1. Wildlife Monitoring and Conservation
AI can analyze data from camera traps and satellite images to track wildlife populations, monitor their movements, and detect poachers, helping in the conservation of endangered species and their habitats.
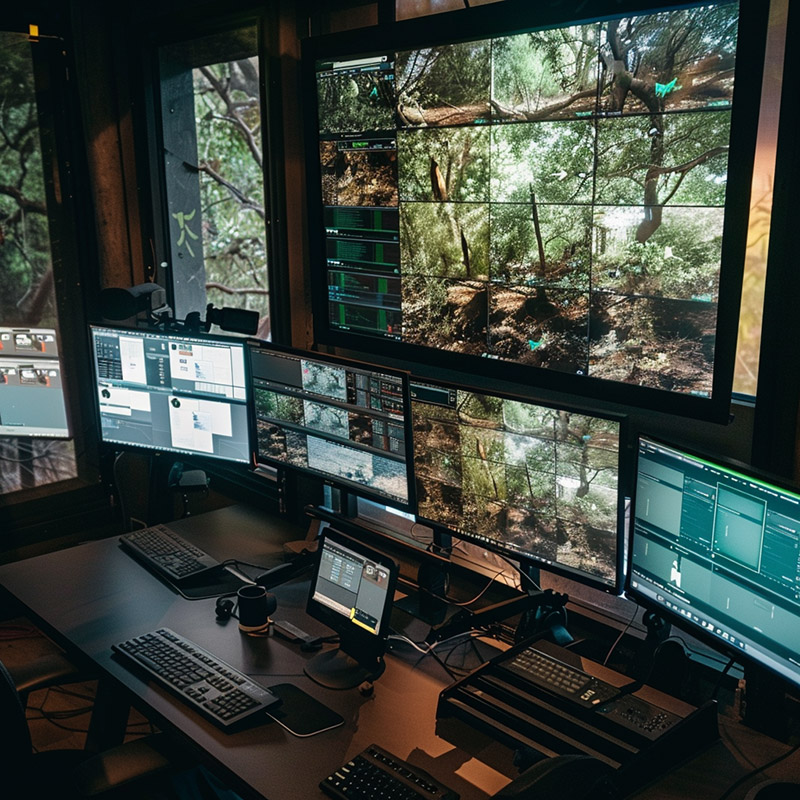
Wildlife Monitoring Before AI
Wildlife monitoring relied heavily on manual tracking and observation, which was time-consuming, labor-intensive, and often invasive, potentially disturbing natural habitats. Poaching and habitat destruction sometimes went undetected until significant damage had occurred.
Wildlife Monitoring After AI
AI enhances wildlife conservation efforts by automating the analysis of camera trap images and satellite data, enabling the identification and tracking of species across vast areas with minimal human intrusion. This allows for real-time monitoring and the swift detection of threats like poaching or habitat encroachment, leading to more effective protection measures for endangered species and their ecosystems.
2. Precision Agriculture
AI-driven precision agriculture utilizes data analytics and machine learning to optimize crop yields and reduce waste by monitoring soil conditions, weather data, and plant health, thereby minimizing the use of water, fertilizers, and pesticides.
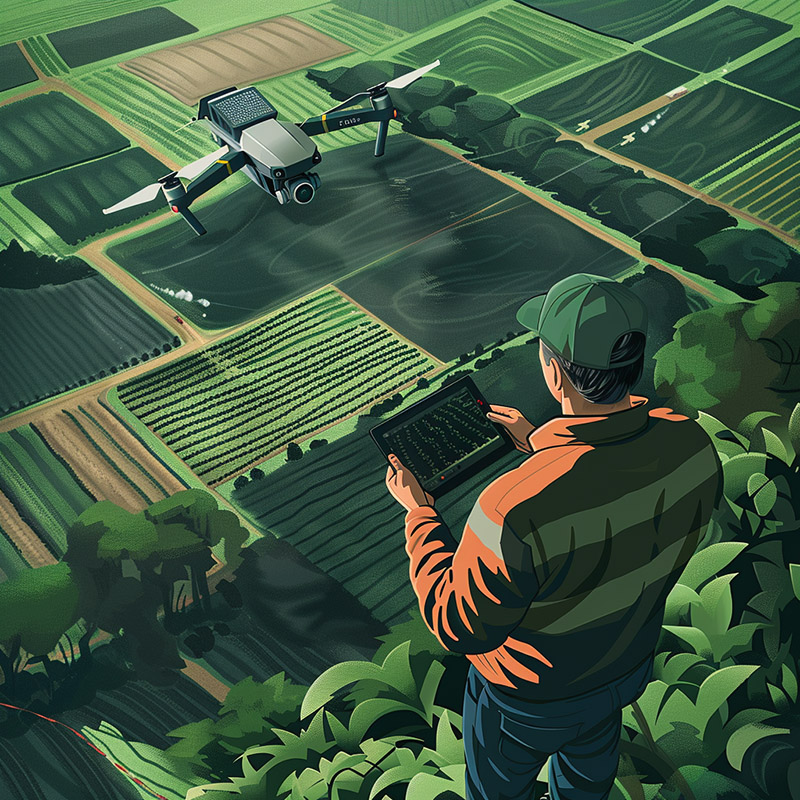
Farming Before AI
Traditional farming practices often resulted in the overuse of water, fertilizers, and pesticides, contributing to environmental degradation, including soil depletion and water pollution.
Farming After AI
AI-driven precision agriculture optimizes resource use, applying water, fertilizers, and pesticides only where needed based on data from sensors and satellites. This targeted approach reduces environmental impact, promotes sustainable farming practices, and increases crop yields, contributing to food security with lower ecological footprint.
3. Energy Efficiency in Buildings
AI systems can manage and optimize energy use in buildings, learning usage patterns and automatically adjusting heating, cooling, and lighting to reduce energy consumption and carbon footprints.
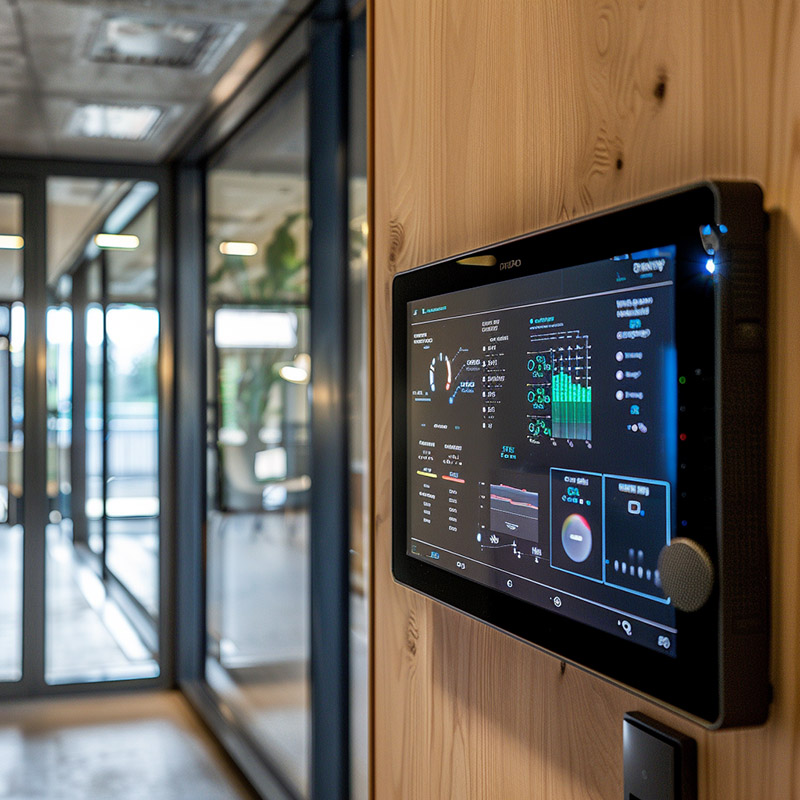
Energy Use Before AI
Buildings traditionally consumed energy inefficiently due to generic heating, cooling, and lighting systems that didn't adapt to occupancy or weather conditions, contributing significantly to carbon emissions.
Energy Use After AI
AI-powered systems now manage energy consumption in buildings dynamically, adjusting to real-time occupancy patterns and external weather conditions. This reduces energy waste, lowers carbon emissions, and cuts operational costs, making buildings more sustainable and environmentally friendly.
4. Air Quality Monitoring
AI can process vast amounts of environmental data from sensors to monitor air quality in real-time, identify pollution sources, and predict pollution levels, helping cities to implement timely measures to protect public health and the environment.
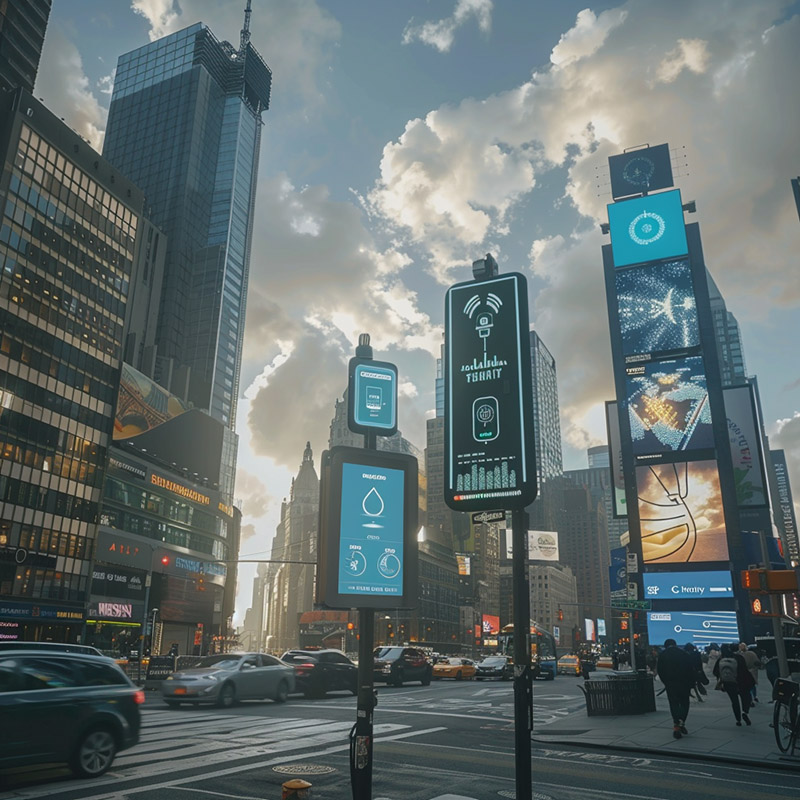
Air Quality Monitoring Before AI
Air quality monitoring was often limited to specific locations with stationary sensors, providing incomplete data that could delay responses to pollution events, impacting public health and the environment.
Air Quality Monitoring After AI
With AI, air quality is monitored comprehensively using data from a widespread network of sensors, including mobile and satellite sources. AI algorithms analyze this data in real-time, identifying pollution sources and predicting air quality trends, enabling proactive measures to protect public health and the environment.
5. Smart Grid Management
AI enhances the efficiency of electricity distribution through smart grids, integrating renewable energy sources seamlessly and balancing supply and demand in real-time, thereby reducing reliance on fossil fuels and lowering greenhouse gas emissions.
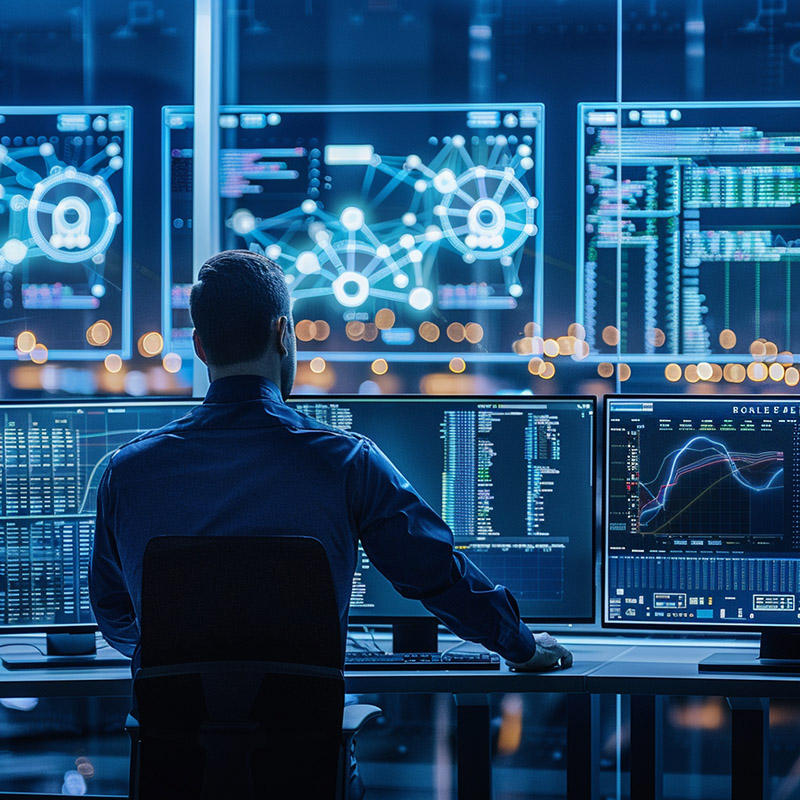
Electrical Grids Before AI
Traditional electricity grids operated on a centralized, one-way system that struggled to integrate renewable energy sources efficiently, leading to wasted energy and higher reliance on fossil fuels.
Electrical Grids After AI
AI revolutionizes smart grid management by facilitating the integration of renewable energy, optimizing electricity flow based on demand and supply, and preventing outages. This leads to a more resilient, efficient, and sustainable energy system that reduces greenhouse gas emissions and supports the transition to clean energy.
6. Forest Fire Detection and Management
By analyzing satellite imagery and sensor data, AI can detect early signs of forest fires, predict their spread, and optimize the allocation of firefighting resources, mitigating damage to ecosystems.
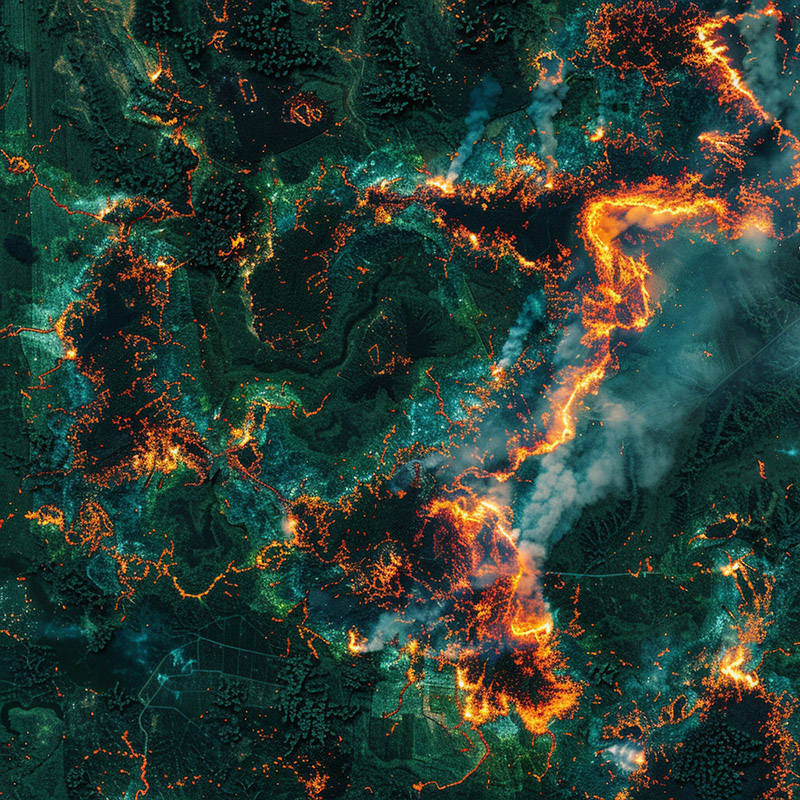
Fire Management Before AI
Detecting and managing forest fires relied on manual observation and reporting, often resulting in delayed responses that allowed fires to grow uncontrollably, causing extensive environmental and property damage.
Fire Management After AI
AI significantly improves early detection of forest fires through satellite imagery and sensor data analysis, enabling quicker response times and more effective resource allocation for firefighting. Predictive modeling also helps manage and mitigate the risk of future fires, protecting ecosystems and reducing carbon emissions from large-scale burns.
7. Water Resource Management
AI algorithms can predict water demand and supply, monitor water quality, and optimize the distribution of water resources, ensuring sustainable water management and reducing wastage.
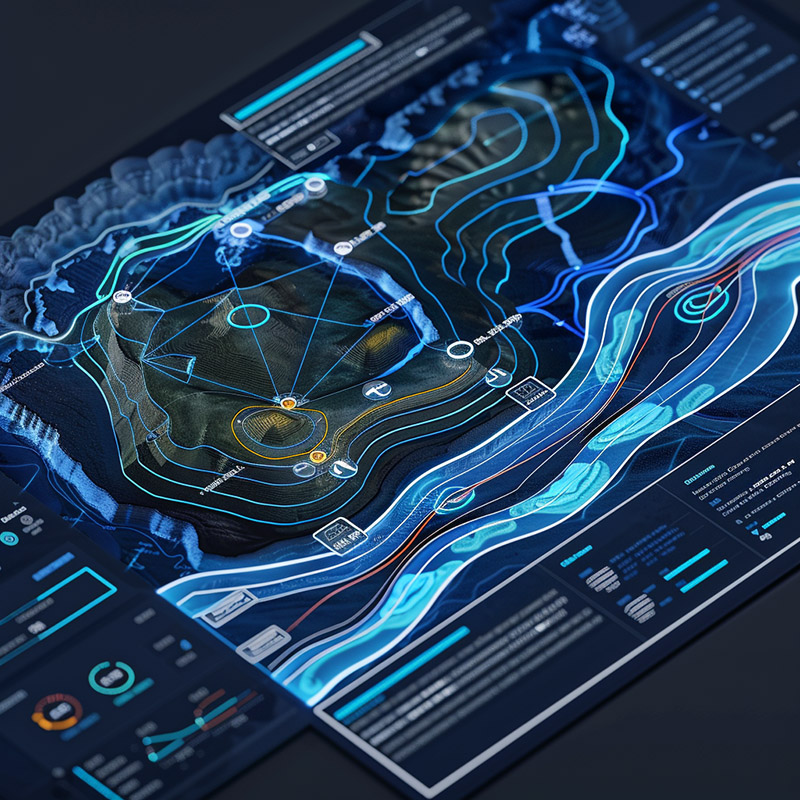
Water Management Before AI
Water resource management was often reactive and inefficient, with limited ability to predict demand, leading to water scarcity or excess in various regions, negatively impacting both human populations and natural ecosystems.
Water Management After AI
AI transforms water management by predicting supply and demand with high accuracy, optimizing distribution, and enhancing conservation efforts. This ensures sustainable water use, reduces waste, and protects aquatic ecosystems, contributing to the overall health of the planet.
8. Waste Management and Recycling
AI can streamline waste management processes, from identifying and sorting recyclable materials more efficiently to optimizing collection routes and reducing operational costs, thereby enhancing recycling rates and minimizing landfill use.
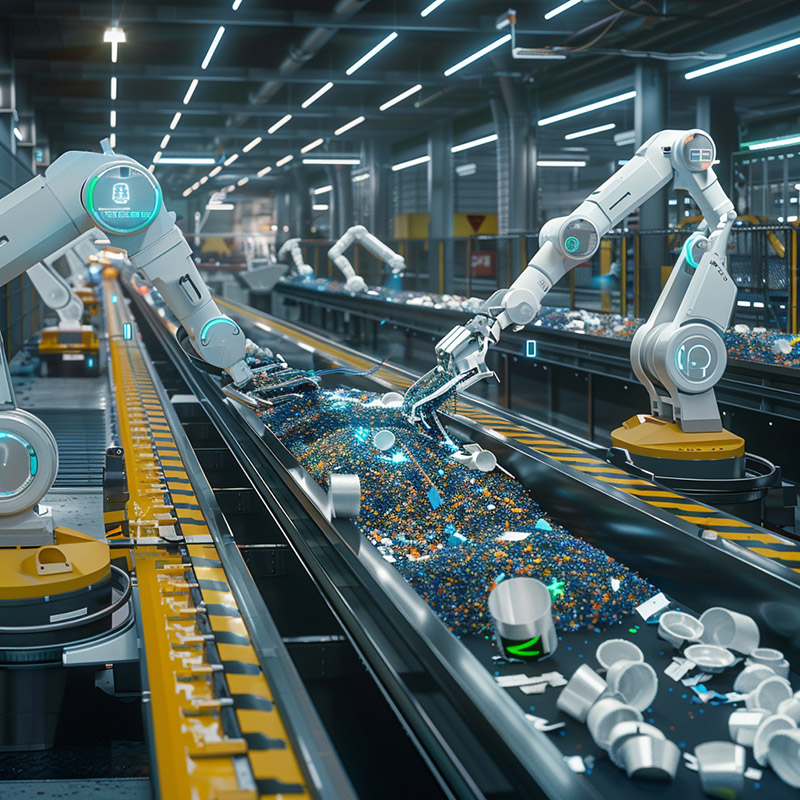
Waste Management Before AI
Waste management systems were inefficient, with much recyclable material ending up in landfills due to inadequate sorting processes, contributing to environmental pollution and resource depletion.
Waste Management After AI
AI revolutionizes waste management by automating sorting processes, enhancing the efficiency of recycling, and reducing landfill use. This contributes to a circular economy, minimizes environmental impact, and promotes the sustainable use of resources.
9. Carbon Footprint Analysis
AI tools can assess and track the carbon footprint of individuals, companies, and cities, providing insights and recommendations for reducing carbon emissions through changes in behavior, policies, and practices.
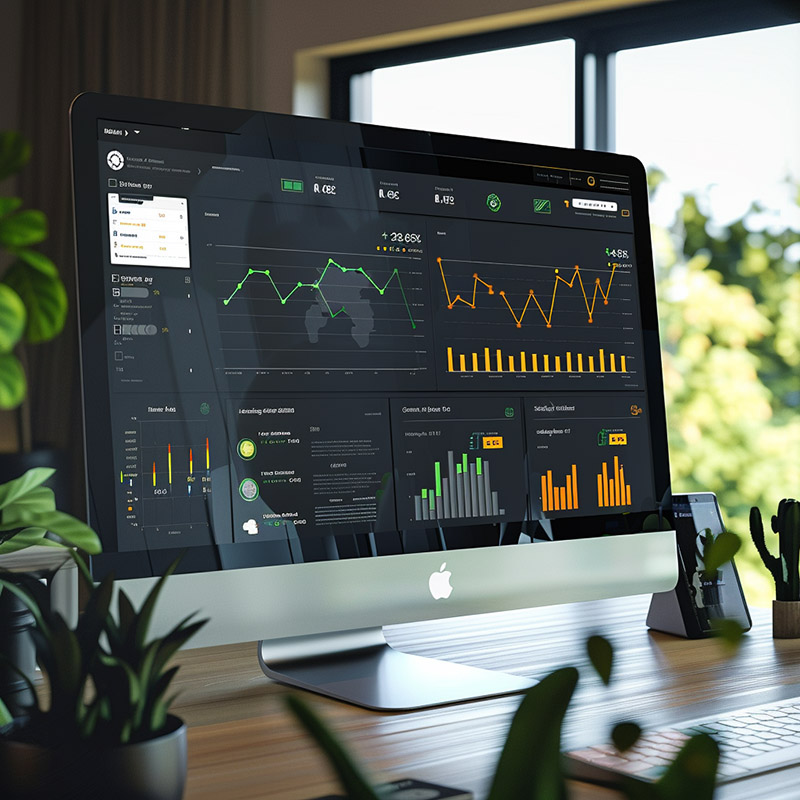
Carbon Analysis Before AI
Carbon footprint analysis was complex and resource-intensive, making it difficult for businesses and individuals to understand and reduce their environmental impact effectively.
Carbon Analysis After AI
AI simplifies and automates carbon footprint analysis, providing actionable insights for reducing emissions. This accessibility encourages more sustainable practices across industries and lifestyles, contributing to global efforts to combat climate change.
10. Climate Change Modeling and Prediction
AI enhances climate modeling, analyzing complex data from multiple sources to improve predictions about climate change impacts, enabling better preparation and adaptation strategies for natural disasters and changing weather patterns.
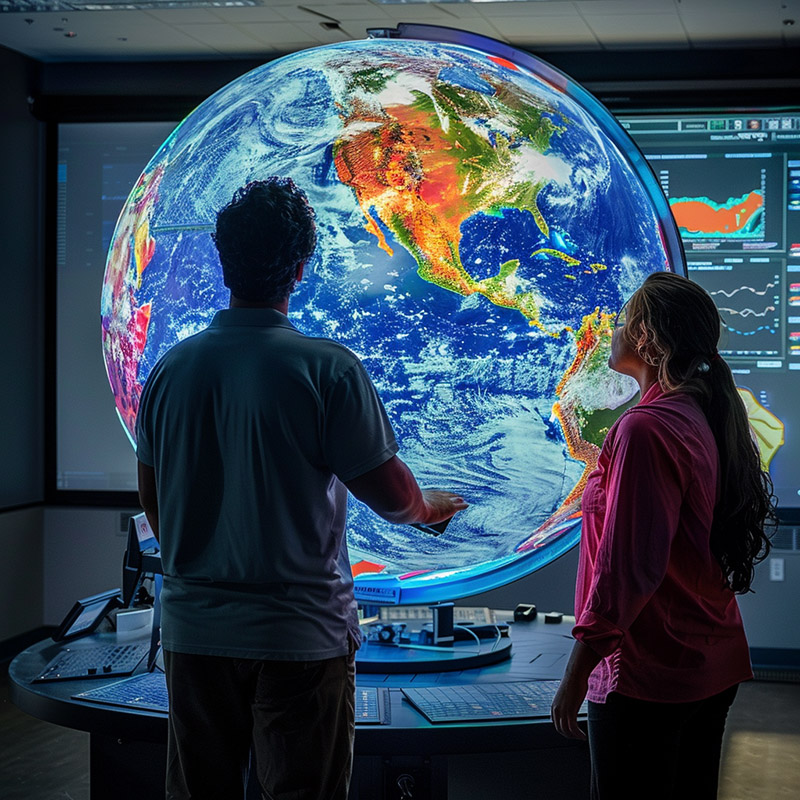
Climate Modeling Before AI
Climate models were less sophisticated, offering only broad predictions that made it challenging to plan for or mitigate the specific impacts of climate change on local and global scales.
Climate Modeling After AI
AI enhances climate change modeling and prediction by analyzing vast datasets for more accurate, detailed forecasts. This allows for better-informed decision-making on environmental policies, conservation efforts, and adaptation strategies, helping societies prepare for and mitigate the effects of climate change more effectively.