1. Multimodal Data Integration
Volcanic activity monitoring benefits from combining multiple data streams into unified analyses. By integrating seismic readings, ground deformation measurements, gas emissions, thermal images, and other observations, researchers can obtain a more comprehensive view of a volcano’s state. Multimodal data fusion helps cross-verify signals – for example, a slight uplift of the ground accompanied by increased gas output is more telling than either signal alone. AI algorithms excel at correlating such diverse inputs, highlighting subtle patterns that might be missed when examining each data type in isolation. Overall, integrating heterogeneous data sources improves eruption risk assessments by providing a holistic picture, reducing the chance that critical precursors go unnoticed.
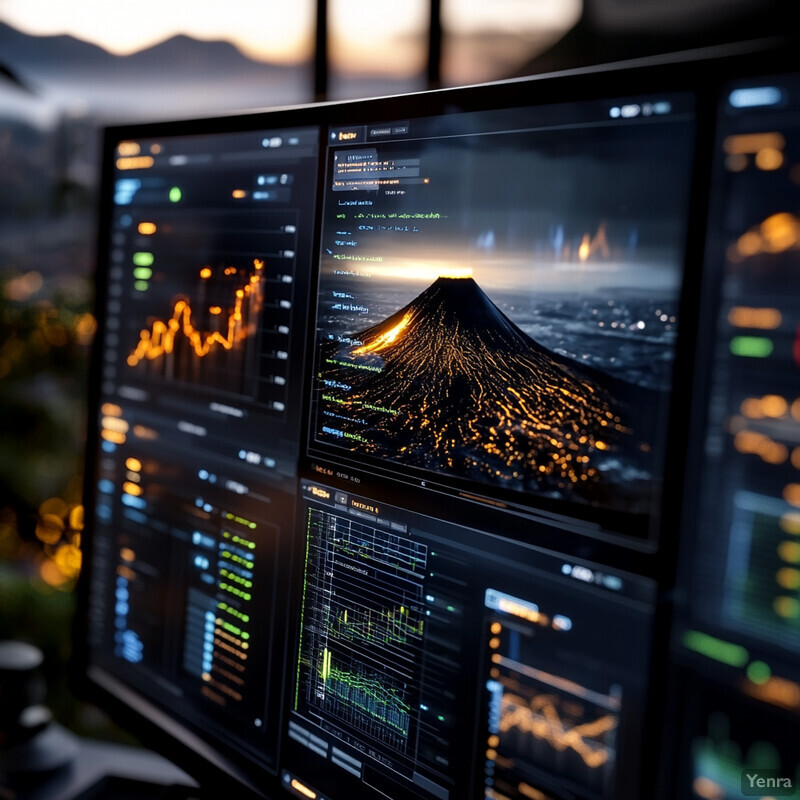
Modern AI-driven systems can merge data from satellites, field sensors, and observatories into a single risk model. For instance, a 2025 project on Mount Etna fused multi-spectral satellite observations (from MODIS, VIIRS, SLSTR, SEVIRI, etc.) to monitor lava output in near real time. The AI processed large volumes of heterogeneous inputs and produced reliable estimates of eruptive parameters such as lava discharge rates and total erupted volume. By demonstrating that machine learning can handle these “big data” streams, the study showed that a unified analysis can detect volcanic changes more efficiently than traditional, separate monitoring approaches. Such multimodal integration is increasingly seen as vital for early warning, as it consolidates various symptoms of unrest into one coherent assessment.
2. Automated Anomaly Detection in Seismic Signals
Machine learning is enabling more sensitive and continuous analysis of volcanic earthquake data. Traditionally, seismologists spend hours scanning seismic records for unusual tremors or quake swarms that might signal magma movement. AI-based anomaly detection can automate this process by learning a volcano’s normal “background” seismic pattern and then flagging deviations in real time. These systems are particularly good at catching subtle or emergent signals – for example, a slight increase in low-frequency tremor amplitude or an unusual clustering of small quakes. Early detection of these anomalies is critical, as they often precede eruptions or shifts in volcanic activity.
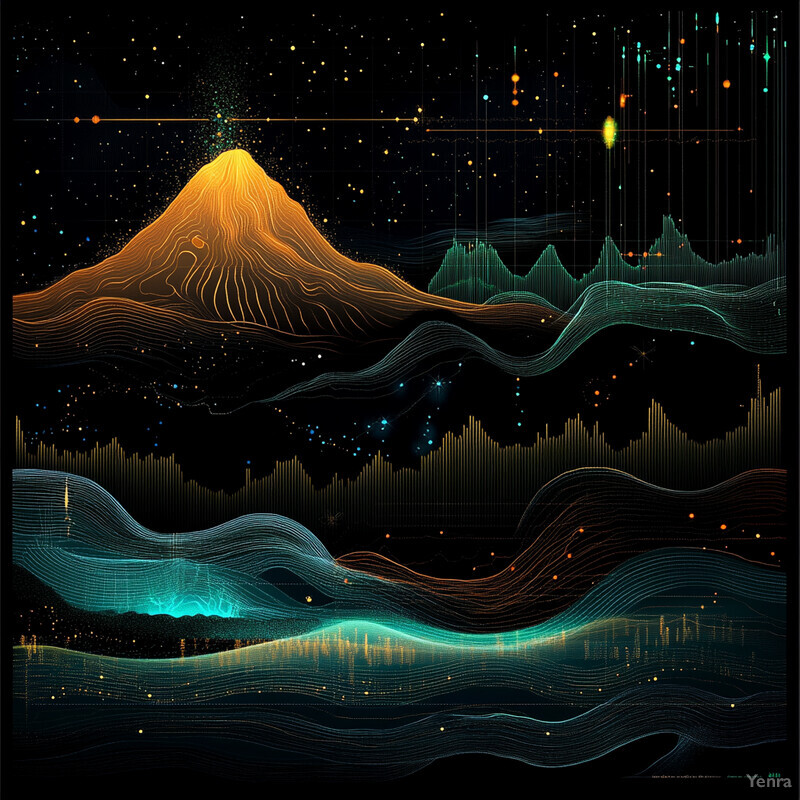
Researchers have developed AI models that identify abnormal seismic signals faster and more accurately than human observers. In Alaska, an automated system was trained to recognize the continuous seismic tremor at volcanoes and to distinguish it from ordinary earthquakes or noise. Implemented at Pavlof Volcano, this machine learning tool eliminated the need for an expert to manually review spectrograms around the clock. It learns the normal seismic “hum” of the volcano and raises an alert when persistent vibrations depart from that baseline. In tests published in 2024, the system successfully detected subtle volcanic tremors that signify magma or gas movement, thus providing earlier warnings of unrest. By catching these elusive vibration patterns, the AI-assisted approach can free up human analysts and improve short-term eruption forecasts.
3. Deep Learning for Ash Cloud Tracking
AI is improving how we monitor dangerous volcanic ash clouds in the atmosphere. When a volcano erupts explosively, it can inject ash high into the sky, posing risks to aviation and communities downwind. Traditional satellite-based tracking uses physical models and manual interpretation, which can be slow. Deep learning now offers a way to rapidly analyze satellite imagery for ash cloud presence, shape, and movement. By training convolutional neural networks on past eruption images, these models learn to recognize the distinct signature of ash (in thermal infrared bands, for example) and to follow its dispersion across frames. The result is near-real-time mapping of ash extent, which is crucial for issuing aviation warnings and public health advisories.
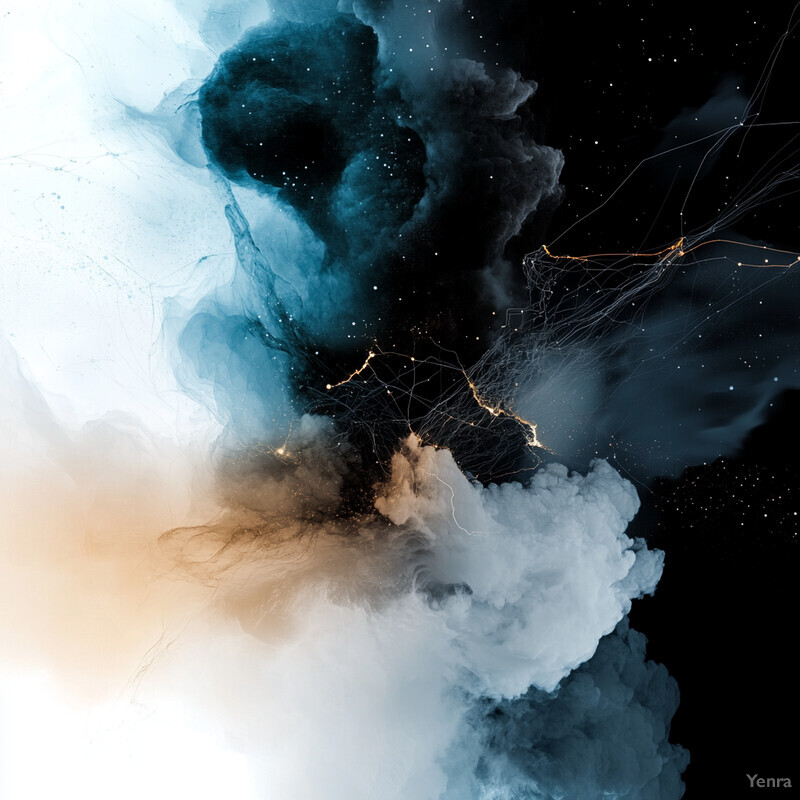
In 2024, scientists demonstrated a neural network that detects and follows volcanic ash clouds from space-based sensors. Torrisi et al. developed a CNN model (using a U-Net architecture with a VGG16 backbone) to process satellite infrared data and highlight ash-bearing pixels. Tested on eruptions in the Alaska–Aleutian region, the model could accurately delineate ash cloud boundaries and track their drift over time. The deep learning system achieved high detection performance – on a labeled test dataset it correctly identified ash cloud pixels with about 98% accuracy (F1-score 0.95). Using this AI method, the team generated automated ash cloud maps for 22 years of historical eruptions, showing how ash from specific volcanoes traveled under different wind conditions. These results indicate that deep learning can significantly speed up ash cloud monitoring, providing timely hazard information to aviation authorities compared to purely manual tracking approaches.
4. Long-Term Eruption Forecasting
AI techniques are being applied to improve eruption forecasting on timescales of weeks to months. Forecasting volcanic eruptions is notoriously difficult because each volcano has unique behavior and eruptions are rare events. Machine learning offers new ways to extract precursor patterns from historical data and even transfer knowledge from well-monitored volcanoes to those with sparse data. By analyzing long time series of seismicity, deformation, and other signals, algorithms can identify statistical changes that consistently occur before eruptions. These methods yield probabilistic forecasts – instead of a yes/no prediction, they provide the likelihood of an eruption in a given future window. Such long-term forecasting tools can support decision-makers in raising alert levels or evacuating areas when persistent unrest indicates an eruption may be likely.
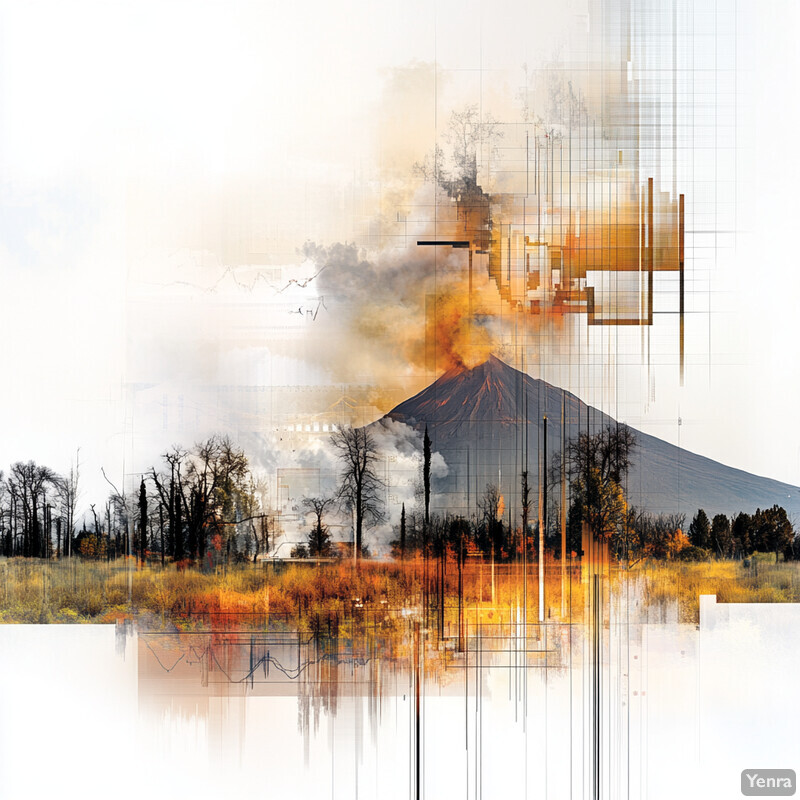
A recent breakthrough showed that transfer learning can enable eruption forecasting at volcanoes with limited data. Ardid et al. (2025) trained a machine learning model on seismic records from 41 eruptions across 24 different volcanoes. The model learned generic “precursor” patterns (such as increases in small earthquake rates or tremor energy) that often precede eruptions. Tested on a volcano outside its training set, this approach achieved forecasting accuracy comparable to a model trained specifically on that volcano. In practical terms, it means observations from well-studied volcanoes can help predict activity at similar, less-monitored sites. Separately, other researchers have focused on single-volcano forecasting with AI. For example, a 2024 study at Mount St. Helens built a neural network using decades of monitoring data and retrospectively “predicted” past eruptions with 95% accuracy at least three days in advance. These advances suggest that, given enough data, machine learning can enhance long-term eruption forecasts by identifying subtle warning signals and assigning eruption probabilities, something traditional methods struggle to quantify.
5. Real-Time Volcano Deformation Monitoring
Ground deformation (inflation or deflation of a volcano’s surface) is a key eruption precursor, and AI is helping to monitor these changes in real time. Satellites (InSAR) and GPS stations produce vast amounts of deformation data, often too much for human analysts to evaluate quickly. Machine learning models can be trained to pick out significant deformation signals from the noise and even detect subtle patterns that might be overlooked. By automating InSAR image analysis and continuously scanning GPS time series, AI systems ensure that signs of magma rising (which causes the ground to swell) are caught early. This is especially crucial during rapidly evolving unrest, when timely interpretation of deformation can inform eruption forecasts and hazard mitigation.
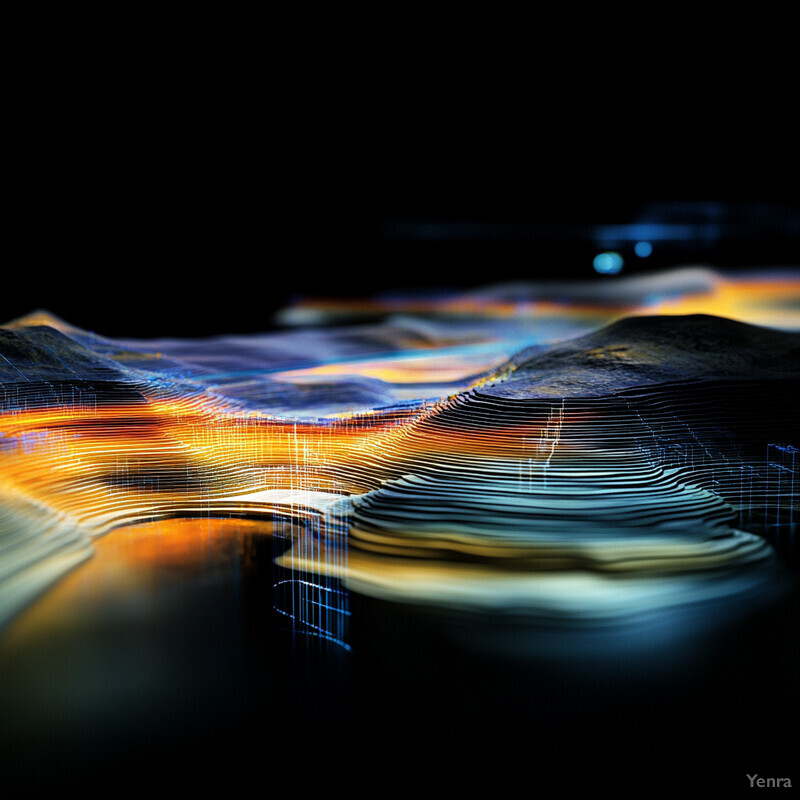
Researchers have begun applying deep learning to continuously analyze ground deformation data. A 2025 review notes that convolutional neural networks have been used to detect and locate deformation “fringes” in satellite radar interferograms, automating what was previously a labor-intensive visual process. These AI models can filter out atmospheric noise and isolate genuine uplift or subsidence signals, enabling the study of small (centimeter-scale) deformation that classical methods might miss. One case study demonstrated that a trained neural network could scan years of InSAR images from multiple volcanoes and successfully flag those containing eruption-related deformation with high confidence. Moreover, by coupling automated data processing with cloud computing, such systems can deliver deformation updates in near-real time. Early experiments indicate that this approach would greatly reduce the time between data acquisition and alert dissemination during volcanic crises, when rapid assessment of swelling or deflating volcano surfaces is essential.
6. Early Warning Signal Classification
Volcanic early warning involves recognizing certain “alarm” signals in the continuous noise of monitoring data. Machine learning is enhancing the classification of these critical signals – such as distinguishing volcano-tectonic earthquakes, long-period (LP) events, and harmonic tremor – which all have different implications for eruptive activity. By training on labeled examples, AI algorithms learn to rapidly classify incoming signals and determine if they match known eruption precursors. This reduces delays in interpretation and ensures that subtle warning signs (like a change in earthquake type or frequency) are promptly recognized. In practice, better signal classification means volcano observatories can issue alerts with greater confidence when truly threatening patterns emerge.
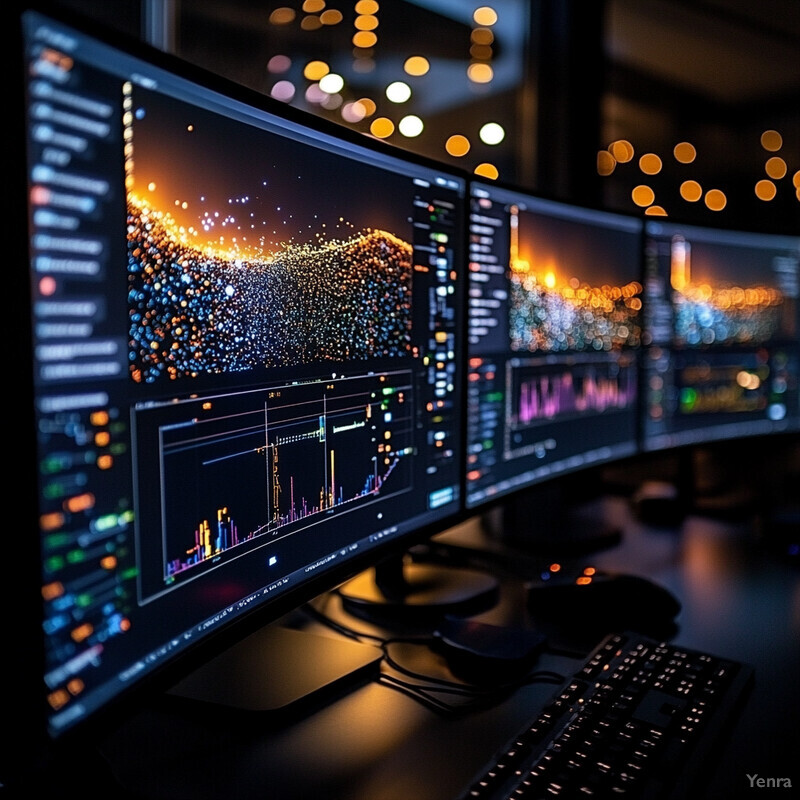
An illustrative example comes from an undersea volcano where unusual seismic signals were identified before an eruption. Using an unsupervised learning approach, researchers detected a distinct class of “mixed-frequency” earthquakes that surged about 15 hours before Axial Seamount’s 2015 eruption. These signals – not easily noticed by standard analysis – were automatically clustered by a machine learning algorithm as an anomaly, effectively classifying them as a new early warning indicator. Indeed, the mixed-frequency quakes spiked and peaked roughly an hour before lava erupted on the seafloor. Similarly, other projects have used neural networks to categorize continuous seismic data streams from active volcanoes. In 2023, Mitchinson et al. applied clustering algorithms to decades of Mt. Ruapehu’s earthquakes, successfully grouping earthquake swarms that preceded eruptive phases versus background seismicity. Such efforts show that AI can not only differentiate event types (e.g., explosion-quake versus regular quake) but also learn which combinations of seismic changes tend to foreshadow an eruption. By automatically classifying these patterns in real time, the system helps human experts focus on genuine early warning signals while reducing false alarms.
7. Probabilistic Hazard Modeling
AI is being used to improve probabilistic hazard models, which estimate the likelihood and impact of various volcanic events (like lava flows, ash fall, pyroclastic flows). Traditional hazard modeling often involves running many simulations with different assumptions to see a range of possible outcomes. Machine learning can accelerate this by serving as a smart surrogate for complex physical models or by directly learning from past eruption data to predict hazard extents. Importantly, AI models can output probabilities – for example, the probability that a given town will experience ash fall over 1 cm thick – incorporating uncertainty in a systematic way. This helps officials in contingency planning, as they can get a sense not just of a single “worst-case” scenario, but a full distribution of possible impacts with associated confidence levels.
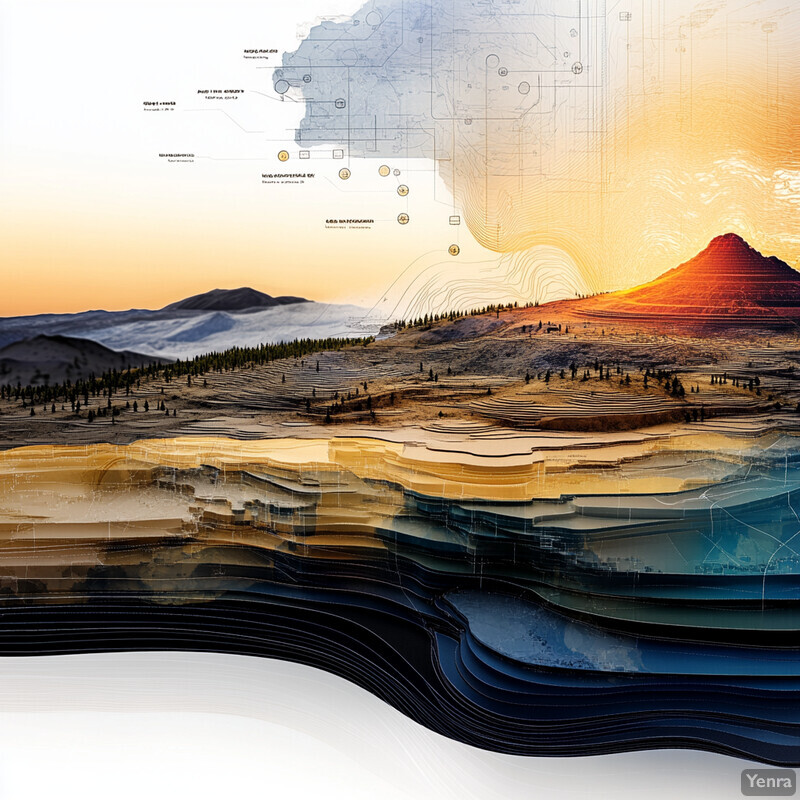
A notable advance in 2024 was the development of a Bayesian network model for multi-hazard eruption forecasting. In New Zealand, researchers built an AI system for Whakaari/White Island that combines seismic tremor data, earthquake counts, and volcanic gas emission rates to calculate eruption probabilities. The model was tested on the last decade of activity and showed skill in retrospectively “predicting” the volcano’s major eruptions weeks to months ahead of time by outputting high probabilities during prolonged unrest. For instance, it consistently raised an eruption probability above 80% in the lead-up to each of the three largest eruptions, effectively providing a quantitative forecast trigger. Importantly, this AI-driven approach could tolerate missing data – even if one data stream (say gas emissions) failed, the model still produced useful probabilistic forecasts by relying on the remaining inputs. This robustness and explicit uncertainty quantification (probabilities rather than binary yes/no) represent a significant improvement in hazard modeling. It allows volcanologists to communicate risks in terms of probabilities and confidence, which is essential for making informed emergency decisions.
8. Remote Sensing Feature Extraction
Volcano monitoring relies heavily on remote sensing – satellites and aerial sensors that detect heat, gas, and deformation from afar. AI is dramatically speeding up the extraction of meaningful features from these remote observations. Instead of an expert laboriously examining each satellite image for signs of activity, machine learning models can be trained to spot those signs automatically. Key features include “hotspots” (areas of elevated thermal infrared radiation indicating lava or hot gas), ash plumes in imagery, gas plume extents, and new ground cracks or landslides visible in optical data. By automating feature extraction, AI not only saves time but can also catch transient or subtle features that a human might miss due to data overload or fatigue. This leads to more timely detection of eruptions and better tracking of ongoing eruptive processes.
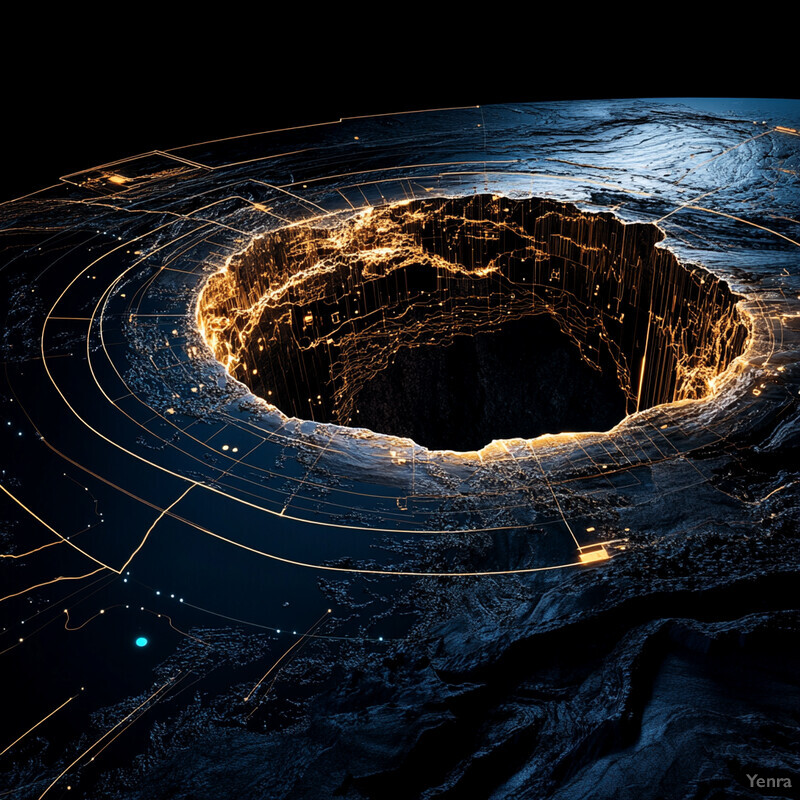
A concrete example is the HotLINK system (Hotspot Learning and Identification Network) deployed for Alaskan volcanoes. Presented in 2024, HotLINK uses a deep neural network to analyze satellite thermal infrared images and automatically identify volcanic thermal hotspots. In tests, it was applied to 22 years of MODIS satellite data (and 10 years of higher-resolution VIIRS data) for eight volcanoes in Alaska. The model successfully detected known eruptions by flagging the appearance of new hotspots and quantifying their radiant power (a measure of eruptive heat output). In one validation using Mount Veniaminof’s eruptive episodes, HotLINK achieved about 98% detection accuracy, demonstrating it can reliably separate true eruption signals from false alarms. The system also generalizes across sensors – a network trained on newer VIIRS data performed well on older MODIS images, indicating robustness. This AI-driven feature extraction now provides Alaska Volcano Observatory with an automated pipeline: as new satellite images come in, hotspots are immediately identified and measured, enabling faster response to eruptive activity.
9. Integrating Meteorological and Atmospheric Models
Volcanic hazards like ashfall and gas dispersion are strongly influenced by weather conditions, so integrating meteorological data with eruption models is crucial. AI has the potential to combine real-time weather forecasts (wind speed, direction, precipitation) with volcanic plume models to improve predictions of where ash and gas will travel. For example, a machine learning model could take outputs from a weather model and an eruption column model to rapidly estimate ash cloud movement without running a full physics-based simulation each time. By accounting for atmospheric dynamics, these integrated AI systems aim to produce more accurate and localized hazard forecasts (such as which communities might receive ash given the current winds). This fusion of volcano and atmosphere modeling is an emerging area that promises more complete situational awareness during eruptions.
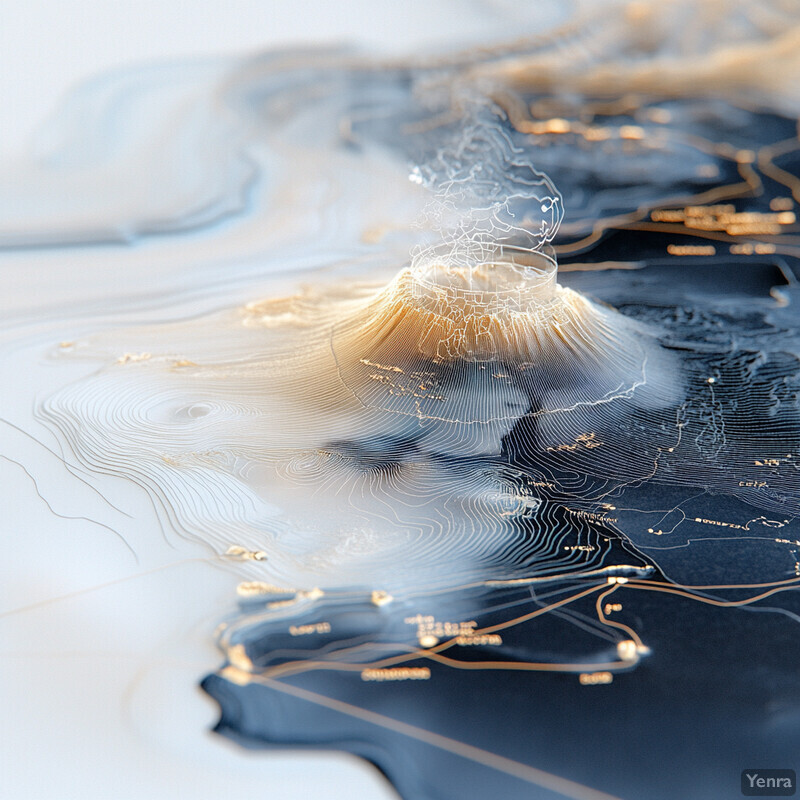
Despite its promise, this integration of meteorology and volcanology via AI has seen little published progress as of 2023–2025. Operational volcano plume forecasts do routinely use meteorological models (for wind and weather input), but those systems are largely physics-based rather than AI-driven. Some experimental studies have hinted at machine learning approaches – for instance, using historical eruption and wind data to train models that predict ash dispersal patterns – but no peer-reviewed study has yet demonstrated a fully AI-based meteorological-volcanic hazard model in practice. Progress is likely to come as data from volcanic eruptions and weather events are combined into training datasets. However, as of now there is no publicly documented deployment of an AI system that dynamically integrates atmospheric model outputs with volcanic activity data for hazard assessment.
10. Geochemical Signature Recognition
Volcanoes often exhibit chemical “fingerprints” in their gas emissions or erupted rocks that can reveal important clues about magma behavior. AI tools are increasingly used to recognize and interpret these geochemical signatures. One application is to link tephra (ash and rock fragments) to their source volcano by analyzing chemical composition – essentially letting the AI classify which volcano a particular sample came from. Another use is detecting changes in gas ratios (like SO₂/CO₂) that might signal magma moving upward. By training on large geochemical datasets, machine learning algorithms can find subtle patterns or combinations (for example, trace element ratios in ash) that human analysts might overlook. This helps volcanologists quickly process lab analyses or real-time sensor data and flag notable changes that could precede eruptive activity.
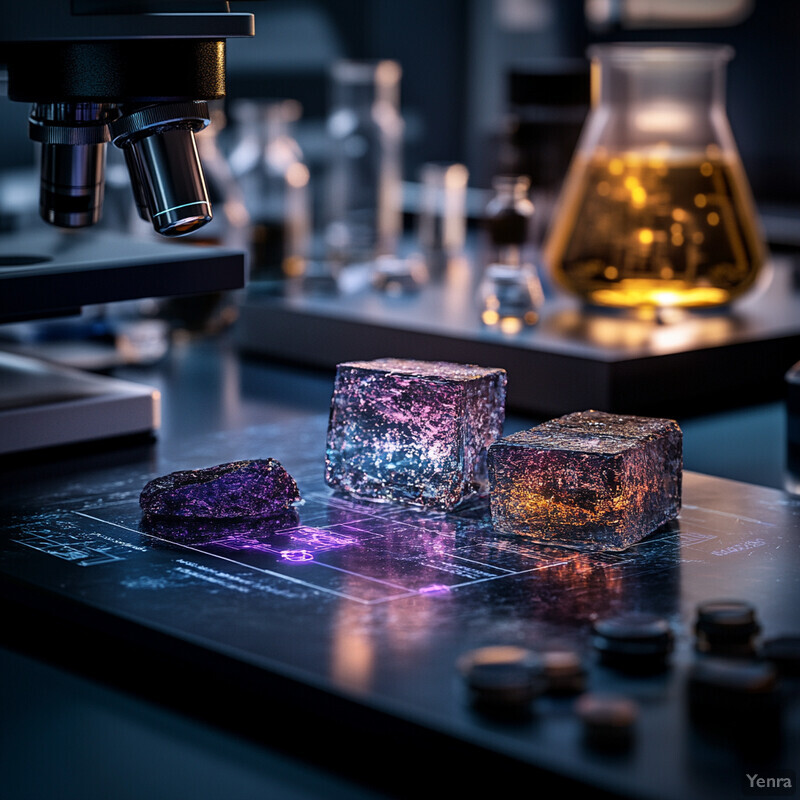
In late 2023, researchers demonstrated an ML-based method to identify the source volcano of far-traveled ash deposits using trace element chemistry. Lubbers et al. (2023) compiled geochemical analyses of ash layers found in seafloor drill cores in the Gulf of Alaska. Each ash layer’s major and trace element profile was input to a machine learning classifier that had been trained on known eruptions from various Alaskan volcanoes. The result was a probabilistic classification of which volcano likely produced each ash layer. Impressively, the model traced the majority of ash layers (spanning 750,000 years of eruptions) back to three key volcanoes – Redoubt, Katmai, and Emmons Lake – with a high degree of confidence. This confirms those volcanoes as the predominant sources of big explosive eruptions in that region, as the model’s predictions aligned with geologic evidence. Beyond ash tracking, AI is also being applied to real-time gas monitoring. For example, pilot studies have used pattern recognition on multi-gas sensor data to detect when volcanic CO₂ and SO₂ levels deviate from baseline, which can indicate magma degassing changes. These successes in geochemical pattern recognition illustrate AI’s value in rapidly analyzing complex chemical data to support eruption forecasting and hazard assessment.
11. Continuous Learning and Model Updating
Volcano monitoring AI systems perform best when they can learn continuously from new data. Continuous learning means that as more eruptions occur or more observations are collected, the models update themselves to improve accuracy. This is important because volcanic behavior can evolve – a volcano might develop new eruption styles or precursor patterns over time. An AI model trained on last decade’s data alone might miss these evolving signals if not updated. Implementing continuous learning could involve techniques like online learning (updating model parameters incrementally with each new data batch) or transfer learning (adapting models from one volcano to another as data becomes available). The goal is an AI system that “gets smarter” with every episode of volcanic unrest, maintaining high performance and adapting to any changes in how a volcano behaves.
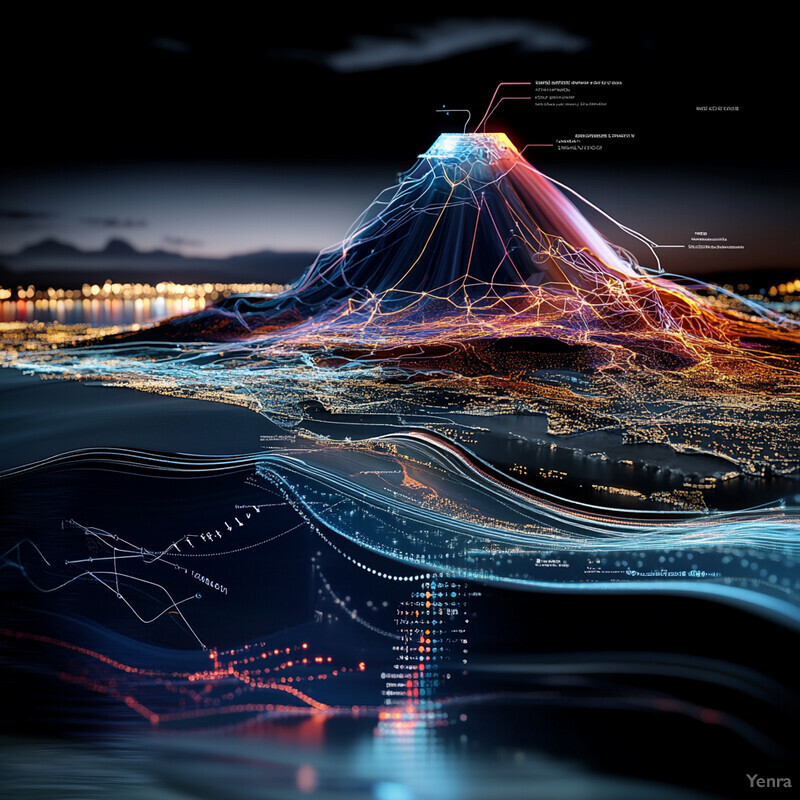
The need for continuous model updates is widely recognized in recent volcanology AI research. Experts emphasize that machine learning models should be retrained or fine-tuned whenever significant new data arrives. For instance, an early 2025 overview by Petrelli et al. noted that many volcanoes lack extensive monitoring records, which limits initial model training, but as more data are gathered (e.g. through initiatives like the National Volcano Early Warning System), AI models can be progressively refined to close those gaps. One challenge is ensuring data quality – continuous learning requires robust handling of noisy or heterogeneous incoming data so that model updates do not degrade performance. Researchers are exploring schemes where an AI model flags novel patterns that it was not originally trained on, prompting human experts to verify and incorporate those patterns into the model’s knowledge base. Although there have not yet been published deployments of fully self-updating volcano AI systems, the trend is moving toward more adaptive frameworks. The expectation is that future monitoring algorithms will operate more like “living” models, steadily improving as they ingest each new earthquake, gas reading, or thermal image from the volcano.
12. Uncertainty Quantification and Error Reduction
Modern AI approaches in volcanology place a strong emphasis on quantifying uncertainty and reducing errors (like false alarms or missed events). Unlike deterministic methods, machine learning models can naturally provide probabilities or confidence intervals – for example, “an 85% chance of eruption in the next month” – which communicate the inherent uncertainty in forecasts. Quantifying uncertainty is critical for decision-makers who need to weigh the risks. Additionally, AI models are being designed to minimize false positives (raising an alert when nothing happens) and false negatives (failing to alert for a real event). Techniques such as including a “noise” class in classification (so the system learns to ignore irrelevant signals) or using ensemble models (to get a consensus and measure of spread in predictions) are improving reliability. The overall goal is to make eruption warnings not only timely but also trustworthy, with known error rates and confidence levels.
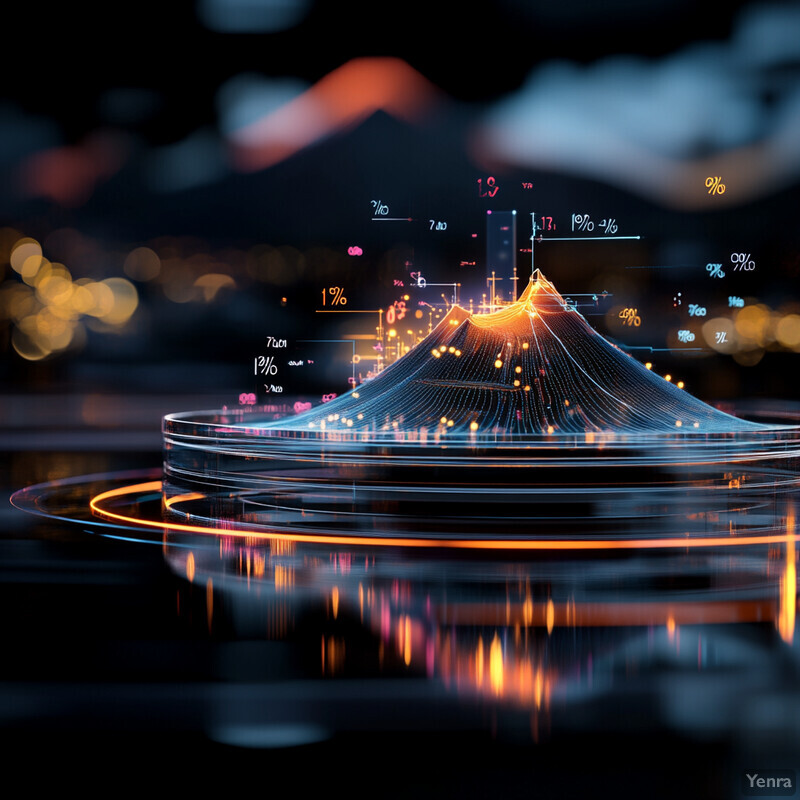
Recent implementations illustrate these principles. The Bayesian network eruption-forecast model for White Island mentioned earlier outputs an explicit probability of eruption, which inherently gives an uncertainty measure. Operators can set a probability threshold (e.g. 80%) that must be exceeded before an alert is issued, thereby controlling the balance between sensitivity and false alarms. In practice, this meant the model provided early warnings with a quantified confidence – eruptions were consistently preceded by probability spikes rather than a binary “yes/no” alarm, allowing officials to see the growing certainty of an event. In terms of error reduction, machine learning developers have taken steps like expanding training datasets to include non-eruptive “noise” periods. For example, Tan et al. (2024) incorporated a class for “noise or normal seismicity” when training their tremor detection network, so the algorithm learned to distinguish genuine volcanic tremor from benign seismic background signals. This significantly reduced false triggers, as the system would ignore minor vibrations that did not fit an eruption precursor profile. These examples show how AI models in volcanology are increasingly being built with transparency (through probabilistic outputs) and robustness (through error-aware training), making their predictions more actionable in the real world.
13. Machine Learning-Driven Acoustic Analysis
Volcanic processes often emit distinctive sounds at low frequencies (infrasound) that can travel long distances. Analyzing these acoustic signals can reveal eruption onsets, explosions, or even internal magma movements. Machine learning is increasingly applied to this acoustic data to automatically detect and interpret events. This is valuable because infrasound monitoring can work in real time and in all weather, supplementing seismic and visual observations. AI models can classify different types of volcanic sounds – for example, differentiating a loud explosion versus continuous jetting versus background noise – and issue alerts accordingly. They can also integrate with seismic data to improve source localization (figuring out exactly which vent an explosion sound came from). The end result is a more robust remote listening system for volcanoes, with AI sifting through the continuous rumble to pick out key acoustic signatures of activity.
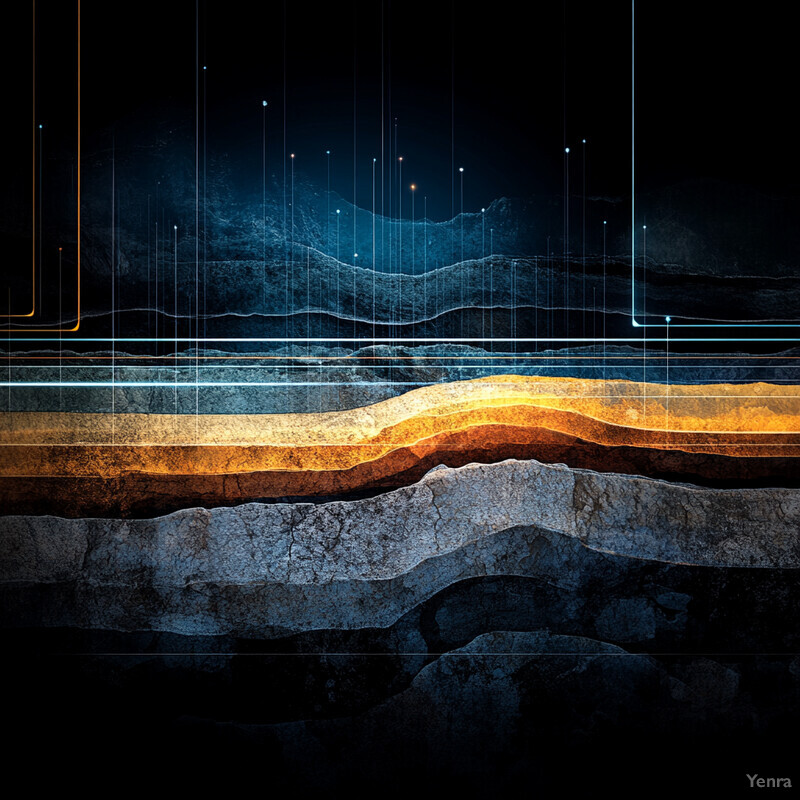
In 2024, a study in Alaska highlighted the power of machine learning for infrasound monitoring. The research team extended a convolutional neural network (originally trained on seismic spectrograms) to also ingest infrasound spectrograms from the eruptive Pavlof Volcano. This “dual-modal” model (seismic + acoustic), nicknamed VOISS-Net, was able to detect eruption-related signals that might have been ambiguous in only one data type. For instance, the network identified certain low-frequency pressure waves as explosion booms and correctly paired them with seismic spikes from the same events, confirming they were volcanic explosions rather than unrelated thunder or sonic booms. By learning the patterns of volcano-acoustic events, the AI achieved near-real-time detection of eruptions: during testing, it recognized an explosive event’s infrasonic boom within seconds and could immediately flag it for observatory staff. Additionally, the model’s false positive rate remained low because it had learned to ignore common noise (like wind or distant human-made sounds) that doesn’t match volcanic signatures. These advancements show that machine learning can serve as an ever-vigilant “ear” on the volcano, greatly enhancing the capability to monitor eruptions via sound.
14. Social Media and Crowdsourced Data Integration
People on the ground often provide the first reports of volcanic events via social media (tweets, Facebook posts, etc.) or crowdsourced platforms. Integrating these unconventional data sources can enhance situational awareness. AI can sift through huge volumes of social media in real time, extracting relevant information about an eruption’s impacts, like ashfall locations or evacuation status, directly from eyewitnesses. This “social sensing” can complement instrument data – for example, confirming an eruption column sighting or detecting distress signals from communities. By using natural language processing and image recognition, machine learning systems can automatically categorize posts (e.g., “seeing ash in town X” or “lava flow reached highway”) and pass this information to responders and scientists. The challenge is filtering out misinformation and noise, which AI can help address by learning trusted sources and keywords associated with genuine events.
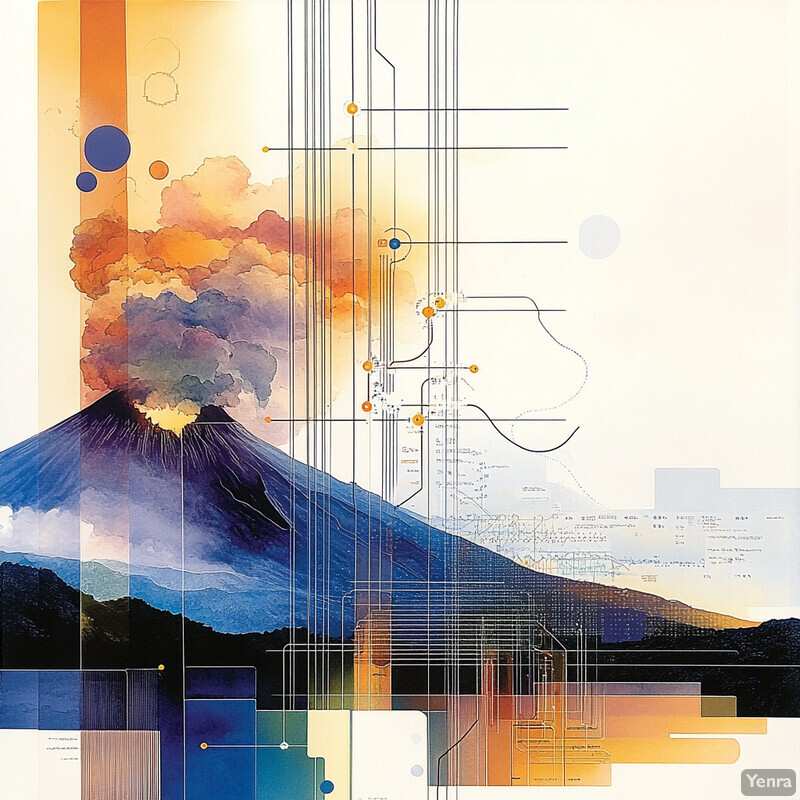
A study of Twitter activity during the December 2021 eruption of Semeru (Indonesia) underscored the value of social media integration. Researchers analyzed the hashtag #Semeru and found a network of users who were key in disseminating eruption early warnings and updates. An automated social network analysis identified eight accounts – including local journalists and the Indonesian meteorology agency (@daryonoBMKG) – that acted as central nodes, relaying critical information to the public. This indicates that by focusing on certain credible accounts, an AI could efficiently gather real-time eruption reports. In another effort, published in 2025, investigators applied “social sensing” techniques to the 2018 Kīlauea eruption. Their machine learning tools mined thousands of tweets and Facebook posts for mentions of ashfall, fissures, and evacuations. The AI successfully tracked the progression of the eruption through social media trends – for instance, spikes in posts about “evacuation” correlated closely with official evacuation orders, and sentiment analysis showed increased fear and urgency as the lava flows expanded. These examples illustrate that crowdsourced data, when intelligently filtered, can provide timely and spatially rich information to emergency managers. Volcano observatories are beginning to experiment with these integrations, using dashboards that display both sensor readings and live social media feeds related to the volcano.
15. Holistic Eruption Lifecycle Understanding
AI is contributing to a more holistic understanding of the entire “lifecycle” of eruptions – from the initial magma intrusion, through eruption onset, to aftermath and rebuilding of a volcano. Traditionally, different phases of volcanic activity were studied in isolation (e.g., geochemists study pre-eruptive magma, geophysicists study eruption dynamics). Machine learning encourages a synthesis because it can handle multifaceted data across all phases. By training on data spanning long periods, AI models can link subtle pre-eruptive changes to eruption intensity, or connect eruption plume data with post-eruption environmental effects. This big-picture perspective helps scientists grasp how various processes interrelate over time. Ultimately, it moves volcanology toward a systems approach – seeing a volcano not just at the moment of eruption, but as a living system with feedback loops and cycles that AI can help decode.
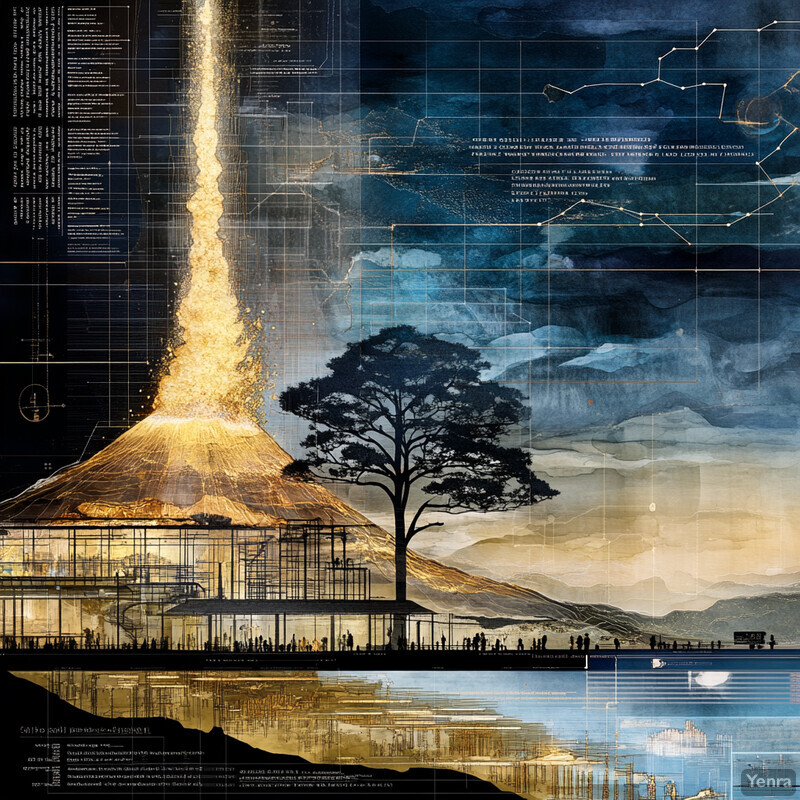
Recent reviews highlight this integrative potential of AI. Petrelli et al. (2025) noted that machine learning is being used from “deciphering the architecture of magma feeding systems and pre-eruptive processes to eruption forecasting”. In other words, the same toolkit can analyze geochemical signals that reveal magma chamber changes, and also improve forecasting models – bridging pre-eruption and eruption timescales. Additionally, AI is applied in post-eruption analysis, such as mapping eruption deposits with remote sensing to learn how the eruption unfolded and to update hazard models for the future. By learning from the complete sequence of events (before, during, after), AI models have started to uncover patterns that span the lifecycle. For instance, a model might recognize that a volcano’s seismic noise months before an eruption correlates with the volume of lava ultimately erupted, giving insights into magmatic buildup processes. These holistic findings, made possible by crunching datasets that no single human could fully absorb, are gradually filling gaps in our knowledge of why eruptions start the way they do and how volcanoes recover. As data volumes grow, the expectation is that AI will continue to illuminate the full timeline of volcanic activity, helping scientists weave formerly separate observations into a unified narrative of a volcano’s behavior.