1. Automated Disease Identification
AI-driven image recognition enables robots to detect signs of mildew, rot, or fungal infections on leaves and grapes earlier and more accurately than human scouts.
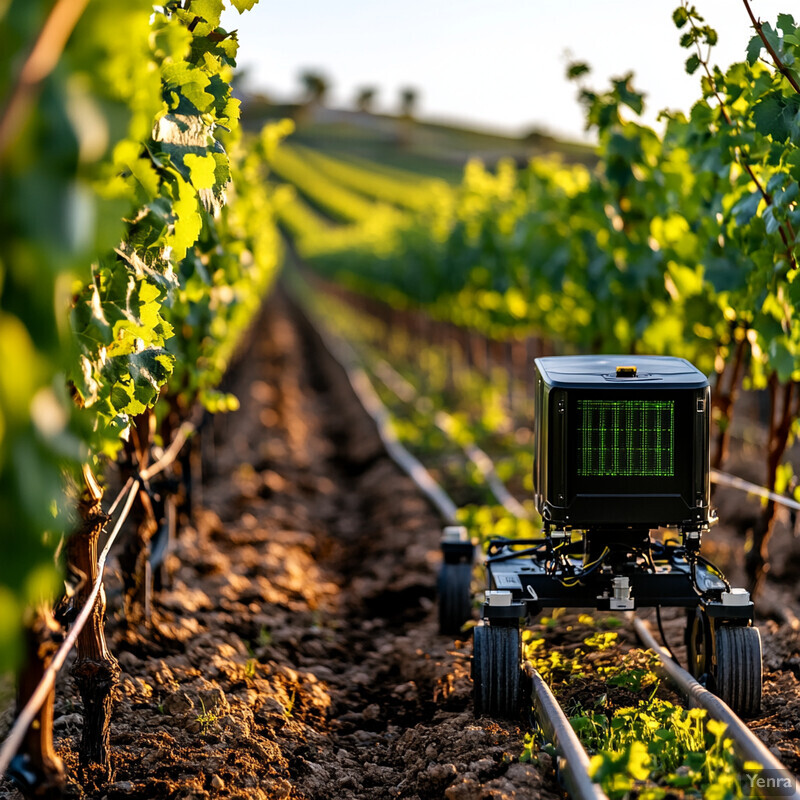
With advanced image recognition algorithms and high-resolution sensors, AI-equipped vineyard robots can closely inspect leaves, stems, and grape clusters to detect early signs of diseases like powdery mildew, downy mildew, or botrytis. By continuously analyzing imagery and comparing it to large, annotated datasets, these robots gain the ability to identify subtle discolorations, unusual leaf shapes, or small spots that might go unnoticed by human eyes. Early disease detection enables more timely interventions, reducing the severity of outbreaks and minimizing the need for broad chemical treatments. As a result, growers can maintain healthier vines and higher-quality grapes, while also decreasing operational costs and environmental impact.
2. Pest Detection and Targeted Intervention
Advanced algorithms allow robots to identify insect infestations at a micro-scale, enabling precision spot treatments rather than broad-spectrum pesticide use.
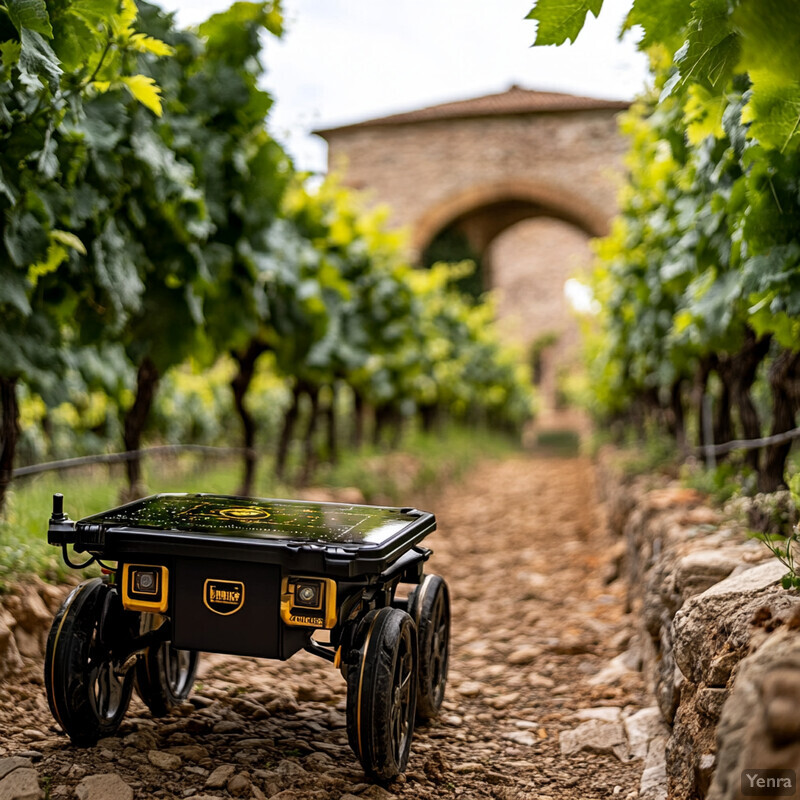
AI-driven detection algorithms allow robots to identify specific insect pests and their larvae at a granular level. By analyzing close-up images and correlating them with known pest signatures, these systems can pinpoint infested areas and apply treatments directly to affected plants, rather than spraying the entire vineyard. This precise targeting reduces the reliance on chemical pesticides, lowers input costs, and preserves beneficial insects and overall vineyard biodiversity. Moreover, detecting early infestations prevents exponential pest population growth, protects yields, and ensures that fruit remains free from damage that could compromise quality and market value.
3. Canopy Density Analysis
Machine learning models interpret multispectral and 3D imagery, helping robots understand canopy density and vine vigor for more informed pruning decisions.
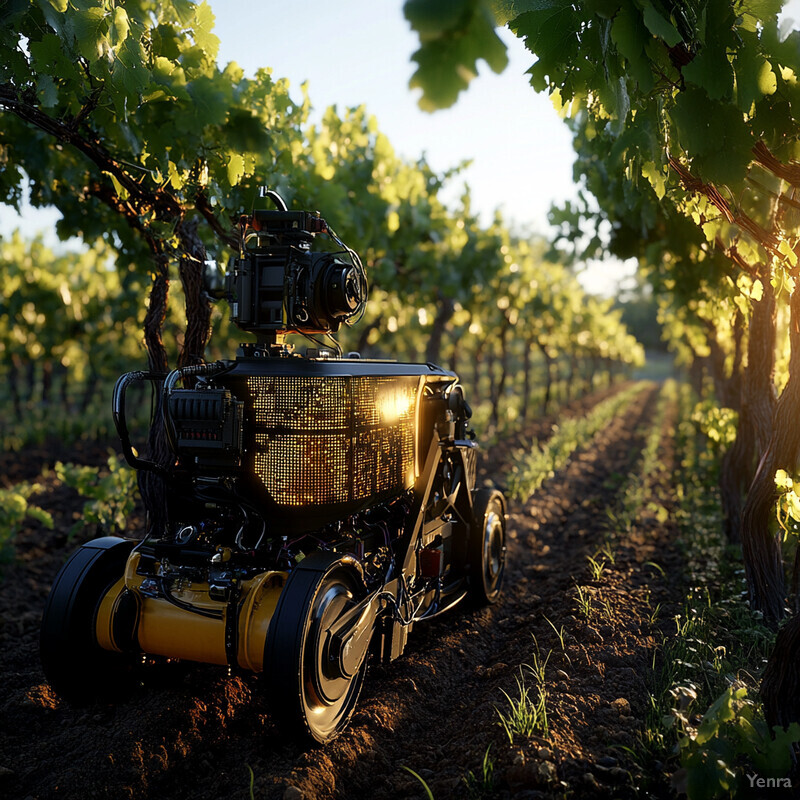
Advanced machine learning models can interpret multispectral images, LiDAR scans, and other sensor data to gauge canopy density and vine vigor across the vineyard. By understanding the spatial distribution of leaves and shoots, vineyard robots can help managers make informed pruning decisions to balance leaf area and fruit load. This leads to better air circulation, improved sunlight penetration, and more uniform grape maturation. Additionally, real-time canopy data can guide actions such as adjusting trellis systems, refining mechanical trimming, and optimizing spray penetration, resulting in healthier vines, improved crop quality, and more efficient use of resources.
4. Yield Estimation and Forecasting
AI-powered predictive models integrate historical data, weather patterns, and plant health indicators to forecast grape yields more reliably and well in advance of harvest time.
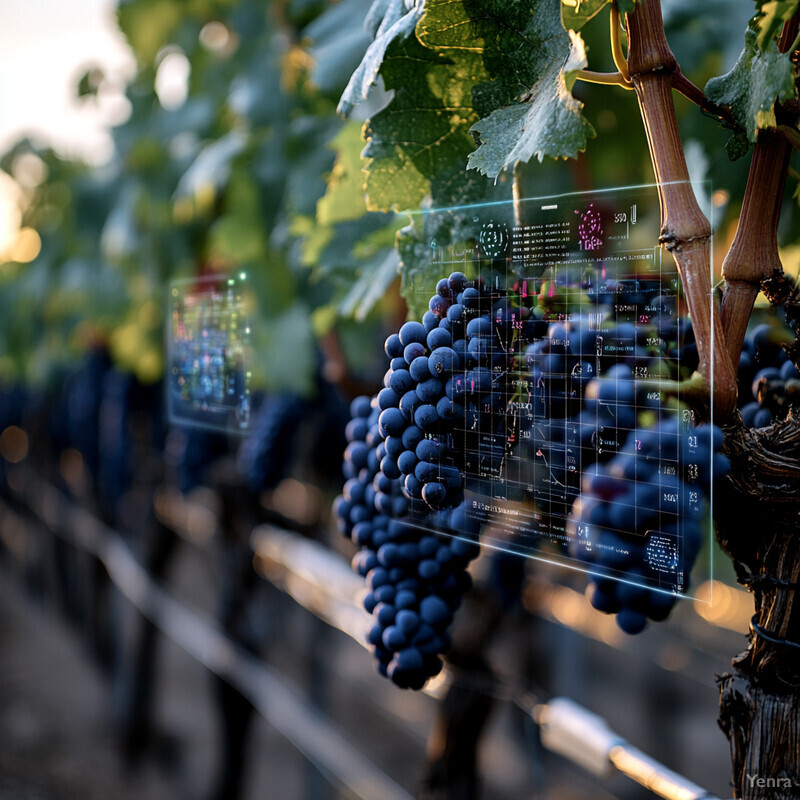
AI-integrated robots combine historical records, real-time sensor measurements, and weather forecasts to model future grape yields. By analyzing factors such as cluster number, berry size, and vine vigor, these predictive models provide accurate yield estimates weeks or even months before harvest. This foresight allows vineyard managers to schedule labor, machinery, and logistics more effectively and to engage in more strategic marketing and pricing decisions. Reliable yield forecasting also helps identify long-term trends, enabling more informed vineyard planning, variety selection, and investment strategies.
5. Optimal Harvest Timing
Robots equipped with AI sensors analyze sugar content, color, and phenolic maturity of grapes, ensuring the crop is harvested at the optimal ripeness for maximum quality.
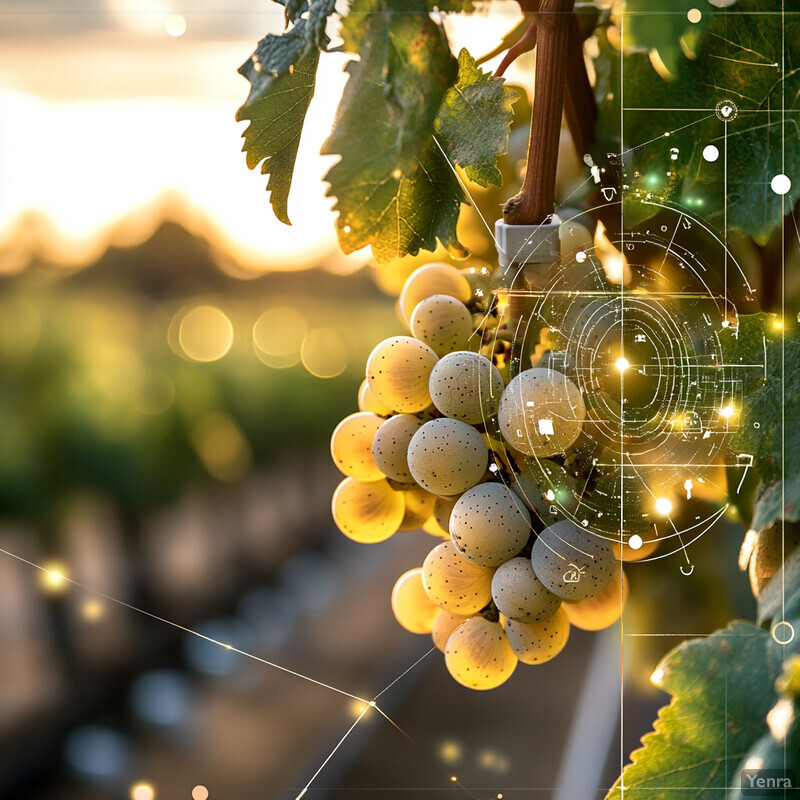
Harvest timing is crucial to achieving peak grape quality, and AI-driven robots are equipped with sensors that measure parameters like sugar levels (Brix), pH, acidity, color intensity, and phenolic content. By analyzing these metrics and comparing them to ideal ripeness profiles, the system can signal the best time to harvest. This ensures that grapes have reached their full flavor, aroma, and structural potential, contributing to the production of premium wines. Furthermore, precise timing reduces waste from overripe or underripe fruit and fosters better consistency across vintages, enhancing the winery’s reputation and brand value.
6. Autonomous Navigation and Obstacle Avoidance
Reinforcement learning and computer vision algorithms guide robots through complex vineyard terrain, allowing safe and efficient operations without human intervention.
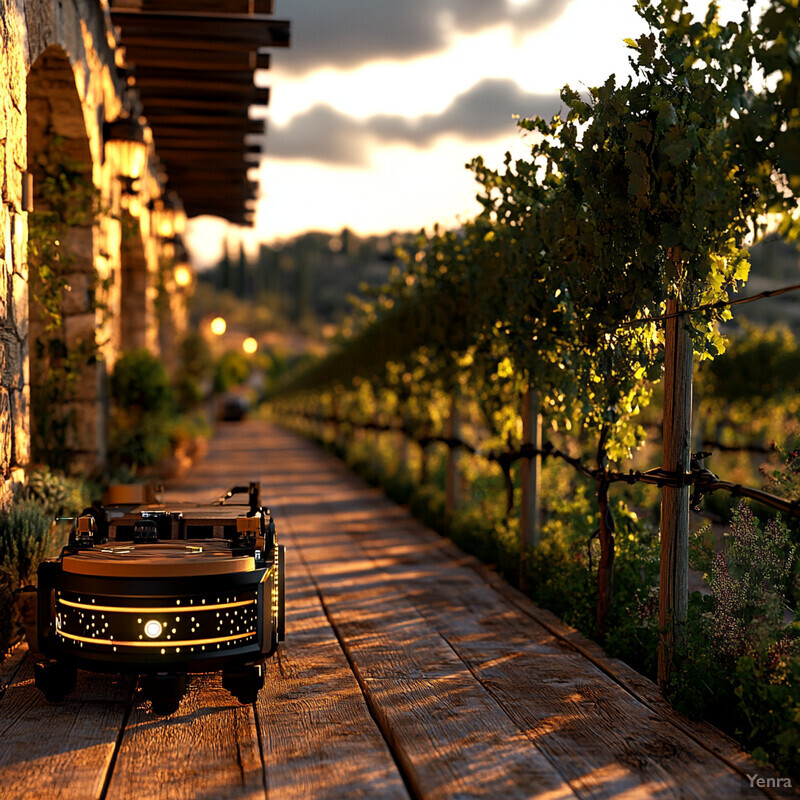
Vineyards are characterized by uneven terrain, tight row spacing, and obstacles such as trellis posts or irrigation lines. AI-driven robots use computer vision and reinforcement learning techniques to navigate these challenging environments safely and efficiently. Sensors and cameras continuously map the surroundings, allowing the robot to plan routes that minimize downtime, avoid collisions, and maintain a consistent operational pace. This ability to operate autonomously frees human workers from repetitive or hazardous tasks and ensures that vineyard operations, such as spraying or scouting, occur reliably, even during labor shortages or difficult working conditions.
7. Precision Irrigation Scheduling
By integrating AI models with soil moisture and evapotranspiration data, vineyard robots can deliver water precisely when and where it’s needed, reducing water waste and improving fruit quality.
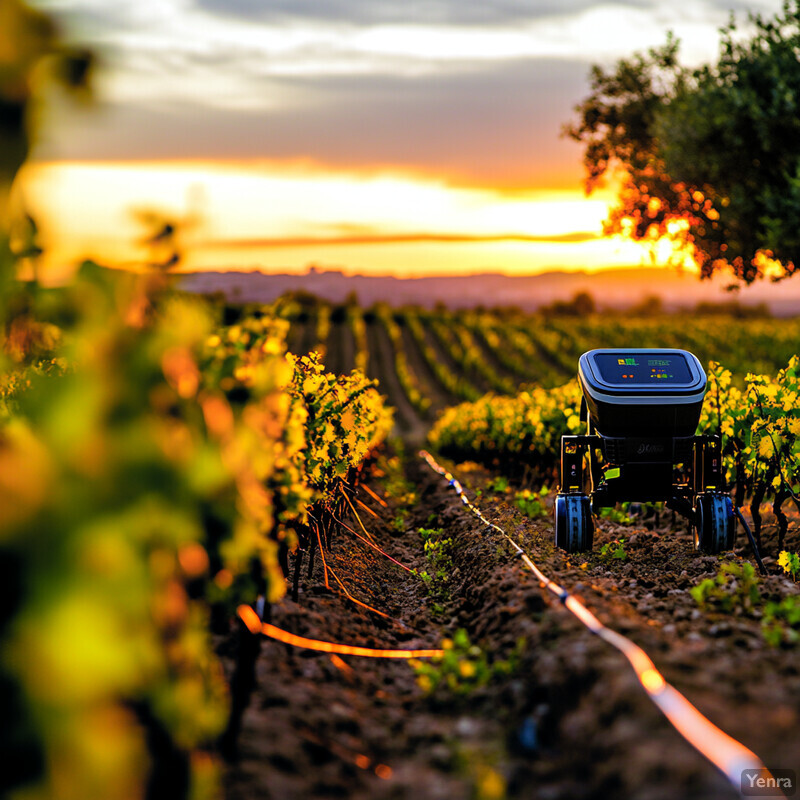
Integrating AI models with data from soil moisture sensors, evapotranspiration rates, and weather forecasts allows robots to determine the exact timing and quantity of water each vine block needs. By watering only when necessary and delivering water directly to the root zone, growers can significantly reduce water waste and energy consumption. This precision approach not only conserves a precious resource in water-scarce regions but also helps maintain optimal vine balance, prevents waterlogging or stress, and enhances grape quality by achieving the desired balance between sugars, acids, and other flavor compounds.
8. Nutrient Management
AI analyzes data from soil sensors, leaf tissue samples, and historical fertilizer applications to inform robots how much and which nutrients to apply, improving vine health and reducing costs.
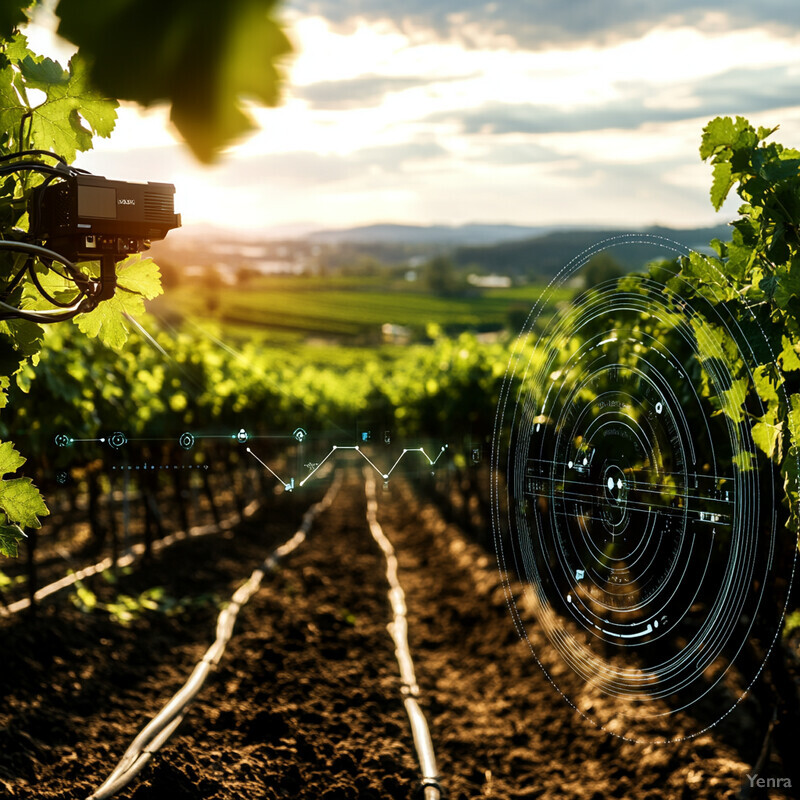
AI-powered analysis synthesizes data from soil tests, leaf tissue analyses, and historical fertilization records to recommend tailored nutrient treatments. Rather than applying a uniform fertilizer regimen across the entire vineyard, robots can deliver nutrients only where deficiencies exist, ensuring that each vine receives the right balance of macro- and micronutrients. This targeted approach enhances vine health, increases resistance to diseases and pests, and improves fruit quality. In the long run, it reduces fertilizer costs, minimizes environmental runoff, and supports sustainable soil management practices.
9. Microclimate Monitoring
AI models synthesize microclimate data (temperature, humidity, wind) to predict disease pressure and stress conditions, allowing robots to adjust interventions in real time.
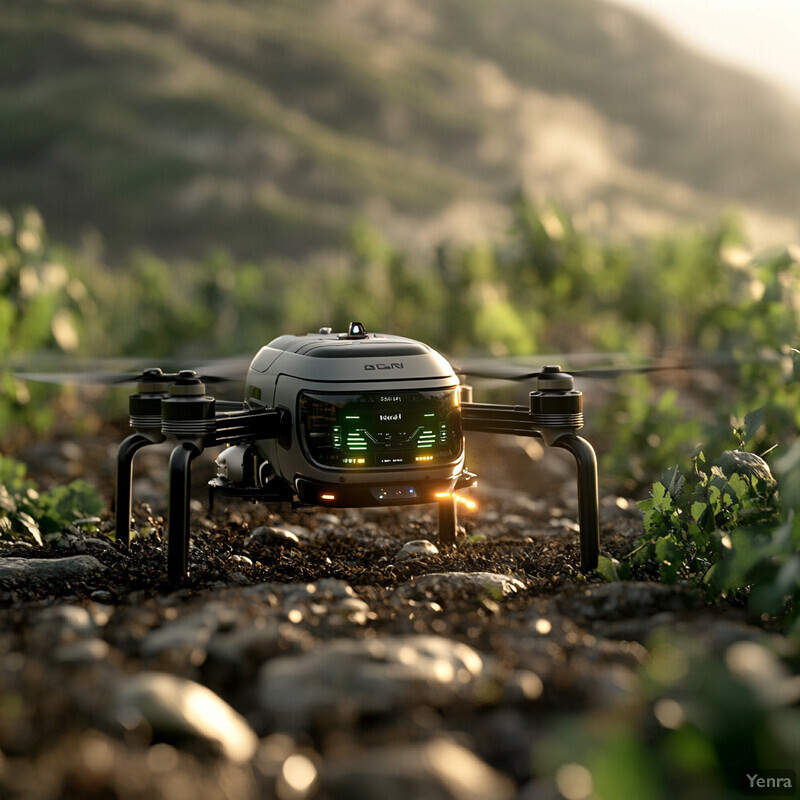
Vineyards often exhibit microclimatic variations due to slope, aspect, elevation, and local weather patterns. AI-enabled robots analyze temperature, humidity, wind speed, and solar radiation measurements to predict how these small-scale climate differences influence vine growth, disease pressure, and fruit maturation. By understanding these localized conditions, vineyard managers can adapt their strategies—whether it’s altering canopy management practices, adjusting irrigation schedules, or modifying pest control measures—to optimize growing conditions in each distinct section of the vineyard. This leads to more uniform crop development, higher quality fruit, and better resource allocation.
10. Enhanced Weed Control
Image classification helps robots differentiate between vines, beneficial ground cover, and weeds, enabling selective mechanical weeding or targeted herbicide use, reducing labor and chemical inputs.
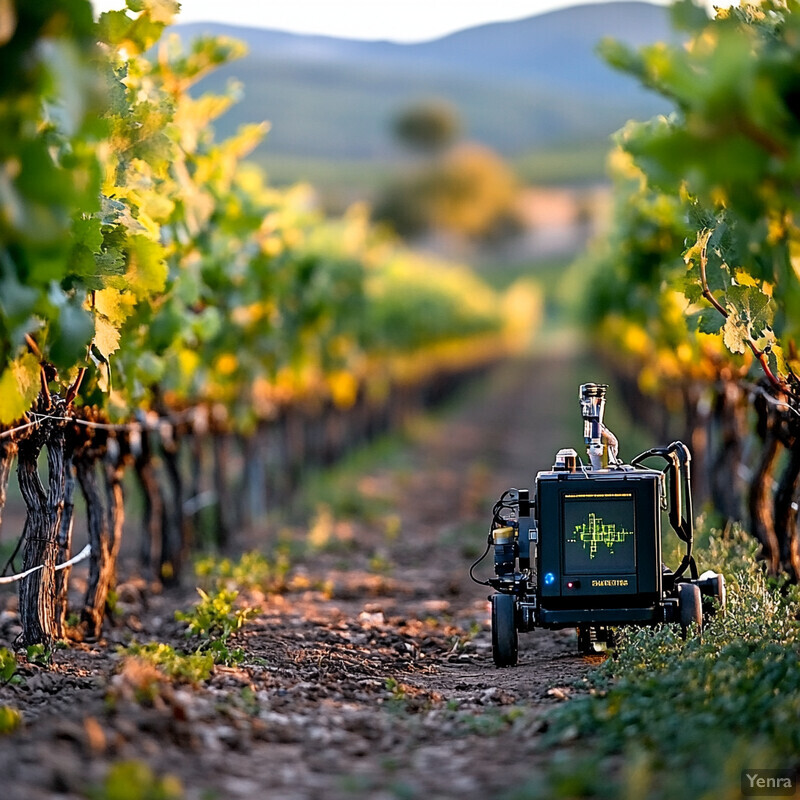
Distinguishing between vine shoots, cover crops, beneficial flora, and harmful weeds is a complex task. AI-based image classification helps robots recognize weed species and their growth stages, enabling them to remove or treat only the unwanted plants. Through mechanical weeding tools or precise herbicide application, this approach reduces chemical usage, lowers labor costs associated with manual weeding, and limits soil disturbance. Effective weed control fosters a healthier vine environment by minimizing competition for water and nutrients, while maintaining beneficial ground covers that support soil health and prevent erosion.
11. Continuous Condition Tracking
AI-driven analytics help robots monitor vine health throughout the season, detecting subtle changes in leaf coloration, texture, and growth patterns before they become serious issues.
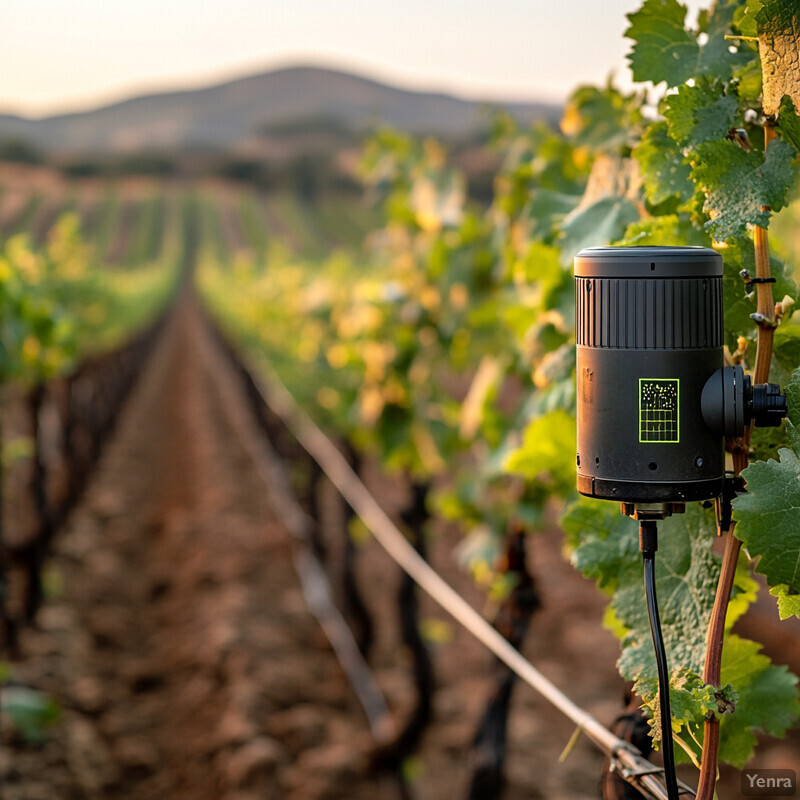
By capturing regular, high-resolution imagery and sensor data, AI models enable robots to continuously monitor vine health indicators—leaf coloration, fruit set, shoot length, and trunk diameter—throughout the growing season. Subtle changes can be detected before they escalate into significant issues. Early intervention might involve adjusting irrigation, adding nutrients, or applying fungicides at precisely the right moment, thereby preventing yield losses or quality downgrades. Over multiple seasons, this continuous tracking helps identify recurring patterns, refine management practices, and build a comprehensive picture of vineyard performance.
12. Thermal Imaging Integration
By interpreting thermal sensor data, AI helps robots understand vine transpiration rates and heat stress, informing interventions like targeted irrigation or canopy adjustments.
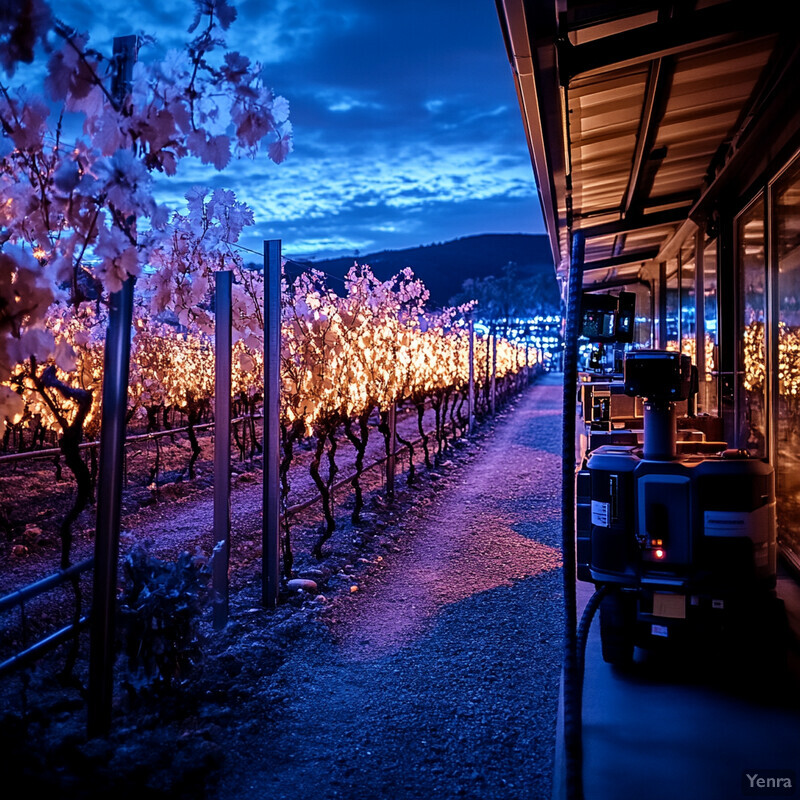
Thermal imaging cameras detect vine stress, irrigation needs, and disease outbreaks by measuring temperature differences and leaf transpiration rates. AI-powered analysis of these thermal images helps interpret subtle variations, guiding targeted responses. For example, if certain vines exhibit higher canopy temperatures, they may require additional watering or shading. Similarly, abnormal thermal patterns might indicate pest infestations or disease onset. Integrating thermal imaging into regular scouting routines leads to precise interventions, better resource efficiency, and healthier, more resilient vines.
13. Early-Season Shoot Thinning Guidance
Robots leverage AI models to determine the optimal number of shoots per vine, instructing mechanical thinning for balanced yields and higher-quality grapes.
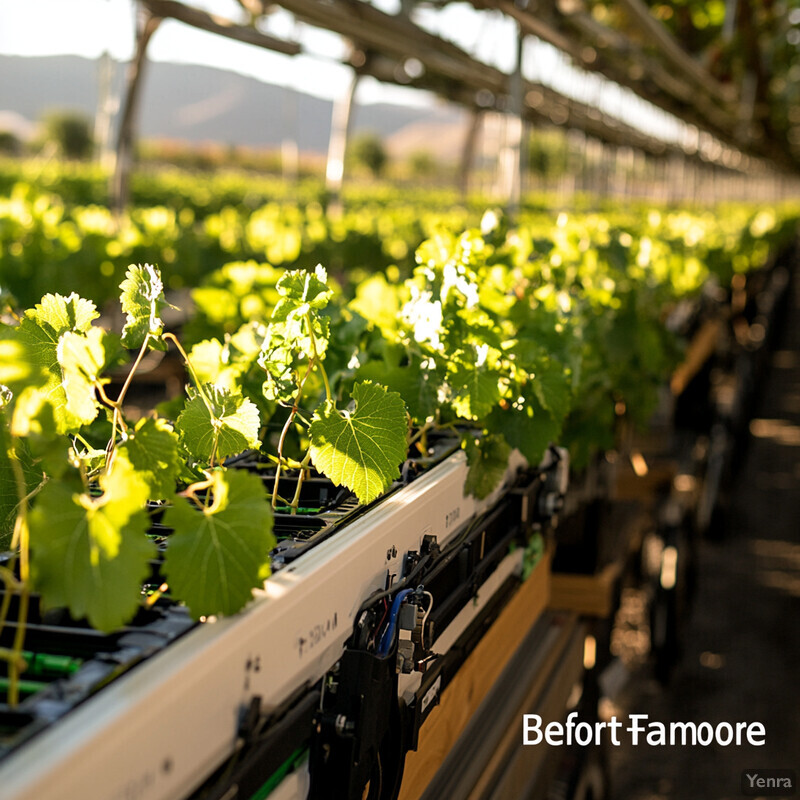
The balance between leaf growth and fruit production is critical for achieving consistent yields and high grape quality. AI models that analyze vine structure, node counts, and shoot vigor can help robots determine which shoots to remove early in the season. By directing mechanical thinning, the robot ensures that each vine’s energy is allocated optimally between foliage and fruit. This results in more uniform cluster development, improved sunlight exposure, and enhanced airflow—key factors in preventing disease and improving wine characteristics.
14. Ground-Level Phenotyping
AI lets robots capture and interpret high-resolution phenotypic data—such as trunk girth, internode length, and leaf size—to inform breeding, selection, and vine management strategies.
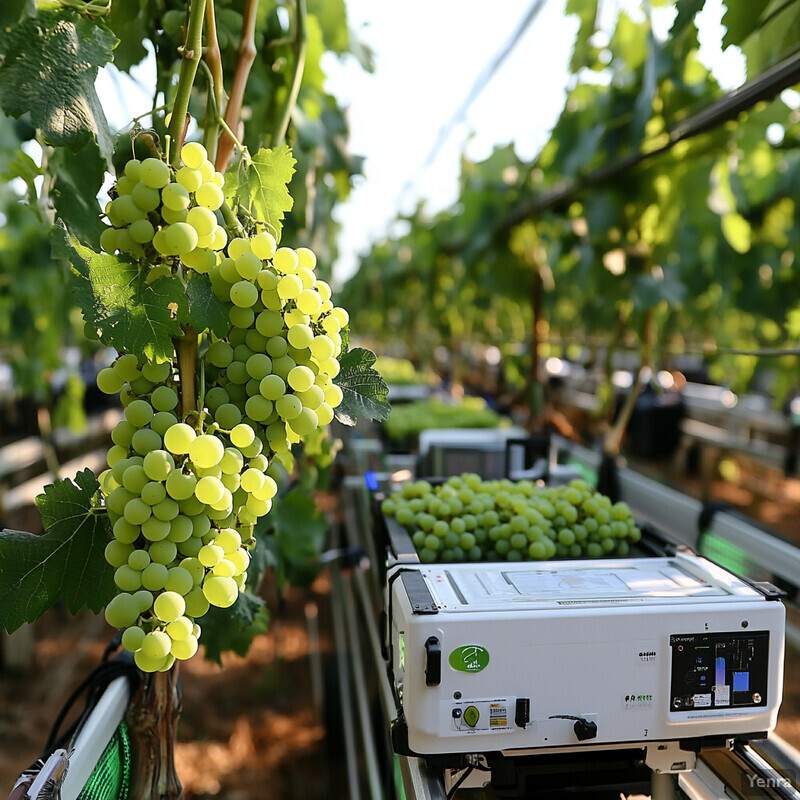
Vine breeders and researchers benefit from phenotypic data on a host of vine traits, including trunk girth, internode length, leaf size, and berry diameter. AI-powered robots can capture and interpret this data efficiently, providing insights into vine genetics and management outcomes. By understanding how different vine varieties or clones respond to specific vineyard conditions, growers can make better planting decisions, optimize rootstock selection, and refine cultivation strategies. Such data-driven approaches lead to the development of hardier, more productive vines and facilitate continuous improvement in vineyard management practices.
15. Vineyard Mapping and Zoning
Clustering algorithms help partition vineyards into management zones based on plant health, soil quality, and growth patterns, guiding more targeted interventions.
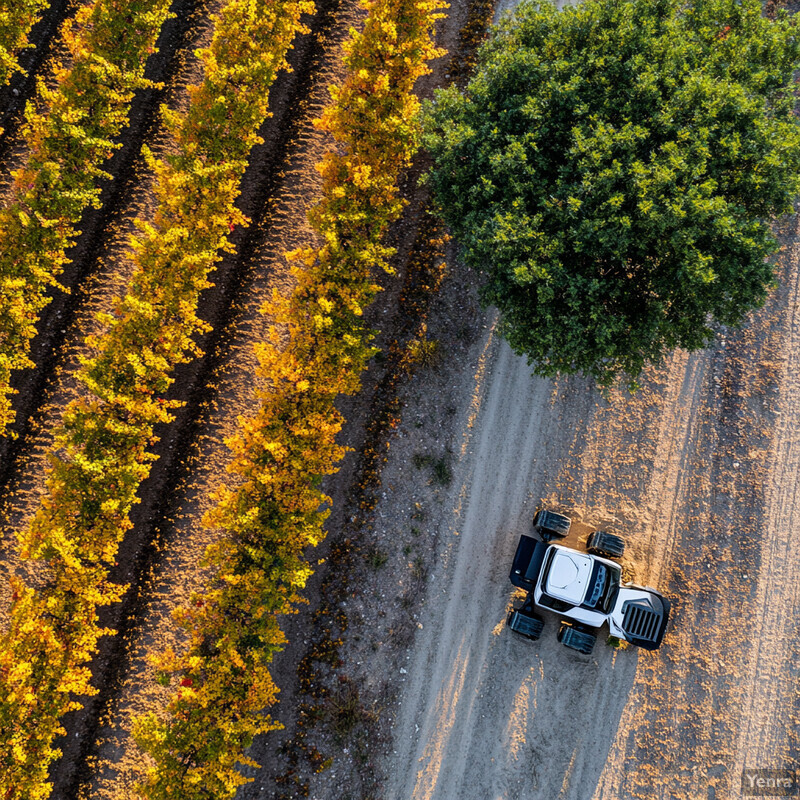
AI-based clustering algorithms process heterogeneous data—soil composition, plant vigor indices, topography, and yield history—to divide the vineyard into distinct management zones. These zones facilitate targeted interventions, ensuring that resources are allocated precisely where they will have the greatest impact. Zone-specific treatments might include tailored irrigation, fertilizer application, or pest management strategies. Over time, this zoning approach improves vineyard uniformity, quality consistency, and cost-effectiveness, while also reducing environmental impacts through more judicious resource use.
16. Historical Data Integration
By learning from past vineyard data, AI allows robots to improve strategies over time, refining disease forecasting models and resource allocation plans for future seasons.
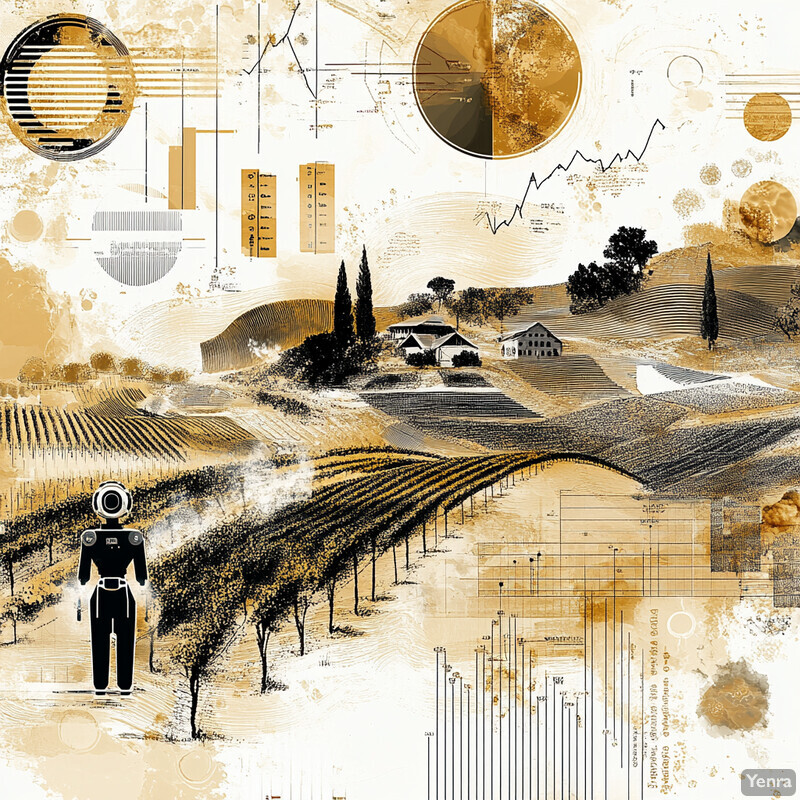
By aggregating and analyzing years of past vineyard records—yield counts, weather data, disease outbreaks, input applications—AI-driven robots learn from historical patterns to refine their current models and recommendations. This long-term perspective allows for continuous improvement of disease prediction models, yield forecasts, and resource allocation plans. As the system learns from past successes and failures, vineyard operations become more resilient to future challenges, ultimately creating more sustainable, profitable, and adaptable viticultural enterprises.
17. Real-Time Alerts and Recommendations
AI-driven analysis provides immediate recommendations on interventions—such as adjusting nutrient inputs or modifying canopy management—directly to farm managers.
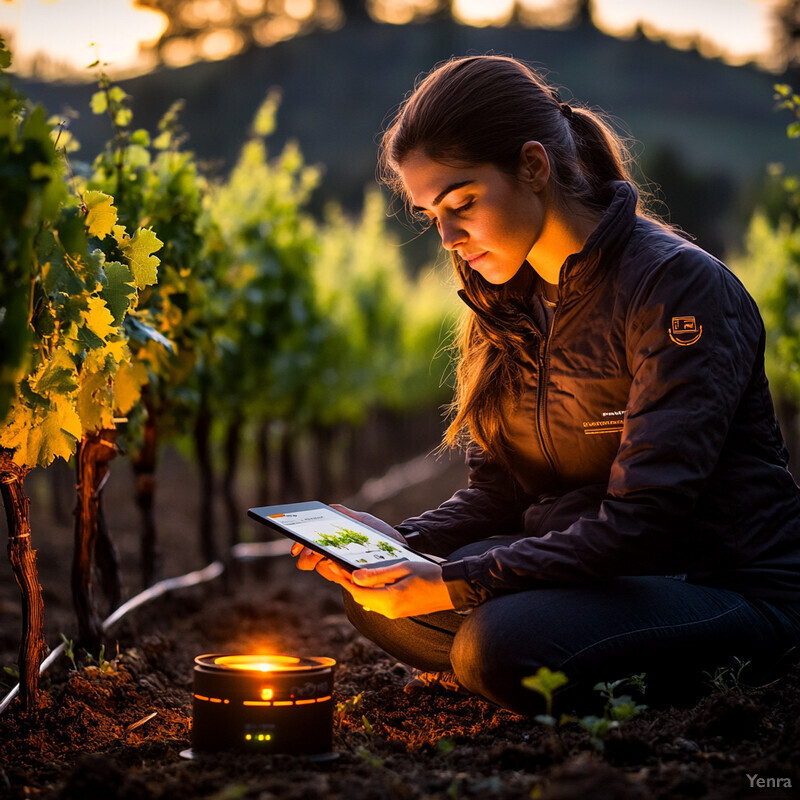
AI-based vineyard robots can deliver immediate alerts to vineyard managers through mobile devices or cloud-based dashboards. When sensors detect unusual conditions—such as a sudden spike in humidity that could trigger fungal growth—the system recommends timely interventions. These instant, data-driven suggestions allow managers to address problems proactively, rather than reacting after damage has occurred. Real-time guidance streamlines decision-making, optimizes labor usage, improves crop outcomes, and increases overall profitability.
18. Autonomous Route Planning
AI optimizes robot travel routes to reduce redundant passes and minimize fuel or battery use, increasing operational efficiency and lowering costs.
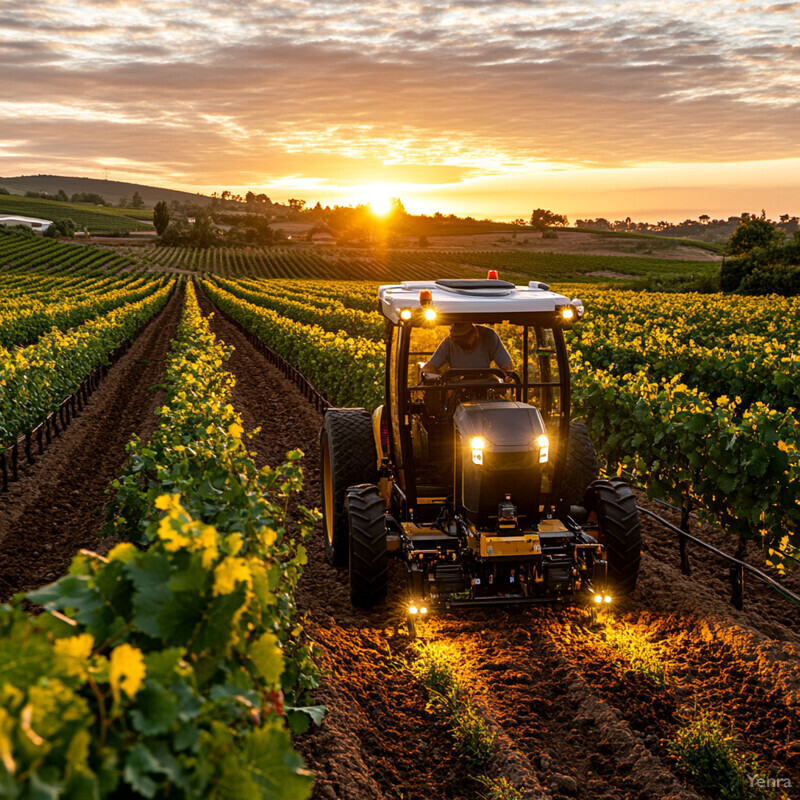
Efficient vineyard management often depends on covering large areas quickly and systematically. AI-driven route planning algorithms calculate the most effective paths for robots to scout, spray, or harvest with minimal overlap and travel time. This reduces wear on equipment, saves fuel or battery energy, and ensures that daily tasks are completed more swiftly. Over multiple operational cycles, the resulting efficiency gains lower costs, enhance productivity, and support more scalable vineyard operations.
19. Quality Sorting and Selection
Robots can use AI-driven optical sensors to grade grapes in-field, separating high-quality fruit from lower-grade harvest, ensuring more consistent product standards.
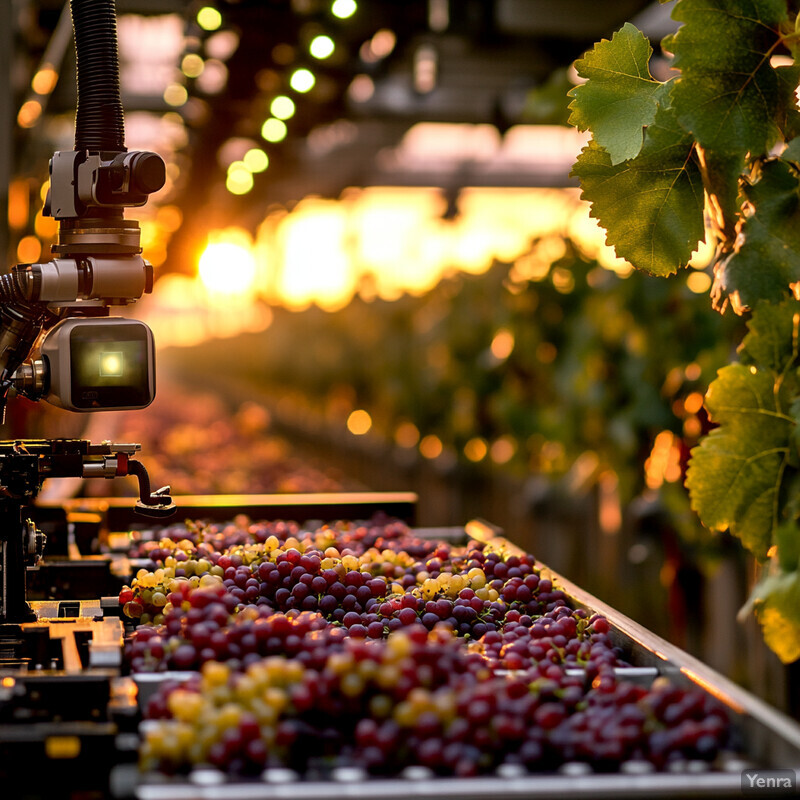
Implementing AI-based optical sensors in the vineyard enables robots to evaluate grape quality right on the vine. By analyzing color, berry size, and blemishes, the system can identify which clusters meet premium standards and which do not. This early sorting can influence harvesting decisions, channeling top-grade grapes into premium wine production and directing lower-quality fruit for alternate uses. Such precision in quality control enhances brand reputation, improves consumer satisfaction, and enables more strategic market segmentation.
20. Long-Term Sustainability Metrics
Over multiple seasons, AI analytics help vineyard managers understand the environmental and economic impacts of their decisions, guiding strategies that lower inputs, reduce environmental harm, and improve vineyard longevity.
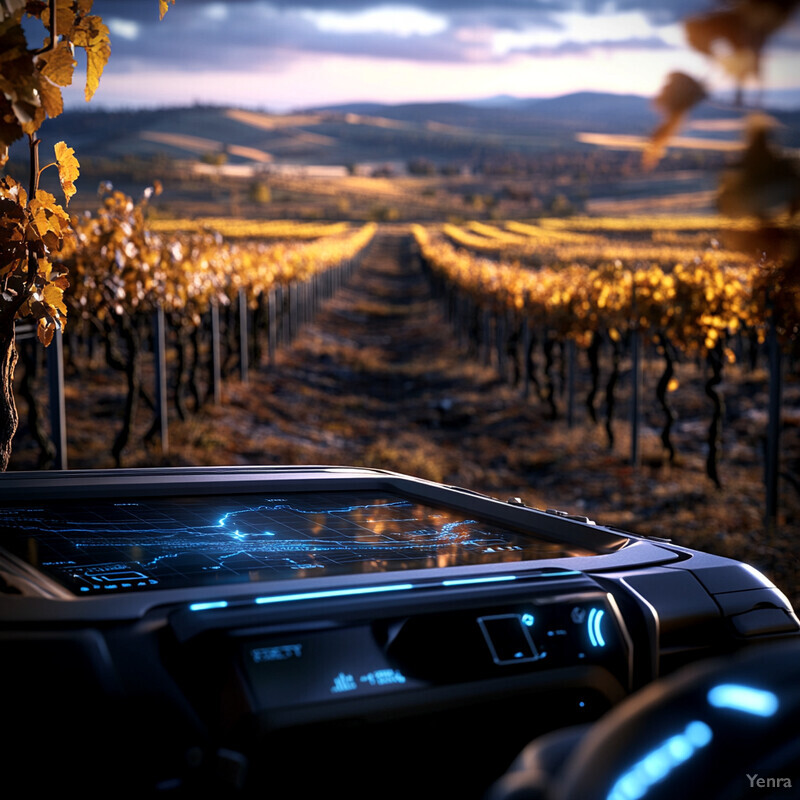
Beyond short-term profitability, vineyards must consider the long-term impact of their practices on the environment, soil health, and resource availability. AI-based analytics track inputs (water, fertilizers, pesticides), outputs (yields, wine quality), and environmental parameters over time to evaluate the sustainability of vineyard operations. By revealing the correlations between practices and long-term outcomes, these metrics guide vineyard managers in making more responsible decisions. Reducing inputs, maintaining soil health, and improving ecosystem services all contribute to the vineyard’s ability to thrive for generations and meet growing consumer demand for sustainably produced wines.