1. Data-Driven Planograms
AI can automatically generate optimized planograms by analyzing historical sales, margin data, and product attributes, minimizing manual guesswork.
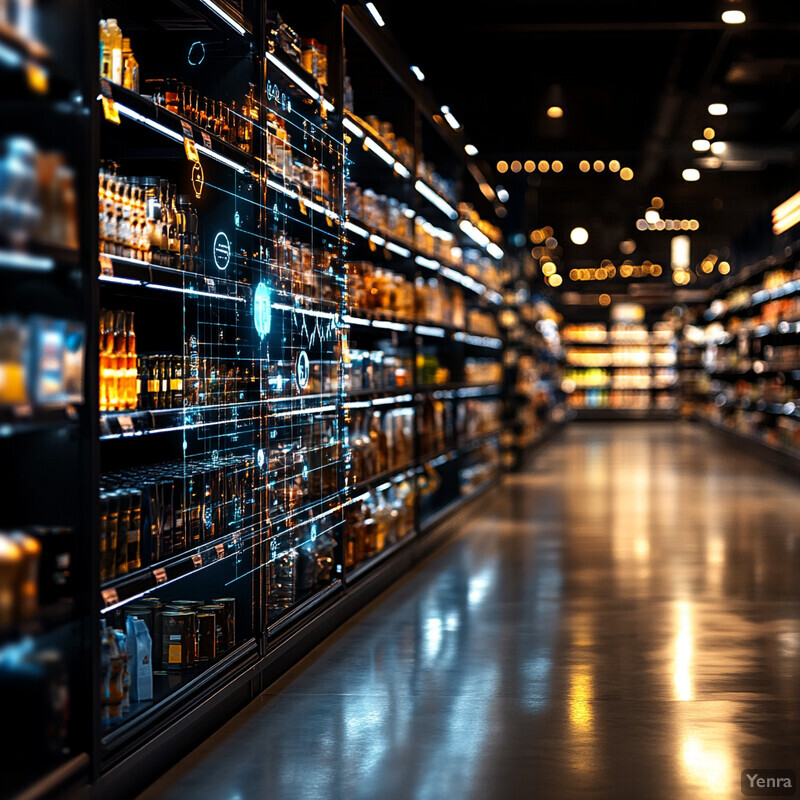
AI-powered systems use large volumes of historical sales data, product category information, and item-level performance metrics to create planograms that no longer rely on intuition or static guidelines. By examining patterns in consumer demand, seasonal fluctuations, and profit margins, these algorithms can assign prime shelf space to best-sellers and identify the ideal arrangement of products to maximize both visibility and revenue. The result is a data-driven blueprint that is dynamic rather than one-size-fits-all, improving store efficiency and ensuring a more appealing assortment for customers.
2. Predictive Demand Forecasting
Machine learning algorithms predict future demand at a granular level, enabling shelves to be stocked with the right products at the right times.
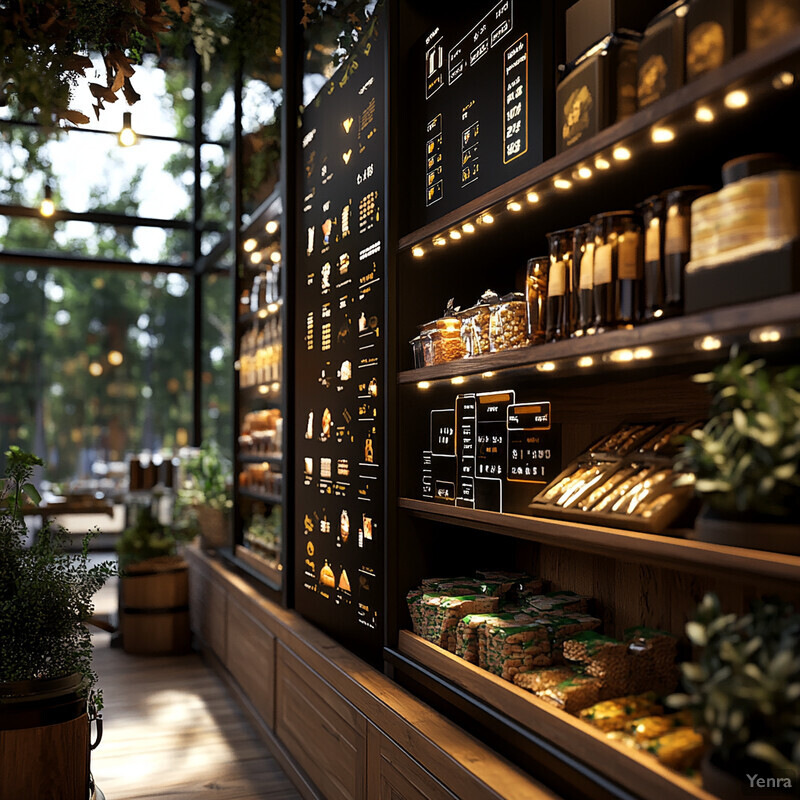
Instead of relying on past performance or broad market trends alone, AI-driven models leverage advanced forecasting techniques to predict product demand with granular accuracy. These models consider factors such as local weather patterns, upcoming holidays, economic indicators, and competitor promotions. By foreseeing changes in purchasing behavior, retailers can stock shelves with the right quantities at the right times. This not only mitigates the risks of overstock and stock-outs but also ensures that high-demand items are always available, enhancing customer satisfaction and driving sales.
3. Dynamic Product Placement
AI can determine the ideal placement for each product to maximize visibility and increase basket size, continuously adjusting to shifts in sales patterns.
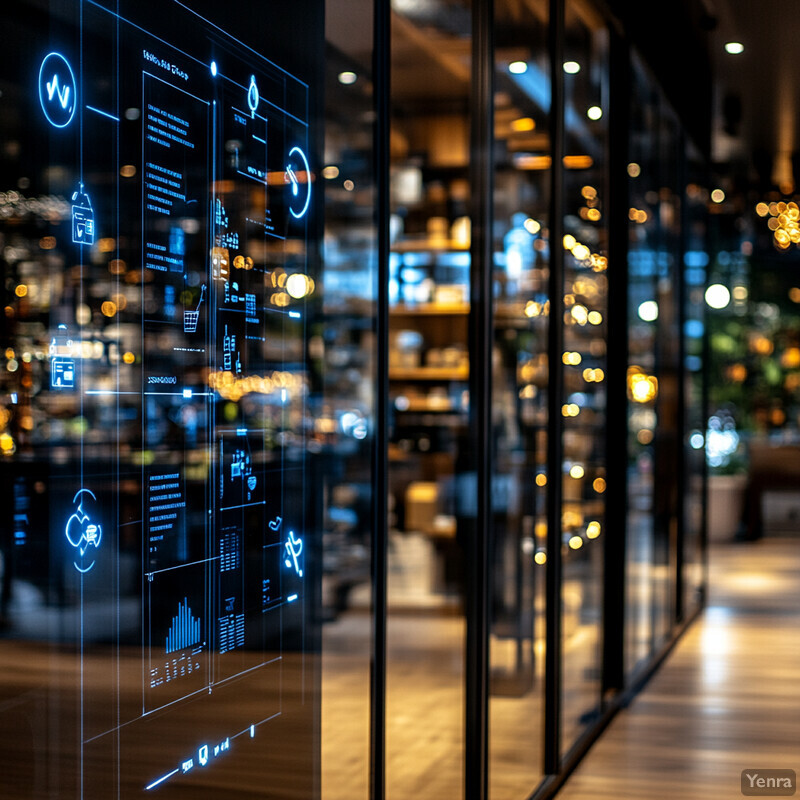
AI enables stores to continuously refine which products go where, based on real-time performance metrics. Through machine learning, systems identify which shelf locations garner the most attention and how product adjacencies influence purchasing decisions. For instance, if certain snack items sell more when placed near beverages, AI-driven layout optimization will rearrange product placements accordingly. Over time, this dynamic approach maximizes shelf productivity and ensures that store layouts remain in lockstep with changing shopping patterns and consumer preferences.
4. Automated Space Allocation
Advanced optimization models allocate shelf space more efficiently, balancing product breadth with inventory depth to reduce stock-outs and overstock.
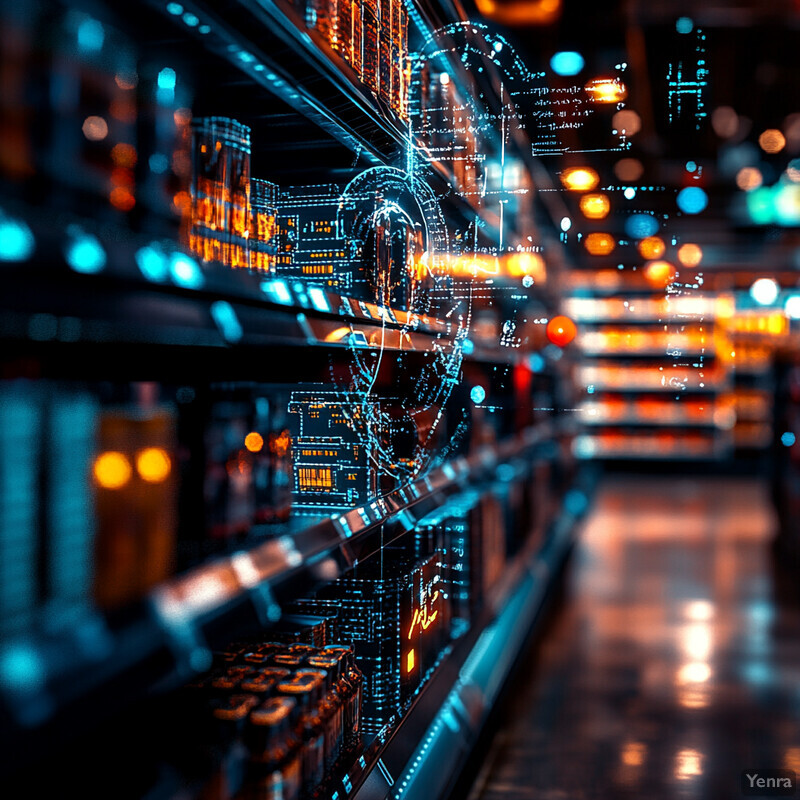
Shelf space is a finite and valuable resource, and AI excels at allocating it efficiently. Algorithms weigh factors like product size, profitability, sales velocity, and brand guidelines to determine the optimal amount of space each SKU should occupy. This approach eliminates guesswork and manual recalculations as consumer trends shift. By automatically balancing variety against inventory depth, AI reduces both wasted space and the labor cost involved in constantly tweaking displays, ultimately creating a more profitable and organized retail environment.
5. Real-Time Shelf Auditing
Computer vision, combined with AI-driven analytics, can identify empty spots, misplaced products, and planogram non-compliance, alerting staff for immediate fixes.
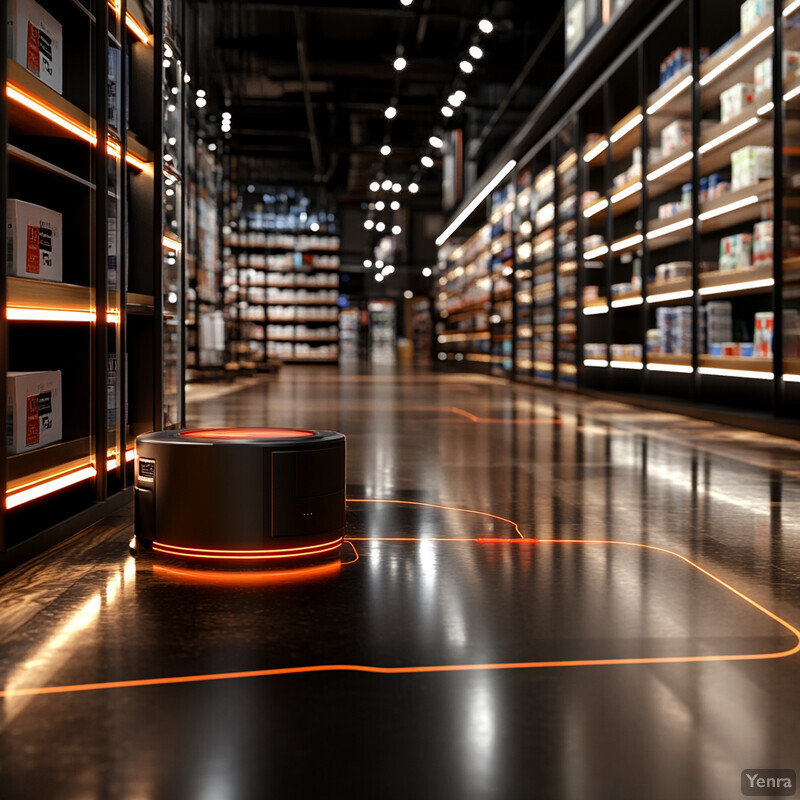
With the help of computer vision and image recognition, AI-powered cameras and sensors can continually survey the shelves, identifying missing or misplaced products as soon as issues arise. These systems compare the current shelf state against the ideal planogram and instantly flag any discrepancies. As a result, staff receive timely alerts to correct errors, fix gaps, or rotate products, guaranteeing that customers encounter consistent and compliant shelf presentations. This real-time auditing minimizes lost sales due to empty spots and maintains brand merchandising standards.
6. Personalized Store Layouts
By leveraging localized demographic and foot-traffic data, AI tailors layouts for each store, ensuring products are displayed where targeted customers are more likely to browse.
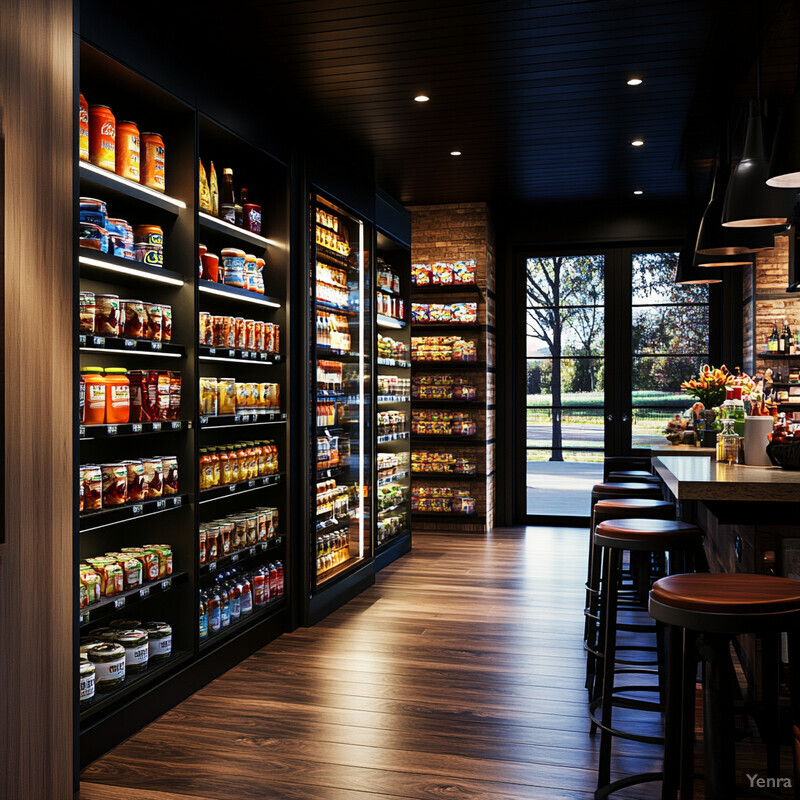
Different stores serve different communities, and AI helps tailor store layouts to these unique demographics. By combining local buying habits, neighborhood income levels, cultural preferences, and foot-traffic data, AI can create store-specific planograms that appeal directly to the local customer base. For example, a store in a university town may highlight quick, affordable snacks near the entrance, while a location in a wealthy suburb might emphasize gourmet or premium brands. This localized optimization increases relevancy, shopper satisfaction, and overall sales performance.
7. Scenario Simulation and Testing
Virtual simulations powered by AI test multiple shelf configurations, predicting outcomes and refining layouts before actual implementation.
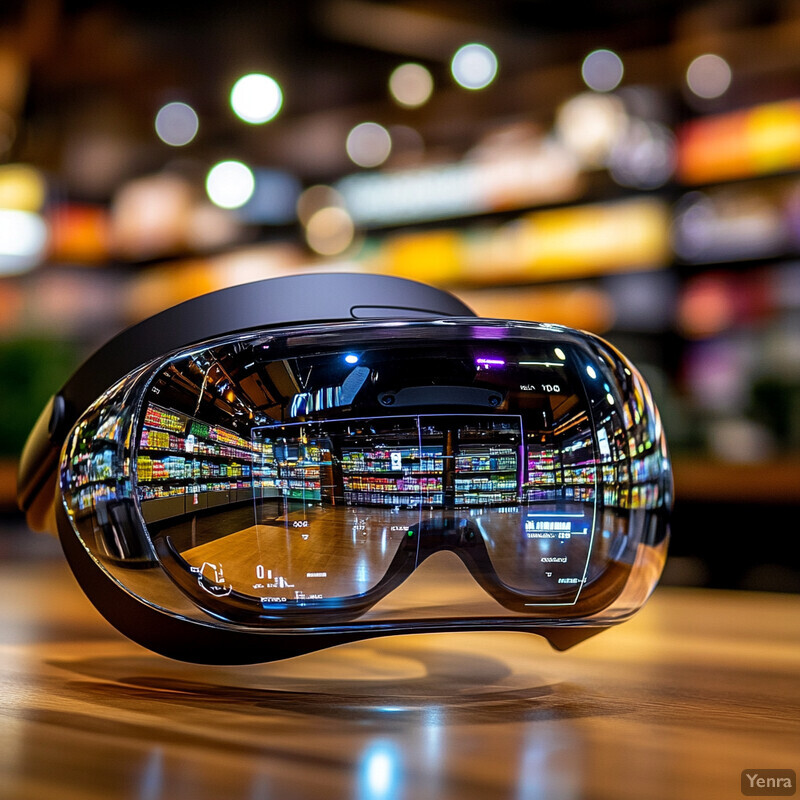
Before committing to a new shelf arrangement, AI enables retailers to run virtual simulations of proposed layouts. Using predictive models, managers can test how rearranging certain items might affect traffic flow, cross-category sales, and overall revenue. By evaluating various 'what-if' scenarios and analyzing the projected outcomes, retailers can adopt the most promising approach without wasting time, labor, or resources. Once implemented, these pre-vetted layouts have a higher likelihood of delivering the desired results.
8. Micro-Merchandising Adjustments
AI identifies subtle opportunities for improvement, such as repositioning related products together or adjusting shelf heights to improve visibility and sales.
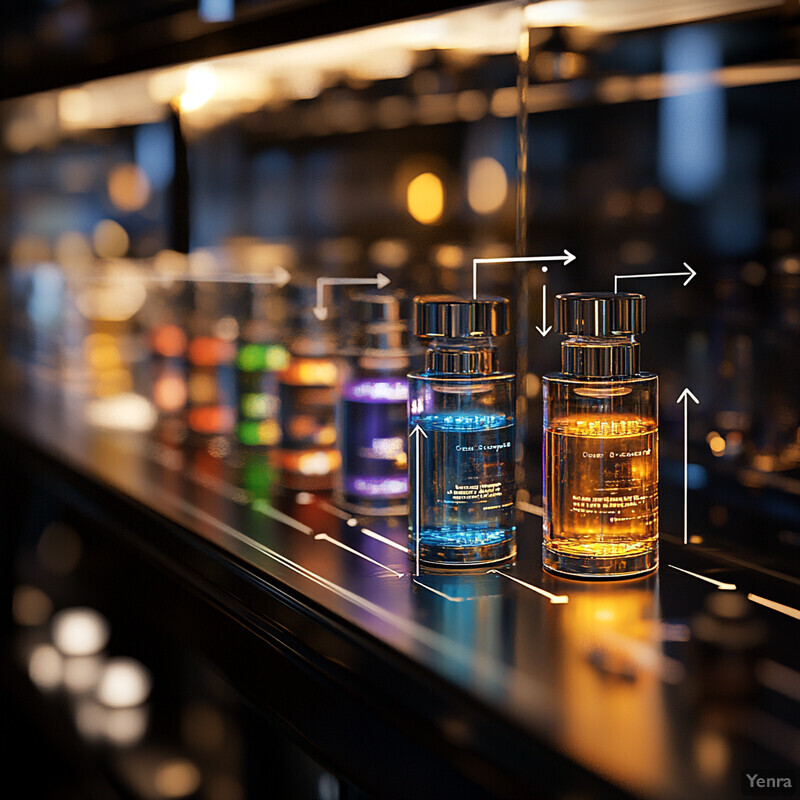
AI detects subtle improvement opportunities that often go unnoticed by the human eye. By carefully analyzing shopper behavior patterns, it can suggest small-scale adjustments, such as placing complementary products closer together, optimizing shelf heights to improve visibility, or clustering items that are frequently bought together. These micro-merchandising tweaks may seem minor, but collectively, they create a more intuitive shopping experience, encourage impulse buys, and incrementally boost revenue while maintaining a polished and shopper-friendly presentation.
9. Integration with IoT and Robotics
Connected shelf sensors and robotic restockers work in tandem with AI to maintain optimal shelf conditions, ensuring timely replenishment and correct placement.
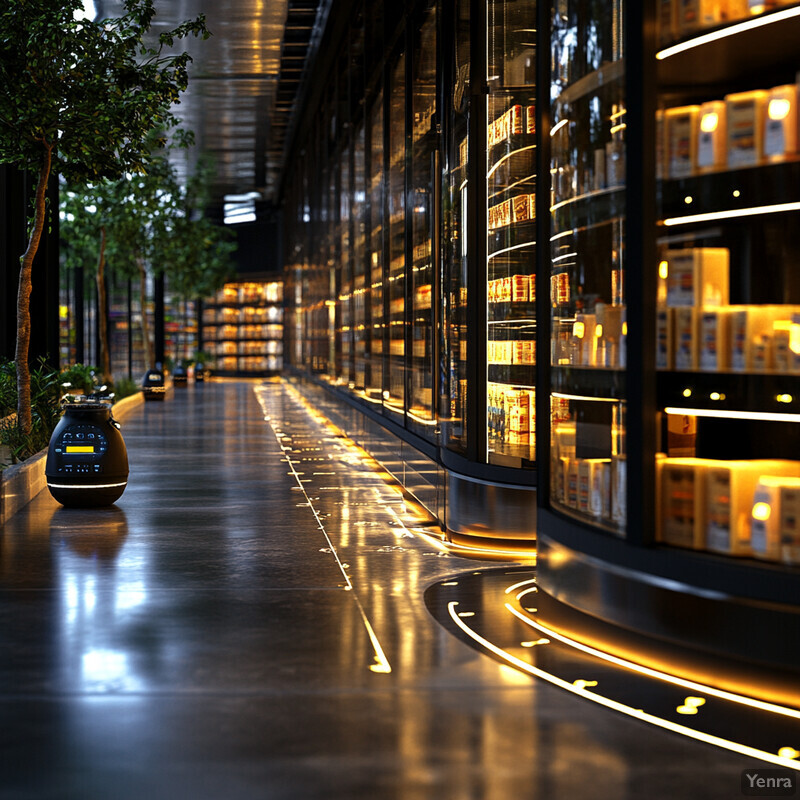
Modern retail shelves are becoming smarter, equipped with sensors that track product weight, inventory levels, and shopper interactions. AI integrates these data streams with robotic restockers or automated shelf facers, ensuring that shelves are always correctly stocked and organized. When a product runs low or is placed incorrectly, connected systems can intervene immediately—either by notifying staff or deploying a robotic unit to correct the issue. This creates a seamless cycle of monitoring and maintenance that improves operational efficiency and enhances the in-store experience.
10. Dynamic Pricing and Promotions
AI engines optimize pricing and promotional product placement based on real-time competitor insights, sales velocity, and inventory levels.
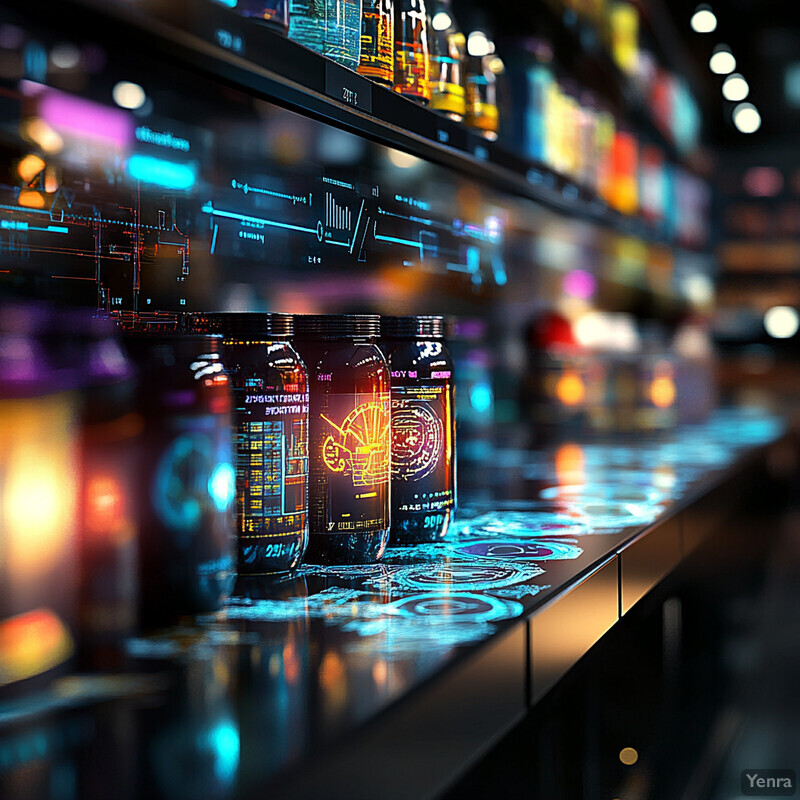
Shelf layout optimization goes beyond product placement. AI can also recommend dynamic pricing and promotional strategies in response to real-time sales data, competitor activity, and inventory levels. For example, if a certain product is overstocked, the system might suggest placing it at eye level along with a timely discount to spur sales. Conversely, hot-selling items might be strategically positioned and priced to maximize profit margins. This highly adaptive approach ensures that the shelf and its messaging respond quickly to market conditions.
11. Enhanced Inventory Management
AI-driven replenishment models align shelf layouts with inventory availability, preventing excess stock from piling up in storage.
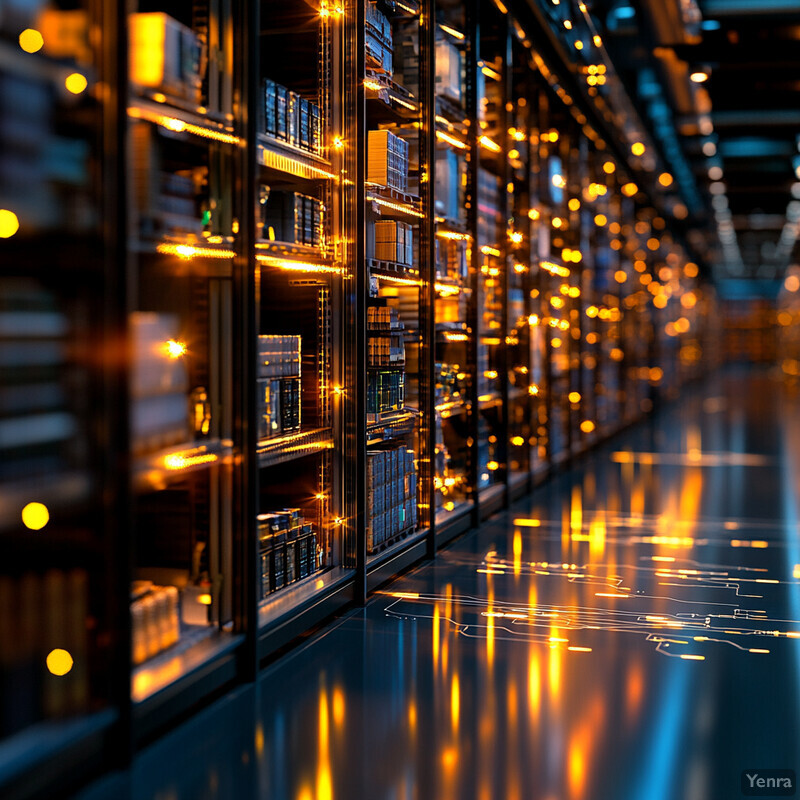
By aligning shelf layouts with intelligent inventory management systems, AI prevents mismatches between what is displayed and what’s stored. These models link planograms directly with warehouse and backroom data, ensuring replenishments align with current sales trends and shelf configurations. The result is a more harmonious relationship between what customers see on the shelf and what is available behind the scenes. Such alignment reduces the need for emergency restocks, cuts carrying costs, and improves the overall efficiency of the supply chain.
12. Shopper Behavior Analysis
By processing data from in-store cameras, AI learns which areas shoppers visit most, adapting layouts to guide them toward high-margin or promotional items.
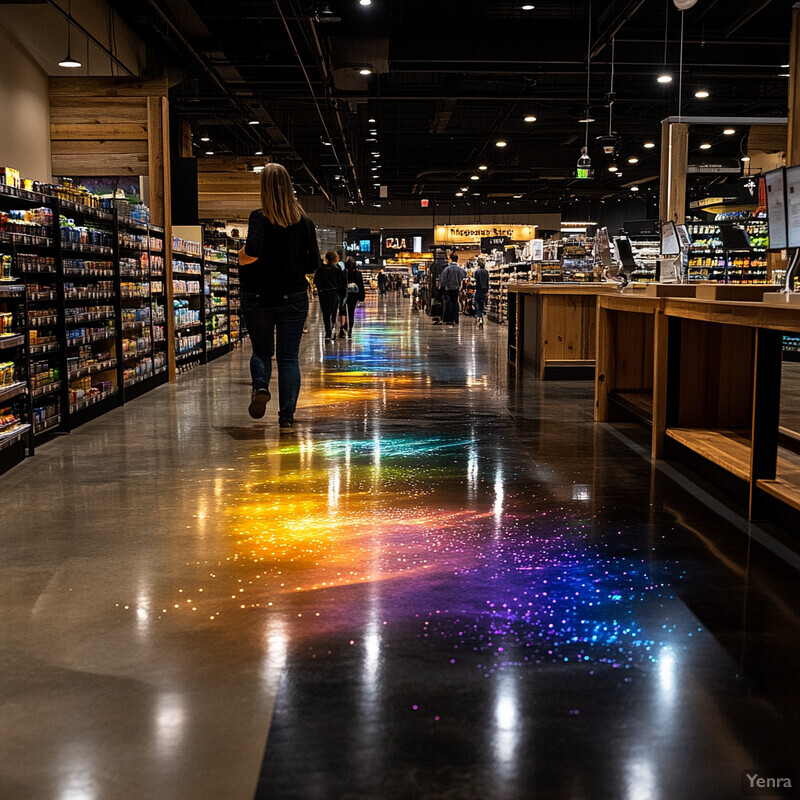
Through in-store cameras and sensors, AI can study how customers move through aisles, where they pause, and what they pick up. By combining this data with machine learning insights, retailers understand which areas receive the most foot traffic, how long shoppers engage with certain products, and what visual cues trigger purchases. Using these learnings, shelves can be rearranged to highlight popular items in high-traffic zones, cluster related products together, or create thematic displays that resonate better with consumer browsing habits.
13. Localized Seasonal Adjustments
Using seasonal and regional data, AI shifts product prominence accordingly—e.g., prime shelf space for grilling supplies in summer or holiday items in winter.
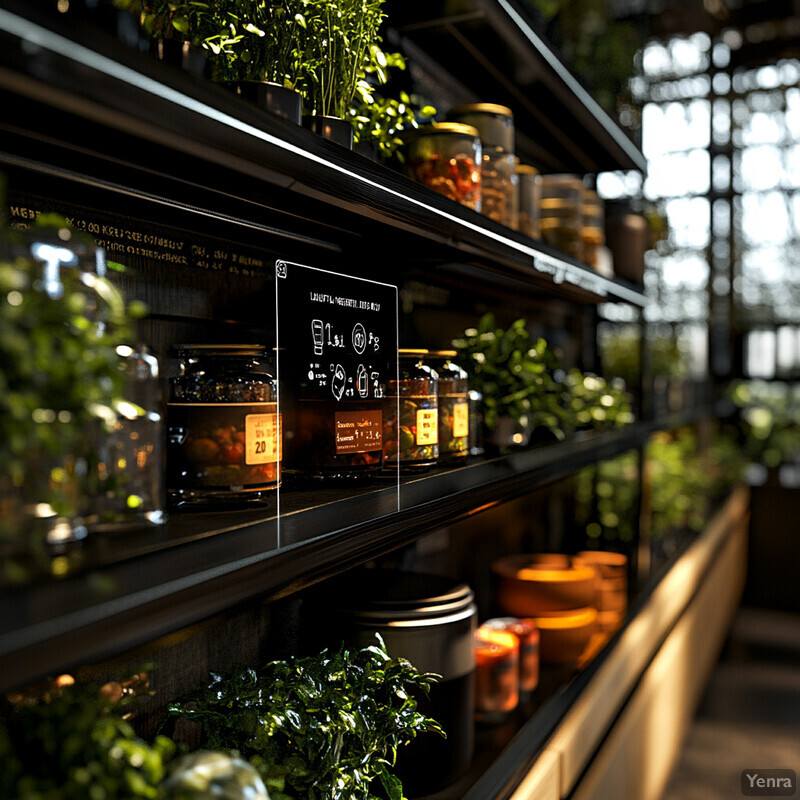
Seasons and holidays heavily influence purchasing behavior, and AI can quickly adapt planograms to reflect these patterns. As weather changes or major holidays approach, the system re-prioritizes products—grilling accessories gain prominence in summer, while baking supplies and festive decorations move to the forefront in winter. By automatically adjusting the planogram to highlight relevant seasonal items, the retailer captures more seasonal sales opportunities, maintains a fresh look, and engages customers with timely offerings.
14. Cross-Category Insights
AI identifies cross-category purchasing patterns, positioning complementary products near each other to increase impulse buys.
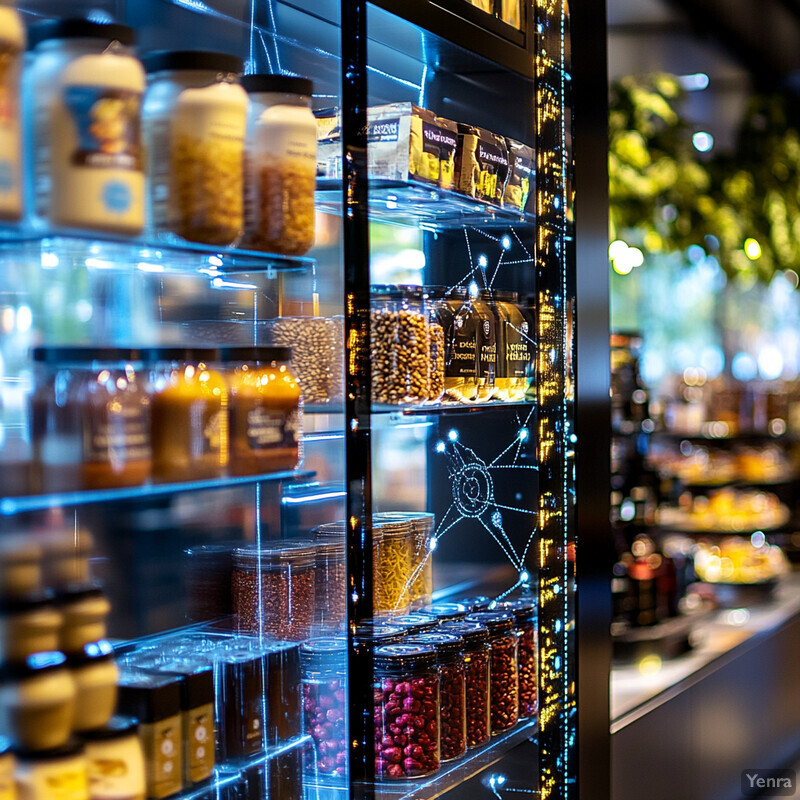
AI doesn’t just optimize shelves within single product categories; it looks across all categories to discover unexpected correlations. For example, data might show that customers who buy a certain brand of coffee also tend to purchase organic granola bars. Recognizing these patterns, AI might suggest placing these products near each other to encourage bundled purchases. This cross-merchandising approach can enhance the shopping experience, prompt impulse buys, and lead to higher overall basket sizes, increasing the retailer’s bottom line.
15. Waste Reduction
By factoring in product shelf life and turnover rates, AI rearranges products to highlight items nearing expiration, reducing spoilage and lost revenue.
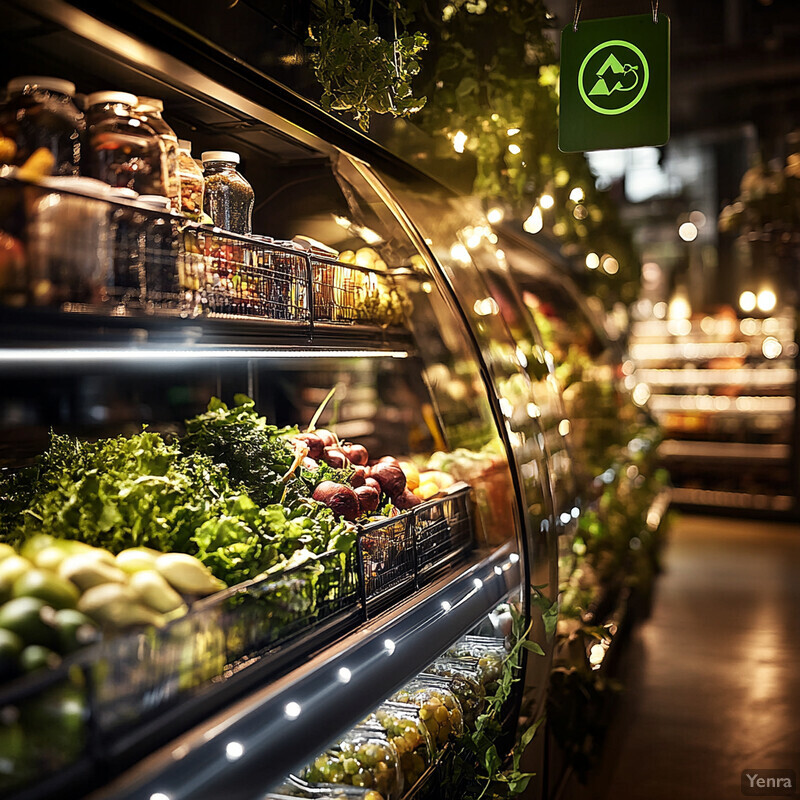
Preventing product waste is a significant goal for both financial and environmental reasons. AI algorithms factor in product shelf life, turnover rates, and expiration patterns when designing shelf layouts. By placing items that have shorter shelf lives in more prominent positions, retailers can move them faster and reduce the likelihood of unsold goods expiring. This not only cuts losses and improves profit margins but also supports sustainability initiatives and resonates with increasingly eco-conscious consumers.
16. Planogram Compliance Monitoring
Natural language processing and image recognition help ensure brand partners’ specifications are met, prompting corrective actions if a layout deviates from agreed-upon standards.
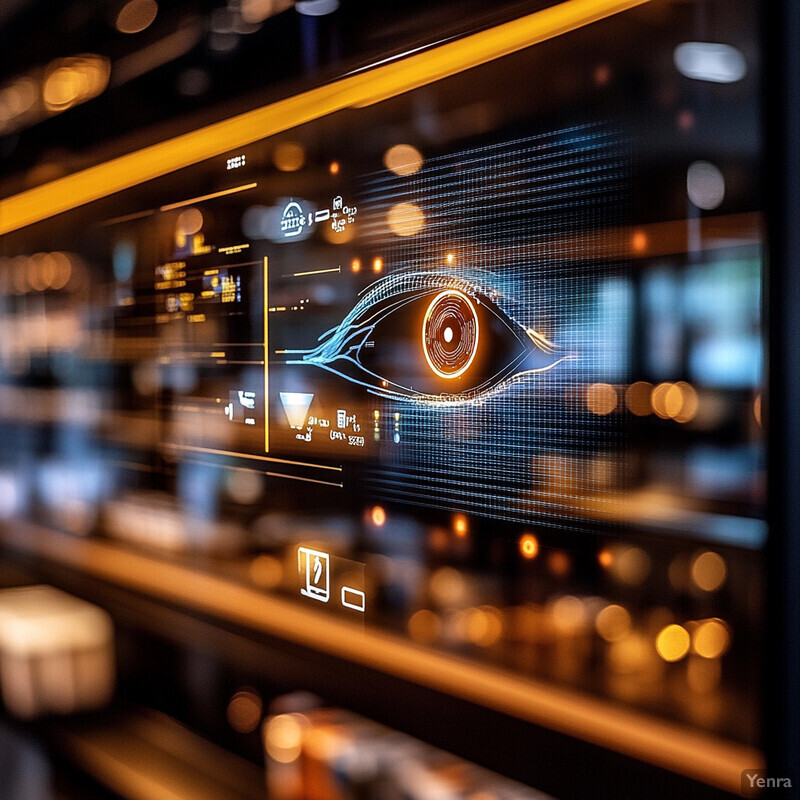
Retailers often have agreements with brands specifying how their products should be displayed. AI systems use image recognition and natural language processing to verify that these planograms are followed to the letter. When discrepancies occur—such as an incorrect product placement or a missing promotional sign—alerts are issued so staff can correct them quickly. Maintaining strict adherence to brand guidelines builds stronger supplier relationships, ensures legal compliance, and preserves the aesthetic integrity of the store.
17. Cost-to-Serve Optimization
AI weighs product handling costs and margins to recommend layouts that minimize operational complexity and maximize profitability.
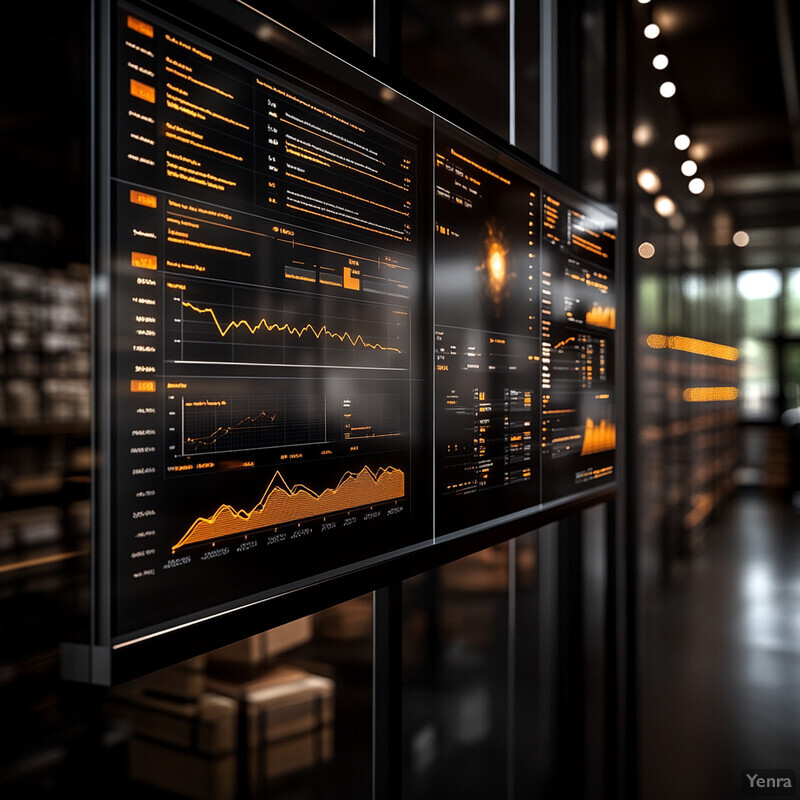
Behind the scenes, handling products involves labor, transportation, storage, and shelf stocking costs. AI solutions evaluate all these factors alongside product margins, using optimization models to prioritize items that minimize total cost-to-serve. By aligning shelf layouts with these efficiency metrics, retailers can simplify restocking schedules, reduce time spent by staff on complicated arrangements, and ultimately improve profitability. Lower operational complexity often translates into more stable pricing and better value for customers.
18. Consumer Sentiment Integration
AI can integrate feedback from social media, reviews, and surveys, reshaping shelf strategy to reflect customer sentiment and preferences.
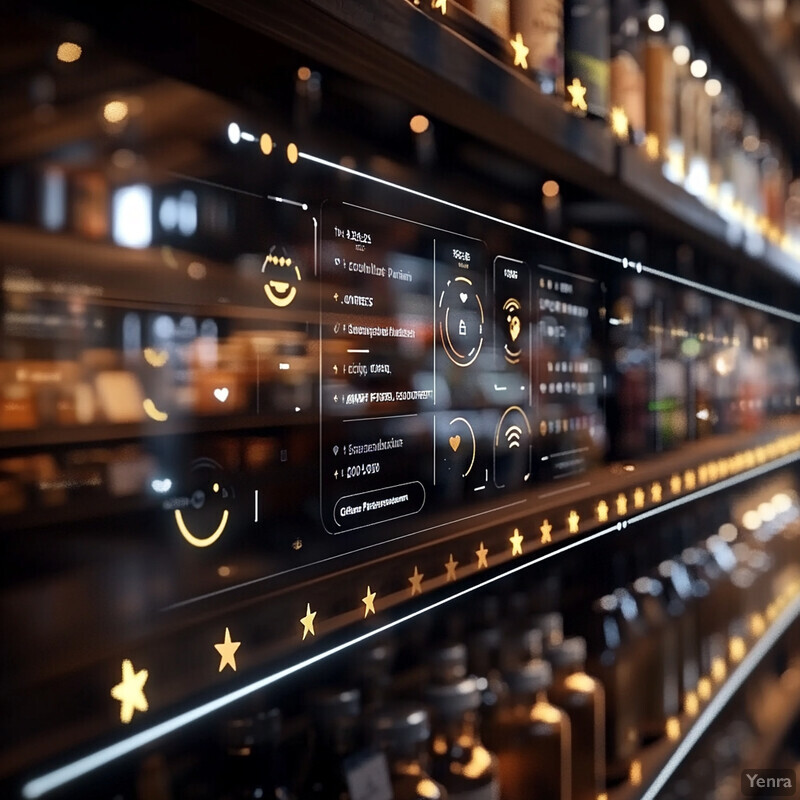
In today’s digital age, customer feedback is abundant. AI can mine product reviews, social media comments, and survey data to gauge consumer sentiment and preferences. If shoppers consistently mention that a certain product is hard to find or that they’d like more variety in a category, the system takes these insights into account when rearranging shelves. By integrating customer feedback directly into the planogram design process, retailers can ensure a shopping environment that aligns more closely with consumer wants and needs.
19. Adaptive Learning Over Time
Machine learning models refine shelf layouts continuously by incorporating feedback loops from sales data, shopper responses, and market trends.
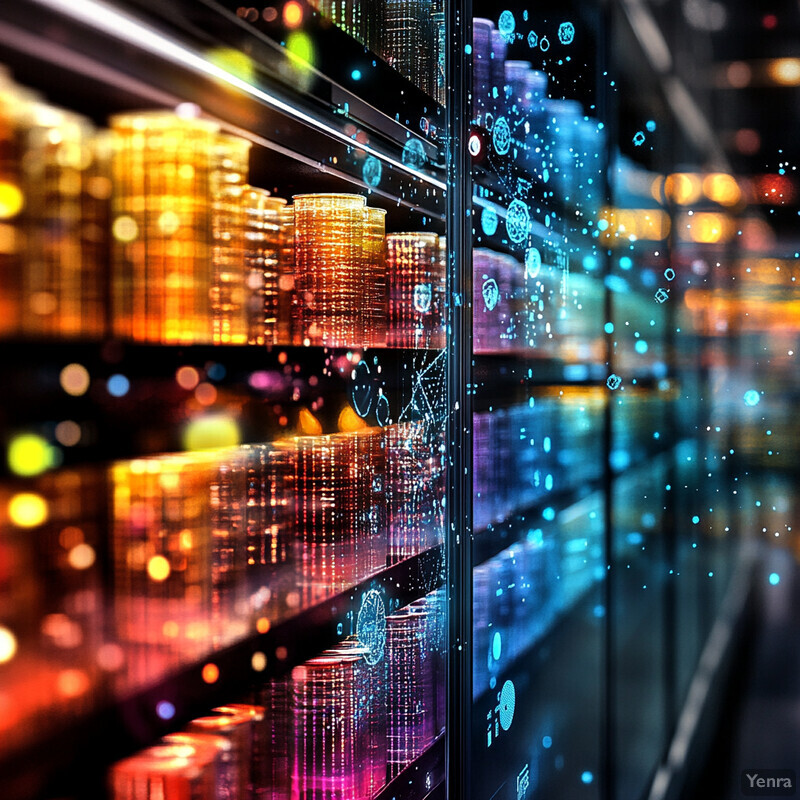
AI systems used for shelf optimization don’t just stop at the initial rollout; they continuously learn and evolve. Every new day of sales data, shopper feedback, and operational metrics helps refine the machine learning models. Over weeks and months, the algorithms grow increasingly adept at making fine-tuned adjustments. This iterative improvement process ensures that shelves remain optimally arranged even as consumer behaviors, market conditions, and business goals evolve.
20. Global-to-Local Scalability
AI allows for consistent best practices across multiple stores while customizing layouts to local market conditions, achieving both brand uniformity and hyper-local relevance.
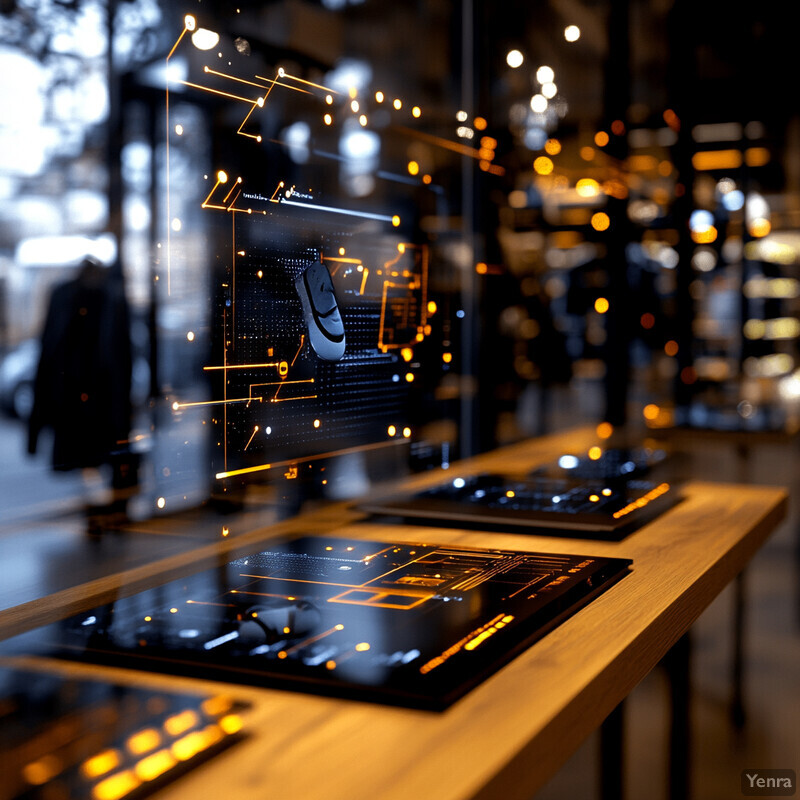
For large retail chains operating in multiple locations, standardizing certain display principles while allowing for local customization can be challenging. AI provides a framework to roll out best practices globally, ensuring brand consistency, while at the same time enabling hyper-local customization. Region-specific planograms take into account local tastes, trends, and economic conditions. This flexible scalability ensures that a global brand image is maintained without neglecting the unique attributes of each store’s community.