1. Personalized Playlists
AI-driven personalization analyzes user listening history, preferences, and behaviors to compile playlists uniquely suited to each individual. These systems employ collaborative filtering and content analysis to surface songs that match users’ tastes, leading to higher satisfaction and engagement. By continuously learning from what the user plays, skips, and likes, AI can keep updating playlists so they feel fresh and relevant. This personalization also helps users feel understood, strengthening loyalty to the service. Moreover, by tailoring music to individuals, services can differentiate their offerings in a competitive streaming market. Over time, personalized playlists have become a core part of the user experience on major platforms.
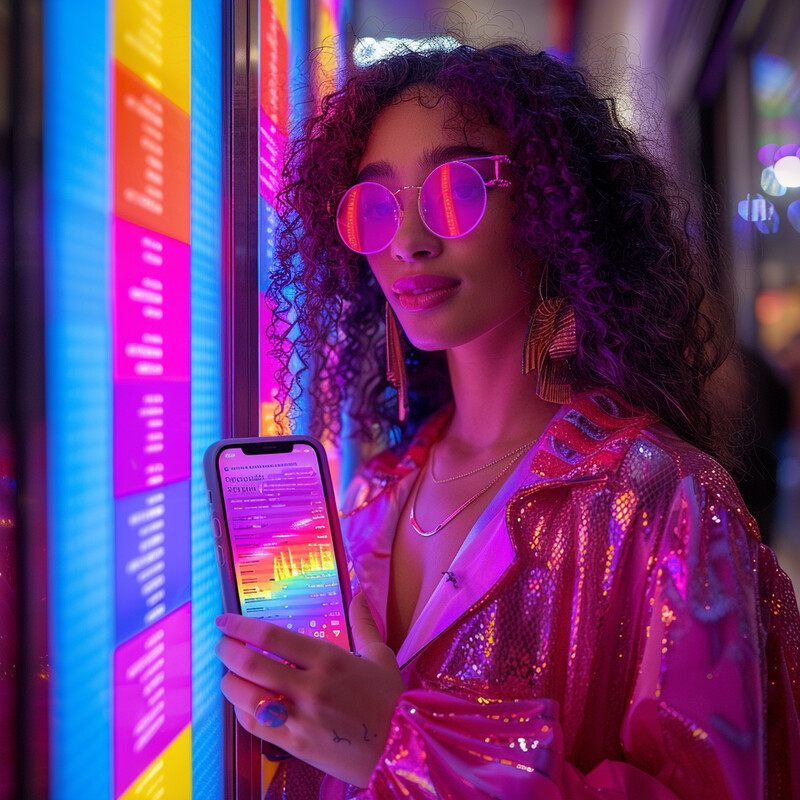
For example, data shows algorithmic “personalized” playlists now drive a significant share of listening. A 2024 industry analysis found that Spotify’s AI-curated playlists (like Discover Weekly and Daily Mix) accounted for over 21% of all streams in a year. This proportion has been growing (it rose by about 12% over the prior year), reflecting how extensively such recommendations affect listening. In practical terms, these AI-generated playlists introduce users to new songs and artists: one report indicated Spotify’s internal data attributes roughly 30% of listening time to personalized lists. This underscores that advanced AI personalization is not just a novelty but a driving force in how people consume music.
2. Mood Detection
AI mood detection tailors music recommendations to a listener’s emotional or situational context. By analyzing features like tempo, key, lyrics sentiment, or even leveraging cameras or wearables to gauge a user’s mood (e.g. happy, calm, energetic), systems can suggest songs that align with how the user feels or wants to feel. This use of affective computing means playlists can be dynamically tuned – for instance, offering uplifting tracks when a user is low or mellow songs during relaxation. Such mood-based curation can enhance user experience by making the service more empathetic and engaging. As mood-detection technology improves (through advances in machine learning and large emotional datasets), its role in recommendation is expanding. In practice, mood-based features help streaming services differentiate their UX and keep listeners in the moment.
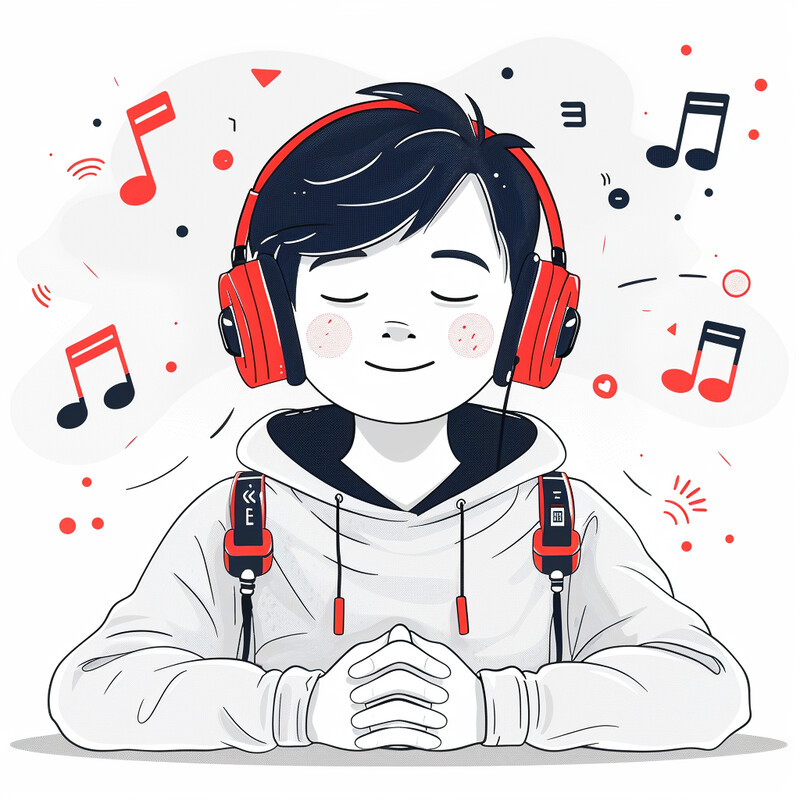
The market for emotion-recognition and mood-analysis AI (a proxy for mood-based recommendation technology) is growing rapidly. Industry research reports the “emotion AI” market was valued at about $2.9 billion in 2024, with a projected compound annual growth rate of roughly 21.7% through 2034. This reflects widespread adoption of mood-detection tech in consumer applications, including music streaming. The high growth rate suggests services increasingly invest in analyzing and responding to user moods. Thus, mood-based recommendation is a maturing area driven by these market forces.
3. Discovery of New Artists and Genres
AI systems dramatically expand users’ horizons by recommending new artists and genres. By learning a listener’s style and cross-referencing that with millions of tracks, AI can surface relevant unfamiliar music – from indie bands to foreign-language hits – that the user might never find manually. This “exploratory” feature enriches the listening experience and helps artists reach new audiences. Algorithms can weigh not just similarities but also novelty, ensuring recommendations strike a balance between comfort and discovery. As a result, users often credit streaming platforms with introducing them to favorite new acts. In summary, AI’s deep catalog analysis and pattern-finding enable serendipitous music discovery at scale.
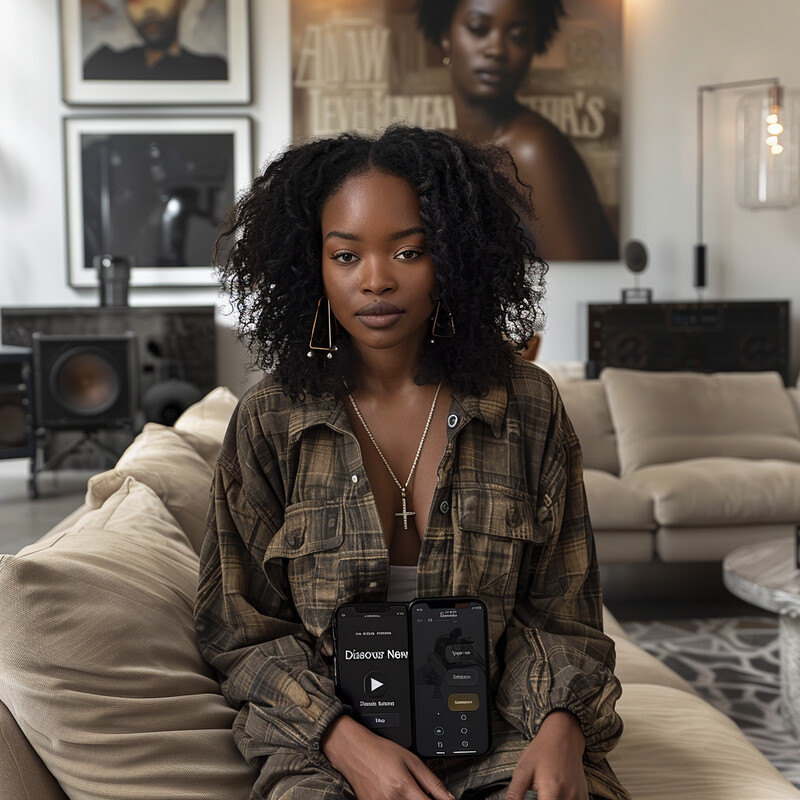
Listeners overwhelmingly value algorithmic recommendations for discovering music. In a 2023 US survey, 86% of streamers said recommendations of new artists/songs from the service were useful (37% found them very useful). Furthermore, about 72% of listeners reported they would continue listening to artists suggested by the platform. Another 62% of streamers said they became more of a fan of a new artist or genre because of those recommendations. These high percentages indicate AI-facilitated discovery strongly influences listener behavior and expands fans’ musical tastes.
4. Context-Aware Recommendations
AI-powered systems tailor recommendations to situational context like time, location, activity, or device. For example, a service might suggest upbeat workout tracks in the morning gym, chill playlists in the evening, or travel-themed music on a road trip. These context-aware features use data (e.g. geolocation, time of day, calendar events, connected devices) to infer what the user is doing or needs. The result is a seamless listening experience where the music fits the moment without manual selection. Contextual recommendations can also consider seasonal factors or current events. By being sensitive to context, AI helps keep playlists relevant and engaging as situations change.
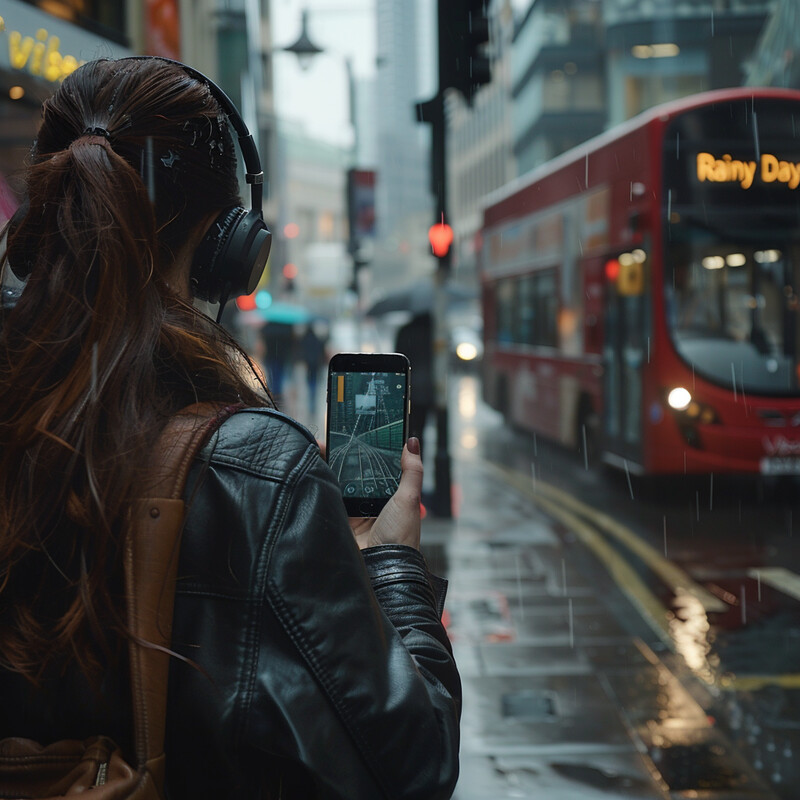
Real-world usage data confirm strong context-driven listening patterns. For instance, Spotify observed that workout playlist streams jumped 39% in January 2023 compared to December 2022 in the US – reflecting New Year fitness goals. Similarly, yoga playlists saw a 42% increase in the same period. These spikes demonstrate how user listening shifts with context (in this case, holiday timing and exercise resolutions). Context-aware recommendation systems exploit such trends by surfacing appropriate playlists when listeners are most receptive.
5. Adaptive Learning
AI systems continuously improve recommendations by learning from each user interaction (skips, repeats, likes, or searches). This adaptive learning means the service refines its model of the user over time, becoming more accurate with every session. Machine learning algorithms update their parameters as new listening data arrives, allowing rapidly changing tastes or contexts to be accommodated. For example, if a user begins a new hobby and listens to related music, the AI will shift suggestions accordingly. This ongoing training loop ensures the recommendation model remains aligned with the user’s current preferences. Adaptive learning also helps platforms react to larger trends and gradually incorporate them into individual profiles. Ultimately, these methods keep the experience personalized even as tastes evolve.
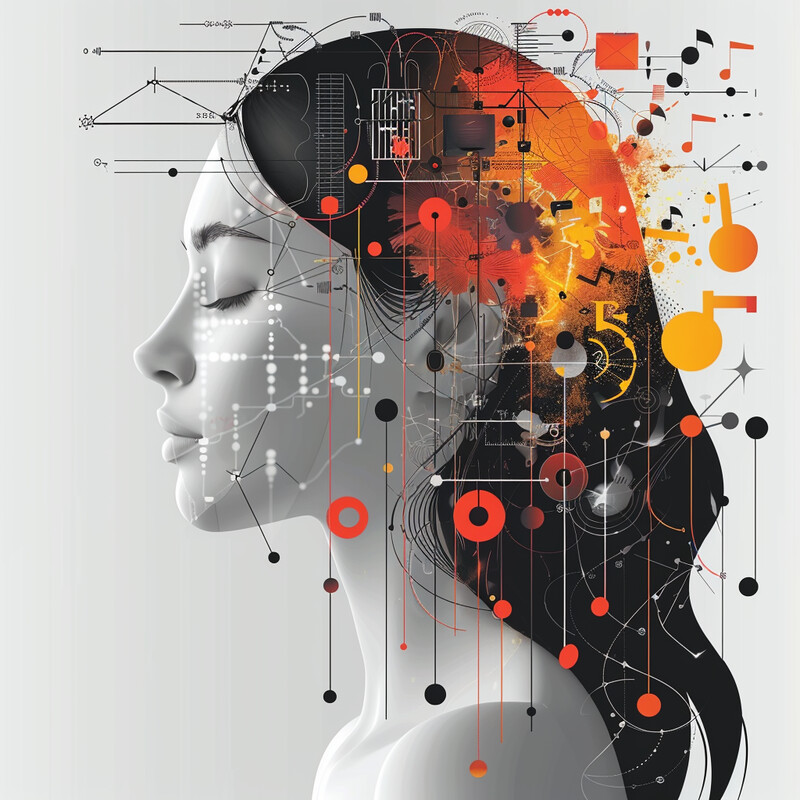
AI-driven personalization tangibly improves engagement and retention on streaming platforms. A recent review noted that advanced personalization “enables platforms to personalize music recommendations…increasing engagement and retention rates”. In other words, systems that adapt to user data help people spend more time listening and staying subscribed. This aligns with observed business data: platforms report higher user loyalty when recommendations closely match individual taste. Thus, the adaptive learning capability of AI is key to sustaining active user bases.
6. Social Listening Insights
Modern recommendation engines leverage social signals and trends to enhance discovery. They incorporate what friends listen to, trending hits on social media, and viral music cues into suggestions. AI can scan social platforms (TikTok, Instagram, etc.) to identify trending tracks and feed them into user playlists. Features like collaborative playlists and “blend” playlists allow friends to mix tastes together. These social mechanisms help users discover music through their communities. In effect, AI taps into crowd behavior: if a song is gaining popularity among peers, the system is likely to recommend it. This social dimension turns listening into a communal, culturally-relevant experience.
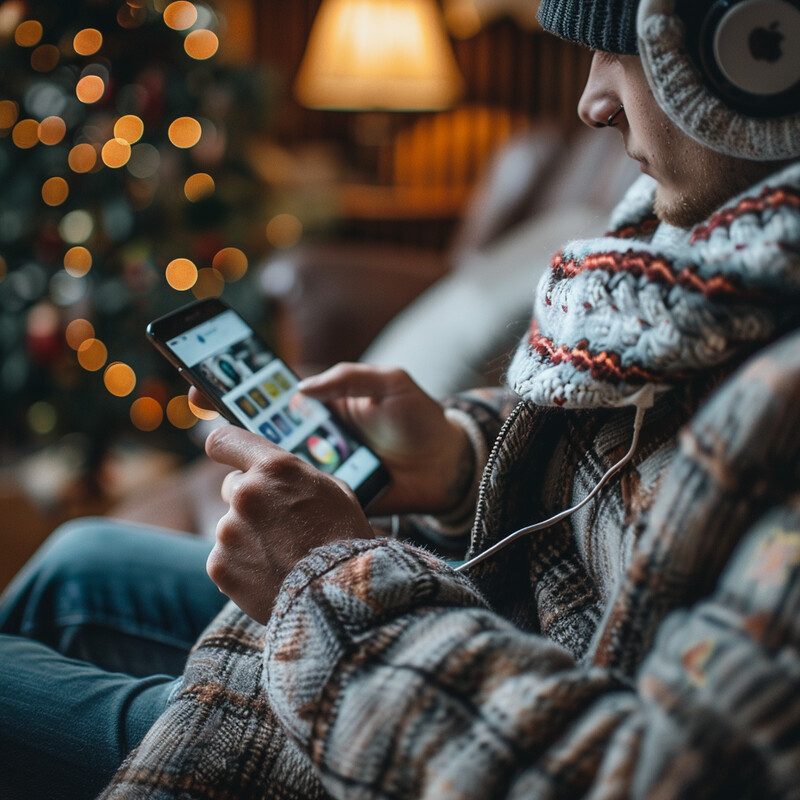
Social media has become a dominant channel for new music discovery. A January 2025 industry report cites a 2023 MusicWatch survey showing 68% of social media users discover new music via short-form video platforms like TikTok or Instagram Reels. (For context, TikTok itself had over 1 billion monthly active users in 2024, with music in 90% of its top videos.) These figures highlight that AI-driven services often look to social trends to recommend songs, as a large majority of listeners now find songs through viral social content rather than traditional radio or ads.
7. Dynamic Playlist Curation
Streaming platforms use AI to keep playlists up-to-date with new releases and trends. Curated playlists (both algorithmic and editorial) are refreshed regularly – some daily or weekly – so listeners always have access to the latest music. AI determines which new tracks align with each playlist theme by analyzing streaming data, chart moves, and editorial inputs. This dynamic curation helps new artists gain exposure quickly. For users, it means stepping into a “living” playlist that evolves with the music landscape. As a result, the platform feels current and responsive, encouraging users to check in often for fresh content.
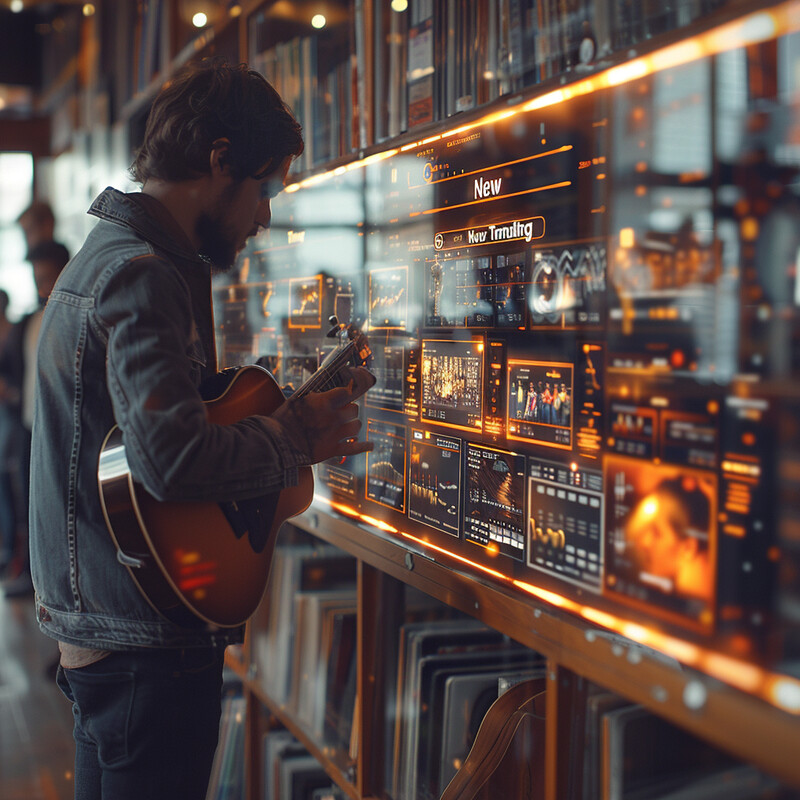
Curated playlists significantly boost attention to fresh music. For example, industry analysis reports that Spotify’s regularly updated playlists like “New Music Friday” contribute to roughly a 20% increase in streams for featured new artists. In practical terms, being added to these dynamic playlists can dramatically amplify an emerging artist’s reach. Similarly, a Rolling Stone study found that about 75% of Spotify users regularly listen to editorial playlists, indicating the widespread influence of these curated feeds on audience listening.
8. Seamless Music Integration
AI enables music services to blend effortlessly into users’ daily environments and devices. Through partnerships and cross-platform compatibility, AI ensures that the right music follows the listener—whether on headphones, smart speakers, cars, or smart homes. Intelligent syncing and voice-control allow playlists to continue playing seamlessly as users move between contexts (for instance, from home to car). This omnipresence means AI algorithms must coordinate context changes, resume positions, and preferences across platforms. The result is that music feels ubiquitously available and personalized no matter how or where the user listens.
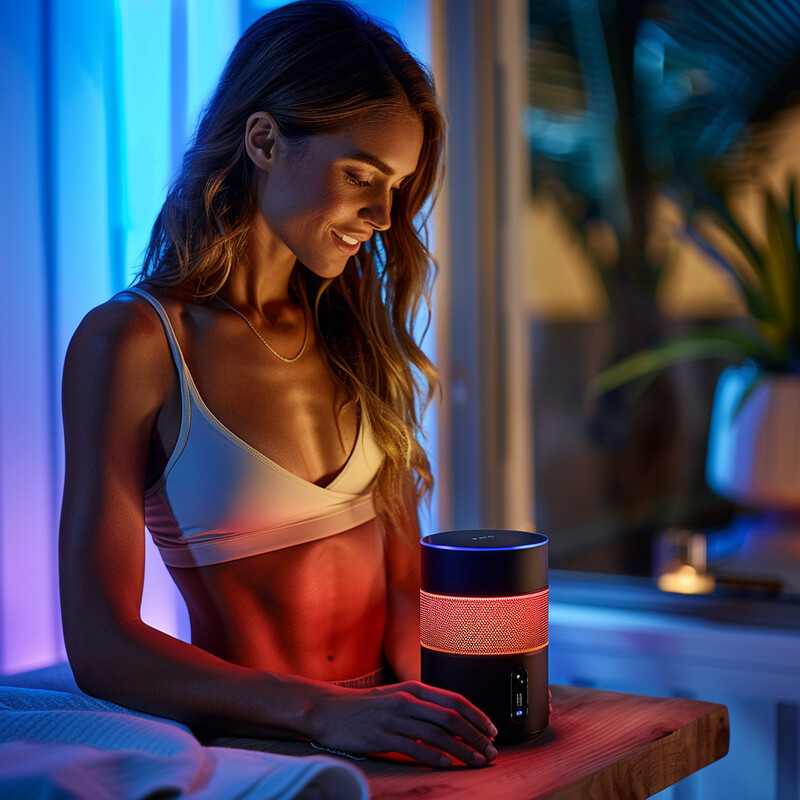
Integrated listening devices are now widespread. According to industry data, 22% of all smart-speaker listening minutes in the U.S. go to Amazon Music, reflecting how many users rely on voice-activated speakers for daily listening. Likewise, automakers report demand for built-in streaming; one study found 68% of drivers prefer cars with integrated music streaming services. These statistics underline the importance of seamless AI-driven integration – platforms must optimize for smart speakers, cars, wearables, etc., because a substantial portion of listening occurs through these connected devices.
9. Lyrics and Music Analysis
AI delves into the content of songs – including lyrics, melody, and audio features – to refine recommendations. By analyzing lyrical themes, sentiment, tempo, key, and instrumentation, AI can match music to a user’s deeper tastes. For example, two songs in different genres might be recommended together if their moods and lyrical content are similar. Natural language processing and music analysis also allow systems to tag tracks by emotion or theme, enriching the metadata. This granular understanding helps the AI recommend niche tracks that traditional genre labels might miss. Such content-based analysis complements collaborative filtering and broadens the range of suggestions.
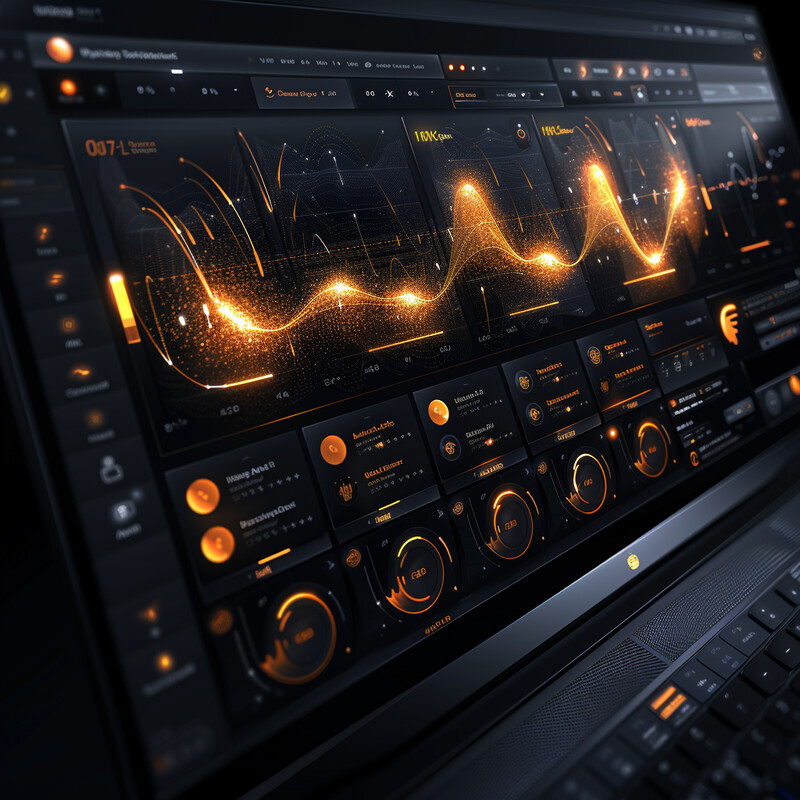
The broader market for AI in music content creation and analysis is rapidly growing. Research estimates the global generative AI in music market (covering AI-composed music, automated mixing/mastering, and related tools) was about $440 million in 2023 and is projected to grow at roughly 30% annually through 2030. This surge implies increasing investment in AI capabilities like lyric analysis and composition. In context, streaming services are adopting these advances: for example, Spotify publicly highlights analyzing track “valence” (positiveness) to understand listener moods. Such trends indicate lyrics and acoustic analysis play an expanding role in how AI curates music.
10. User Feedback Incorporation
AI systems explicitly incorporate user feedback (thumbs-up/down, skips, favorites) to refine future suggestions. Each user action is treated as a data point indicating preference, allowing the recommender to adapt. For instance, if a user thumbs-downs a track, the algorithm learns to avoid similar songs. Conversly, saving a track can increase similar recommendations. Over time, this feedback loop helps the AI create a personalized profile for each listener. Moreover, features like personalized radio or “daily mixes” evolve with ongoing feedback. In short, every user interaction teaches the system how to better serve that user’s tastes.
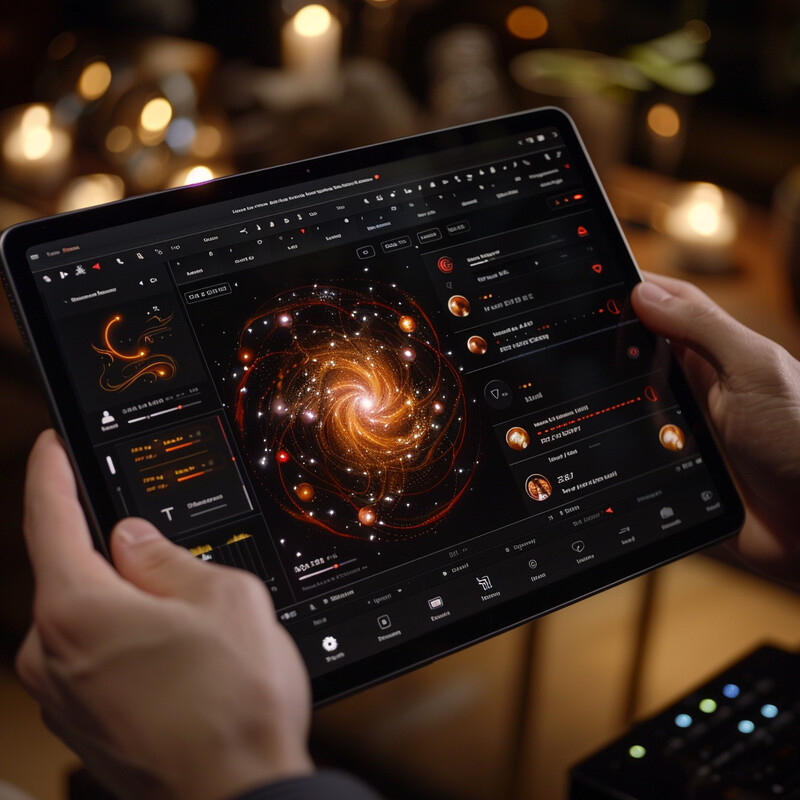
User-driven data can markedly boost engagement. For example, Spotify reports that engaging social features (like collaborative playlists that rely on user input) have raised user engagement by about 20%. While this isn’t a direct like/dislike stat, it illustrates how incorporating social and interactive feedback mechanisms (users curating playlists together) significantly deepens engagement. Overall, platforms find that when user feedback is systematically fed back into AI models, metrics like listening time and satisfaction measurably improve.