1. Resource Assessment and Prediction
Advanced machine learning models process historical tidal data, meteorological information, and oceanographic parameters to accurately forecast long-term tidal flow profiles, enabling better planning for device placement and energy yield estimation.
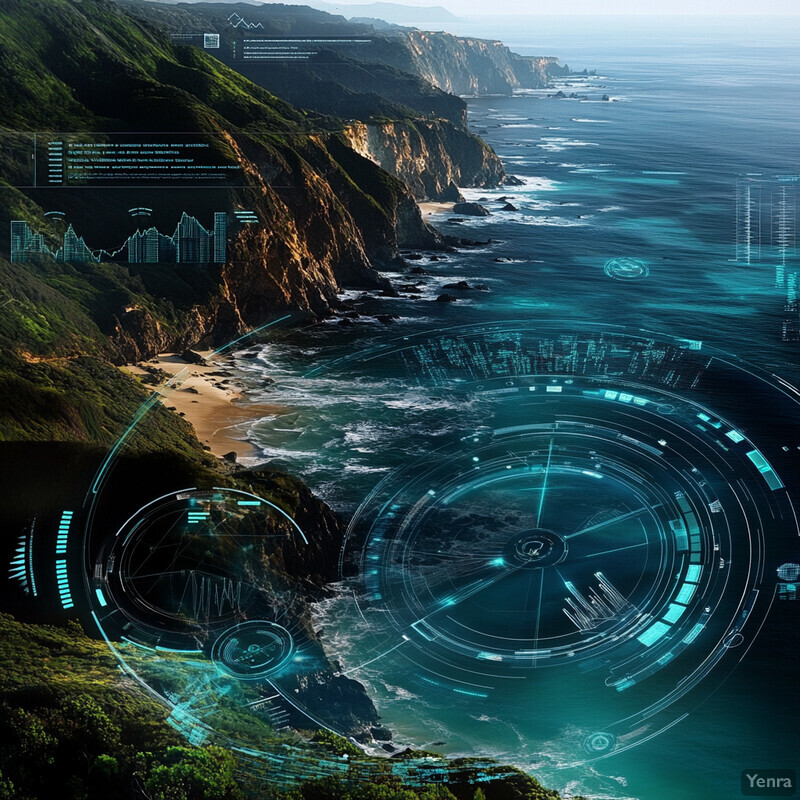
AI-driven resource assessment harnesses extensive datasets that include historical tidal measurements, satellite imagery, and meteorological and oceanographic parameters. Machine learning models, such as deep neural networks and Gaussian process regressors, predict the intensity, timing, and variability of tidal flows over long horizons. These predictions inform feasibility studies, guiding decisions about where and when to deploy tidal turbines. By processing large, complex datasets, AI surpasses traditional analytical approaches, improving the accuracy of tidal current estimation and enabling operators and investors to make data-informed plans. This foresight ensures more stable and predictable energy generation, reducing uncertainty and financial risk in large-scale tidal energy projects.
2. Site Selection Optimization
AI-driven geospatial analysis evaluates numerous environmental, geological, and infrastructure constraints simultaneously, identifying optimal tidal turbine sites that balance high energy density with minimal ecological impact and logistical challenges.
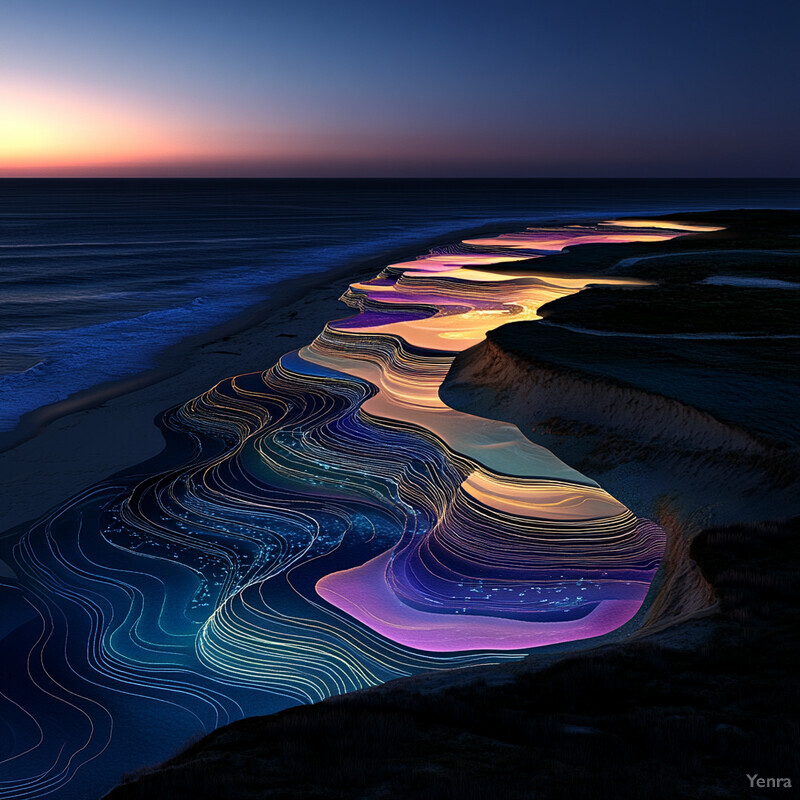
Optimal site selection for tidal energy devices involves evaluating not only where tides are strongest but also where environmental, geological, and logistical considerations align. AI tools integrate factors such as seabed topography, sediment composition, proximity to shore-based infrastructure, marine traffic routes, and ecological habitats. Advanced spatial optimization algorithms, like genetic algorithms and Bayesian optimization, consider multiple constraints simultaneously to identify sites that maximize power output while minimizing environmental disturbance and infrastructural costs. The result is a more holistic approach to site planning that reduces the environmental footprint, enhances the efficiency of operations, and supports the sustainable growth of tidal energy harvesting.
3. Real-Time Power Output Optimization
Reinforcement learning algorithms adjust turbine pitch, yaw, and blade settings on-the-fly to maximize efficiency in response to fluctuating tidal speeds and directions, improving energy capture under dynamic conditions.
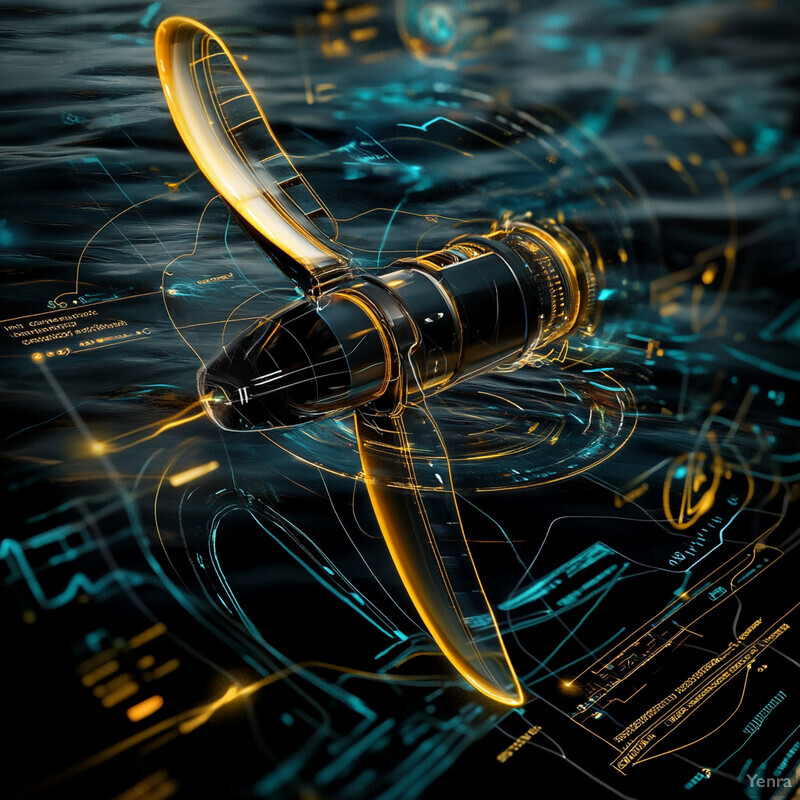
Due to the dynamic nature of tidal currents, maintaining peak turbine efficiency can be challenging. Reinforcement learning and adaptive control strategies continuously adjust turbine parameters—such as blade pitch, yaw angle, and rotor speed—in response to real-time flow changes. By learning from live sensor data, these algorithms fine-tune operational settings to increase power extraction while protecting the equipment from stress and fatigue. Over time, the control system 'learns' the optimal responses to specific flow patterns, ensuring the turbine operates at its highest possible efficiency, even as conditions vary within a single tidal cycle.
4. Predictive Maintenance Scheduling
Predictive analytics, using sensor data (e.g., vibration, temperature, and pressure), anticipate equipment failures before they occur, optimizing maintenance windows and minimizing downtime, ultimately increasing system reliability and uptime.
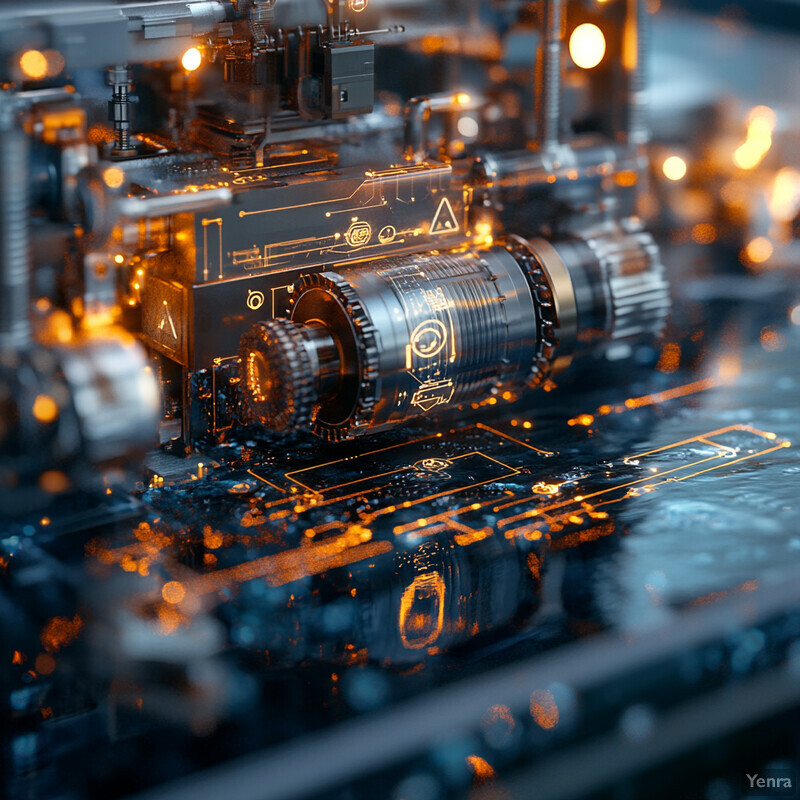
Tidal turbines operate in harsh marine environments, making timely maintenance critical to preventing costly breakdowns. Predictive maintenance powered by AI involves monitoring component health through IoT sensors that measure vibration, temperature, pressure, and other indicators. Machine learning models trained on historical maintenance records and sensor data can predict when parts are likely to fail, allowing maintenance teams to intervene proactively. This approach minimizes unplanned downtime, reduces operational costs, and extends equipment life. By shifting from reactive to predictive strategies, operators ensure more reliable energy production and lower total cost of ownership.
5. Structural Health Monitoring (SHM)
AI models analyze data from structural health monitoring systems—such as strain gauges and acoustic sensors—to detect early signs of fatigue and corrosion, informing proactive repairs and prolonging device lifespan.
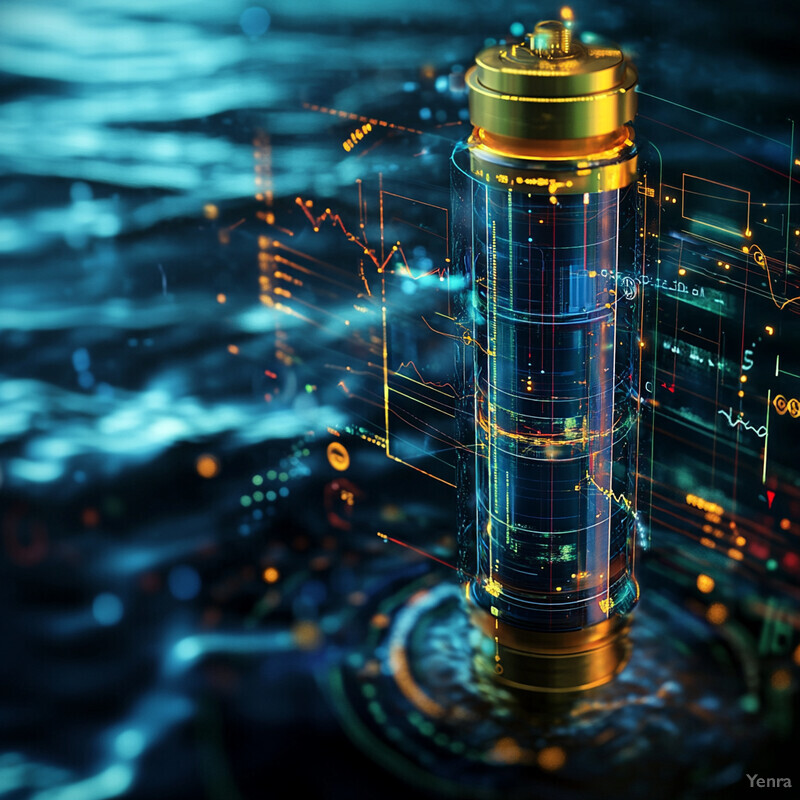
The constant exposure of tidal turbines to harsh currents leads to material fatigue, corrosion, and other structural degradations over time. AI-enabled SHM systems analyze sensor data—from strain gauges, ultrasonic probes, and acoustic emissions—to detect subtle changes in structural integrity. Through pattern recognition and anomaly detection algorithms, these systems pinpoint early-stage damage that might be imperceptible to human inspectors. Early detection allows for targeted maintenance or reinforcement, preventing catastrophic failures, improving safety, prolonging the lifespan of the installation, and ultimately increasing the return on investment.
6. Hydrodynamic Modeling and Simulation
Deep learning techniques refine computational fluid dynamics models, allowing for more accurate simulations of water flow, turbulence, and wake effects, which lead to better turbine designs and improved array layouts.
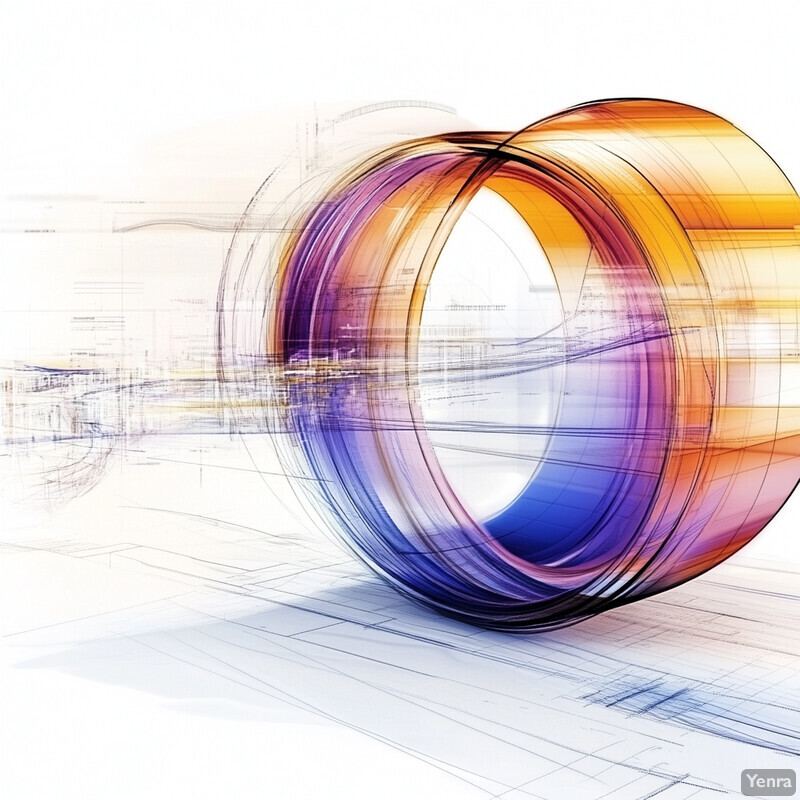
Complex hydrodynamic interactions, including turbulence, wakes, and vortices, are vital considerations in tidal turbine design and placement. AI-enhanced computational fluid dynamics (CFD) leverage machine learning techniques—such as reduced-order modeling and neural network surrogates—to accelerate and refine simulations. These advanced simulations can achieve higher accuracy with less computational cost, enabling engineers to experiment with multiple design iterations rapidly. Enhanced hydrodynamic modeling informs improved turbine geometry, spacing between units, and mooring strategies, leading to more efficient energy extraction and reduced environmental impact.
7. Adaptive Control Systems
Intelligent control algorithms adjust operational parameters in response to complex and changing oceanic conditions, ensuring that tidal arrays remain stable and efficient even under extreme events like storm surges.
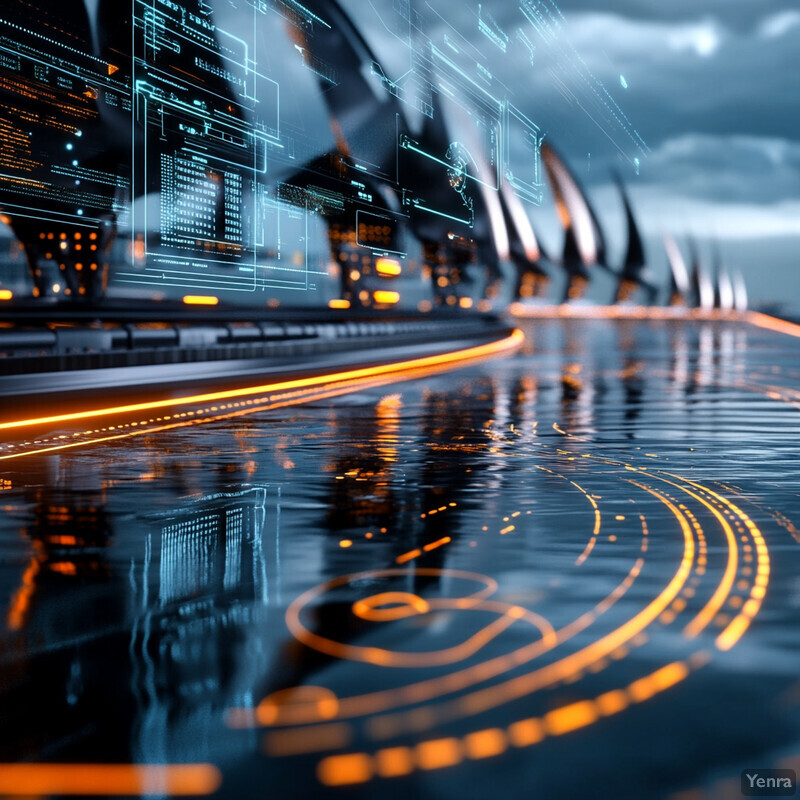
The ocean environment is inherently unpredictable, with tidal currents influenced by coastal topography, weather conditions, and seasonal shifts. AI-driven adaptive control systems, often employing reinforcement learning and fuzzy logic, allow tidal turbines and arrays to respond dynamically to fluctuating conditions. These intelligent controllers fine-tune operational parameters to maintain stability and efficiency even when faced with sudden flow changes or extreme events like storm surges. This adaptability not only improves energy yield but also protects equipment from undue stress and extends operational windows during challenging marine conditions.
8. Array Layout Optimization
Genetic algorithms and other heuristic optimization methods consider wake interactions, environmental constraints, and seabed topography to determine the optimal spatial configuration of multiple turbines for maximum energy extraction.
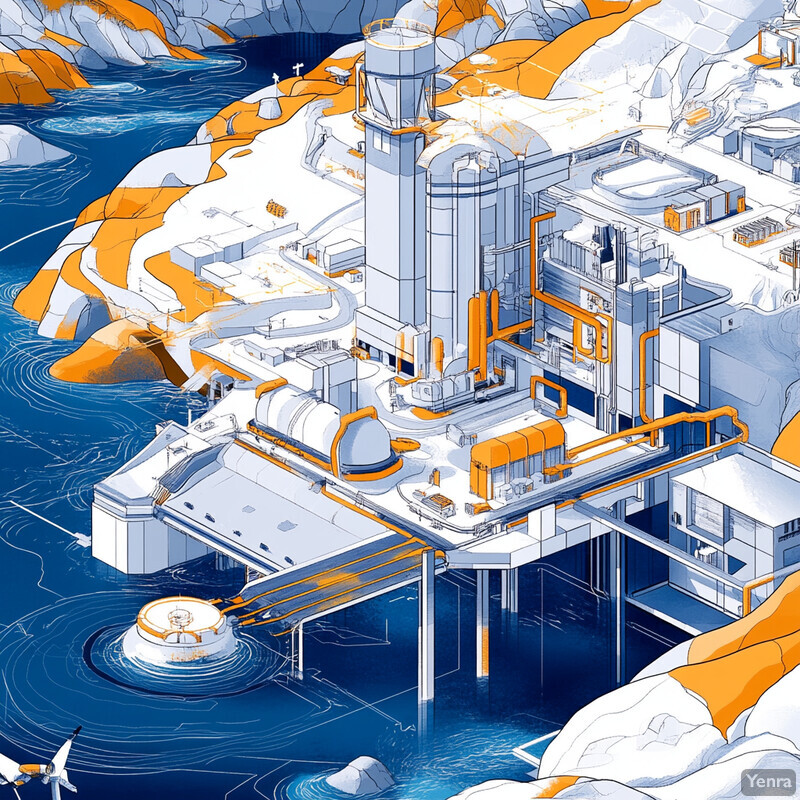
Placing multiple tidal turbines in a single location requires careful planning to avoid turbulent wake effects and to maximize collective output. AI optimization methods, such as genetic algorithms or particle swarm optimization, evaluate countless array configurations, each with slightly different turbine positions, orientations, and depths. By iteratively refining these layouts based on simulated or measured performance, AI identifies array arrangements that capture the most energy with the least interference. This leads to improved efficiency, reduced mechanical stress on individual turbines, and enhanced overall productivity of the tidal farm.
9. Energy Storage Integration
Machine learning tools help balance supply and demand by predicting tidal energy availability and managing hybrid systems that incorporate batteries or pumped hydro storage, resulting in smoother power delivery to the grid.
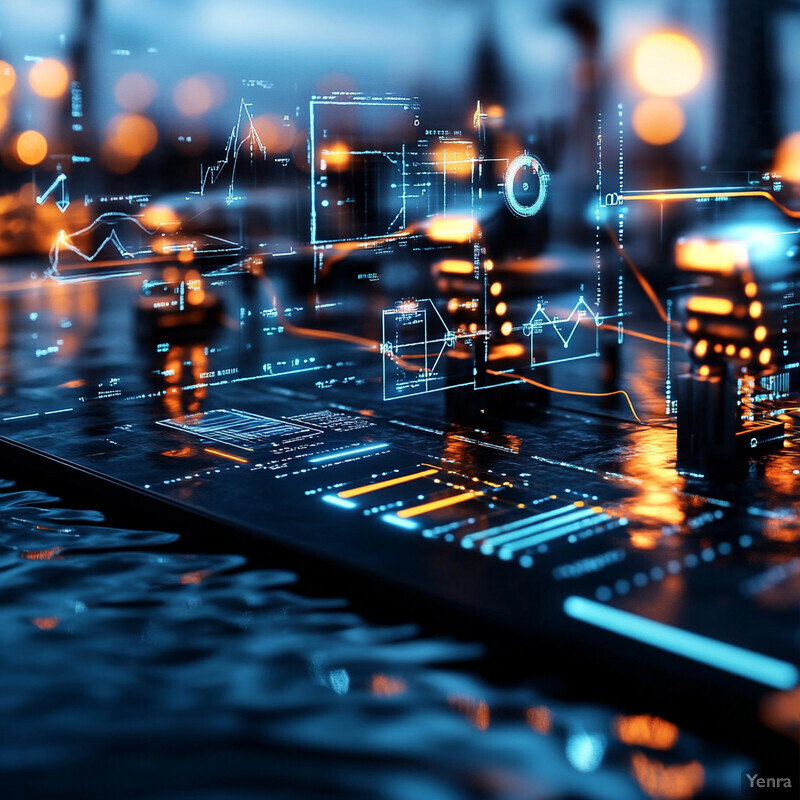
The variability of tidal energy—though more predictable than wind—still poses challenges for consistent power supply. AI helps manage hybrid energy systems by analyzing predicted energy generation and comparing it against anticipated demand. Machine learning models forecast when tidal output will peak or wane and determine optimal times to store excess energy in batteries, flywheels, or pumped hydro systems. This intelligent storage integration smooths out power delivery, reducing stress on the grid and improving the reliability and market value of tidal energy resources.
10. Grid Integration and Load Forecasting
AI-based forecasting predicts when and how much tidal power will be available, allowing grid operators to integrate tidal energy more effectively, reduce curtailment, and maintain grid stability.
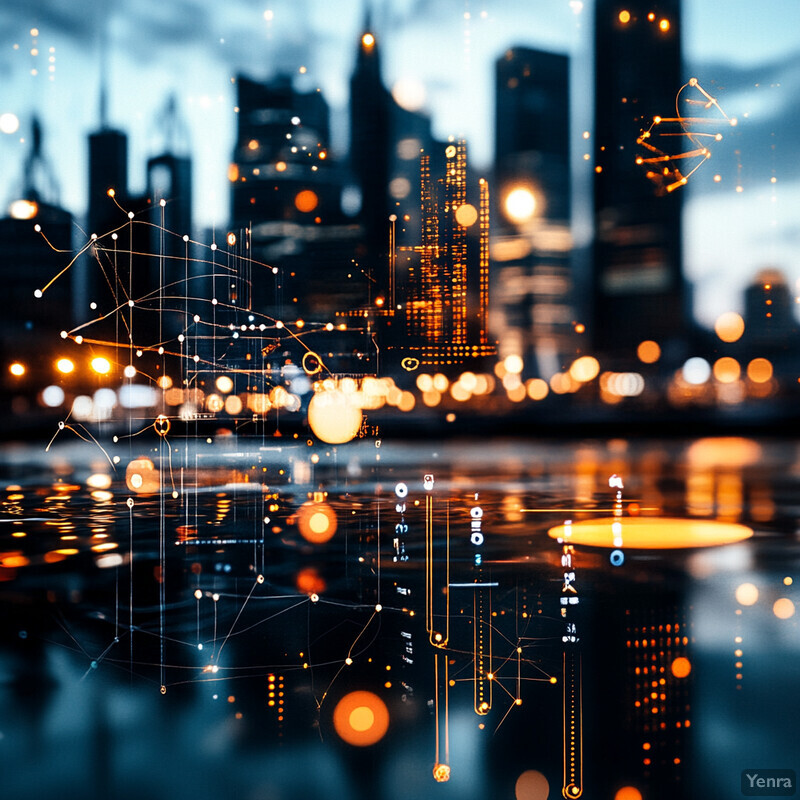
As tidal energy gains a larger share in the energy mix, grid operators need accurate predictions of power availability to maintain balance and stability. AI-based load forecasting models incorporate tidal predictions, historical demand patterns, and other renewable energy inputs to inform grid scheduling. By predicting periods of abundant or scarce tidal power, these tools help utilities plan reserves, orchestrate smart load shifting, and reduce the risk of blackouts. Enhanced grid integration ensures that tidal energy becomes a dependable contributor to the overall energy portfolio, supporting both energy security and renewable targets.
11. Data-Driven Design of Turbine Blades
By applying AI-driven optimization to material selection, blade shape, and structural configurations, engineers can create more efficient and durable turbine blades tailored to local tidal conditions.
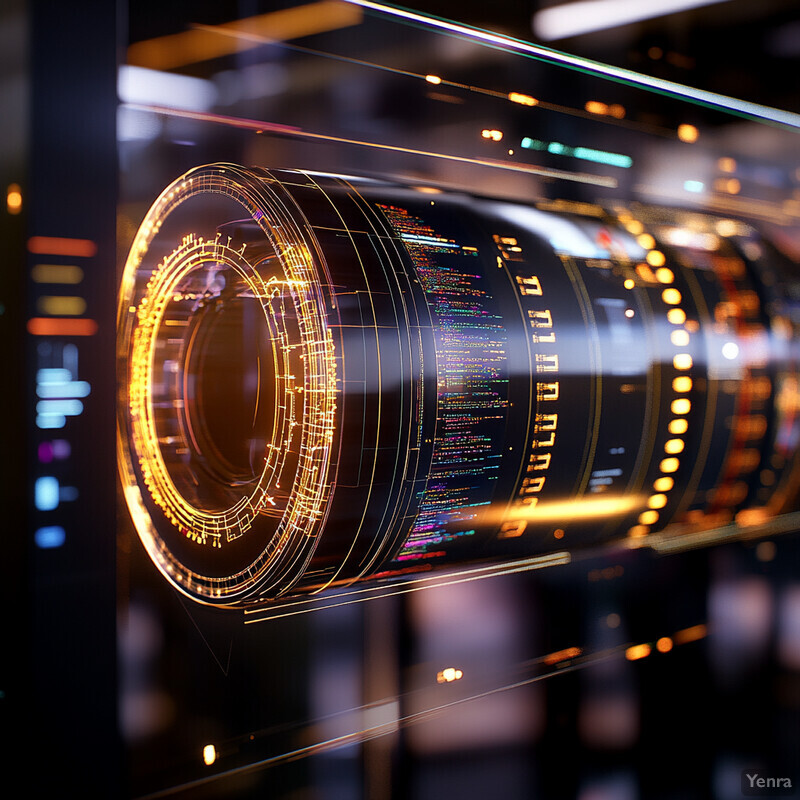
The performance and durability of turbine blades depend on their shape, material composition, and resilience against marine environments. Machine learning models assist in blade design by analyzing large volumes of data on fluid flow patterns, stress distributions, and material fatigue. Designers can experiment with new materials—such as advanced composites—and blade geometries in virtual environments. By iterating rapidly and leveraging AI-driven optimization, engineers produce blades that are lighter, stronger, and more efficient, ultimately leading to higher energy output and reduced maintenance costs.
12. Noise and Environmental Impact Minimization
Intelligent models assess various operational strategies for reducing turbine-induced noise and ecological disturbances, helping operators comply with environmental regulations and protect marine life.
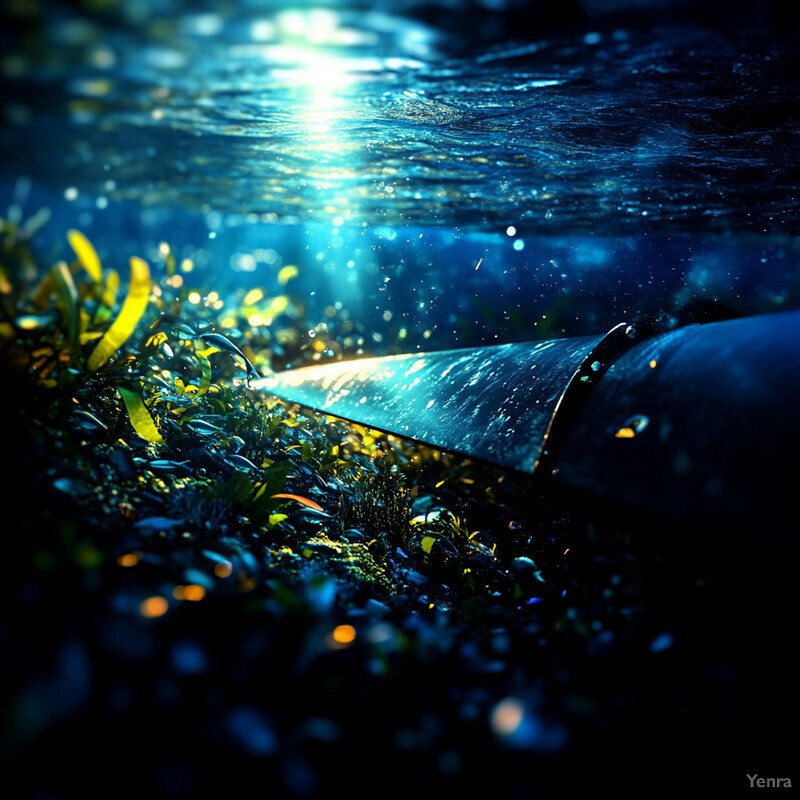
Tidal turbines, like all mechanical systems, generate noise and vibrations that can affect marine life. AI tools analyze complex relationships between turbine operation and acoustic emissions. By adjusting operational parameters—rotor speed, blade angles, or operational cycles—algorithms find configurations that reduce noise levels without significant energy losses. Combined with acoustic monitoring and marine species distribution modeling, this approach helps operators maintain compliance with environmental regulations and minimize disturbances to marine ecosystems. Achieving a balance between technological efficiency and ecological stewardship enhances the long-term viability of tidal energy projects.
13. Predicting Marine Life Interactions
AI-based image and acoustic recognition systems analyze marine fauna presence and behavior near turbines. This helps operators adjust turbine operation in real-time to reduce risks to wildlife.
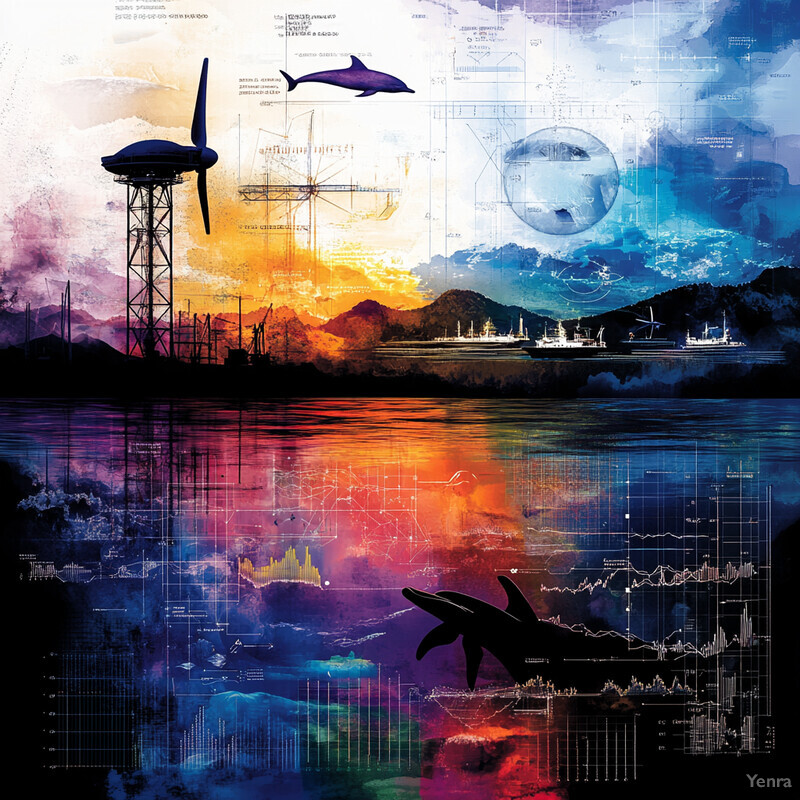
Tidal sites often coincide with habitats for fish, marine mammals, and other fauna. AI-powered computer vision and acoustic classification systems use underwater cameras, sonar, and hydrophones to identify and track marine species near turbines. By recognizing behavioral patterns—such as how marine mammals navigate around devices—machine learning models can predict collision risks or habitat disruptions. Operators can adjust turbine speed or temporarily shut down operations during critical migration periods. This proactive approach protects marine biodiversity, fosters regulatory compliance, and strengthens the social license to operate tidal energy farms.
14. Condition-Based Monitoring of Gearboxes and Generators
Advanced analytics detect subtle anomalies in mechanical components, allowing timely interventions that avoid catastrophic failures and extend the operational life of these costly subsystems.
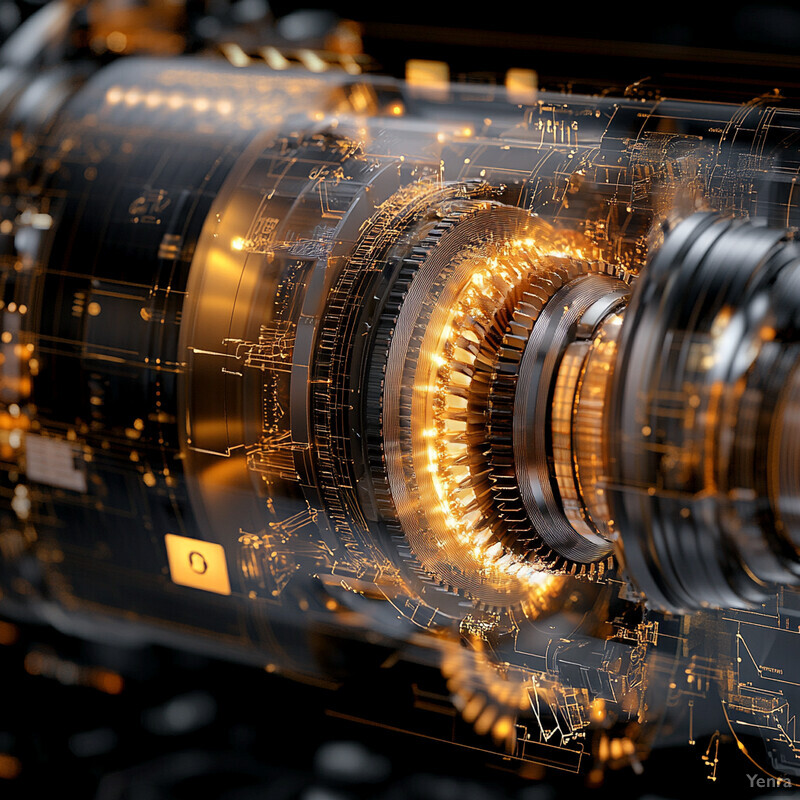
Gearboxes and generators are crucial components that endure continual mechanical stress. AI-based condition monitoring systems interpret sensor inputs—like vibration signatures, torque fluctuations, and oil quality indicators—to detect emerging faults. Machine learning methods can distinguish between normal operational patterns and signs of wear or misalignment. Recognizing early deviations allows maintenance teams to schedule inspections and part replacements before small issues escalate into severe damage. This not only reduces operational costs but also ensures uninterrupted energy output and enhances the economic attractiveness of tidal energy projects.
15. Materials Performance Prediction
Machine learning accelerates the discovery and characterization of new corrosion-resistant materials or coatings, increasing the endurance and performance of submerged turbine components.
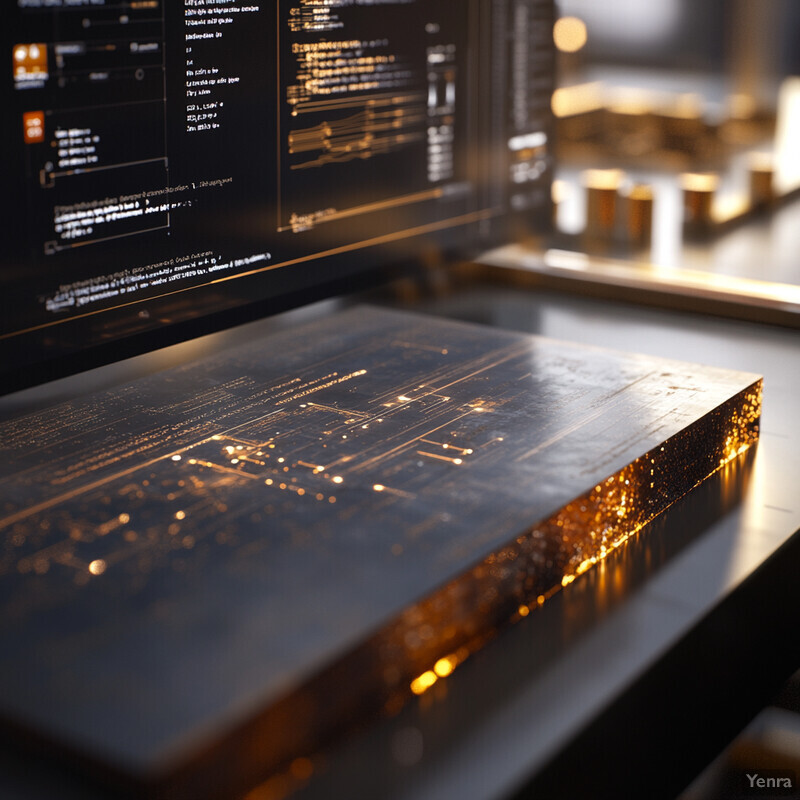
Developing corrosion-resistant and fatigue-tolerant materials for tidal turbines is essential due to constant exposure to saltwater and biological fouling. AI accelerates this materials research by analyzing experimental and simulated data on coatings, alloys, and composites. Machine learning models identify correlations between chemical compositions, material processing methods, and long-term performance metrics. As a result, engineers can rapidly screen candidates and predict which materials will best withstand harsh marine conditions. Improved materials translate to longer-lasting turbines, lower maintenance costs, and greater investor confidence.
16. Long-Term Performance Forecasting
AI tools integrate environmental data, maintenance logs, and historical performance metrics to project future energy output and operational costs, supporting better financial and strategic planning.
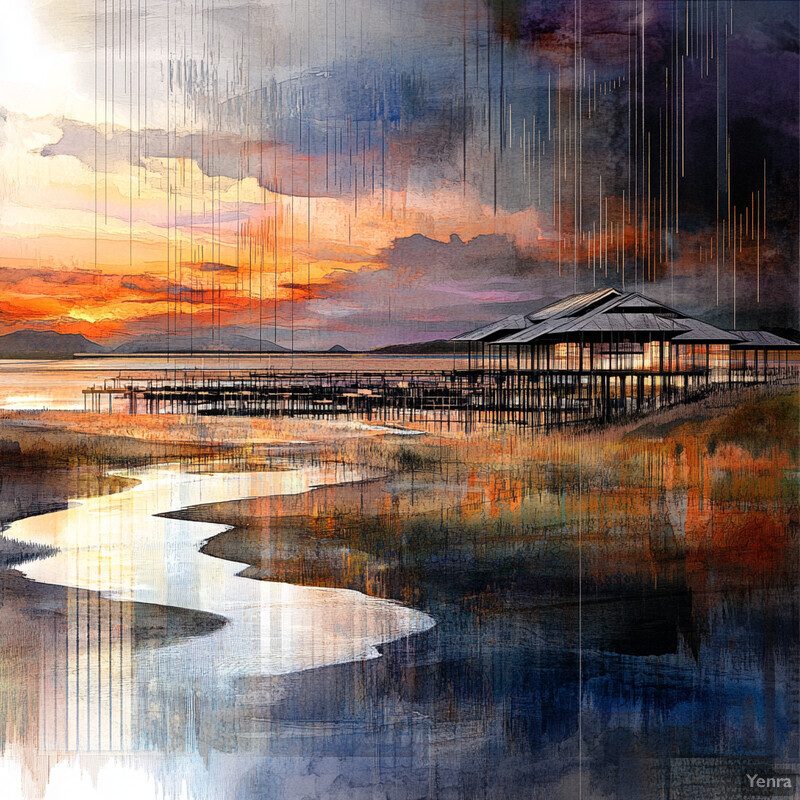
The value of a tidal energy project is determined by its long-term stability and output. AI models integrate multiple data streams—maintenance logs, past operational performance, environmental shifts, and energy pricing trends—to forecast future conditions. These forecasts guide strategic decisions, such as when to upgrade equipment, renegotiate power purchase agreements, or adjust financing structures. By quantifying uncertainty and projecting performance over decades, AI tools help stakeholders optimize investment returns and mitigate risks, ensuring the financial sustainability of tidal energy ventures.
17. Adaptive Learning from Operational Experience
As tidal farms gather more operational data, AI systems learn continuously from real-world experience, improving decision-making, efficiency, and resilience over time without the need for manual reprogramming.
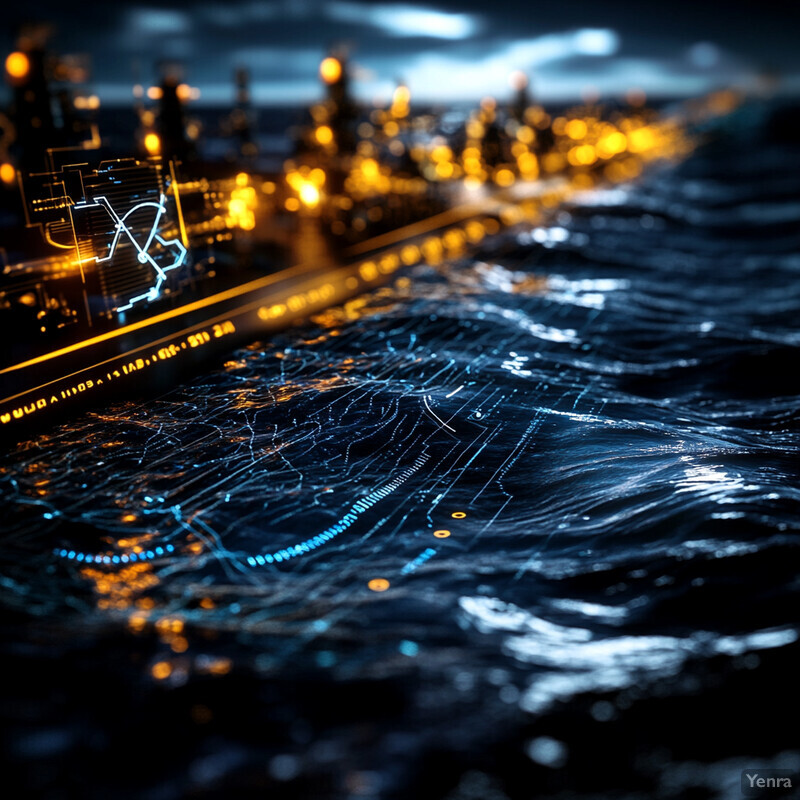
As more tidal turbines are deployed, the volume of operational data grows exponentially. AI systems can continuously learn from this ever-expanding dataset, refining their models for resource assessment, control optimization, and predictive maintenance. Over time, algorithms become more accurate and robust, adapting to subtle changes in environmental patterns or technology improvements. Such 'living' AI systems mean that tidal farms don’t remain static; instead, they evolve and improve continuously, ultimately leading to increased efficiency, cost savings, and resilience in the face of environmental variability and technological advancements.
18. Sensor Fusion and Data Integration
Complex AI models integrate data from multiple sensor arrays—flow velocity sensors, pressure gauges, sonar, LIDAR—to provide a holistic, high-resolution view of turbine operations and the underwater environment.
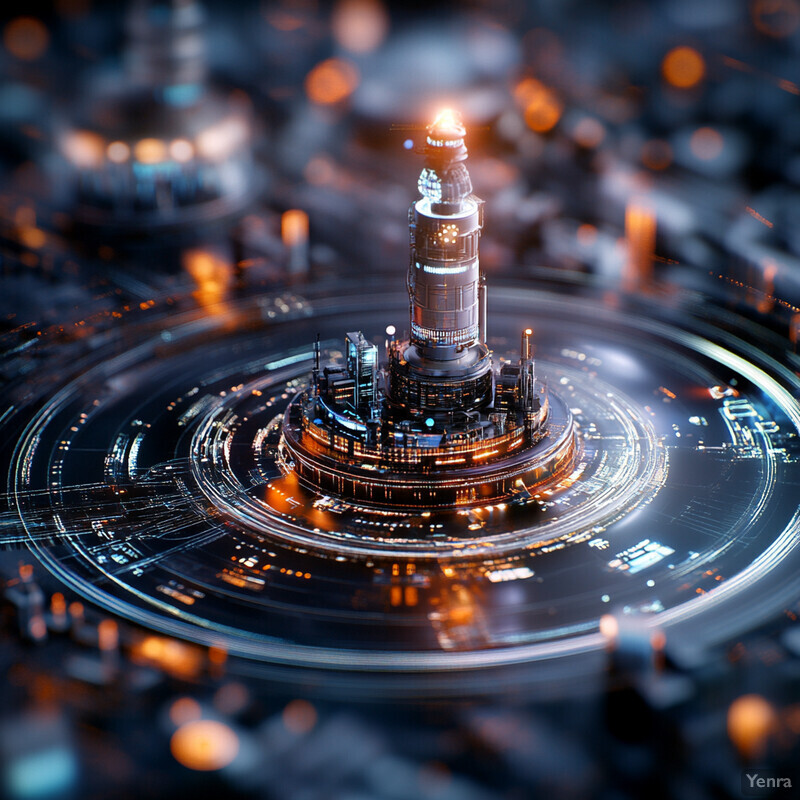
A tidal energy installation produces a multitude of data streams—flow velocity measurements, pressure sensors, sonar imaging, LIDAR scanning, and operational metrics. AI-based sensor fusion techniques integrate these disparate sources into a coherent, high-resolution dataset. By combining and cross-referencing different data types, machine learning models derive richer insights into environmental conditions, turbine performance, and structural health. This holistic understanding allows for more nuanced decision-making, improved diagnostics, and refined optimization strategies, ensuring that the entire system operates at its full potential.
19. Uncertainty Quantification and Risk Management
Probabilistic machine learning methods help operators understand uncertainties in tidal predictions, failure probabilities, and revenue streams, enabling risk-informed strategies for investment and operations.
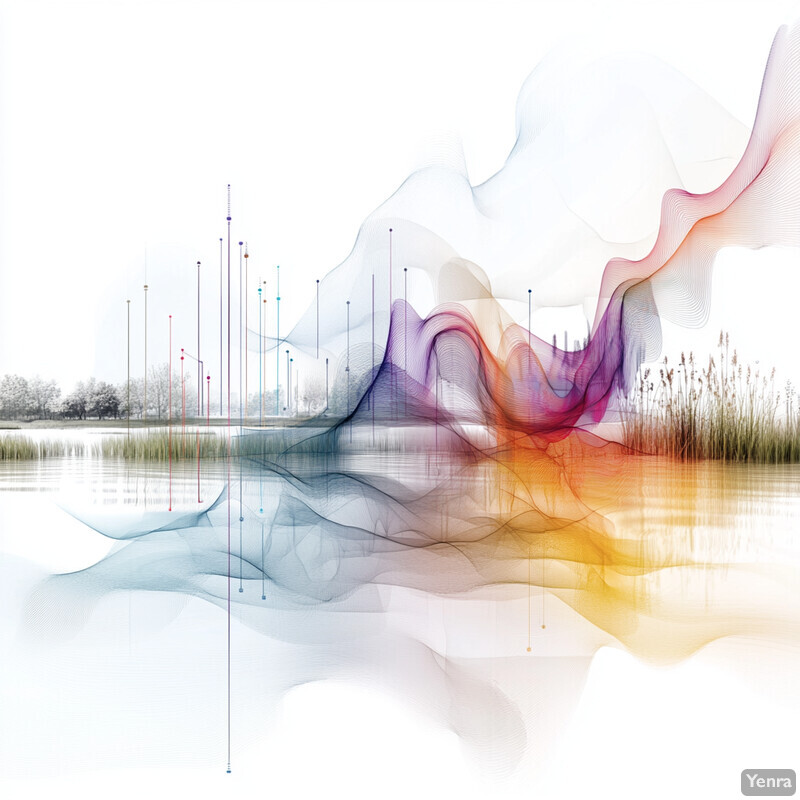
Uncertainty is inherent in predicting tidal patterns and equipment performance. AI-driven uncertainty quantification methods, such as Bayesian neural networks, assess the probability distribution of various outcomes—from expected energy production to potential equipment failures. Understanding the range of possible scenarios allows operators, investors, and regulators to manage risks more effectively. By planning for contingencies and diversifying strategies (e.g., adjusting maintenance schedules or hedging through energy markets), project stakeholders improve resilience, reduce potential losses, and enhance overall confidence in tidal energy systems.
20. Automated Anomaly and Fault Detection
AI systems can rapidly identify unexpected changes in operational patterns—such as sudden dips in power output or unusual vibration signatures—and trigger automated alerts or corrective actions, reducing response times and operational losses.
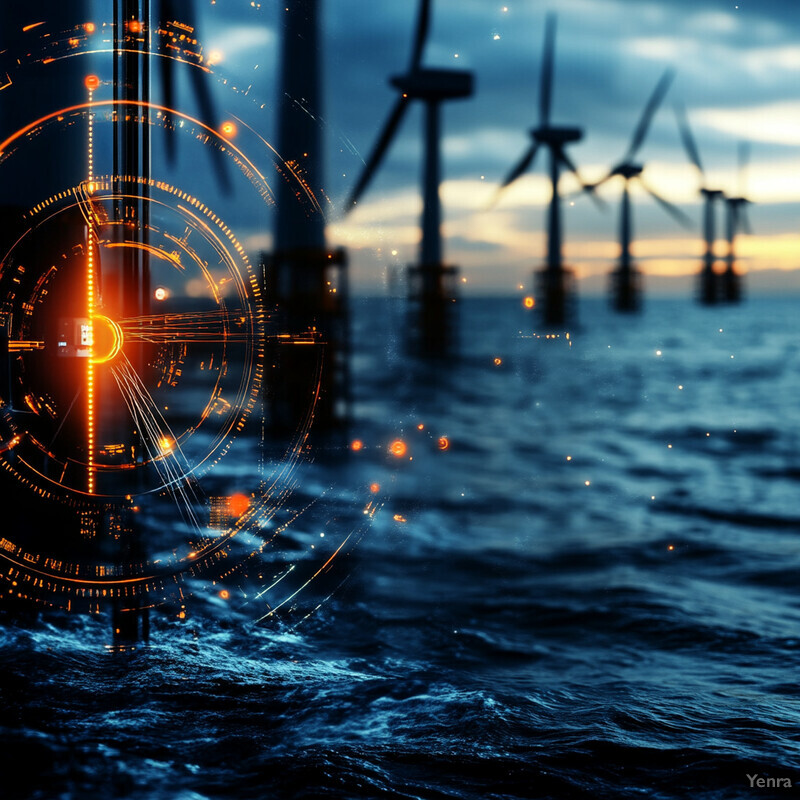
Early detection of anomalies—such as sudden drops in power output or unusual vibration patterns—is crucial to maintaining the efficiency and longevity of tidal turbines. AI-based anomaly detection systems learn what 'normal' operation looks like and quickly flag deviations that suggest mechanical issues, sensor malfunctions, or environmental disturbances. Automated alerts prompt timely inspections, remote diagnostics, or automated adjustments that can prevent cascading failures. By swiftly responding to potential problems, these systems keep downtime to a minimum, ensuring steady energy production, cost savings, and higher return on investment for tidal energy operators.