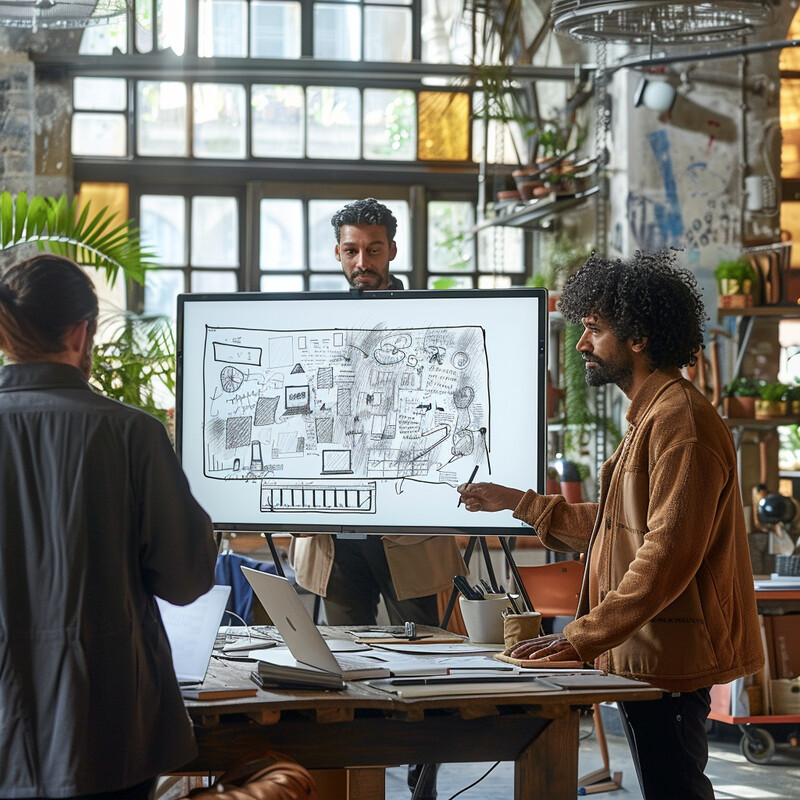
AI supports real-time collaboration among distributed design teams by synchronizing design modifications and communications across different locations and time zones. This ensures that all team members can work together seamlessly, sharing insights and updates instantaneously, which is crucial for complex projects involving multiple stakeholders.
8. Environmental Impact Analysis
AI evaluates the environmental impact of different design choices, helping designers select options that minimize carbon footprint and waste throughout the product lifecycle.
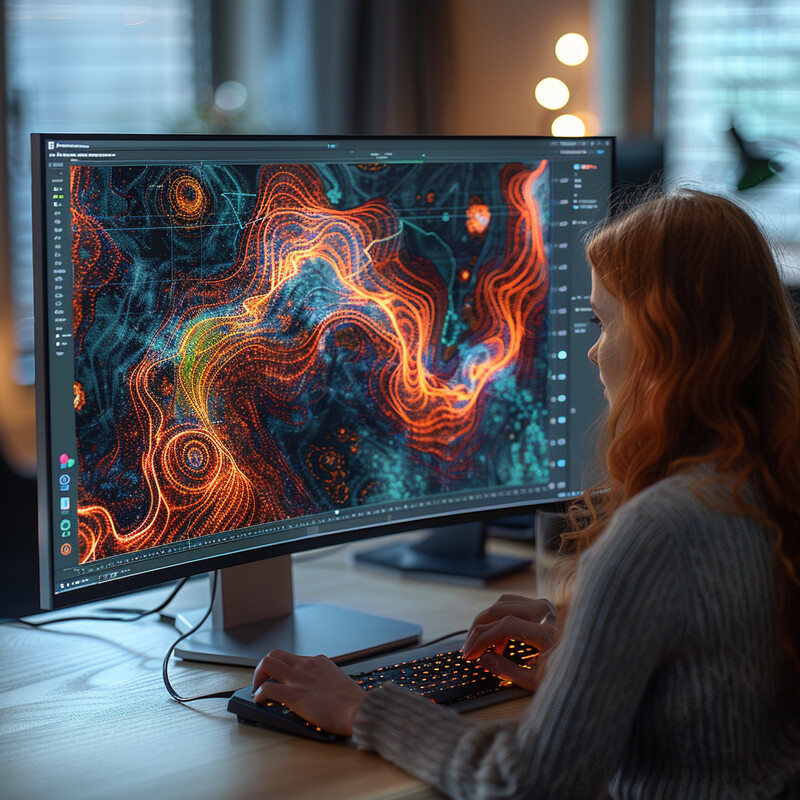
AI evaluates the environmental implications of design choices throughout the product lifecycle, from material selection to disposal. By analyzing data related to energy consumption, emissions, and recyclability, AI helps designers make informed decisions that align with sustainability goals.
9. Design for Manufacturing Optimization
AI analyzes product designs for manufacturability, identifying potential production issues and suggesting design modifications to optimize manufacturing processes, reduce costs, and improve product quality.
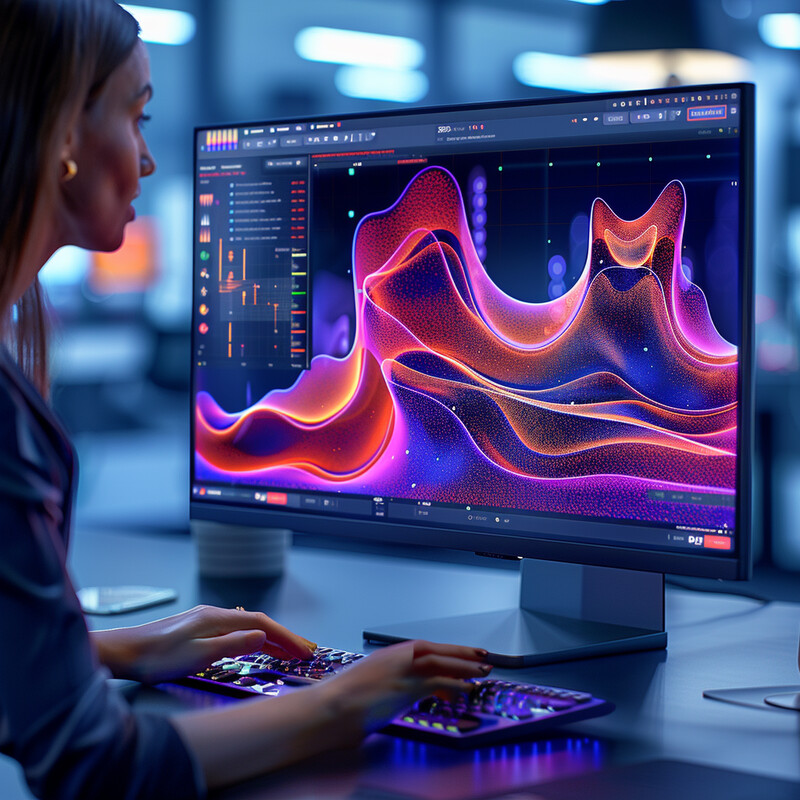
AI analyzes product designs to ensure they are optimized for manufacturing processes. It identifies design elements that could complicate production, increase costs, or affect quality, and suggests modifications. This early detection helps avoid costly redesigns and production delays, ensuring a smooth transition from design to manufacturing.
10. Feedback Loop Integration
AI systems collect and analyze user feedback on existing products to inform the design of new iterations or models, ensuring that updates and new products are aligned with actual user needs and preferences.
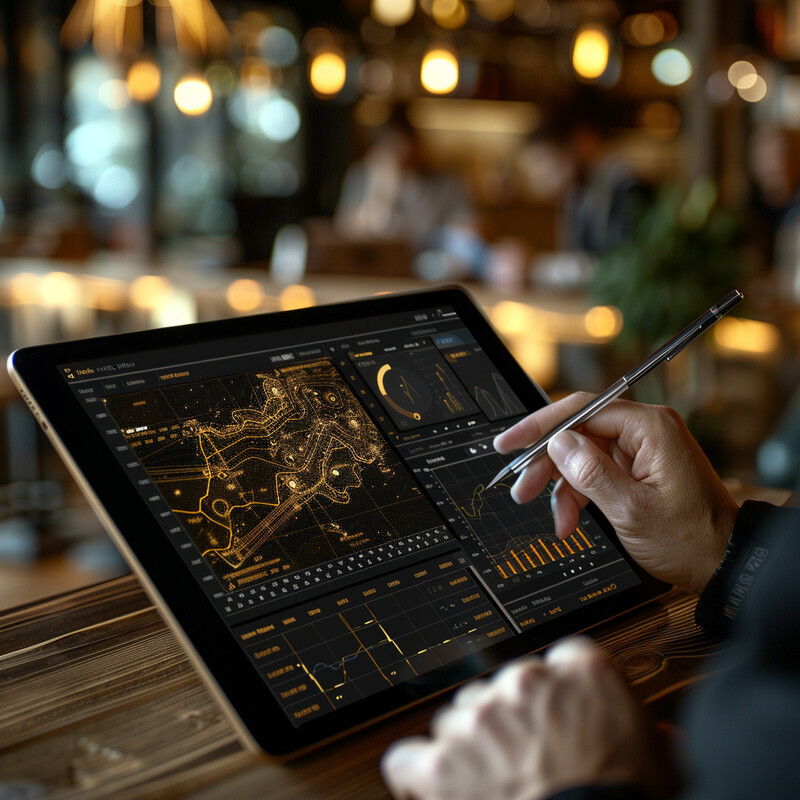
AI integrates feedback from customers directly into the design process. By collecting and analyzing data on how existing products are used and experienced, AI identifies areas for improvement and innovation. This ongoing feedback loop ensures that new products are better tailored to meet user needs and preferences, enhancing customer satisfaction and brand loyalty.