1. Threat Detection
AI algorithms are adept at detecting new and emerging threats by analyzing patterns and anomalies in data, significantly faster than traditional methods.
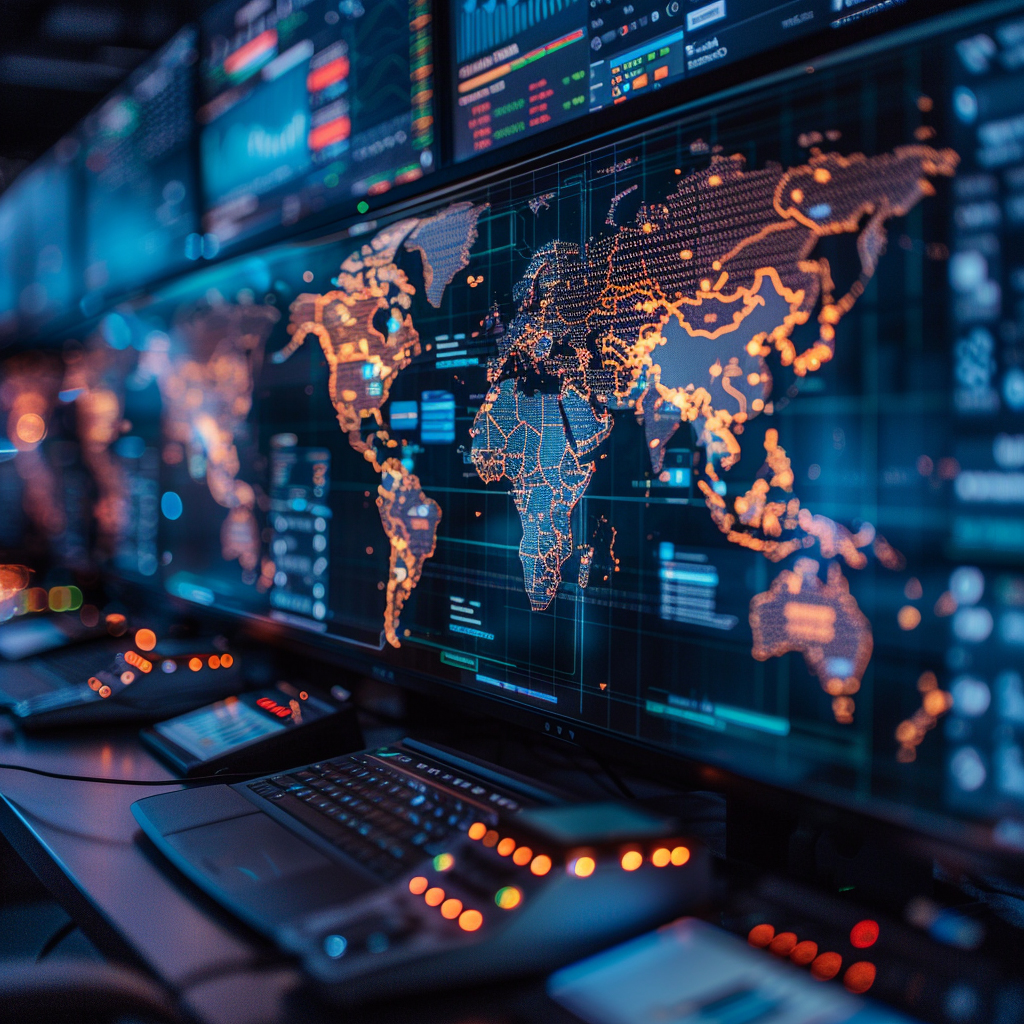
AI algorithms excel at identifying emerging cybersecurity threats by analyzing patterns and anomalies in vast amounts of data quickly and accurately. These systems can adapt to new and evolving threats more efficiently than traditional methods, which often rely on known threat signatures. AI's capability to detect zero-day exploits and previously unrecognized malware helps organizations stay ahead of potential breaches.
2. Behavioral Analytics
AI can monitor user behavior to detect unusual activity that could indicate a security breach, such as unexpected access attempts or large data transfers.
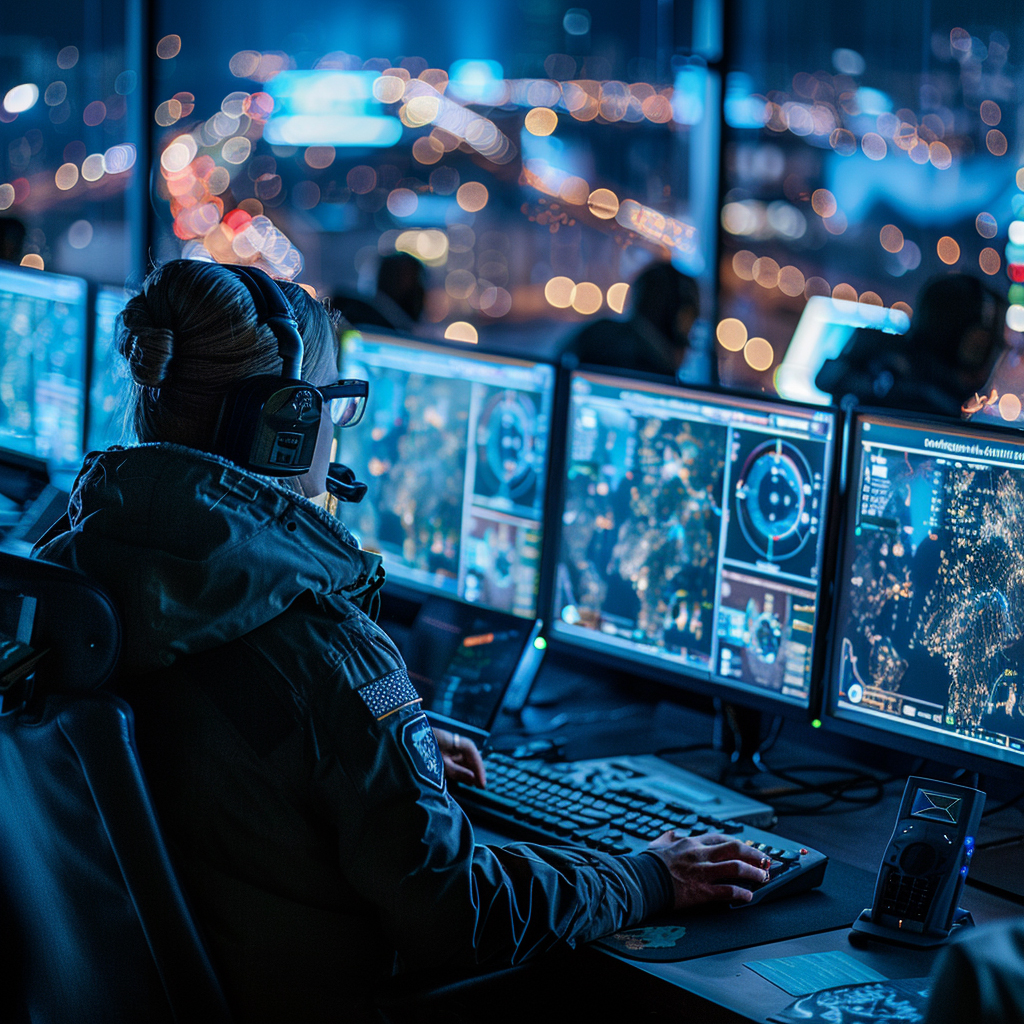
AI-driven behavioral analytics are crucial for detecting insider threats and external attacks by monitoring user activities across networks and systems. By establishing a baseline of normal behavior for each user, AI can flag deviations that may indicate a compromise, such as unusual login times, locations, or unauthorized access attempts. This early detection is key to preventing data leaks or other security incidents.
3. Incident Response
AI enhances the speed and efficiency of incident response by automatically taking action against detected threats, such as isolating affected systems or blocking suspicious IP addresses.
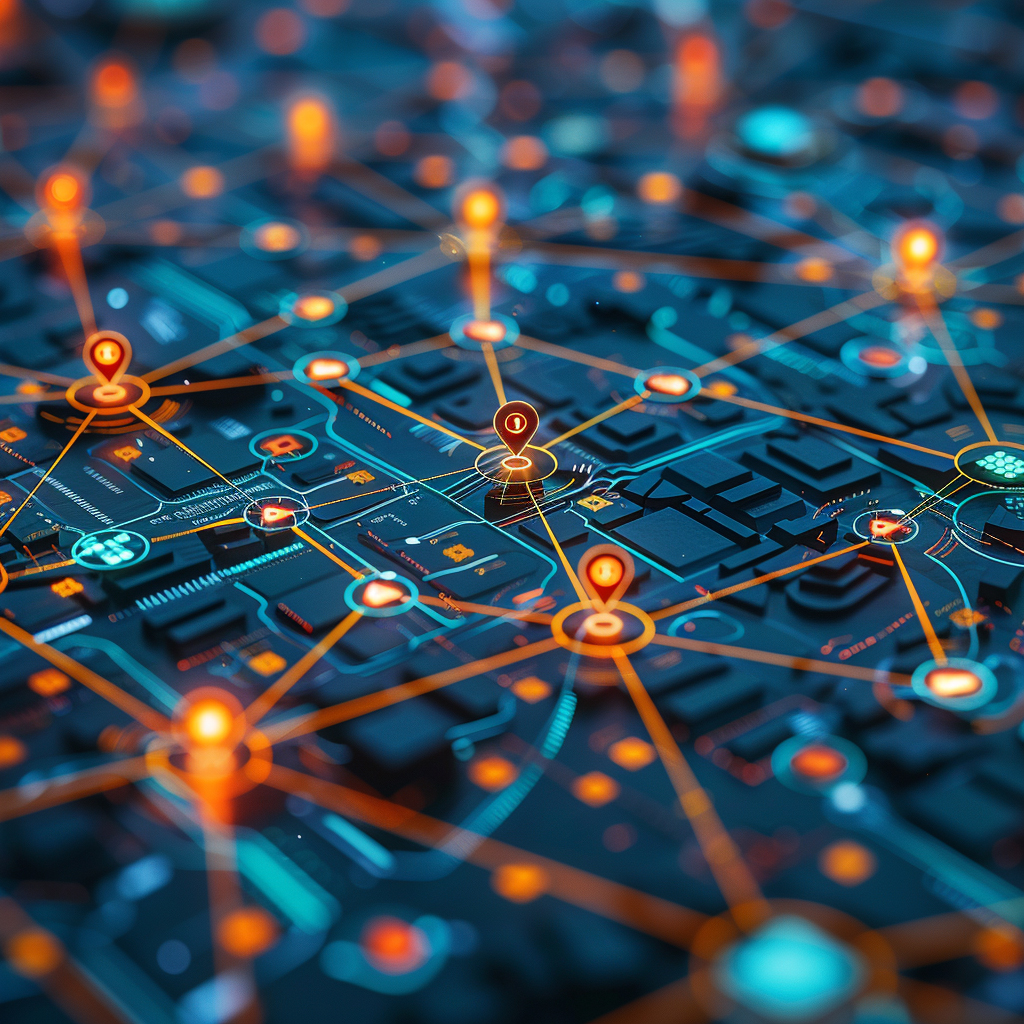
AI enhances incident response by automating reactions to security threats. Once a potential threat is detected, AI systems can initiate responses such as isolating affected systems, shutting down certain operations, or blocking suspicious IP addresses. This rapid response can limit damage and prevent the spread of the attack, significantly reducing the incident's impact.
4. Vulnerability Management
AI systems can identify and prioritize vulnerabilities in software and networks based on their risk level, helping organizations to patch critical weaknesses before they are exploited.
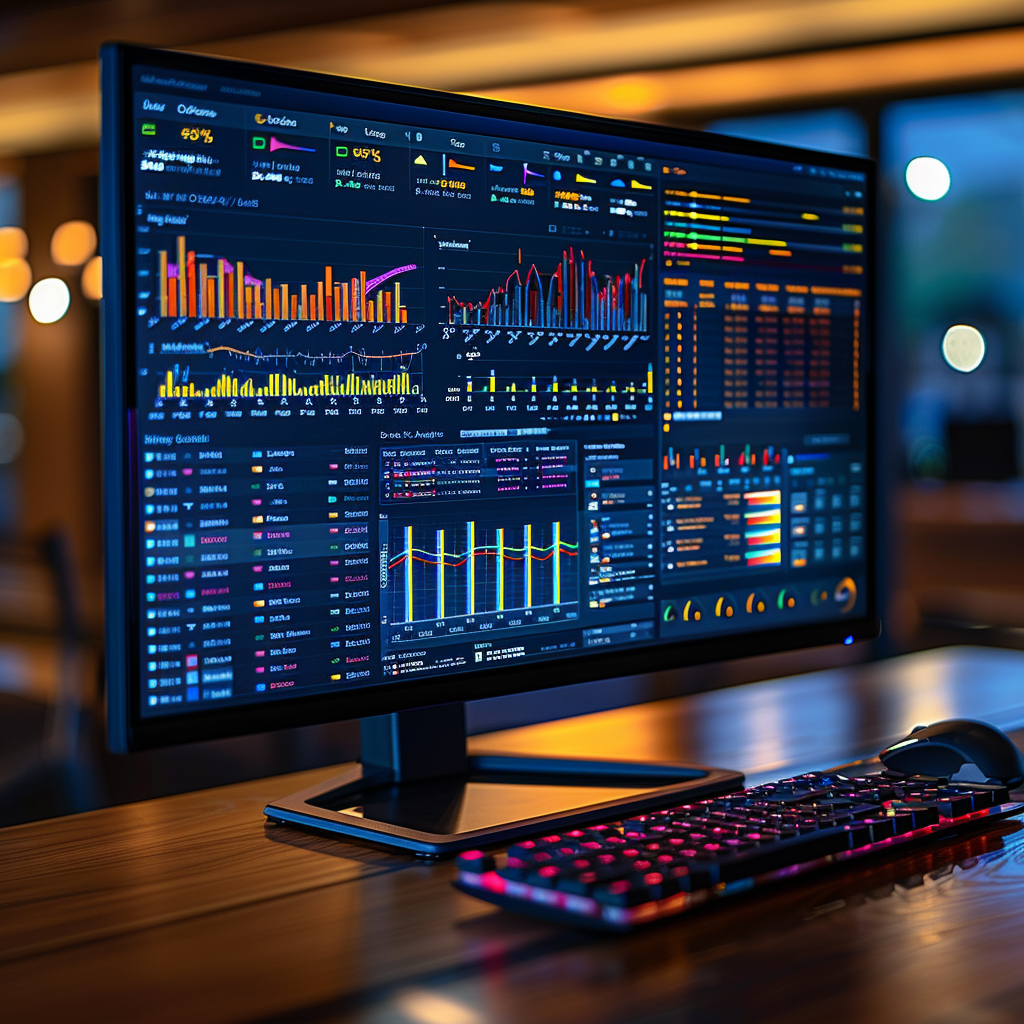
AI systems help in the identification and prioritization of vulnerabilities within an organization’s networks and applications. By analyzing the potential impact and exploitability of each vulnerability, AI can help security teams focus on patching the most critical weaknesses first, thereby optimizing resource allocation and strengthening the security posture more effectively.
5. Phishing Detection
AI improves the detection of phishing attempts by analyzing the content of emails and web pages to identify malicious intent, even when traditional signature-based methods fail.
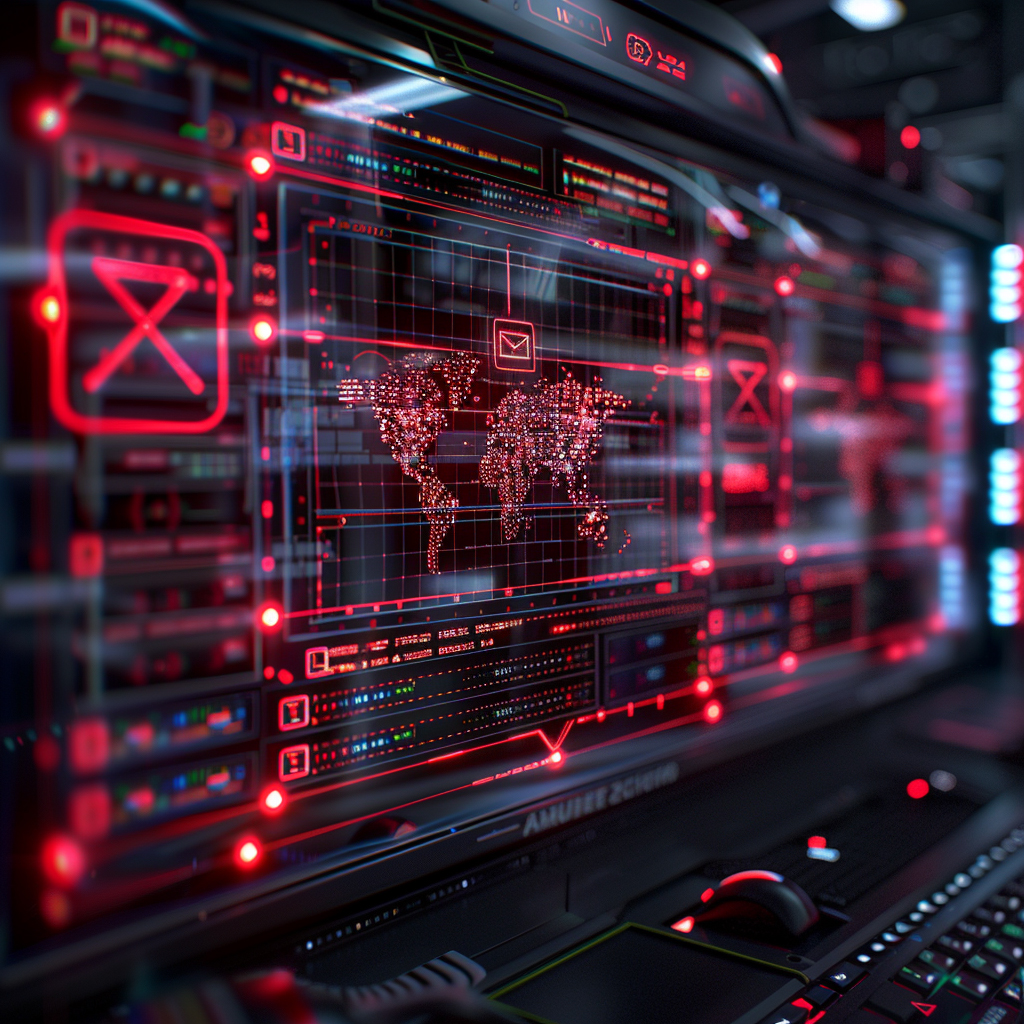
AI significantly improves the detection of phishing attempts by analyzing the text and metadata of emails, as well as the content of linked websites. AI models are trained to recognize subtle cues that indicate phishing, such as slight abnormalities in sender addresses or malicious links, providing a robust defense against one of the most common vectors for cyber attacks.
6. Network Security
AI models can monitor network traffic in real time to detect unusual patterns that may signify a cyber attack, such as distributed denial of service (DDoS) attacks.
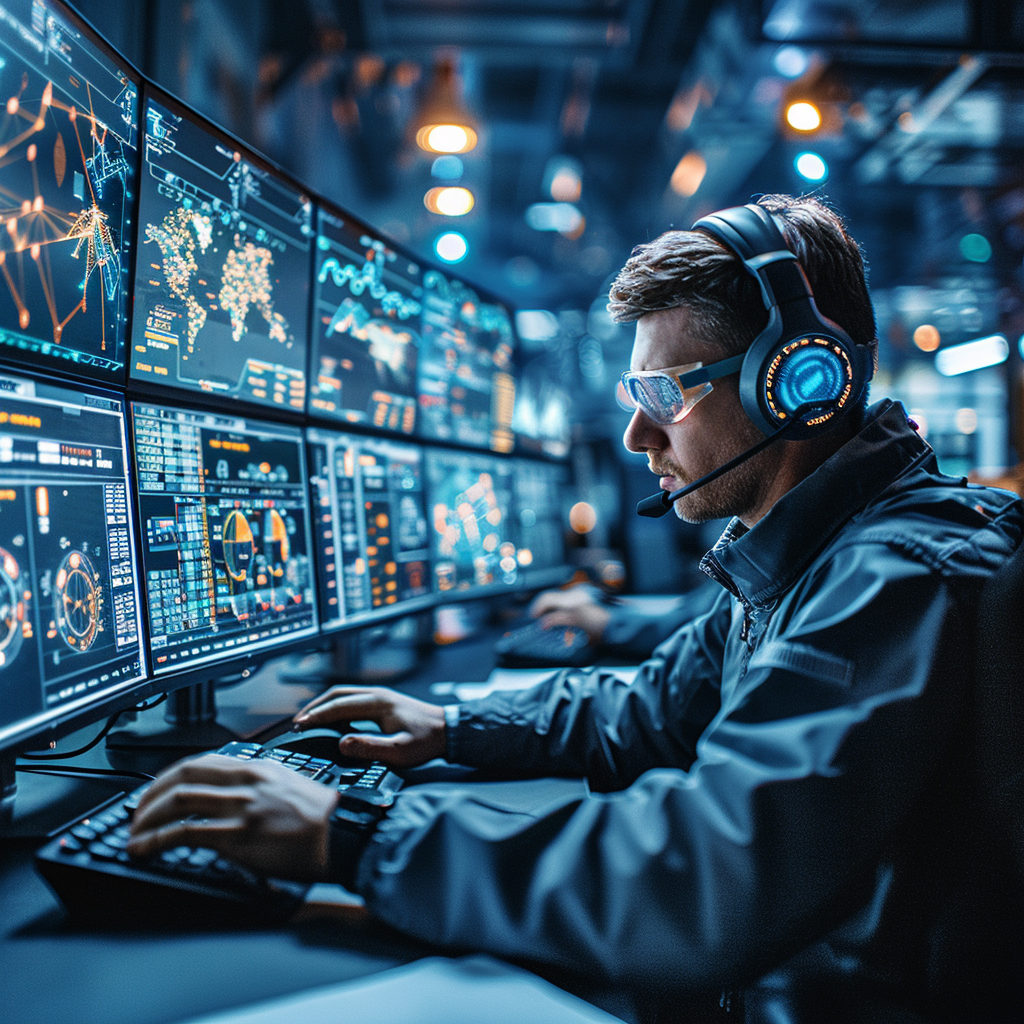
AI models continuously monitor network traffic to detect anomalies that could indicate cyber threats, including DDoS attacks or unauthorized data exfiltration. By analyzing traffic flows and comparing them to established patterns, AI can identify suspicious activities and initiate protective measures in real time, safeguarding network integrity.
7. Fraud Detection
AI is used in detecting fraudulent activities in various sectors, especially in financial services, by analyzing transaction patterns and flagging irregularities.
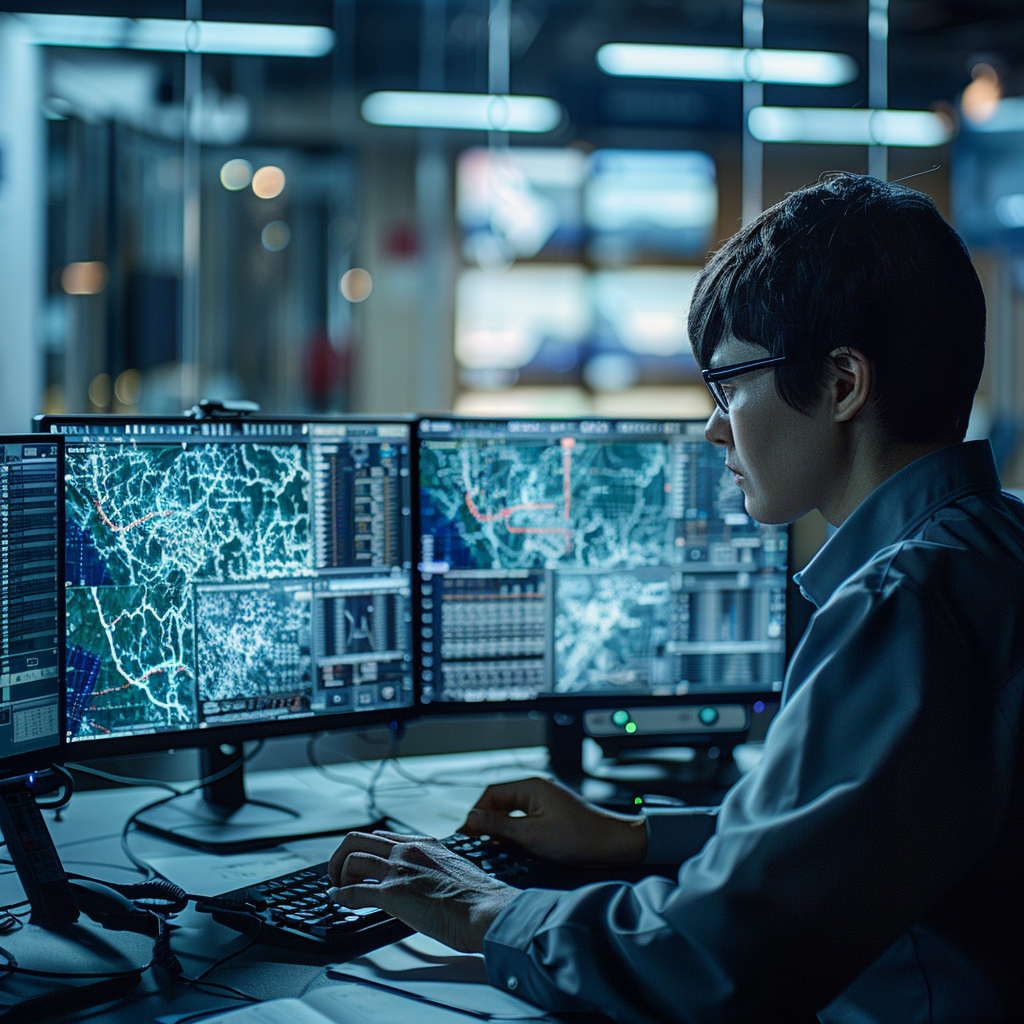
In sectors like banking and e-commerce, AI algorithms analyze transaction patterns to detect fraudulent activities. These systems can identify inconsistencies or anomalies that deviate from typical user behavior, such as unusual transaction locations or amounts, alerting security teams and helping prevent financial losses.
8. Secure Authentication
AI enhances security by supporting biometric authentication methods, such as facial recognition and fingerprint scanning, making unauthorized access much more difficult.
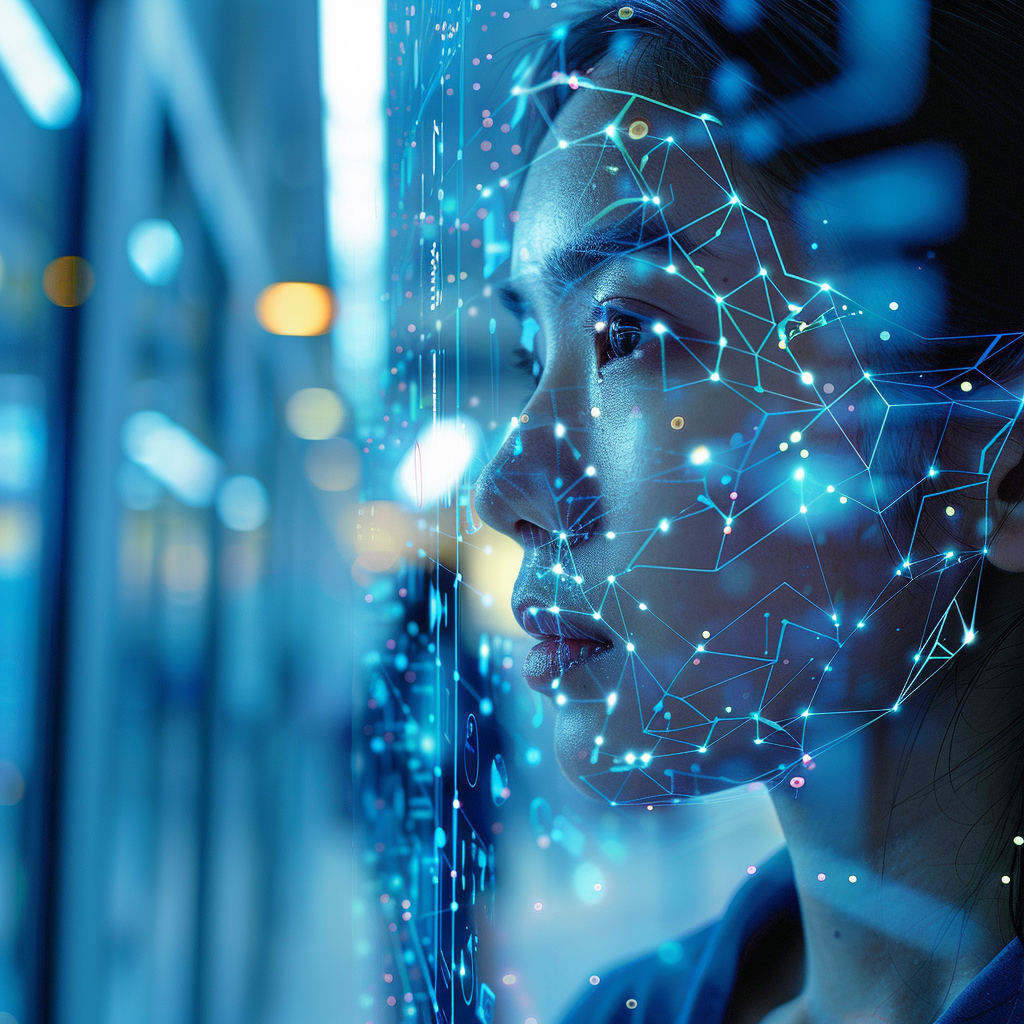
AI supports more secure authentication methods by integrating advanced biometric technologies, such as facial recognition, iris scanning, and fingerprint analysis. These methods provide a higher level of security than traditional passwords, as they are difficult to replicate or forge, thereby reducing the risk of unauthorized access.
9. Automated Security Audits
AI can conduct continuous and automated security audits to ensure compliance with security policies and standards, significantly reducing the manual workload.
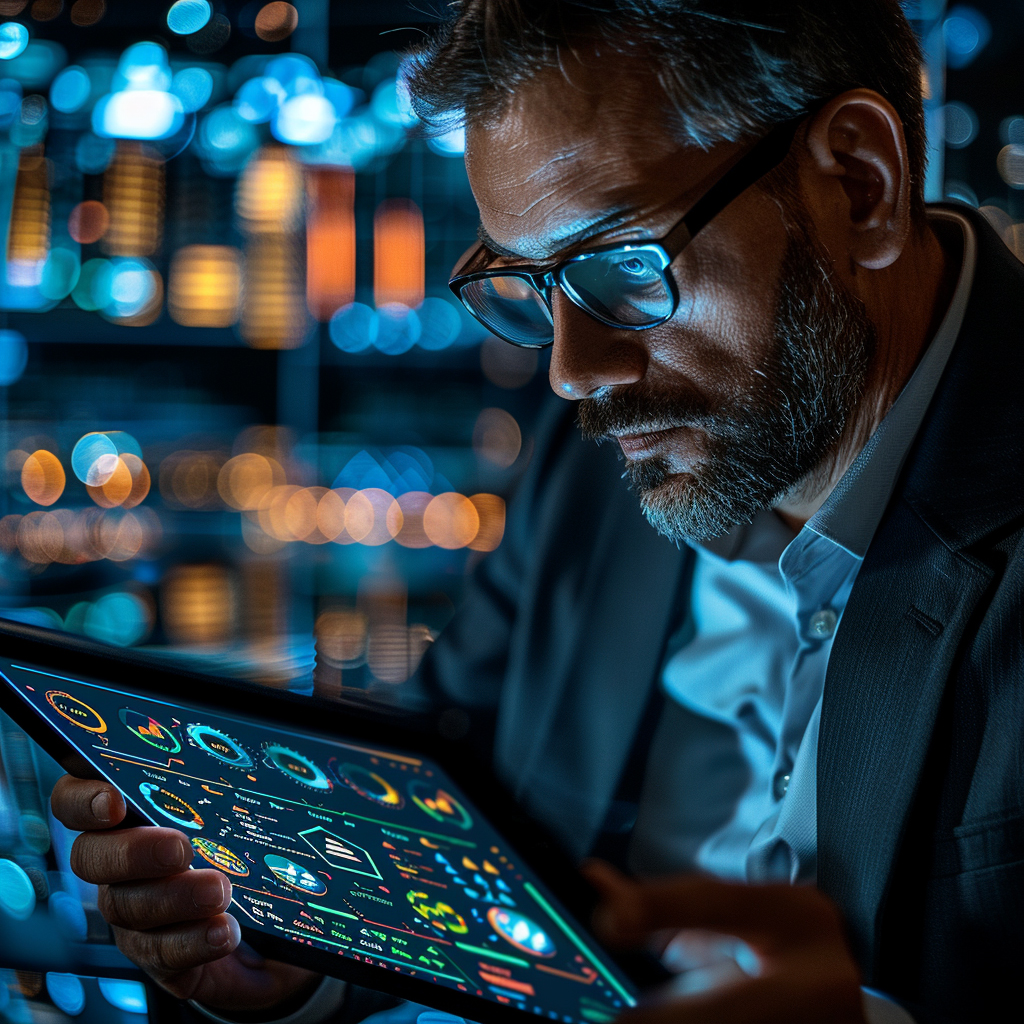
AI can automate the process of security audits, continuously checking an organization’s adherence to security policies and regulatory requirements. This automation helps ensure consistent compliance and reduces the burden on security teams by identifying and rectifying lapses in real time.
10. Advanced Encryption
AI aids in developing more complex encryption algorithms and managing encryption keys, improving the security of data transmissions and storage.
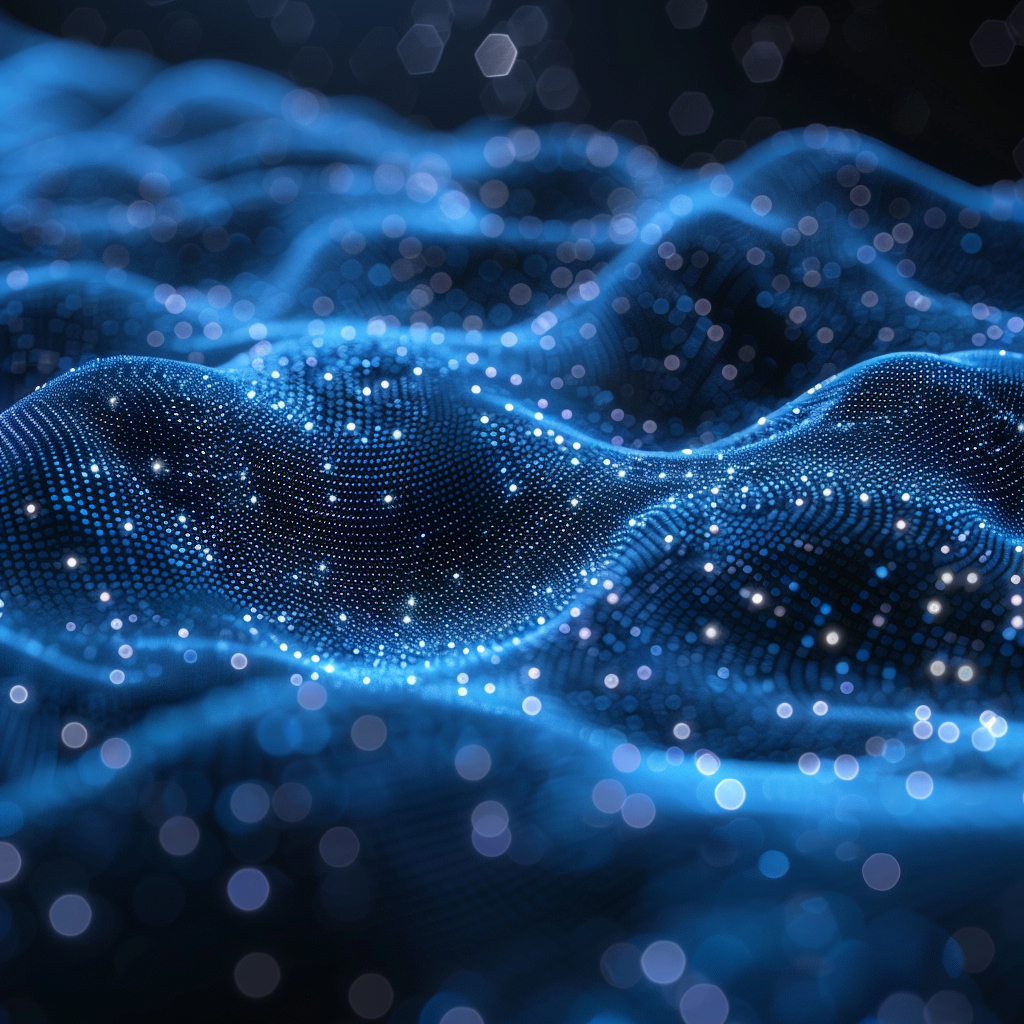
AI contributes to the development and management of advanced encryption techniques, enhancing the security of data in transit and at rest. AI can help manage encryption keys, generate more complex encryption algorithms, and ensure that data is protected against interception or unauthorized access.