1. Automated Sign Recognition
Advanced computer vision algorithms and deep learning models can now accurately detect and recognize signs from live video, providing immediate feedback to learners on their handshape, movement, and facial expressions.
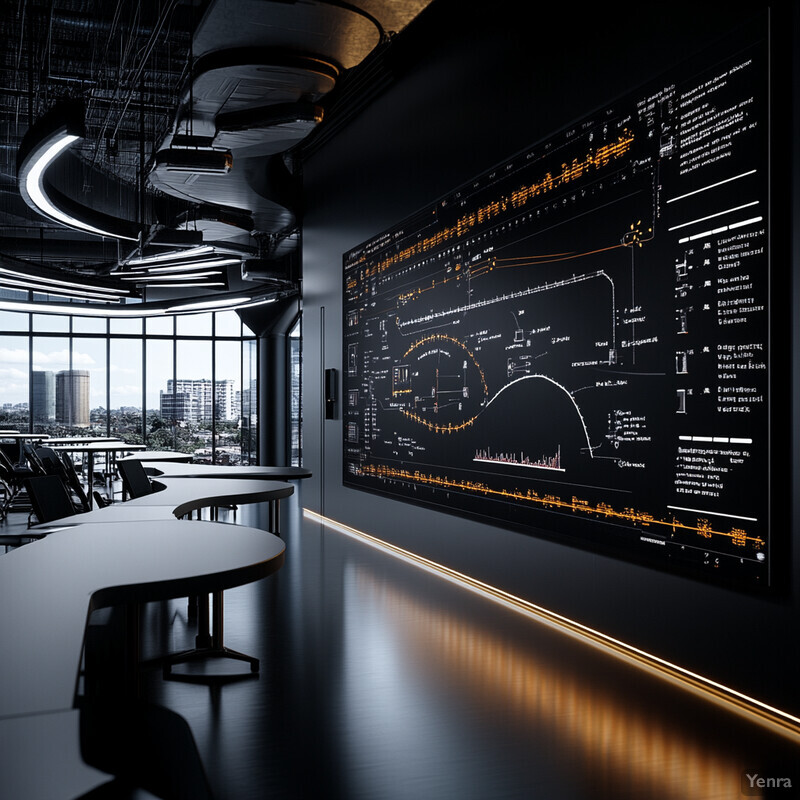
Advancements in computer vision and deep learning have led to sophisticated models that can accurately detect and interpret signs from live video input. These models use extensive training sets of recorded signers to learn variations in handshape, orientation, location, and movement. When a learner attempts a sign, the system compares it against known templates or learned patterns, assessing correctness, clarity, and similarity to native-speaker models. This automated recognition capability allows learners to receive immediate, objective feedback on each sign they produce, accelerating the process of error correction and skill acquisition.
2. Gesture and Movement Tracking
AI-driven motion tracking systems use cameras or wearable sensors to capture a learner’s gestures in real-time, analyzing subtle differences in hand position, orientation, and motion.
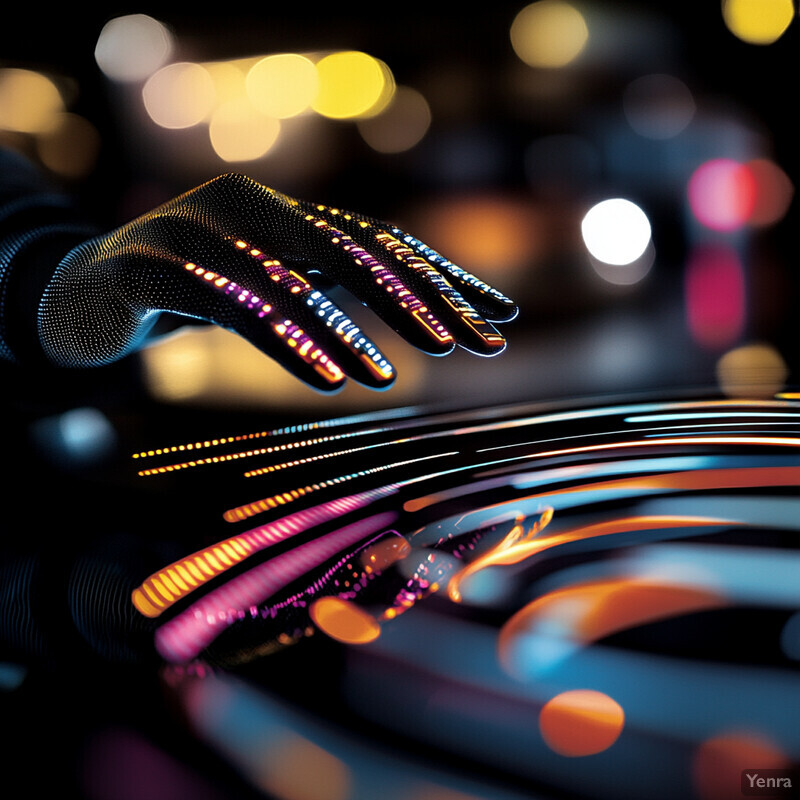
AI-driven motion tracking integrates specialized sensors or high-definition cameras with machine learning algorithms to monitor the subtle details of a learner’s hand movements. These systems capture three-dimensional coordinates of the learner’s hands and body in real time, allowing the software to assess alignment, curvature, speed, and fluidity. Through continuous feedback loops, learners gain insights into their technique—such as correcting a handshape’s angle or adjusting the speed of a sign—much earlier than traditional, human-only feedback environments. This meticulous guidance supports deeper muscle memory development and more native-like signing performance.
3. Pronunciation and Fluency Feedback
These AI tools assess the fluidity and speed of sign transitions, offering personalized corrections to improve fluency and naturalness.
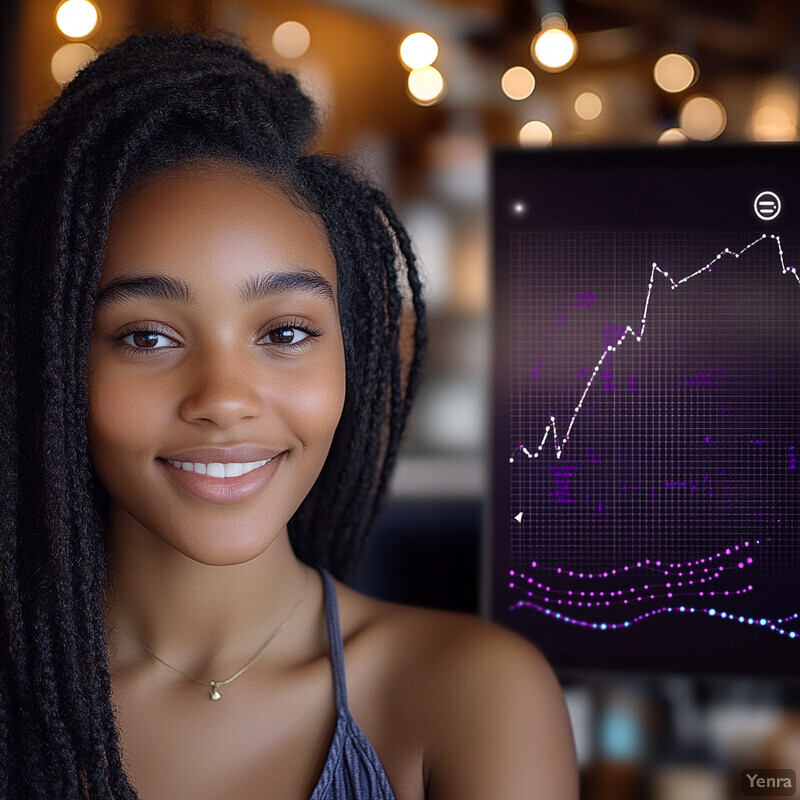
In spoken language learning, speech recognition tools offer phonetic accuracy checks; similarly, AI in sign language tutoring can measure the fluency and “pronunciation” quality of signed utterances. By analyzing sign transitions, rhythm, and overall temporal structure, these systems can determine if the learner’s signing pattern resembles that of fluent signers. Fluent signing is not just about producing the right sign, but also about how seamlessly signs are connected. AI tools identify if the learner’s transitions are too abrupt, too slow, or lack the natural flow. This feedback improves not only accuracy, but also the learner’s comfort and efficiency in communicating in sign language.
4. Adaptive Curriculum Personalization
By analyzing a user’s learning patterns, errors, and progress, AI can adjust lesson difficulty and create individualized learning paths.
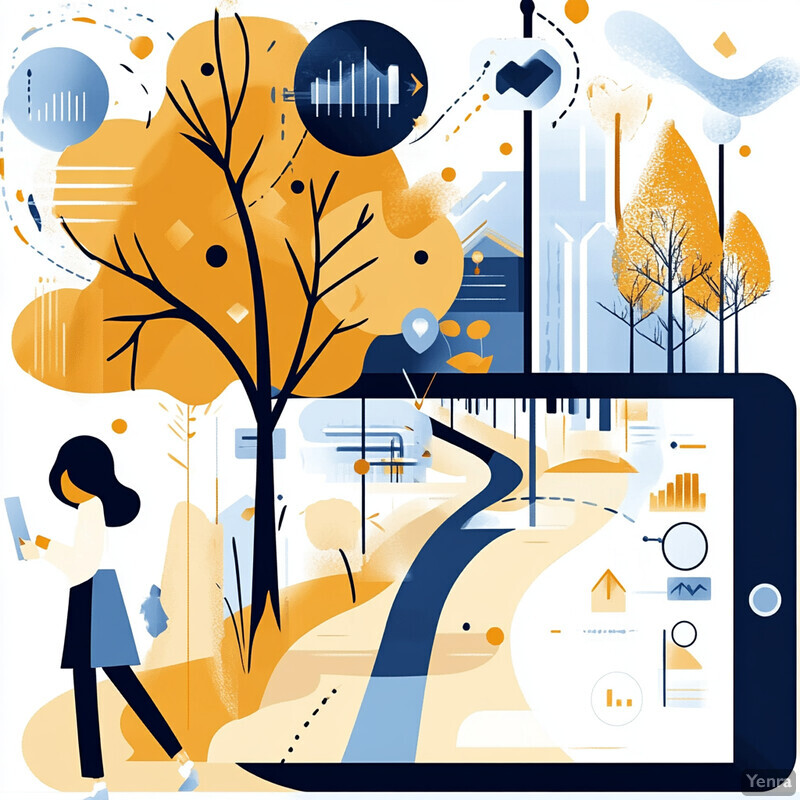
Artificial intelligence excels in pattern recognition and predictive modeling. By monitoring a learner’s progress, including which signs cause repeated difficulty and the nature of their errors, AI systems can dynamically adjust the lesson plans. Instead of a static, one-size-fits-all curriculum, learners receive tailored exercises that focus on their weaker areas, gradually introducing more complex signs as their proficiency improves. This personalization helps maintain motivation and ensures that each learner’s unique path through the language is as effective and efficient as possible. Over time, this adaptive approach produces learners who have a more balanced and well-rounded skill set.
5. Contextual Understanding of Signs
AI systems increasingly understand the context in which signs are used, helping learners grasp nuances and usage in real-world scenarios.
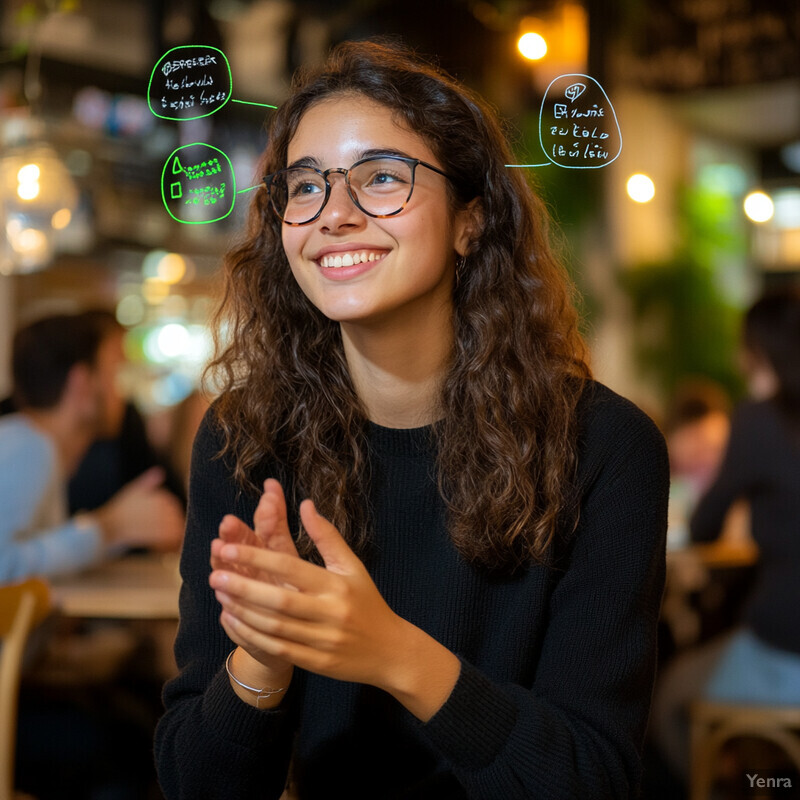
Sign languages are not merely collections of isolated signs; context, sentence structure, facial expressions, and the broader communicative environment all influence meaning. AI models trained on large datasets can differentiate between signs that look similar yet have distinct meanings depending on context. By teaching learners how to recognize and produce signs in appropriate contexts—such as understanding when a certain sign should be accompanied by a specific facial expression—these systems help learners internalize the pragmatic and semantic nuances of sign language. This results in more natural and culturally accurate communication skills.
6. Facial Expression Recognition
AI models can detect subtle facial cues that carry grammar and emotional meaning in sign languages, providing feedback on accuracy.
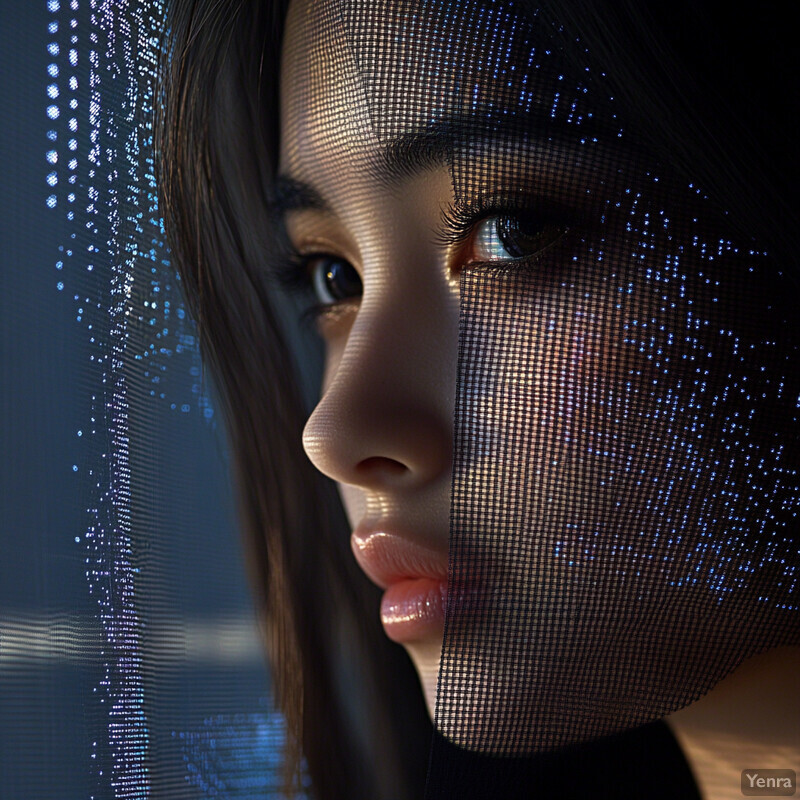
Facial expressions, head tilts, and eyebrow movements are integral parts of sign languages, adding grammatical and emotional layers that words alone cannot convey. AI-equipped tutoring systems now incorporate advanced facial recognition algorithms to capture and analyze these non-manual signals. By providing feedback on whether the learner’s facial expressions are consistent with the sign’s intent, these systems guide users in mastering subtle cues. Over time, this leads to more authentic and precise communication, ensuring that learners not only sign correctly but also convey tone, emotion, and grammatical features accurately.
7. Interactive Virtual Tutors
AI-driven avatars, modeled after skilled deaf signers, can converse interactively with learners and respond to their signing in real time.
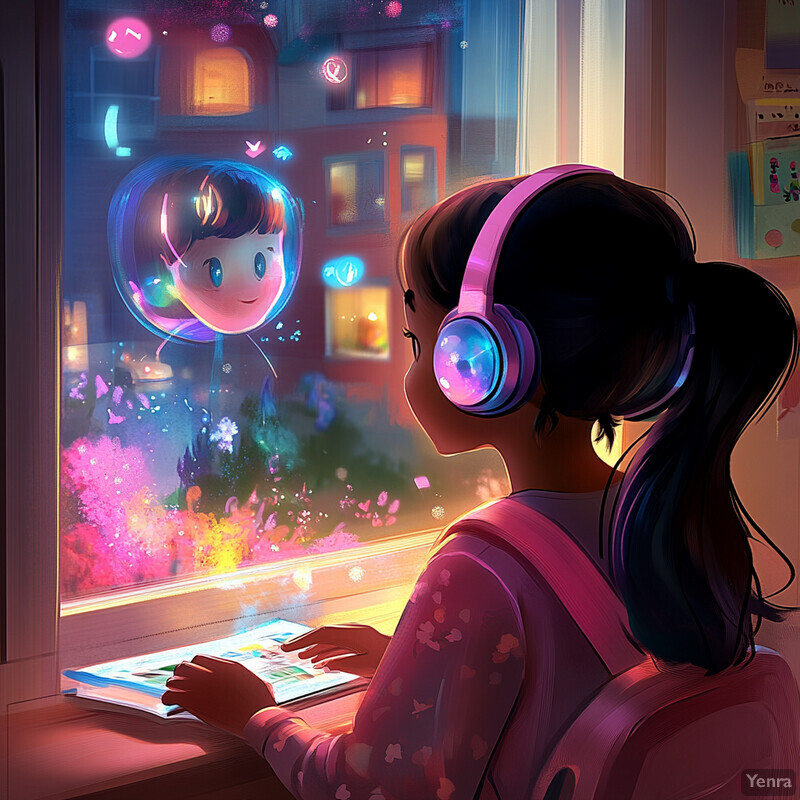
The development of AI-driven avatars and virtual signing tutors has revolutionized the self-learning experience. These avatars can model correct sign production, interact in simulated conversations, and respond dynamically to the learner’s input. Unlike prerecorded video lessons, virtual tutors can ask questions, offer corrections, and suggest new vocabulary in real time. Learners benefit from a responsive, conversational partner who is always available, breaking down geographical and temporal barriers to language acquisition. The result is a more immersive learning environment that can mimic the benefits of a live tutor without the scheduling constraints.
8. Real-Time Feedback Systems
Learners can receive instantaneous comments on their sign accuracy, speeding up the learning cycle and boosting confidence.
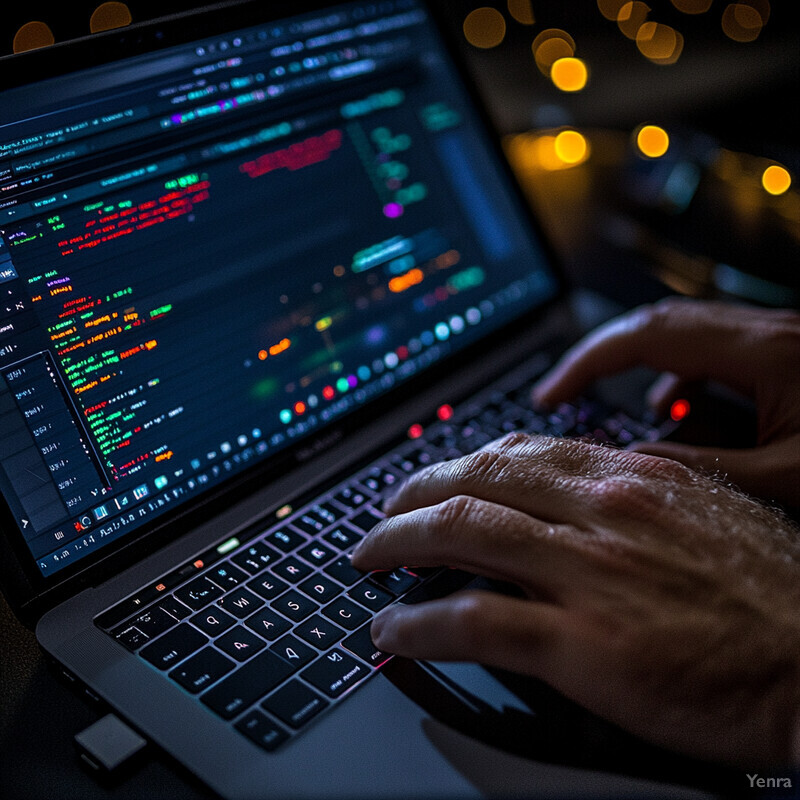
Instantaneous feedback is key to maintaining engagement and accelerating improvement. AI-powered sign language tutoring systems can immediately highlight errors—be it an incorrect handshape, a misplaced sign location, or an unnatural transition—right as they occur. This differs significantly from traditional methods where learners must wait until a teacher is available for correction. With instant guidance, learners can adjust their production on the fly, reinforcing correct techniques and avoiding the formation of bad habits. Over time, this approach builds confidence and leads to more rapid progress.
9. Gamified Learning Environments
AI-enhanced platforms integrate gamification—quizzes, memory challenges, and sign recognition games—to keep learners motivated.
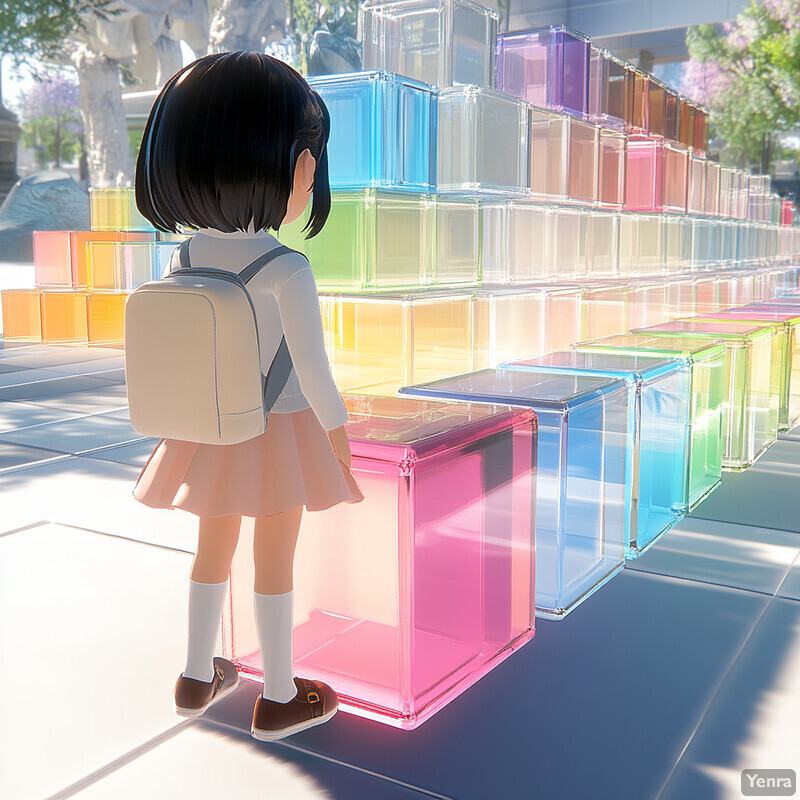
To sustain motivation and enhance the learning experience, many AI-enhanced platforms employ gamification elements. Learners might earn points or badges for correctly signing difficult vocabulary, improving fluency over time, or completing interactive quizzes. The AI monitors their performance, adapting the difficulty level and recommending the next challenge at just the right time. This keeps learners in the zone of proximal development—neither bored nor overwhelmed. As a result, learners remain engaged, and their progression feels natural and rewarding, contributing to better long-term retention of language skills.
10. Pronunciation Variation Modeling
AI models trained on diverse datasets recognize regional variations, helping learners adapt to different dialects and styles of signing.
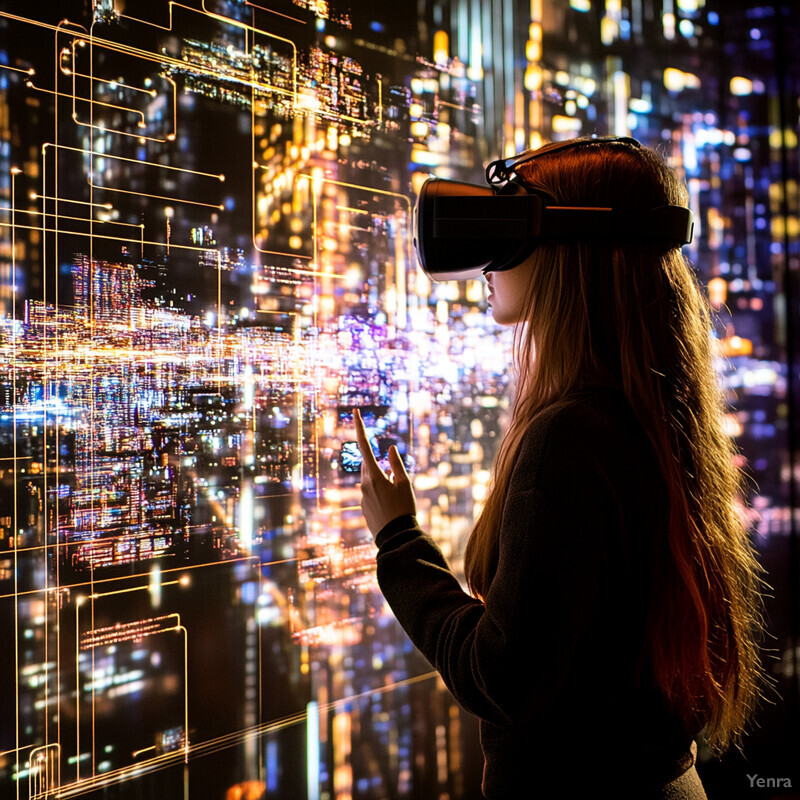
Just as spoken languages have dialects and regional accents, sign languages also vary by region and community. AI models trained on diverse datasets learn to recognize these differences. By exposing learners to a wide range of signing styles, they become familiar with regional nuances, slang, and stylistic variations. This ensures that learners are not limited to one “standard” form of the language but are better prepared for real-world interactions with different signing communities. Over time, this cultural and linguistic breadth leads to more versatile and socially competent communicators.
11. Error Pattern Analysis
AI can identify recurring learner mistakes, highlighting weak points and guiding targeted practice.
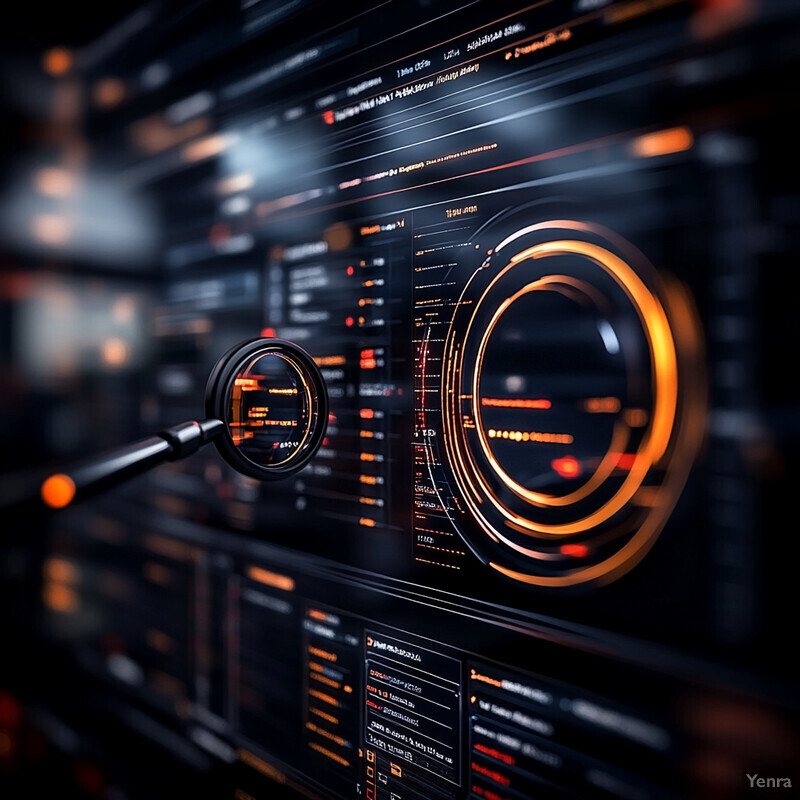
Advanced data analytics allow AI systems to identify recurring error patterns in a learner’s signing. Rather than treating each mistake as isolated, the system groups related issues—such as persistent trouble with a certain handshape or consistent confusion between two similar signs. This pattern recognition helps both educators and learners understand the root causes of difficulties. Armed with this insight, learners can focus on targeted drills, and instructors can refine teaching strategies. The result is a more efficient learning process, as time is spent addressing core issues rather than correcting random, unconnected errors.
12. Natural Language Processing Integration
By combining NLP with sign recognition, AI systems can translate signed input into written language and vice versa for a multimodal experience.
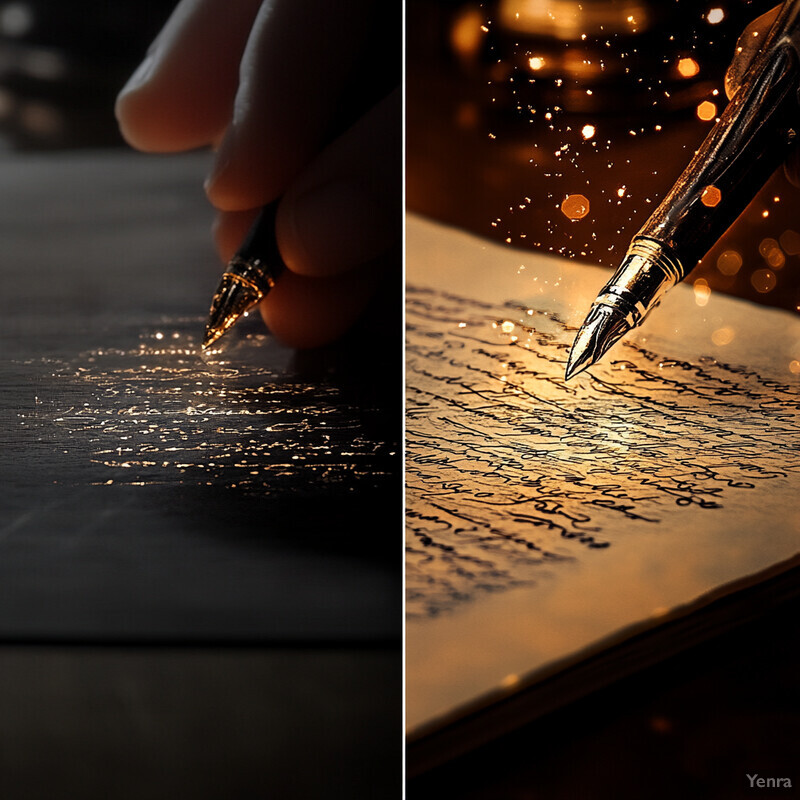
Sign languages and written/spoken languages can complement each other in learning environments. By integrating Natural Language Processing (NLP) with sign recognition, AI systems can provide translations of signed input into textual output, or vice versa. This multimodal approach allows learners to see how signs correspond to words, phrases, and sentences in a spoken language, and to practice receptive skills by watching signed videos and answering comprehension questions in text. By bridging the gap between modalities, learners gain a more holistic understanding of language structure and usage.
13. Content Recommendation Systems
AI suggests supplemental videos, interactive tasks, or community forums based on user skill level, interests, and goals.
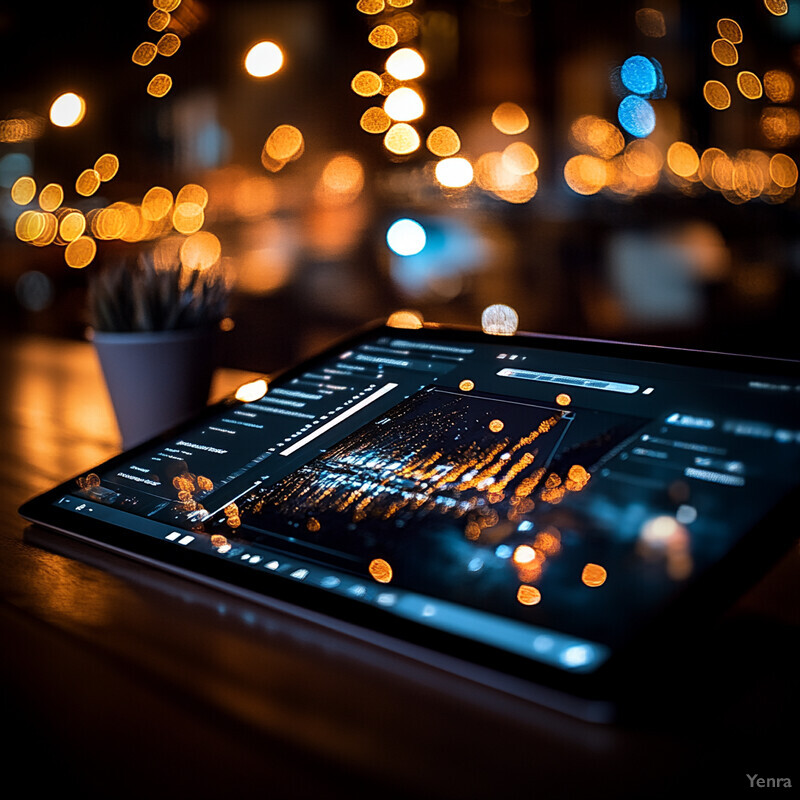
Recommendation engines, powered by AI, curate personalized content based on the learner’s progress, preferences, and challenges. If a learner struggles with particular signs or grammatical constructions, the system suggests supplementary videos, targeted exercises, or additional reading material. Conversely, if they excel in certain areas, more advanced content can be introduced. This adaptive content delivery ensures that learners remain challenged but not frustrated, and that they have a steady stream of fresh, relevant practice materials to maintain engagement and foster continuous growth.
14. Data-Driven Curriculum Refinement
Institutions can use AI analytics from large learner cohorts to pinpoint common pitfalls and improve instructional design.
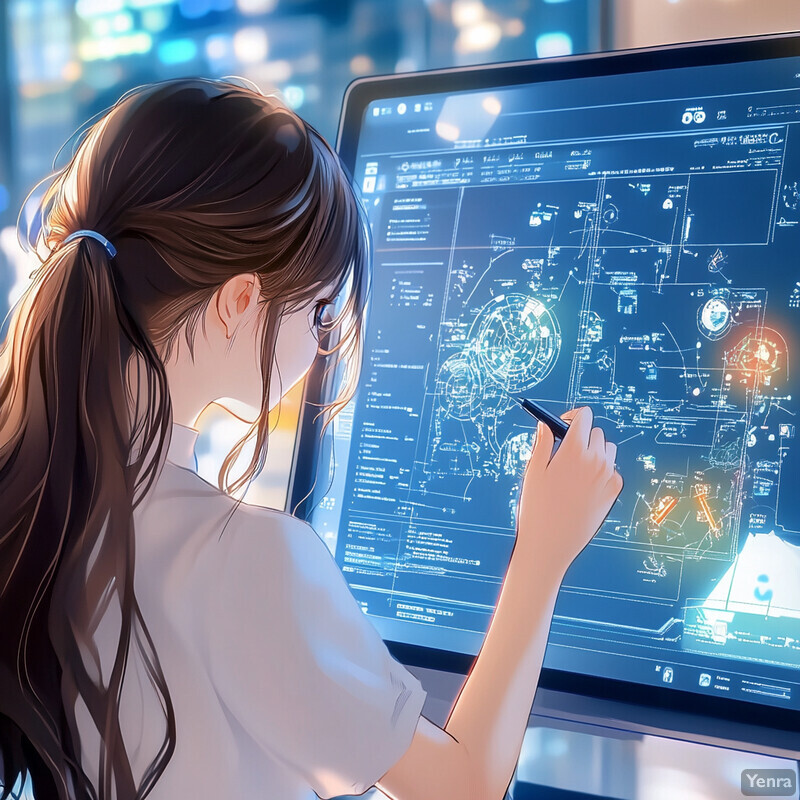
At a higher level, educational institutions and language programs can leverage anonymized and aggregated AI analytics. By studying large groups of learners, AI can identify which parts of the curriculum lead to common misunderstandings or slow progress. Armed with these insights, curriculum developers and educators can modify their lesson plans, incorporate clearer examples, or design improved instructional materials. This continuous improvement loop ensures that future learners benefit from an ever-more effective and evidence-based teaching approach.
15. Progress Visualization Tools
Learners receive dashboards and analytics that display their improvement over time, highlighting strengths and weaknesses.
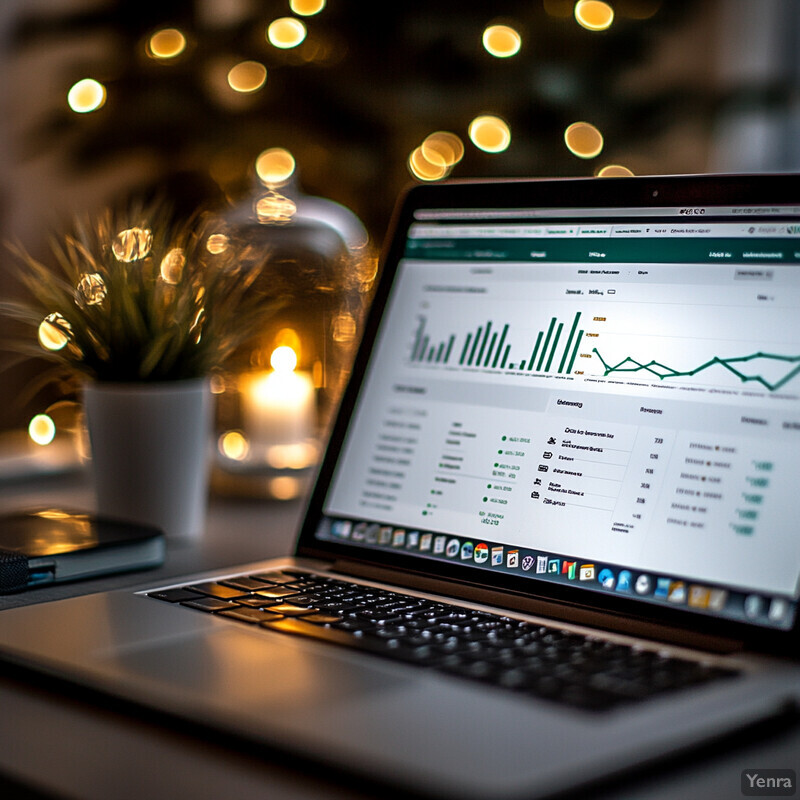
Visualizing improvement is a strong motivator. AI-driven analytics can create detailed dashboards and charts showing how a learner’s accuracy, fluency, and vocabulary size evolve over time. Graphs can highlight which areas have shown improvement and which remain challenging. Seeing tangible evidence of one’s growth helps maintain motivation and allows learners to set informed, realistic goals. This transparency turns a possibly abstract journey—mastering a sign language—into a measurable progression, making it easier to celebrate milestones and understand the path ahead.
16. Integration with Wearable Technology
Innovative AI systems can leverage wearables—such as gloves or wristbands—to capture precise hand orientation and motion data in real time.
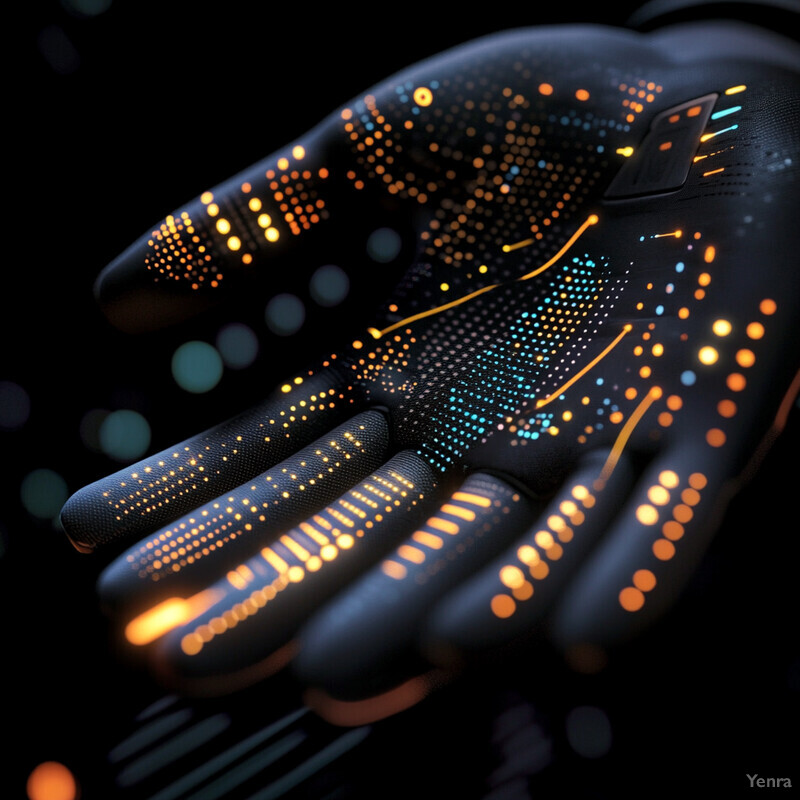
Wearable devices like sensor-laden gloves or wristbands can capture tactile and kinesthetic data about a learner’s signing. These devices relay fine-grained information about muscle tension, hand orientation, and movement patterns to AI-driven analysis tools. The immediate feedback—sometimes via haptic signals—helps learners adjust their techniques in ways that would be impossible with traditional instruction alone. Integrating wearables introduces a multi-sensory learning approach, helping learners refine their skills more precisely and remember correct movements through muscle memory.
17. Linguistic Rule Enforcement
AI engines check if learners are forming sentences accurately, respecting the grammar and syntax of the target sign language.
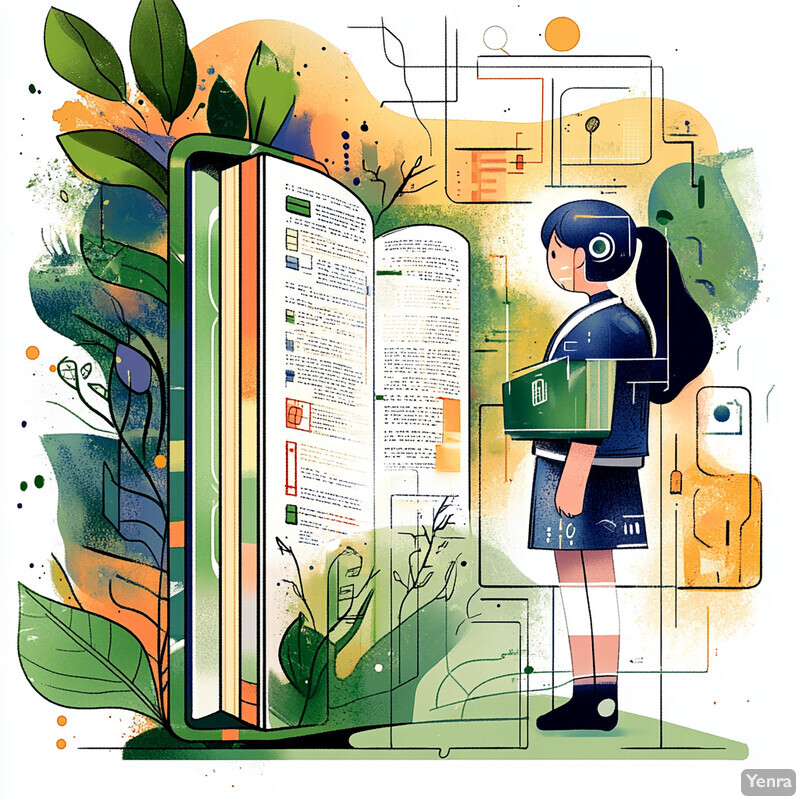
Sign languages have their own grammatical structures, which may differ significantly from spoken languages. AI systems can be programmed with the linguistic rules governing sign order, facial expressions as grammatical markers, and sentence structure. When learners produce a sentence, the AI evaluates not only the correctness of individual signs but also the compliance with these linguistic rules. Offering constructive feedback when learners deviate from standard grammar ensures they develop not just vocabulary and expressions, but also the essential linguistic competence that underpins fluent and meaningful communication.
18. Cross-Lingual Transfer Learning
AI models trained on multiple signed languages help learners quickly understand differences and similarities between them.
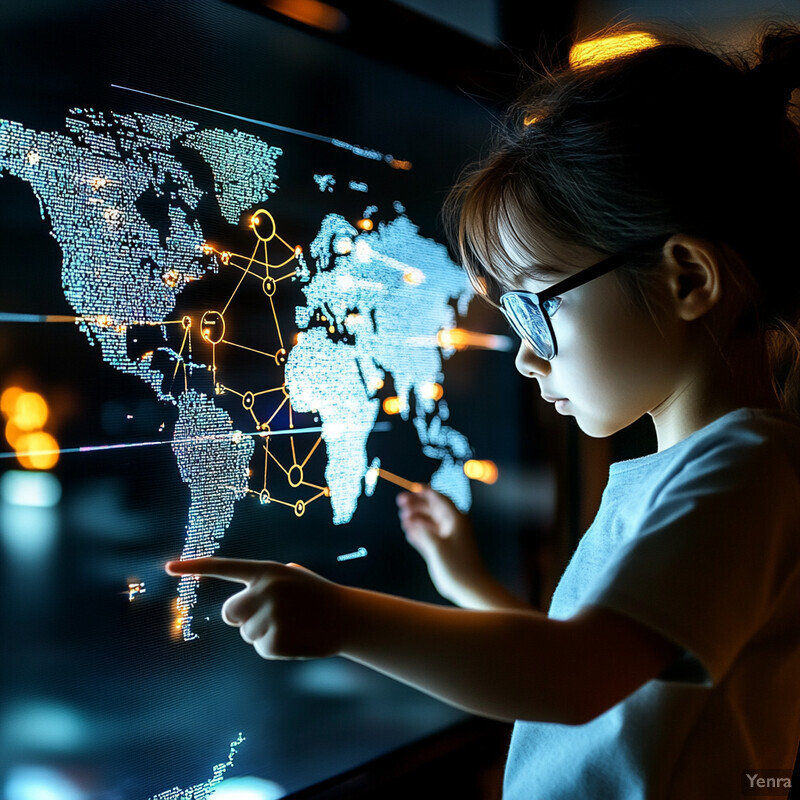
Transfer learning models can be trained on multiple sign languages, recognizing patterns that span across languages. By comparing similarities and differences, these systems can help learners quickly adapt when learning a new sign language. For instance, knowledge of American Sign Language may accelerate learning British Sign Language if the system highlights parallel structures or related signs. Over time, this “linguistic scaffolding” allows more experienced learners to become polyglots of sign languages, deepening their cultural and communicative competencies.
19. Augmented Reality Assistance
AR tools can overlay visual guides on the learner’s real-world environment, showing correct hand positioning and transitions.
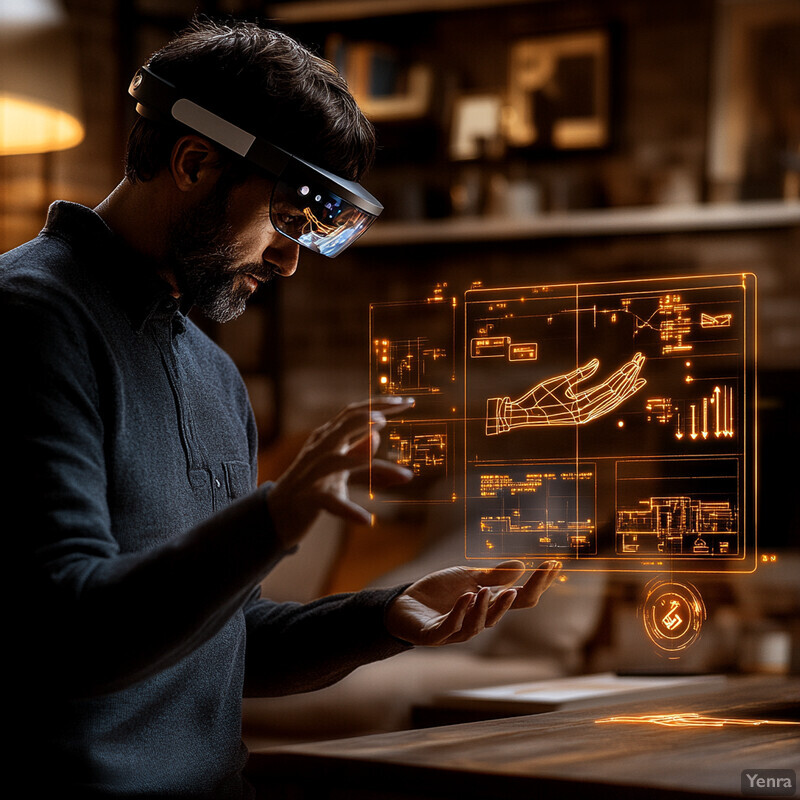
Augmented Reality (AR) applications, powered by AI, can overlay digital elements onto the learner’s physical environment. By using a smartphone or headset, learners see their own hands and body with guides or markers indicating correct hand positions and movements. As they attempt signs, the AI system checks their performance and provides instant visual cues for improvement, making the learning experience highly interactive and intuitive. This spatial and visual support helps internalize correct techniques more rapidly and reduces the frustration that often accompanies ambiguous feedback.
20. Access for Diverse Learners
AI technologies are making sign language tutoring more accessible to remote learners, busy professionals, and those with physical constraints.
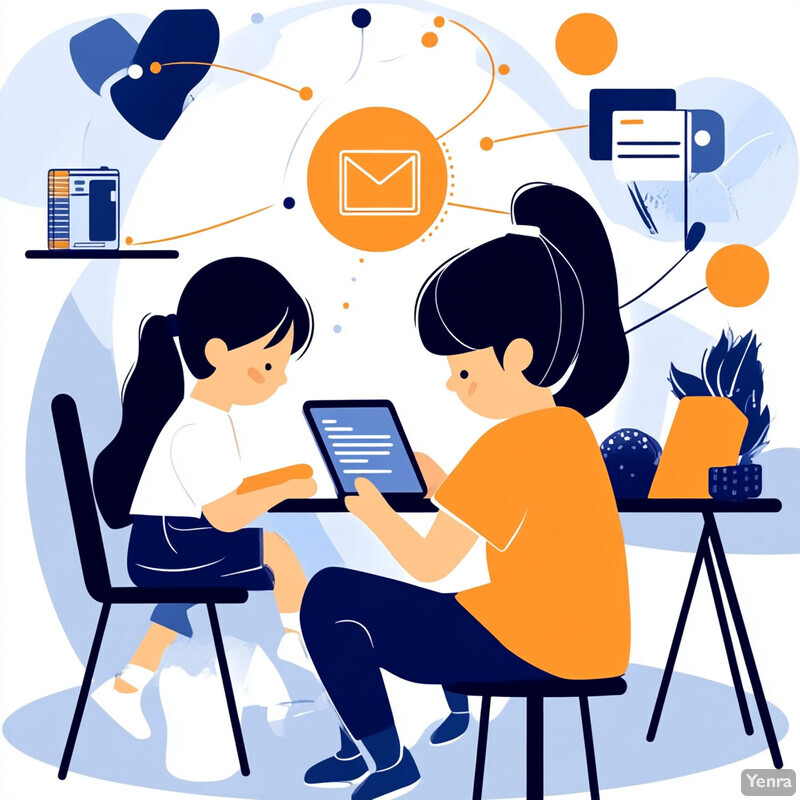
Not everyone has access to skilled sign language instructors, especially those in remote areas or with inflexible schedules. AI-powered tutoring systems democratize the learning process by providing high-quality instruction on demand. They cater to diverse learners—busy professionals, stay-at-home parents, or those with physical disabilities—by adjusting pace, complexity, and focus. Since the system is available online, learners can practice whenever it suits them, thus overcoming geographical, social, and logistical barriers. This inclusivity contributes to broader language adoption and fosters more vibrant, connected signing communities.