1. Predictive Modeling
AI utilizes vast amounts of data to build predictive models that forecast the likelihood of claims for individual policyholders based on their specific risk factors.
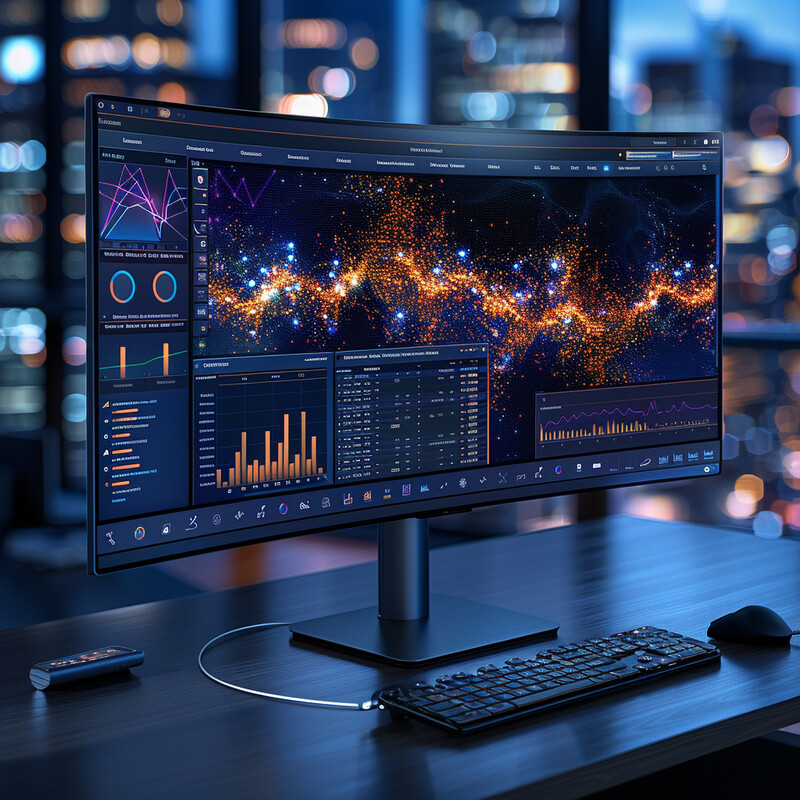
AI leverages large datasets from past insurance claims and user profiles to construct predictive models. These models forecast potential risks and claim probabilities by analyzing correlations between various risk factors and past incidents. This enables insurers to assess risks more accurately and efficiently, leading to better decision-making regarding policy pricing and terms.
2. Fraud Detection
AI algorithms analyze patterns in claims data to identify irregularities that may suggest fraudulent activity, helping insurers prevent losses and maintain fair premiums for honest customers.
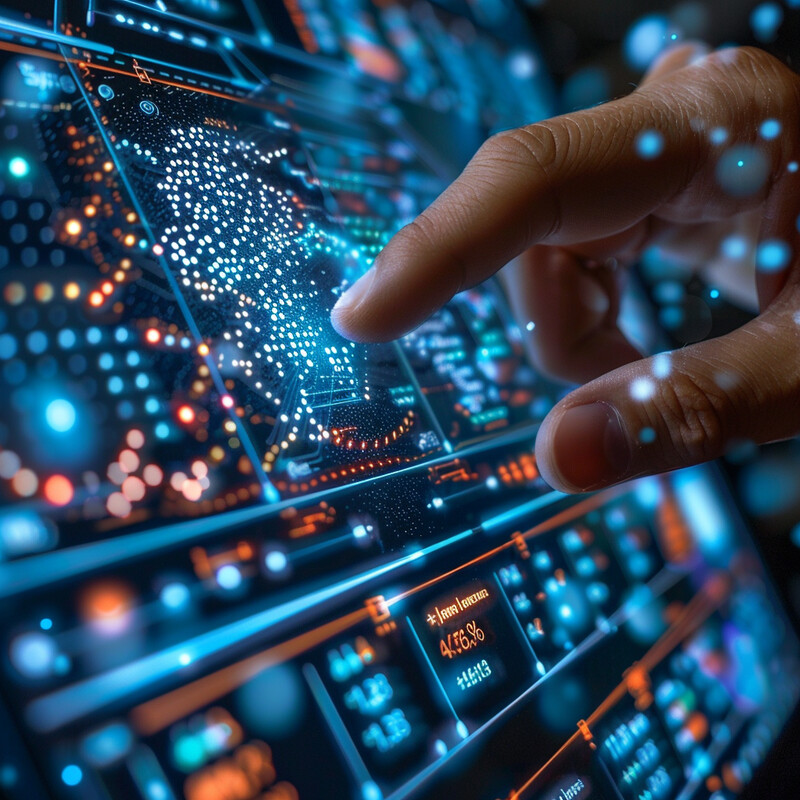
AI algorithms scrutinize patterns and anomalies in claims data to detect potential fraud. By comparing each claim against historical data, AI can identify irregularities such as exaggerated claims, multiple claims for the same incident, or patterns that commonly signify fraudulent activities. This not only helps prevent financial losses but also protects honest policyholders by keeping insurance costs down.
3. Personalized Pricing
AI enables more precise underwriting by assessing individual risk factors, allowing insurers to offer personalized pricing that reflects the true risk of insuring a person or property.
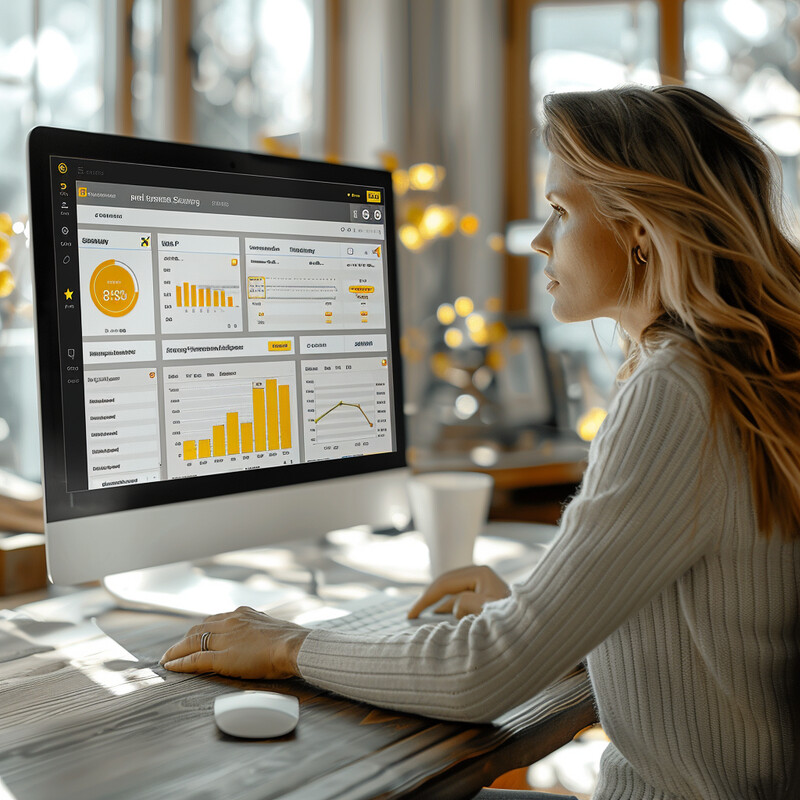
Using AI, insurers can analyze individual risk factors more deeply, such as driving habits in auto insurance or health records in life insurance. This granular analysis allows for personalized pricing models that more accurately reflect the risk associated with insuring a particular individual, offering fairer pricing and helping insurers manage risk better.
4. Telematics for Auto Insurance
AI processes data from telematics devices to monitor driver behavior in real time, such as speed, braking habits, and driving times, to assess risk levels more accurately and tailor auto insurance premiums accordingly.
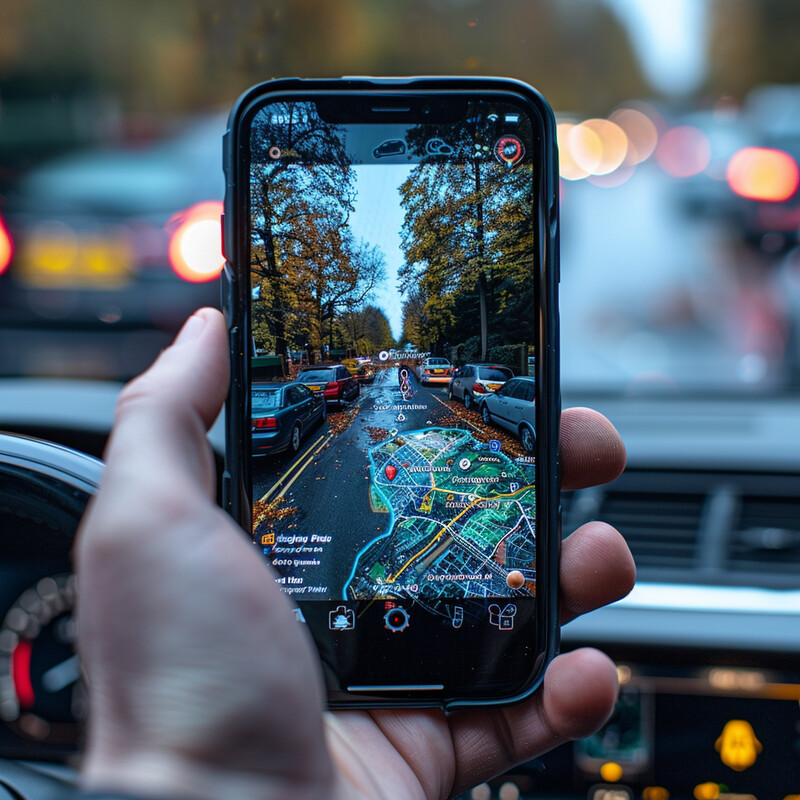
Telematics devices installed in vehicles collect data on driving behavior, including speed, braking habits, and time of driving. AI processes this data to assess the driver’s risk profile based on their actual driving patterns. This enables insurers to offer personalized insurance premiums, rewarding safe driving behaviors with lower rates.
5. Automated Claims Processing
AI streamlines the claims process by automating damage assessment, claims verification, and payments, reducing processing time and human error while improving customer satisfaction.
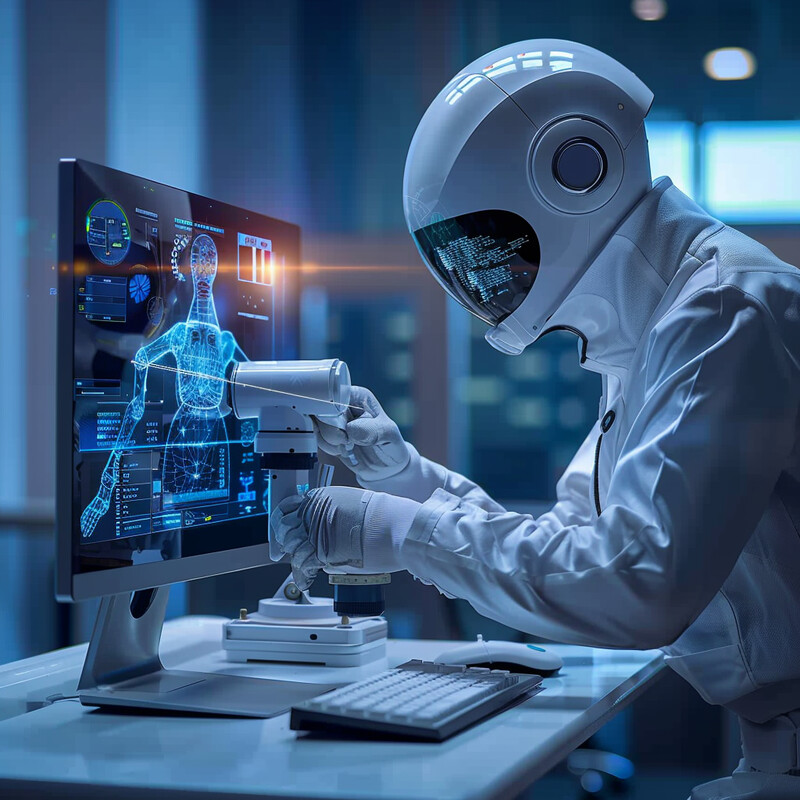
AI enhances the efficiency of the claims processing by automating assessments of damages and verification of claims details. Using image recognition and data analysis, AI can quickly evaluate the extent of damage, authenticate claim information, and even automate payment processes, significantly reducing the time and cost involved in claims handling.
6. Enhanced Customer Profiling
AI analyzes behavioral data and other subtle patterns from various sources to create detailed customer profiles, aiding insurers in understanding their clients' risk profiles better.
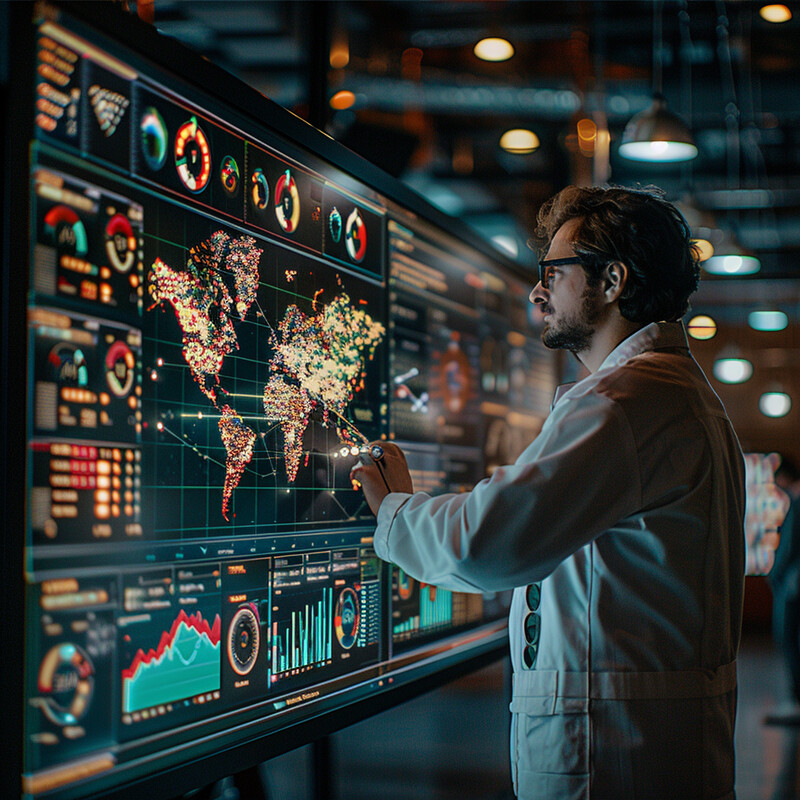
AI digs into vast amounts of behavioral data to craft detailed customer profiles. These profiles help insurers understand the lifestyle, preferences, and risk patterns of their clients, allowing for more tailored insurance products and risk management strategies that align with the specific needs and risk levels of different customer segments.
7. Natural Disaster Prediction and Assessment
AI integrates data from weather stations, satellites, and geological surveys to predict natural disasters and assess the potential impact on insured properties, enabling proactive risk management and disaster response.
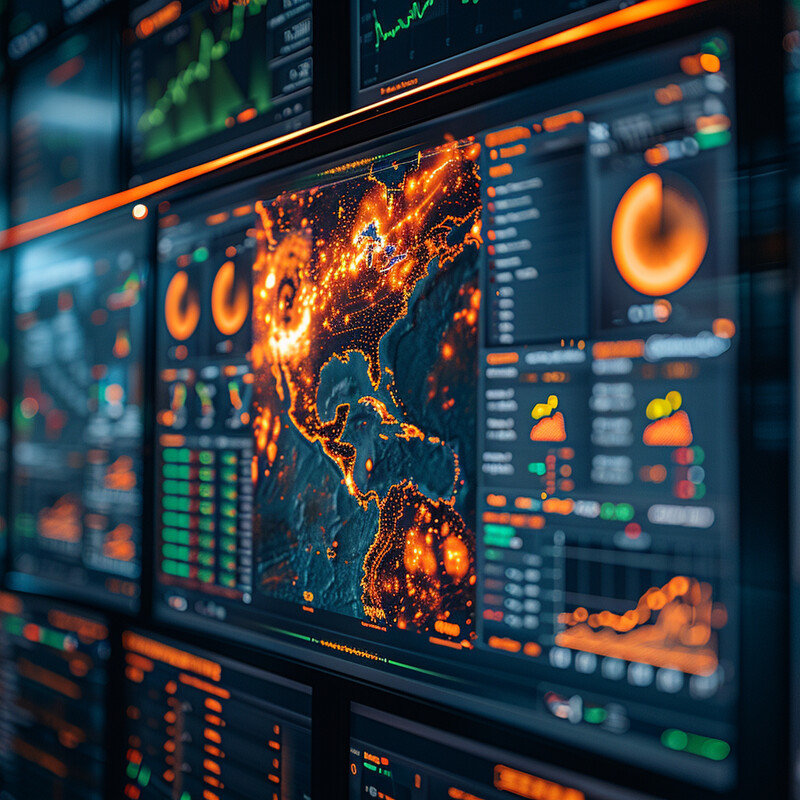
AI integrates and analyzes data from multiple sources, including satellite imagery, weather stations, and geological surveys, to predict natural disasters and assess their potential impact on insured properties. This predictive capability enables insurers to undertake proactive measures in risk pricing and claims management before disasters strike.
8. Property Risk Assessment Using Drones and IoT
AI analyzes images and data collected by drones and IoT sensors to assess property conditions and risks without the need for physical inspections, enhancing the accuracy and efficiency of property insurance underwriting.
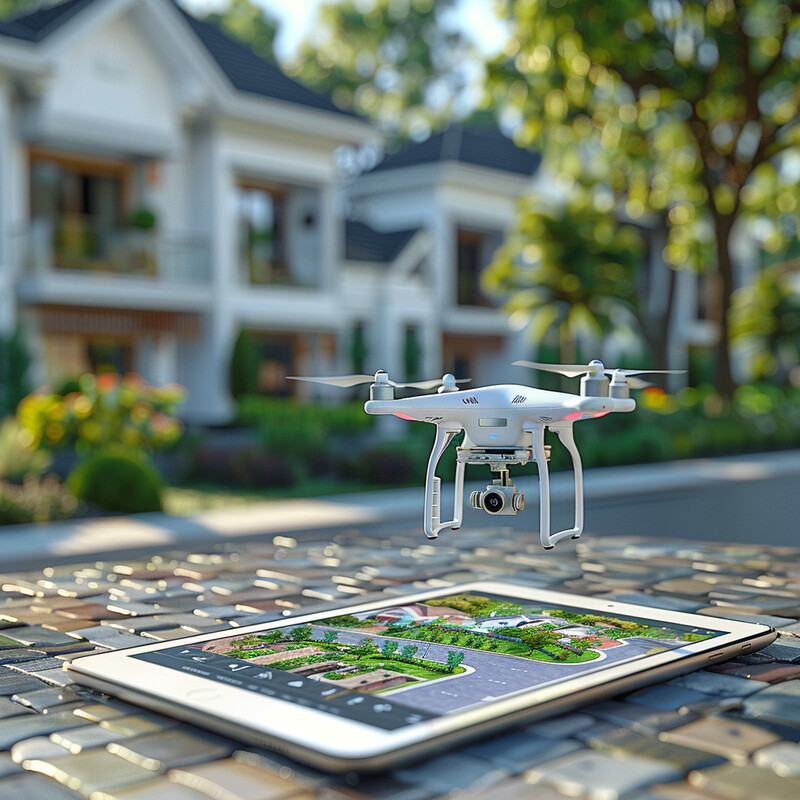
Drones and IoT devices equipped with sensors and cameras gather detailed information about the condition of properties and the surrounding environment. AI analyzes this data to assess risks associated with property conditions, such as structural weaknesses or susceptibility to environmental hazards, improving the accuracy and efficiency of property underwriting.
9. Health Risk Prediction
AI uses health data and lifestyle information from wearable technology to predict health risks and insurance needs, helping life and health insurers tailor their products and services to individual health profiles.
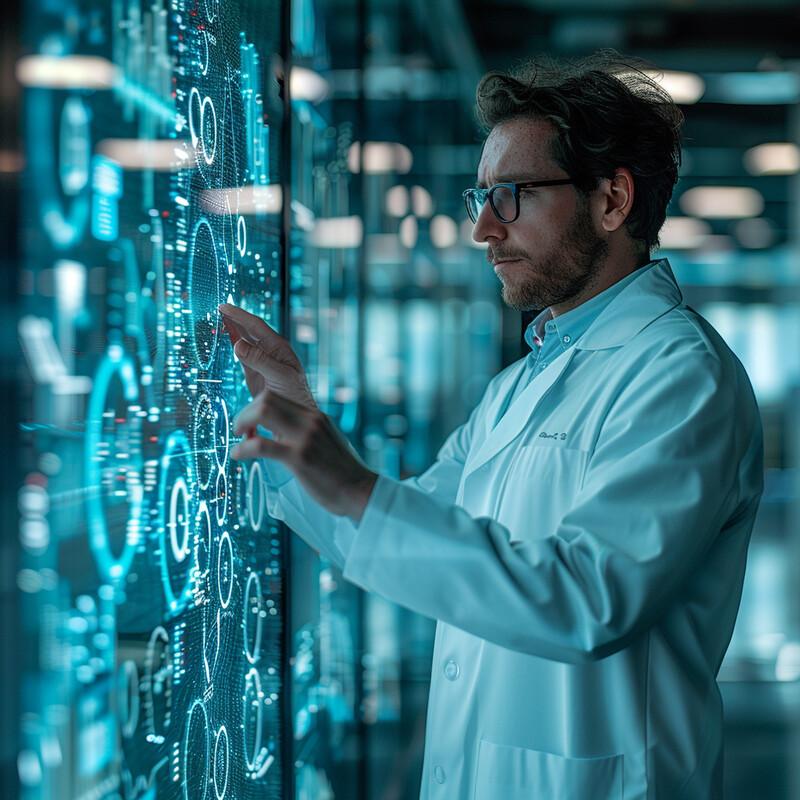
By analyzing data collected from wearable devices, which monitor various health metrics and activity levels, AI can predict future health risks and insurance needs. This allows health and life insurers to develop personalized insurance plans based on predicted health trajectories, encouraging healthier lifestyles among policyholders.
10. Cyber Risk Evaluation
As cyber threats evolve, AI helps in assessing the vulnerability of businesses to cyber-attacks, enabling insurers to accurately price cyber insurance based on dynamic risk assessments.
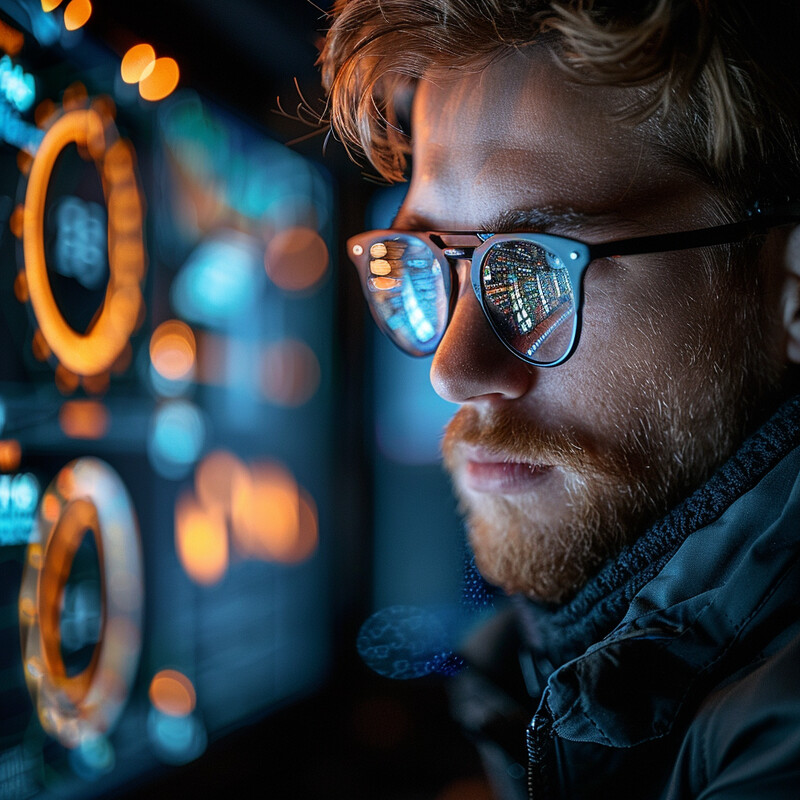
As cyber threats become more complex, AI assists in dynamically assessing an organization's risk exposure to cyber-attacks. By analyzing system vulnerabilities, past attack patterns, and emerging threat trends, AI helps insurers accurately evaluate and price cyber insurance, ensuring coverage reflects current cyber risk landscapes.