1. Climate Change Modeling
AI algorithms analyze vast amounts of climate data to predict future climate conditions and model potential impacts, aiding in the formulation of more effective climate adaptation strategies.
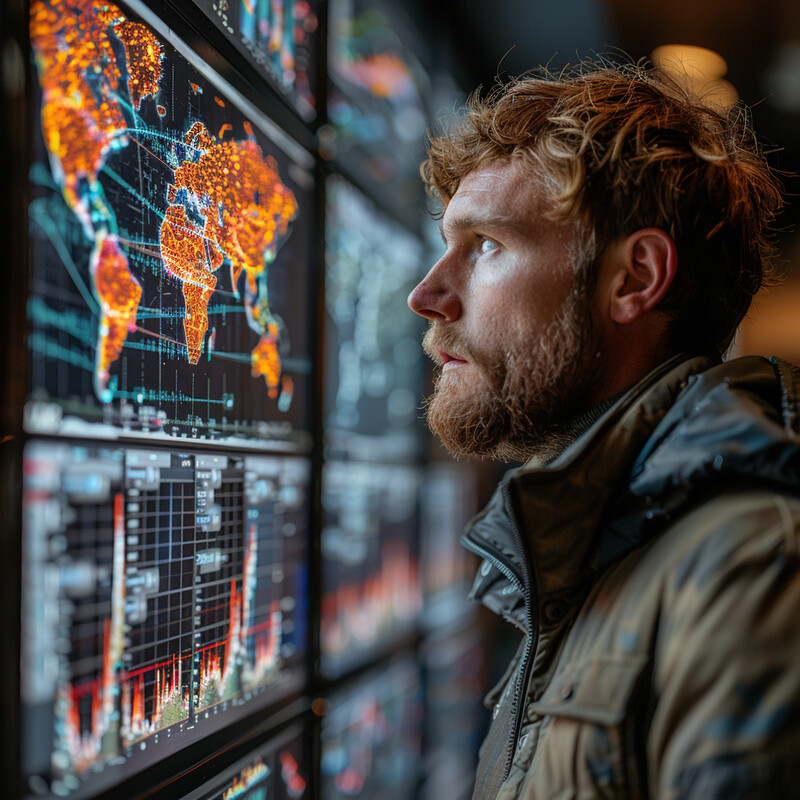
AI utilizes advanced algorithms to process and analyze vast amounts of climate data from satellites, weather stations, and ocean buoys to model and predict future climate conditions. These predictions help scientists understand potential impacts such as rising sea levels, temperature changes, and increased frequency of extreme weather events. AI-driven models aid policymakers in crafting targeted climate adaptation and mitigation strategies, aiming to reduce the adverse effects of climate change.
2. Air Quality Monitoring
AI-powered sensors and systems continuously monitor air quality across various locations, analyzing data in real time to identify pollution sources and trends.
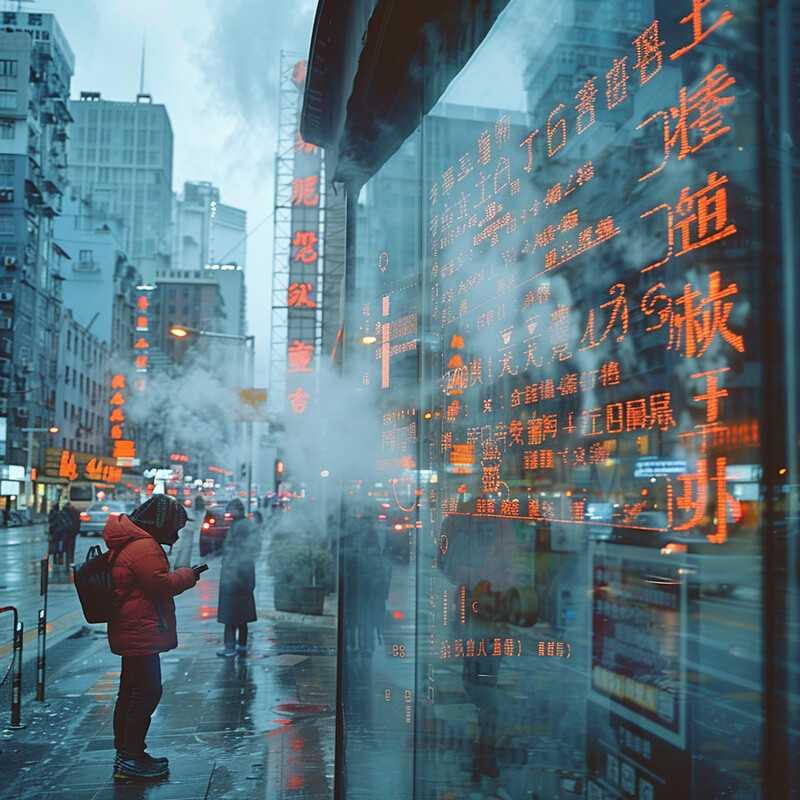
AI-powered systems continuously monitor air quality using sensors distributed across various urban and rural locations. These systems analyze data in real-time to detect pollutants such as particulate matter, nitrogen dioxide, and sulfur dioxide. AI identifies pollution sources and trends, enabling environmental agencies to enforce regulations more effectively and inform the public about air quality issues.
3. Water Quality Assessment
AI helps in monitoring and analyzing water quality by detecting pollutants and assessing changes over time, which is crucial for the conservation of aquatic ecosystems and public health.
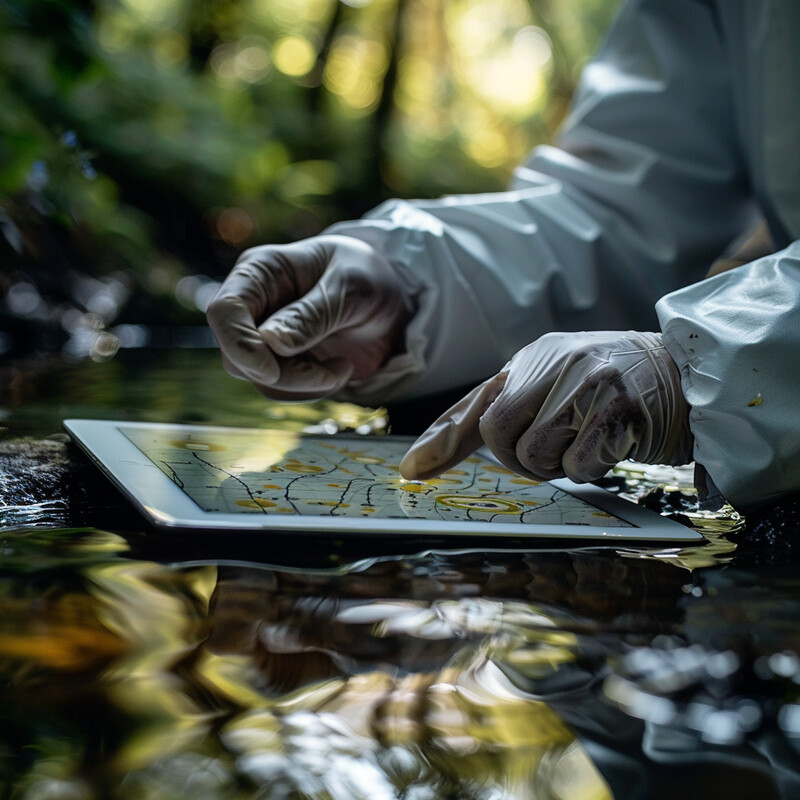
AI technologies are crucial in monitoring water quality in rivers, lakes, and oceans. By analyzing data collected from sensors and satellites, AI detects harmful levels of contaminants like heavy metals, nitrates, and pathogens. This monitoring is essential for protecting aquatic ecosystems and ensuring the safety of drinking water supplies, facilitating timely interventions to prevent waterborne diseases and ecological damage.
4. Wildlife Conservation
AI is used to analyze images and videos from camera traps to monitor wildlife populations and behaviors, significantly improving the efficiency of species identification and population tracking.
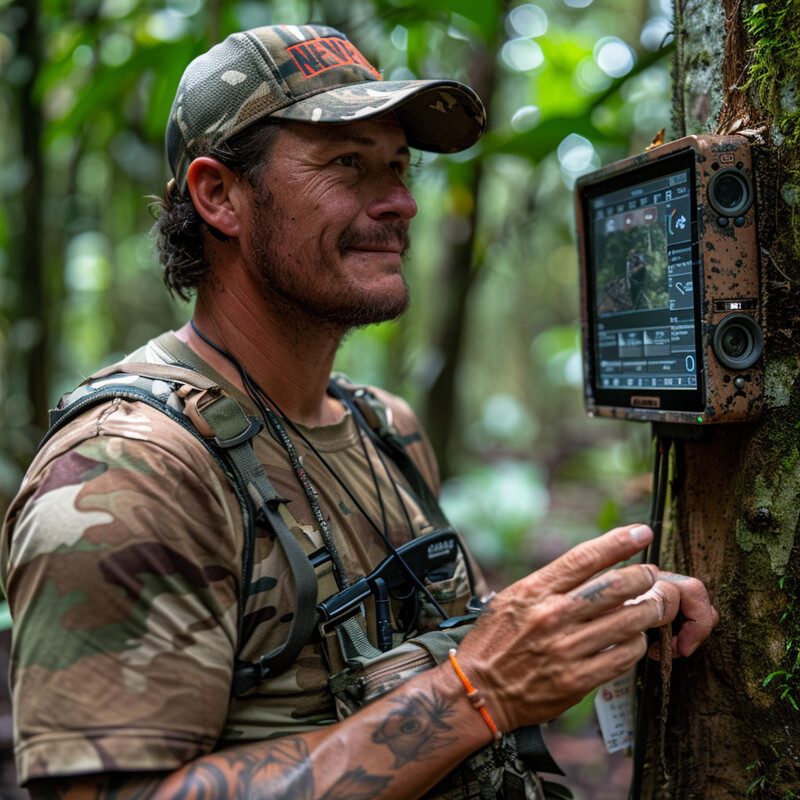
AI enhances wildlife conservation efforts by analyzing images and videos captured by camera traps in natural habitats. Using image recognition and machine learning, AI can quickly identify species, count individual animals, and monitor their behaviors without human bias. This technology enables conservationists to track population trends and study wildlife in a non-invasive manner, contributing to more informed conservation strategies.
5. Deforestation Detection
AI algorithms process satellite images to detect changes in forest cover, helping to identify and respond to illegal logging activities and deforestation quickly.
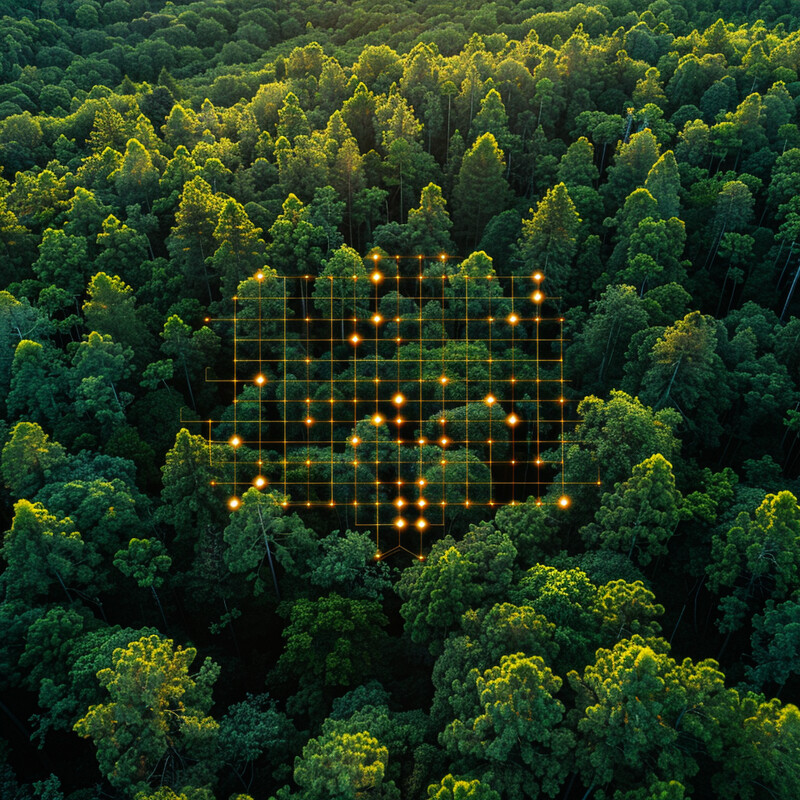
AI processes satellite imagery to monitor changes in forest cover over time, detecting areas affected by deforestation and illegal logging activities. By providing real-time alerts about such changes, AI enables quicker responses from local and global conservation organizations, helping to preserve biodiversity and combat climate change impacts associated with deforestation.
6. Natural Disaster Prediction and Response
AI enhances the prediction of natural disasters such as hurricanes, earthquakes, and floods, and coordinates response efforts by analyzing real-time data from multiple sources.
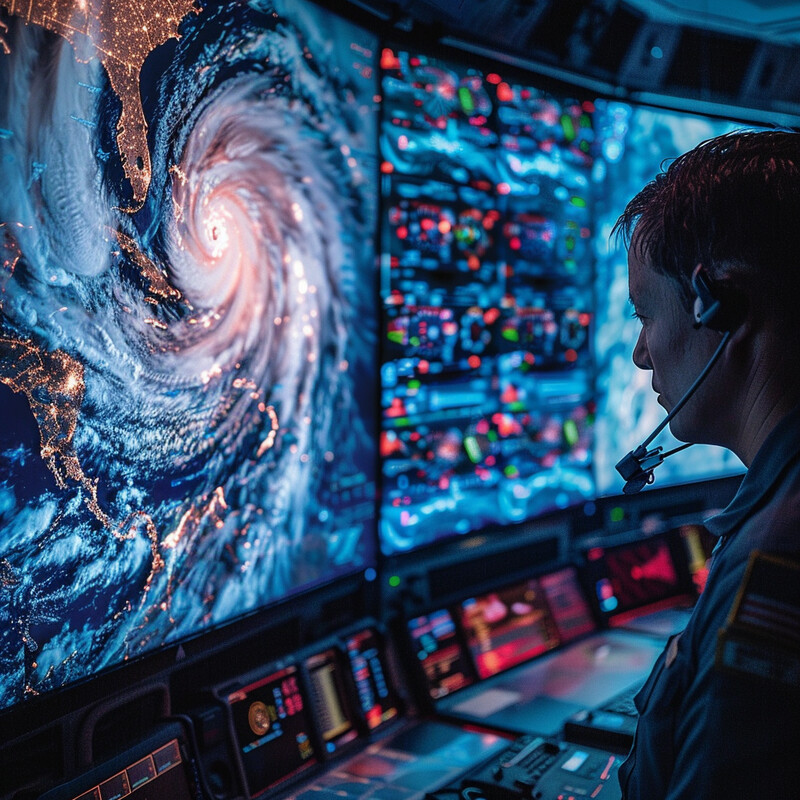
AI improves the prediction and management of natural disasters through real-time analysis of data from seismic sensors, weather satellites, and other monitoring devices. AI models predict the path and intensity of hurricanes, the likelihood of earthquakes, and potential flood zones. This predictive capability allows emergency services to prepare and respond more effectively, potentially saving lives and reducing property damage.
7. Agricultural Monitoring
AI models predict crop yields, detect plant diseases, and suggest optimal planting strategies by analyzing data from drones and satellite images, promoting sustainable farming practices.
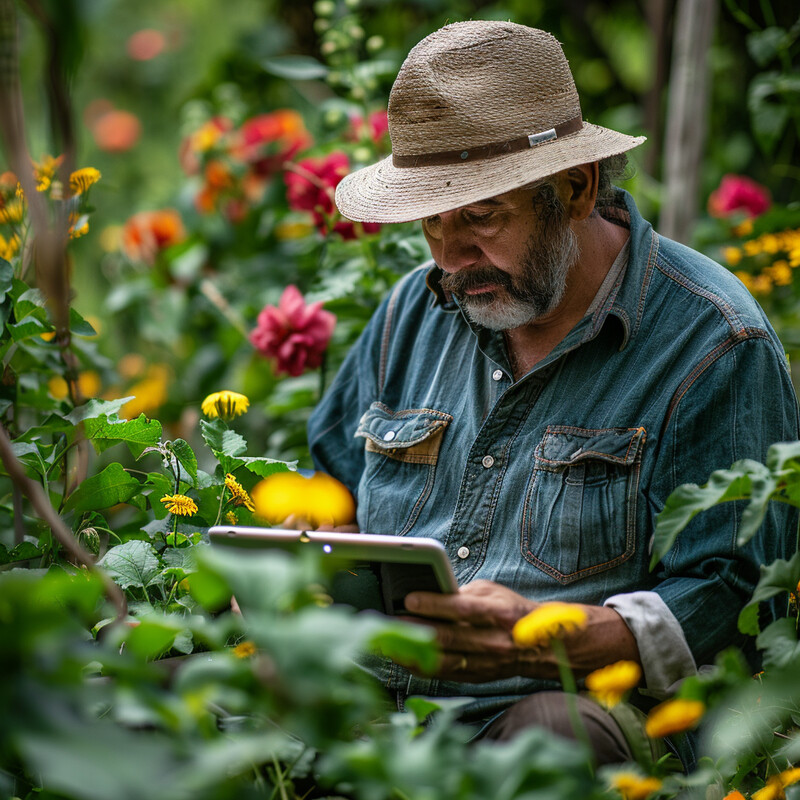
AI-driven agricultural monitoring involves analyzing data from drones and satellites to assess crop health, predict yields, and detect signs of disease or pest infestation. These insights enable farmers to apply water, fertilizers, and pesticides more efficiently, leading to higher yields and more sustainable farming practices that minimize environmental impact.
8. Energy Consumption Analysis
AI monitors and analyzes energy consumption patterns, identifying opportunities for energy savings and helping to reduce the carbon footprint of buildings and industries.
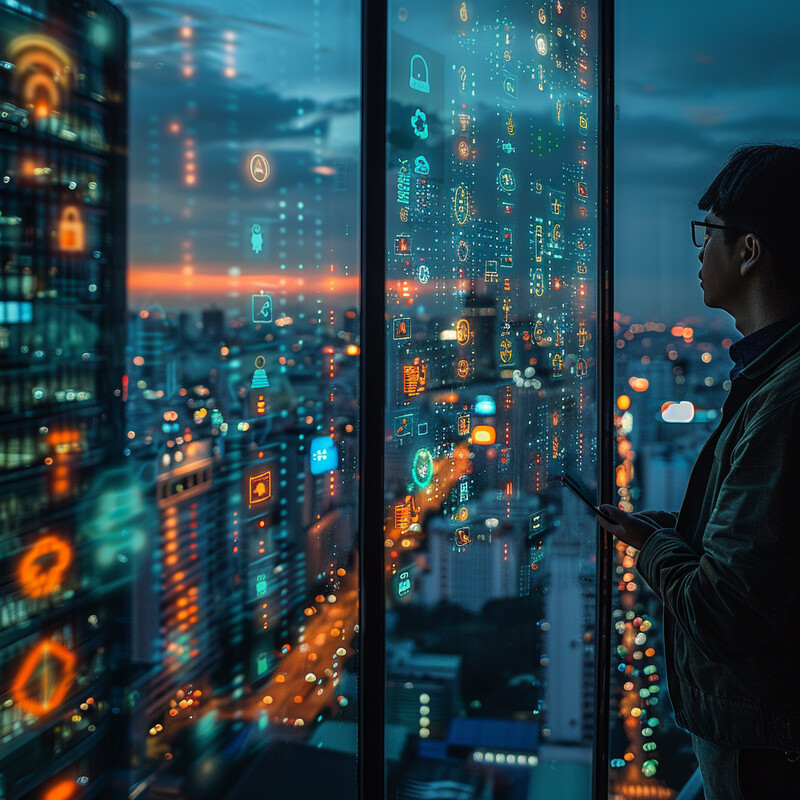
AI analyzes energy consumption data from residential and industrial sources to identify patterns and inefficiencies. This analysis helps to optimize energy use, reducing unnecessary waste and promoting energy conservation measures. AI-driven systems can automatically adjust heating, lighting, and air conditioning in buildings to improve energy efficiency and reduce the carbon footprint.
9. Waste Management Optimization
AI improves waste management by automating the sorting of recyclables and optimizing collection routes based on real-time waste generation data.
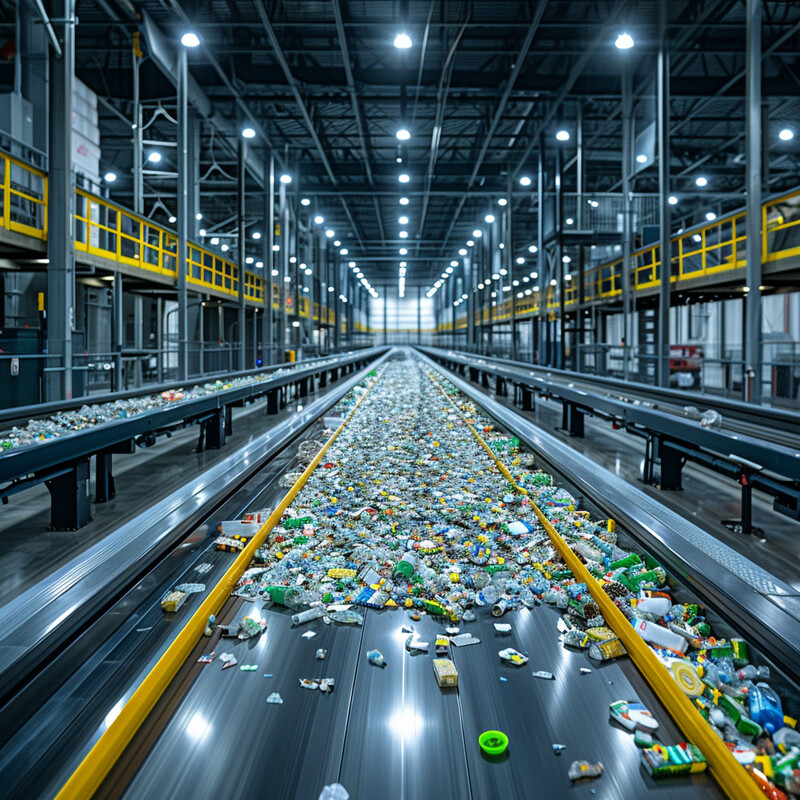
In waste management, AI optimizes the sorting of recyclables from other waste, enhancing recycling rates and reducing landfill use. AI also analyzes waste generation data to optimize collection routes and schedules, improving the efficiency of waste collection services and reducing associated emissions.
10. Ocean Health Monitoring
AI systems analyze data from ocean sensors to track changes in marine environments, such as water temperature, pH levels, and pollution, aiding in the conservation of marine biodiversity.
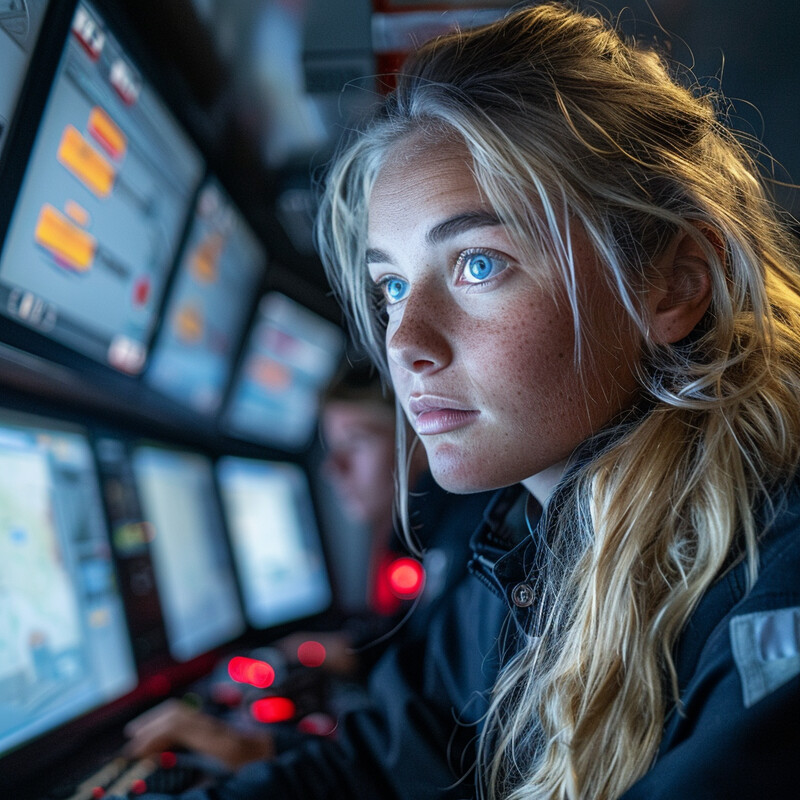
AI monitors the health of marine environments by analyzing data from sensors that measure water temperature, acidity (pH levels), salinity, and contaminant levels. This monitoring helps scientists detect changes that could threaten marine biodiversity, such as coral bleaching or pollution spikes, and provides critical data for efforts to conserve ocean health.