1. Increased Accuracy
AI algorithms have significantly improved in recognizing and distinguishing objects within images with greater precision, reducing errors and improving reliability.
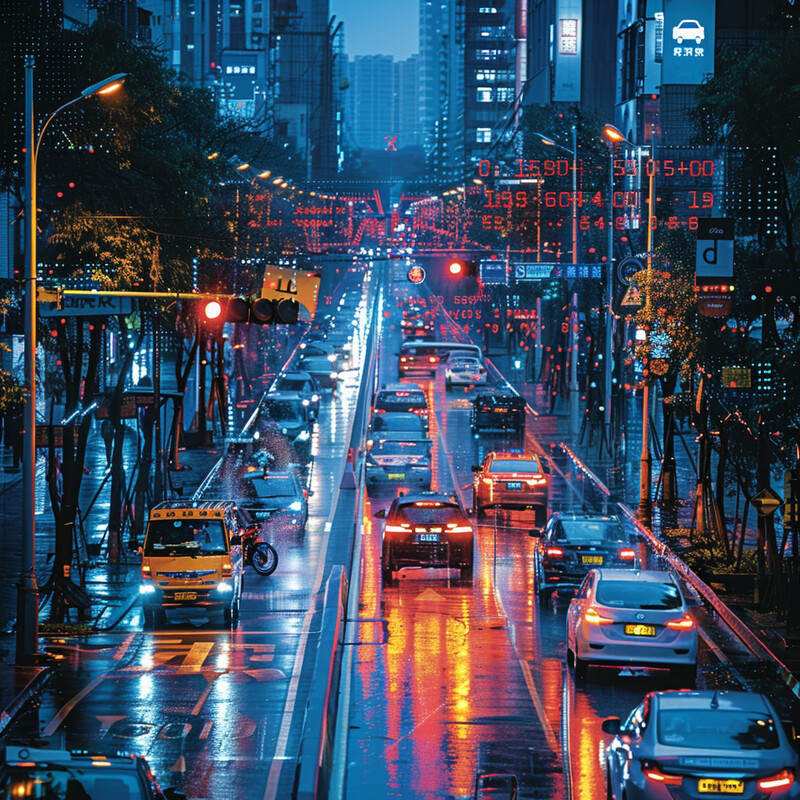
AI-powered image recognition systems have achieved unprecedented levels of accuracy in identifying and classifying objects within images. Through deep learning and neural networks, these systems can differentiate between objects with fine-grained detail, even in complex scenes. This high level of precision is crucial for applications ranging from retail product identification to wildlife monitoring.
2. Real-time Processing
AI enables the real-time analysis of images, allowing for immediate recognition and response, which is critical in applications like autonomous driving and security surveillance.
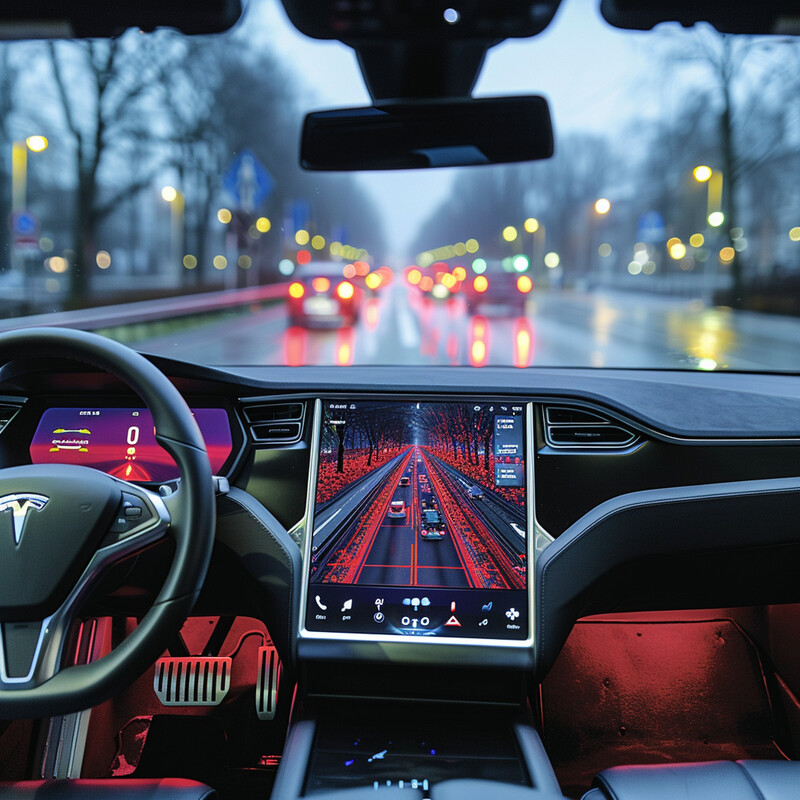
AI enables the real-time processing of images, allowing for instantaneous analysis and response. This capability is vital in scenarios where timing is critical, such as in autonomous vehicle navigation, where decisions must be made in milliseconds, or in security systems where threats need to be immediately identified and addressed.
3. Contextual Understanding
AI can interpret the context of an image, recognizing not just objects but the scene as a whole, which helps in applications like content moderation and targeted advertising.
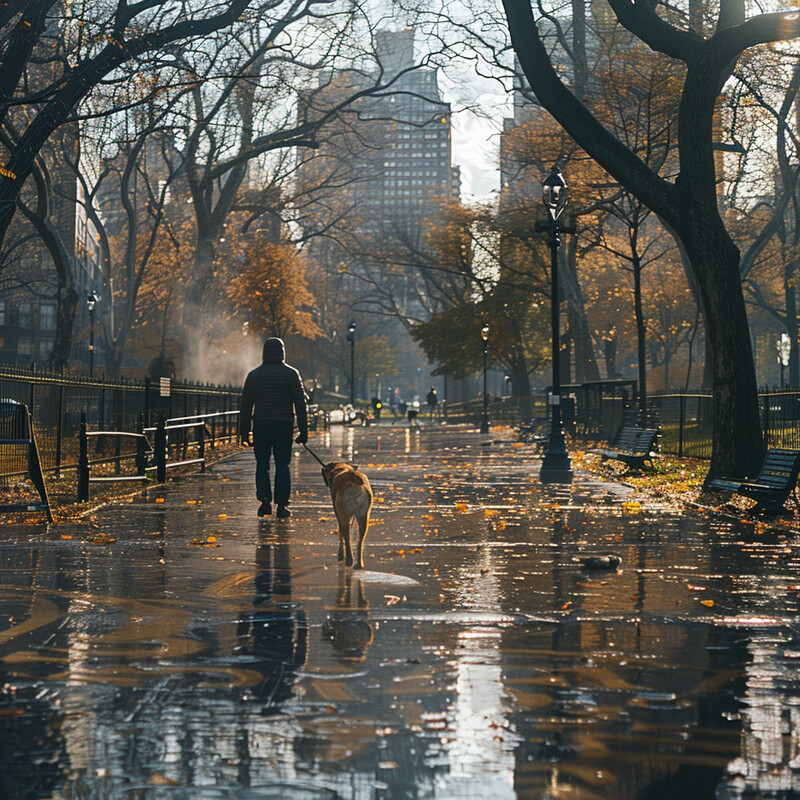
Beyond recognizing individual objects, AI enhances the ability to understand the broader context of an image. For example, in an urban setting, AI can identify not only vehicles and pedestrians but also interpret traffic flow, road conditions, and potential hazards, providing a comprehensive understanding that can inform decisions in smart city management or autonomous driving.
4. Facial Recognition
Advances in AI have refined facial recognition technologies, enhancing their accuracy and the ability to recognize faces across different lighting conditions, angles, and obstructions.
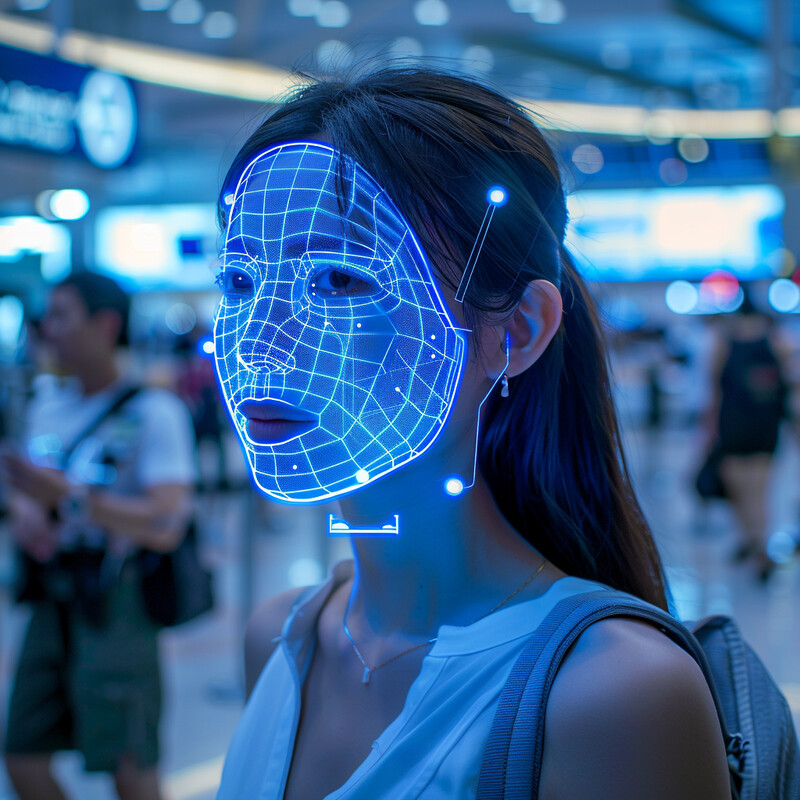
Facial recognition technology has greatly benefited from advancements in AI, improving not only in accuracy but also in its ability to recognize faces under varied conditions such as different lighting, angles, or partial obstructions. This improvement has broad applications, including security enhancements, personalized advertising, and user authentication processes.
5. Pattern Recognition
AI excels at identifying patterns in images, which is useful in sectors such as healthcare for diagnosing diseases from medical imaging, or in finance for detecting fraudulent activities.
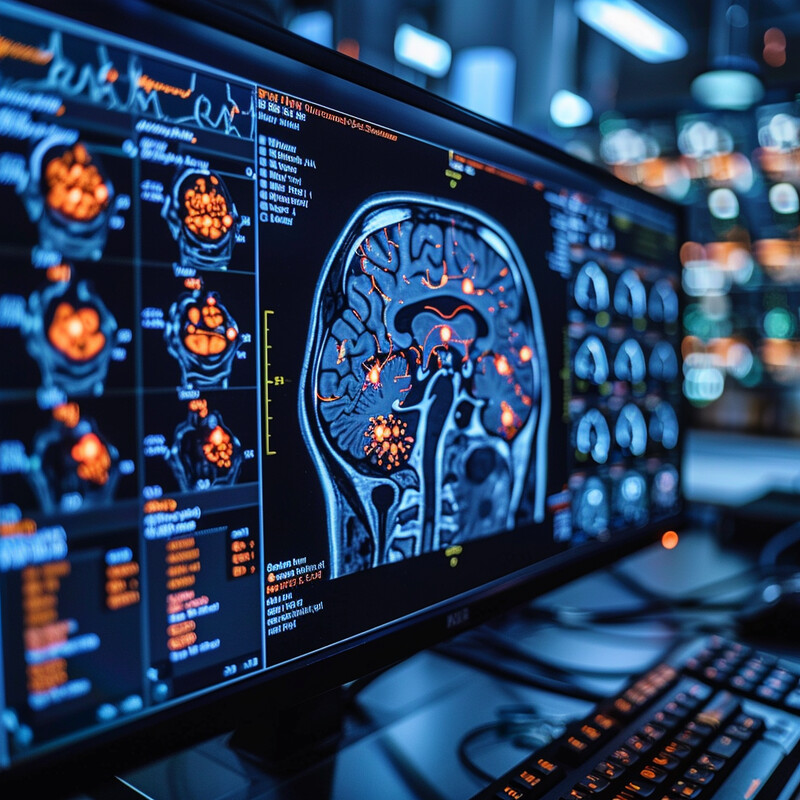
AI's capability to recognize patterns within images is transformative, especially in healthcare, where it is used to detect anomalies in medical imaging, such as X-rays or MRIs, potentially identifying diseases early. Similarly, in financial services, pattern recognition helps detect irregularities that may indicate fraudulent transactions.
6. Object Tracking
AI algorithms can track objects across a series of images or video frames, important for video surveillance, sports analysis, and interactive marketing.
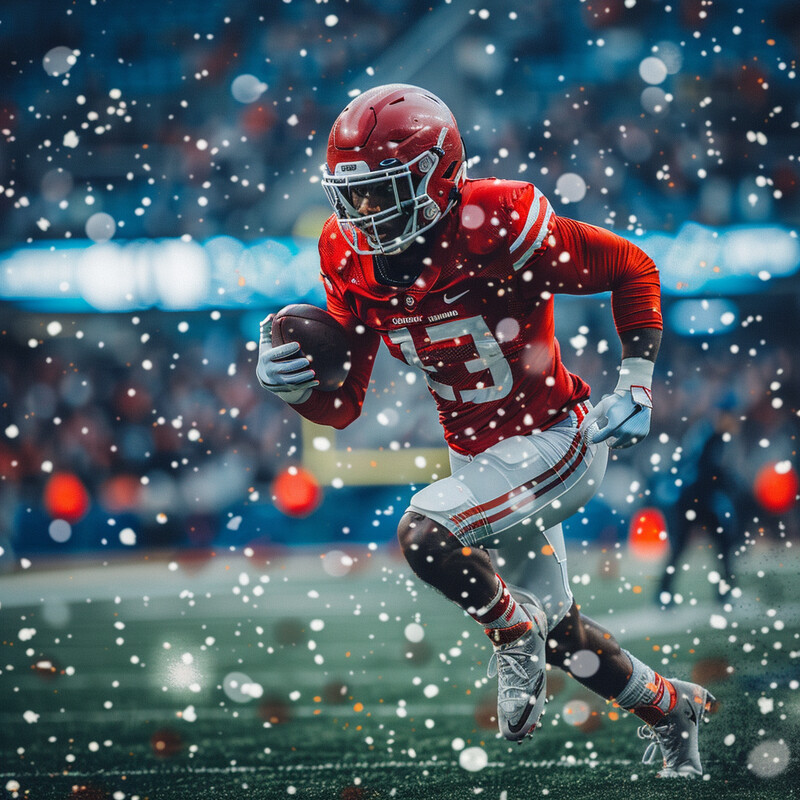
AI facilitates robust object tracking across video frames, which is essential for video surveillance systems in monitoring and security operations. It's also used in sports analytics to track player movements and actions, providing insights that can influence coaching decisions and game strategies.
7. Image Classification
AI has improved the classification of images into categories, facilitating better organization and retrieval of visual information in digital databases.
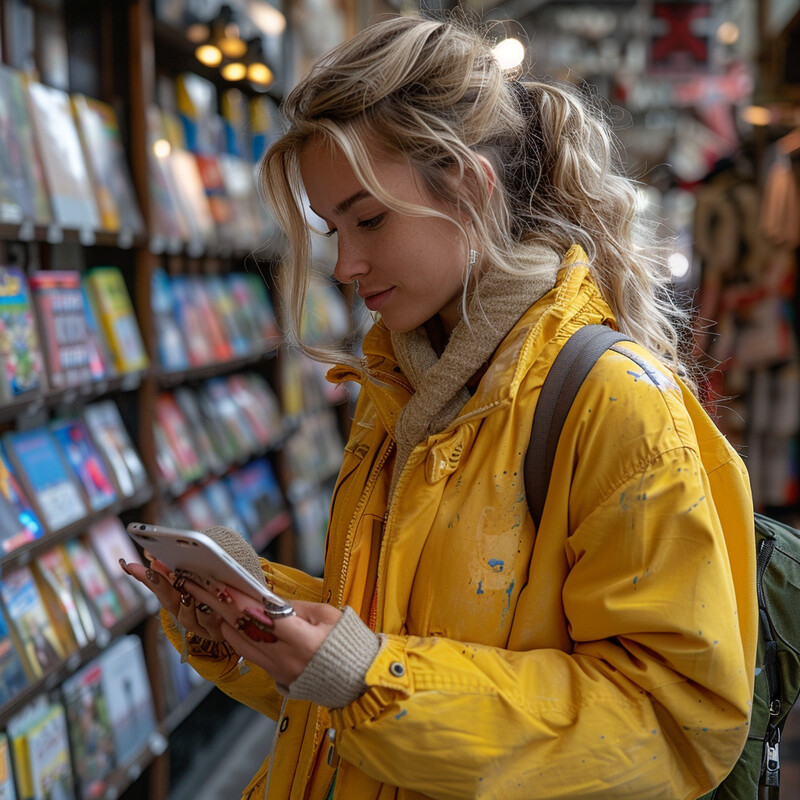
AI-driven image classification categorizes vast numbers of images into coherent groups automatically and with high accuracy. This technology supports everything from organizing personal photo collections to managing extensive stock image libraries, greatly enhancing searchability and accessibility.
8. Image Restoration
AI is used to restore old or degraded images, enhancing clarity and adding color to historical footage, which is valuable in digital archiving and media production.
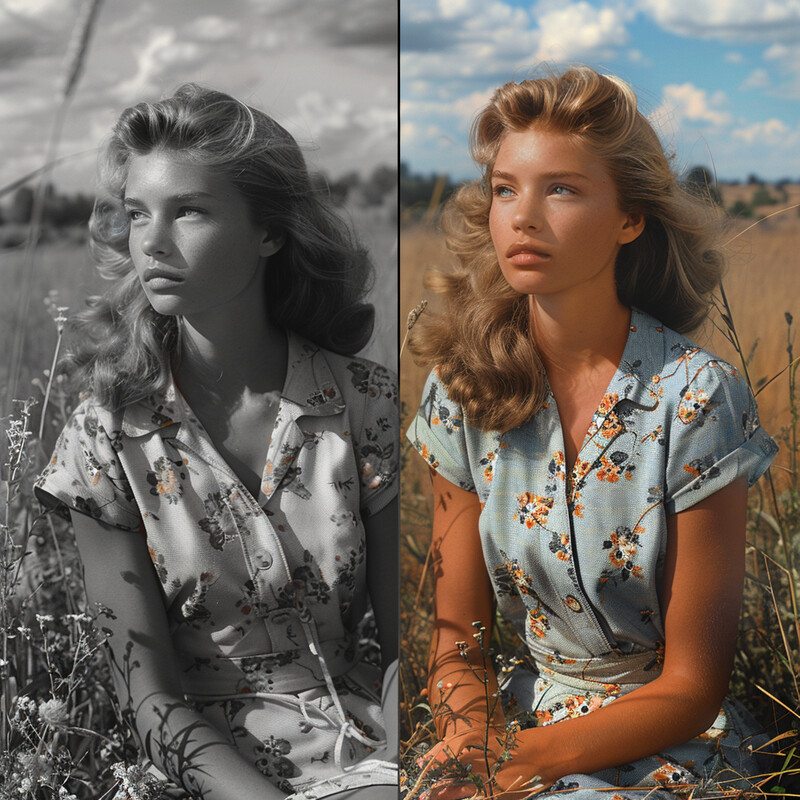
AI algorithms are adept at restoring and enhancing old or degraded images. They can sharpen details, remove noise, and even colorize black and white photos, which is invaluable in film restoration, digital archives, and media production, breathing new life into historical visual content.
9. Automated Tagging
AI automates the tagging of images with relevant keywords, improving the efficiency of managing large image libraries by making searching and sorting more streamlined.
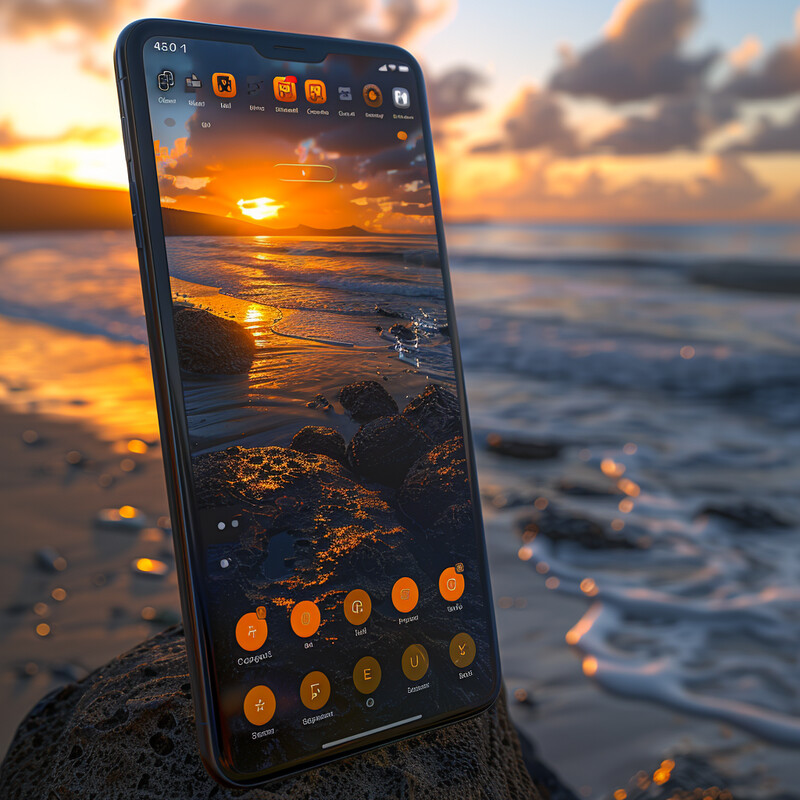
AI algorithms are adept at restoring and enhancing old or degraded images. They can sharpen details, remove noise, and even colorize black and white photos, which is invaluable in film restoration, digital archives, and media production, breathing new life into historical visual content.
10. Augmented Reality
AI enhances augmented reality systems by accurately anchoring digital overlays onto real-world images, improving user interaction and immersion in AR applications.
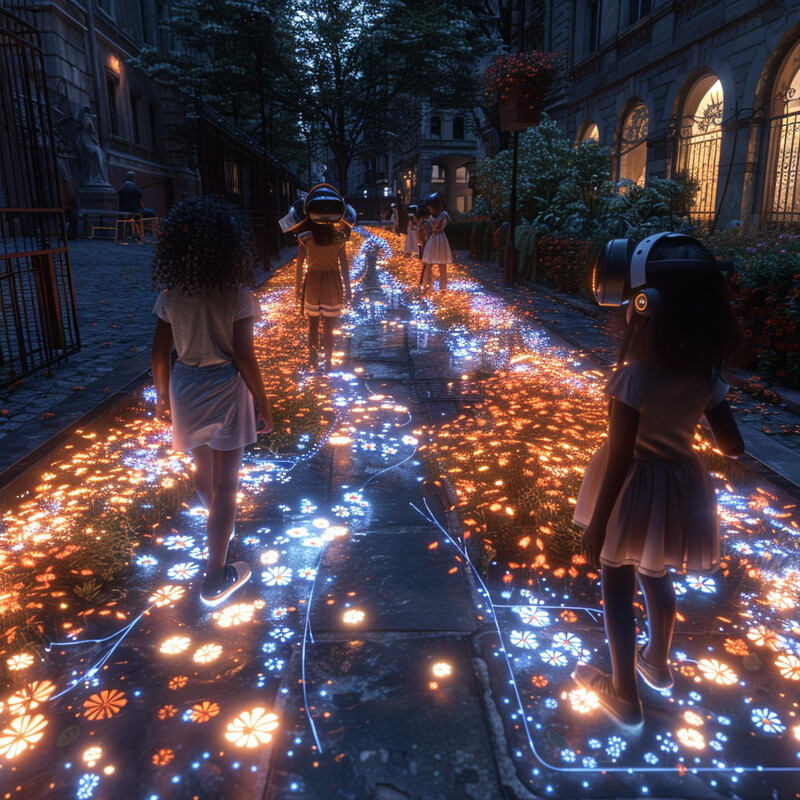
In augmented reality applications, AI improves the placement and interaction of digital objects in a real-world environment, ensuring that virtual overlays accurately align with physical objects in real time. This precision enhances user experience in AR applications, making them more realistic and immersive, applicable in gaming, education, and interactive marketing.