1. Granular Demand Forecasting
AI can dissect historical sales data, seasonal patterns, and promotional impacts to accurately predict future demand.
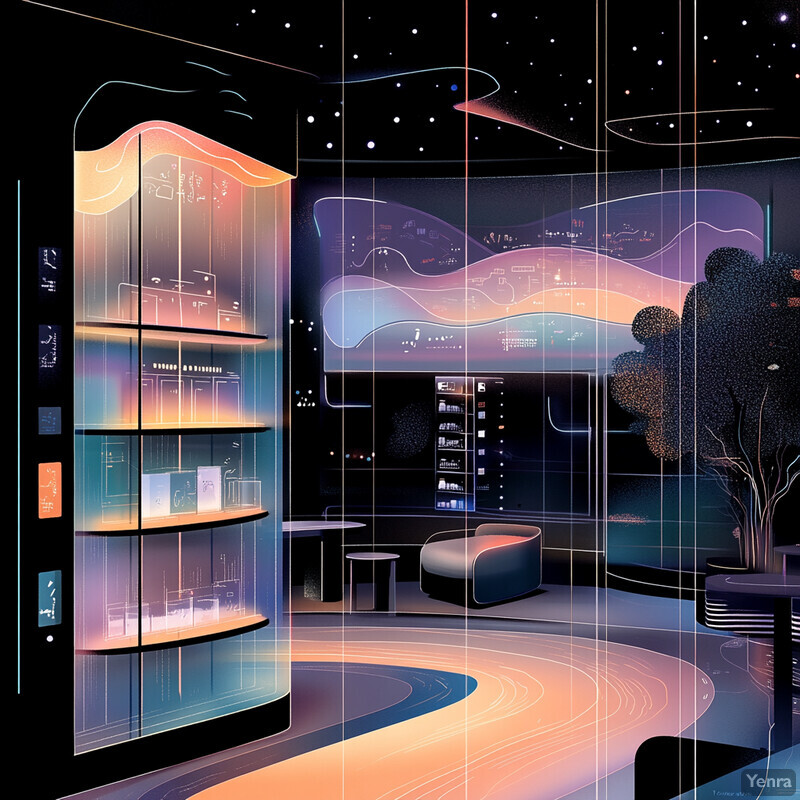
AI-driven demand forecasting uses complex algorithms that go well beyond simple historical averages. By integrating a wide range of factors—including time-based trends, seasonality, macroeconomic indicators, sales histories, and promotional calendars—AI models can predict future consumer demand with high precision. These models also adjust continuously as new data becomes available, learning from shifts in consumer behavior, market events, or emerging trends. The result is more accurate demand predictions that enable retailers to optimize prices proactively, rather than reacting to unforeseen inventory shortfalls or overstocks.
2. Dynamic Pricing Models
Advanced machine learning algorithms empower retailers to dynamically adjust prices based on multiple real-time factors.
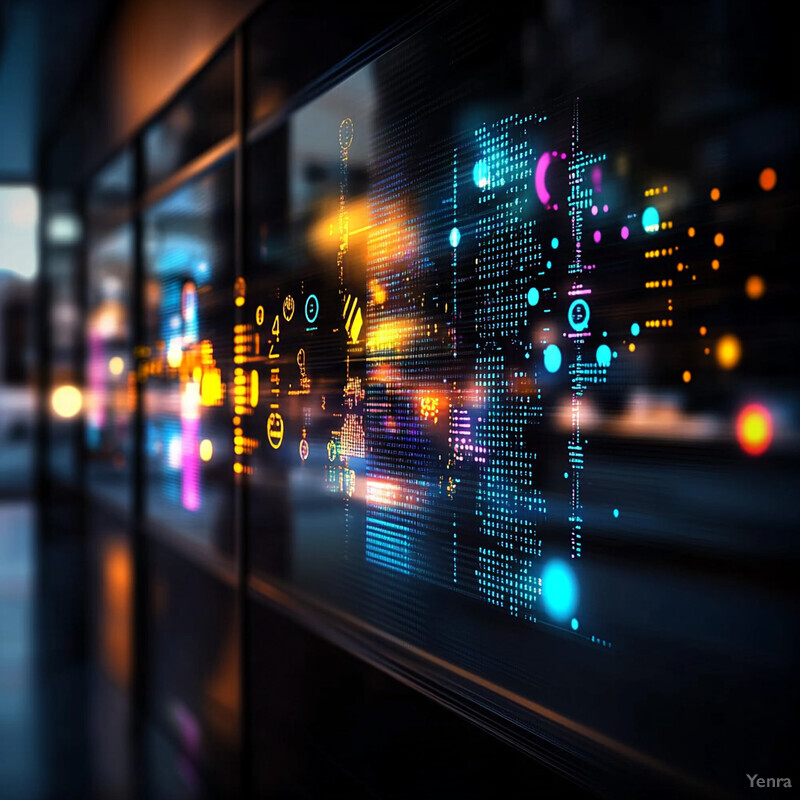
Traditional pricing strategies often involve static or periodically updated price lists that fail to keep pace with rapid market changes. AI-powered dynamic pricing models, on the other hand, continuously evaluate real-time data such as competitor pricing, conversion rates, inventory levels, and even weather conditions. As a result, prices can be adjusted frequently—sometimes multiple times a day—to reflect the current market environment. This agility ensures that retailers can maintain competitiveness, improve margins, and deliver more value to customers by offering the right price at the right time.
3. Real-Time Market Analysis
AI systems constantly scan competitor data and industry sources, providing instant insights for price adjustments.
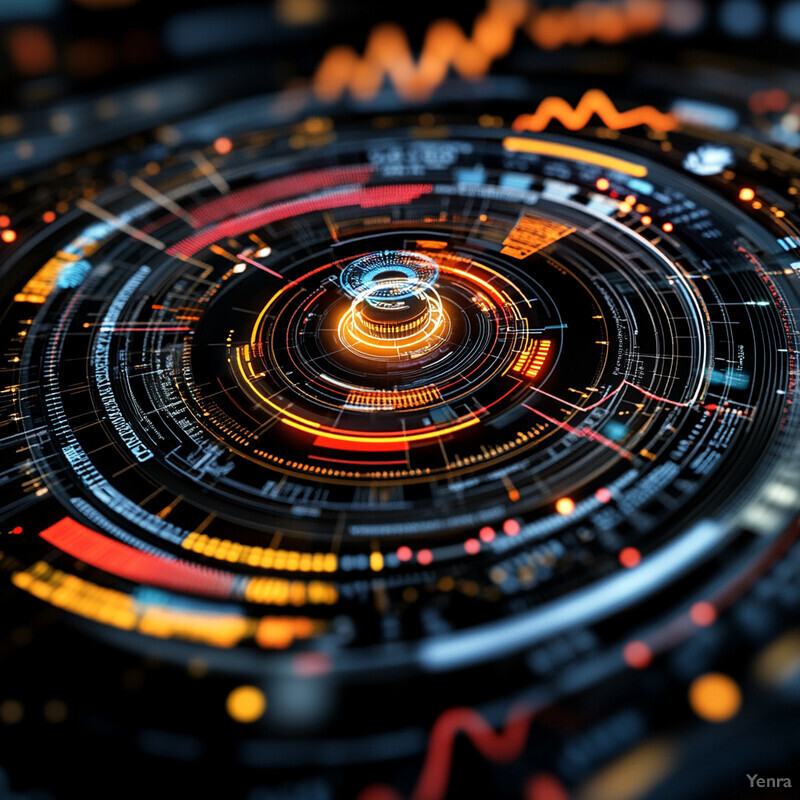
The digital economy moves quickly, and waiting days or weeks for market research to inform pricing is no longer feasible. AI systems provide instantaneous insights by monitoring competitor websites, online marketplaces, and industry data feeds in real time. They track changes in competitor stock levels, promotional activities, and pricing fluctuations, allowing retailers to respond immediately. Rather than relying on historical data or slow human-led analyses, this enables a more dynamic approach, ensuring that pricing remains aligned with current market realities at all times.
4. Automated Price Recommendations
AI-powered tools generate near-instant pricing suggestions, reducing the need for labor-intensive manual calculations.
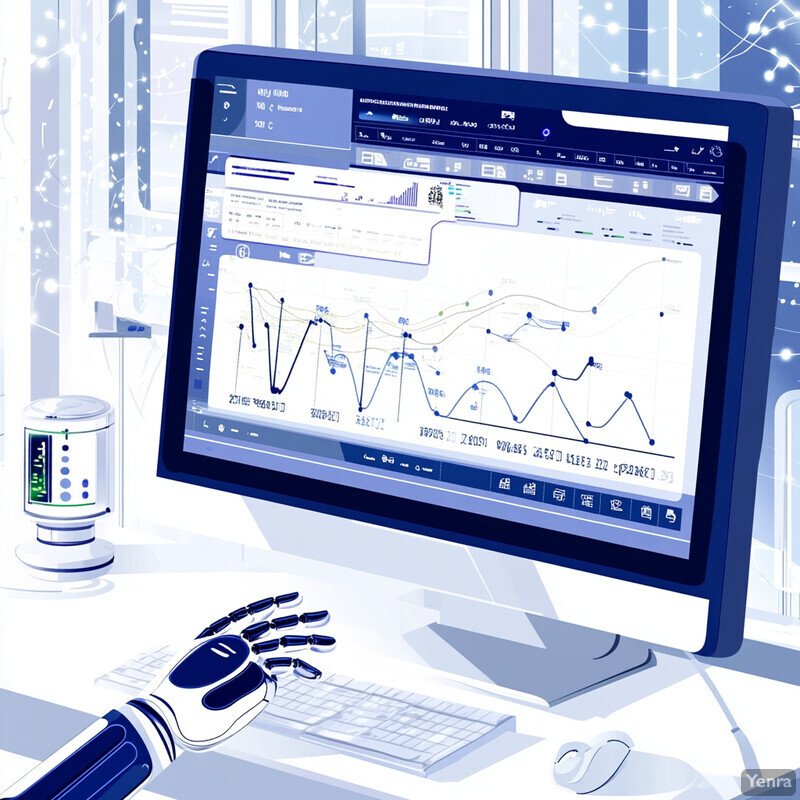
Manual price-setting is error-prone and time-consuming, often involving hours of spreadsheet analysis and gut feel. AI-powered tools streamline this process by automatically generating pricing recommendations based on complex algorithms that weigh costs, target margins, consumer price sensitivity, and past sales performance. These tools can also be integrated into a retailer’s decision workflow, providing easy-to-understand suggestions that can be accepted, modified, or rejected by human analysts. The result is a more efficient, data-driven process that frees up time for strategic thinking while improving pricing accuracy.
5. Optimized Promotions and Discounts
Predictive analytics pinpoint the ideal discount levels and timing, maximizing revenue without eroding margins.
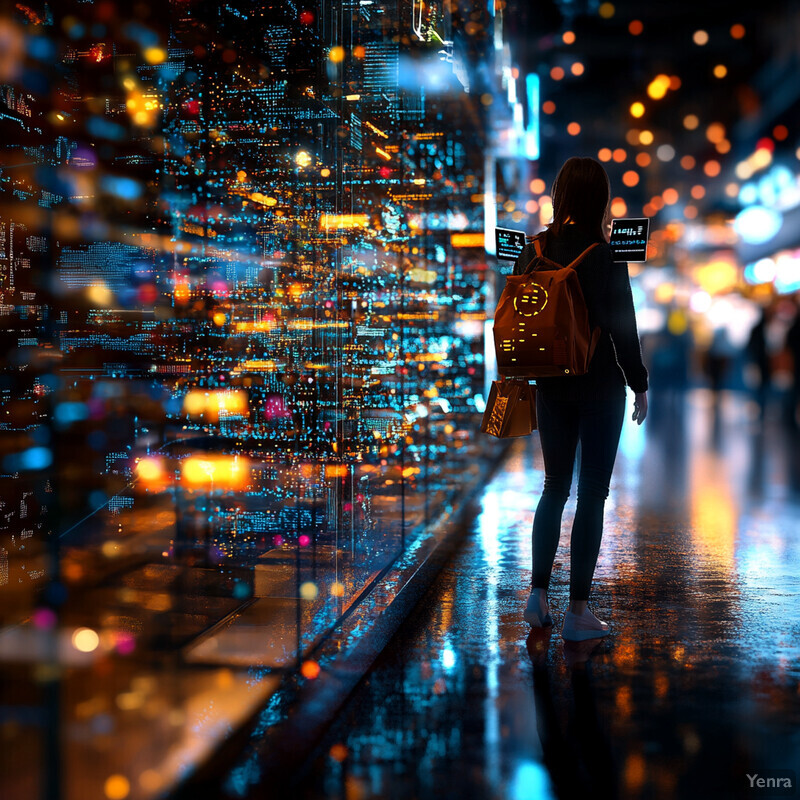
Retailers use promotions to stimulate demand, clear excess inventory, or attract new customers, but unplanned or poorly timed promotions can erode margins. AI solutions help identify which promotions work best under specific conditions, determining the optimal discount level, product mix, and timing. By analyzing historical promotion data and simulating future scenarios, AI can forecast the effect of a sale on both short-term revenue and long-term customer value. This leads to more thoughtful promotional strategies that boost profitability rather than simply driving volume at the expense of margins.
6. Elasticity Modeling
AI can detect how different customer segments respond to price changes, refining strategies based on price sensitivity.
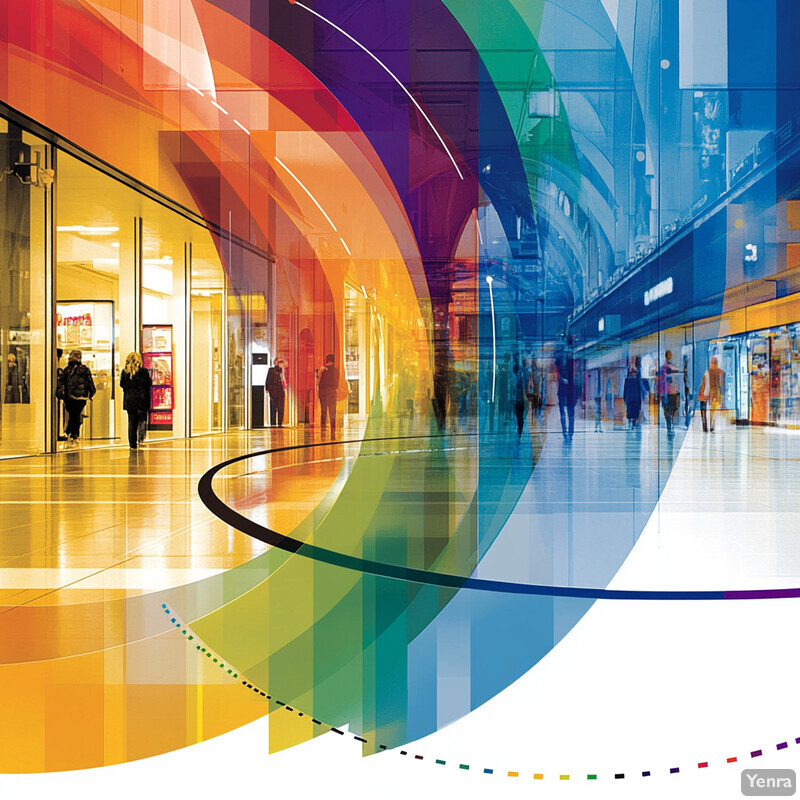
Understanding how demand changes with price adjustments is crucial for effective price optimization. AI excels at modeling price elasticity, using sophisticated statistical and machine learning techniques to measure how different customer segments respond to price changes. The system can distinguish between price-sensitive segments and those willing to pay premium rates, enabling more nuanced pricing approaches. With these insights, retailers can fine-tune prices to maximize profit without alienating customers who might be sensitive to even small increases.
7. Behavior-Based Segmentation
Machine learning algorithms cluster shoppers by browsing patterns, purchase history, and responsiveness to pricing.
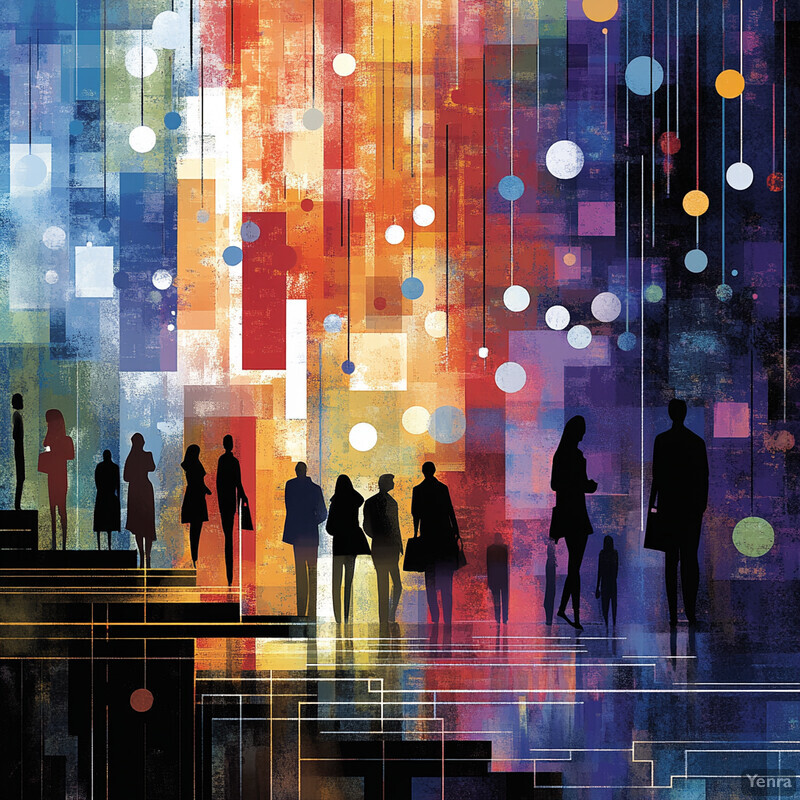
A one-size-fits-all approach to pricing ignores the complex differences in how various customers shop. AI-driven segmentation analyzes browsing behavior, purchase history, and engagement patterns to identify distinct customer groups with unique price sensitivity and buying habits. Retailers can use these insights to offer tailored prices, loyalty rewards, or bundles that resonate with each segment. This personalization not only drives conversion and increases average order value, but also strengthens brand loyalty by delivering more relevant and compelling offers.
8. Multi-Channel Price Consistency
AI ensures unified pricing across brick-and-mortar stores, online platforms, and marketplaces for a seamless brand experience.
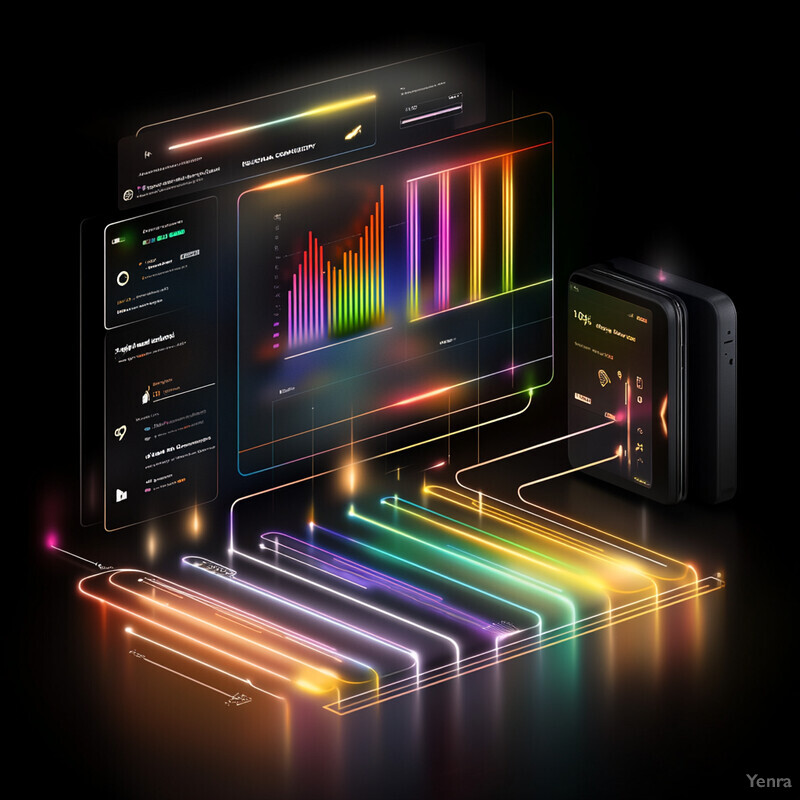
In today’s omnichannel retail landscape, consumers expect consistent experiences and fair pricing across stores, websites, mobile apps, and online marketplaces. AI tools help align pricing strategies across all these channels by factoring in channel-specific costs, consumer behavior, and competitive dynamics. By ensuring that prices are coherent and strategically balanced, retailers can maintain brand integrity and prevent confusion or dissatisfaction. The end result is a unified brand presence, better customer trust, and more stable margins across all touchpoints.
9. Automated A/B Testing of Price Points
Machine learning platforms quickly evaluate multiple price variants, discovering which drives optimal conversions and profit.
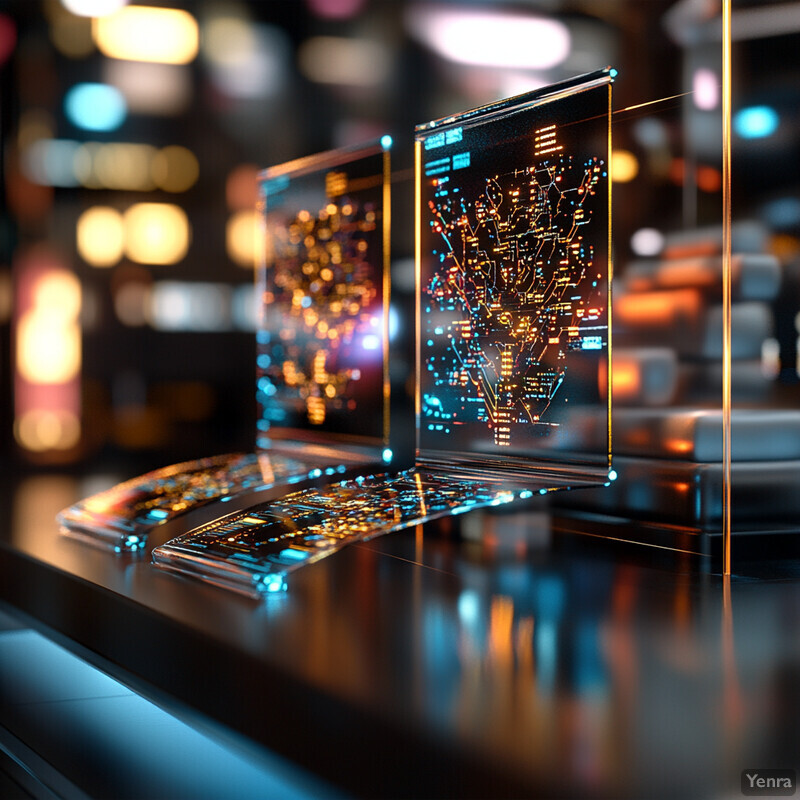
Experimentation is at the core of continuous improvement. AI-driven price optimization platforms support automated A/B or multivariate testing, allowing retailers to experiment with multiple price points or promotional offers simultaneously. These tests run in real time and quickly identify which price structures lead to better conversion rates, higher revenues, or stronger profitability. The speed and scale at which AI can run these tests far surpass traditional manual approaches, enabling retailers to optimize prices much faster and with greater confidence.
10. Efficient Cost Modeling
AI-based systems track supply chain and logistics data to keep prices profitable even when costs shift.
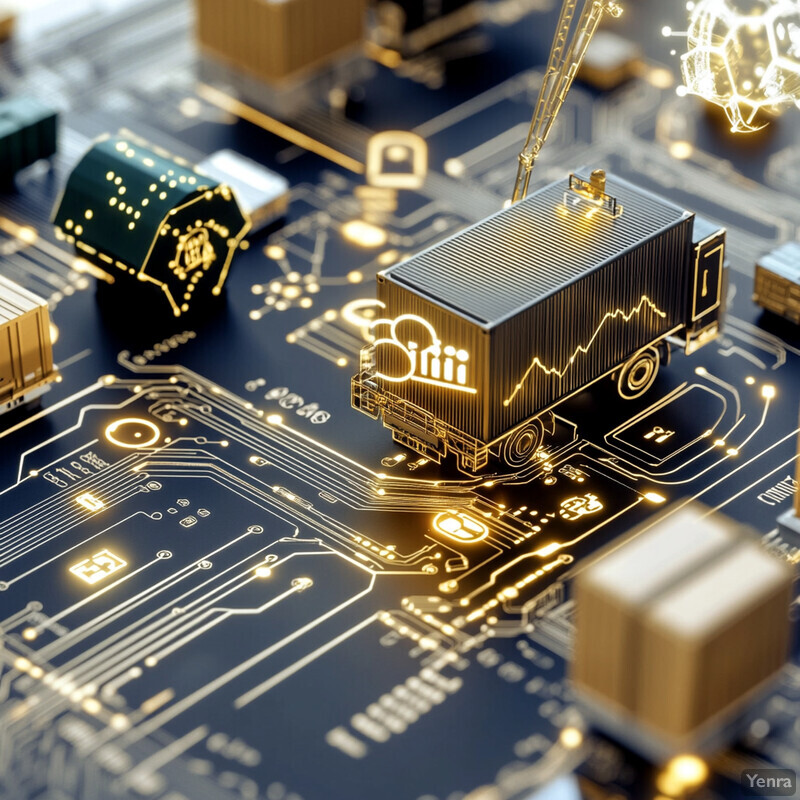
Price setting is not only about revenue; it must also incorporate accurate cost structures. AI can integrate with supply chain and ERP data systems to track changes in raw material costs, labor expenses, shipping rates, and currency fluctuations. By maintaining a real-time view of cost dynamics, the system ensures that recommended prices always preserve desired profit margins. This holistic approach gives retailers better visibility into profitability and prevents them from selling products at unsustainable or loss-inducing price points.
11. Personalized Price Offers to Loyal Customers
By analyzing loyalty data, AI tailors special prices for top customers without compromising profitability.
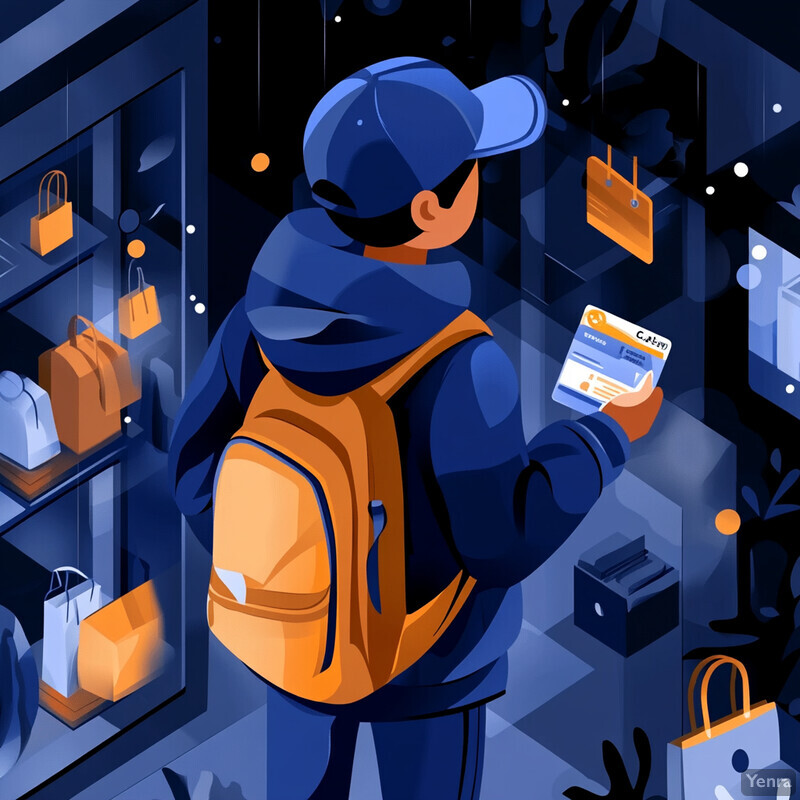
Loyalty programs are designed to reward a retailer’s best customers, but it can be challenging to determine which offers are most effective. AI helps by analyzing transactional data, reward redemption patterns, and historical spending. With these insights, it can recommend personalized discounts, targeted promotions, or early access to new products for valuable customers. Such tailored incentives drive repeat business, improve customer satisfaction, and ultimately increase the lifetime value of the retailer’s most loyal clientele.
12. Competitor Price Prediction
ML algorithms forecast competitor promotions and price trends, allowing retailers to stay a step ahead.
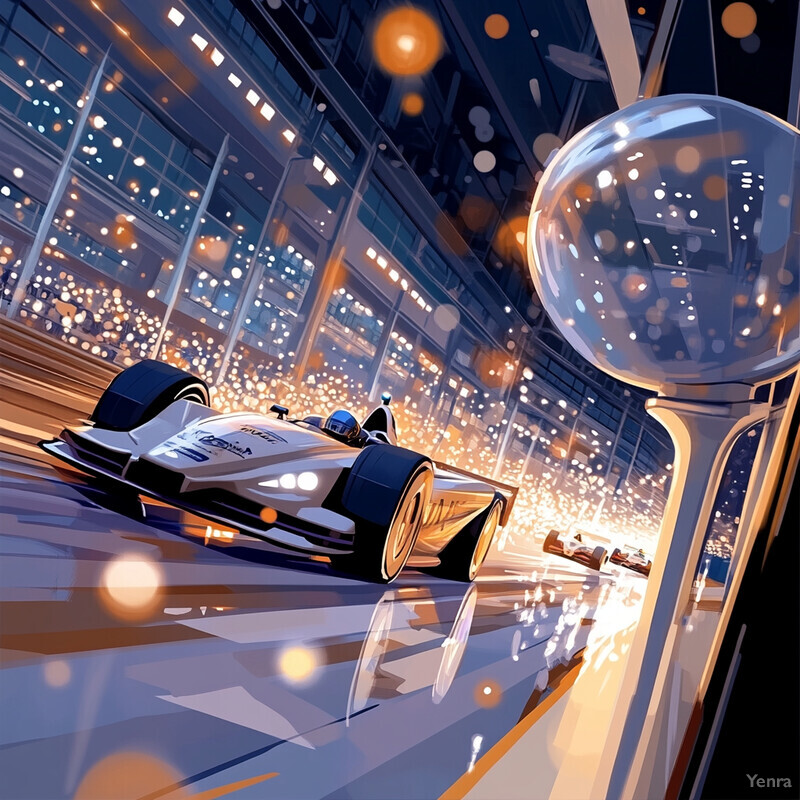
Staying one step ahead of competitors is a key advantage in a crowded marketplace. AI models can forecast competitors’ pricing behaviors by studying historical data, market trends, and external signals like economic indicators or public holidays. Predictive insights help retailers preempt rival moves, allowing them to adjust prices before competitors enact their own changes. This forward-looking approach prevents sudden margin cuts and enables more strategic planning, rather than reacting in a panic to unexpected competitor tactics.
13. Fraud and Anomaly Detection in Pricing
Automated monitoring flags unexpected spikes or drops in price, safeguarding both revenue and brand trust.
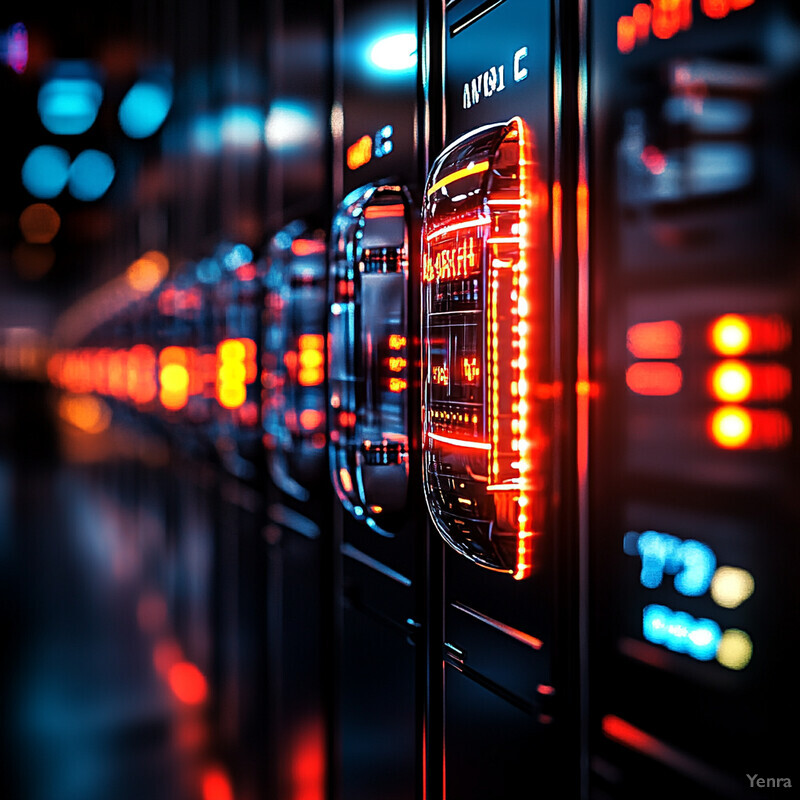
Large retailers manage vast product catalogs and thousands of price changes daily, which can lead to errors, mispricing, or even malicious activities. AI’s pattern recognition capabilities can spot anomalies—such as a sudden drop in price that doesn’t align with demand or a data input error that sets a price far too low. By flagging these issues early, AI helps maintain pricing integrity and reduces the risk of revenue loss or reputational damage. This automated surveillance frees up human analysts to focus on strategic tasks rather than combing through massive data sets for errors.
14. Contextual and Event-Based Pricing
AI adjusts price tags in response to holidays, weather changes, or local events that influence consumer demand.
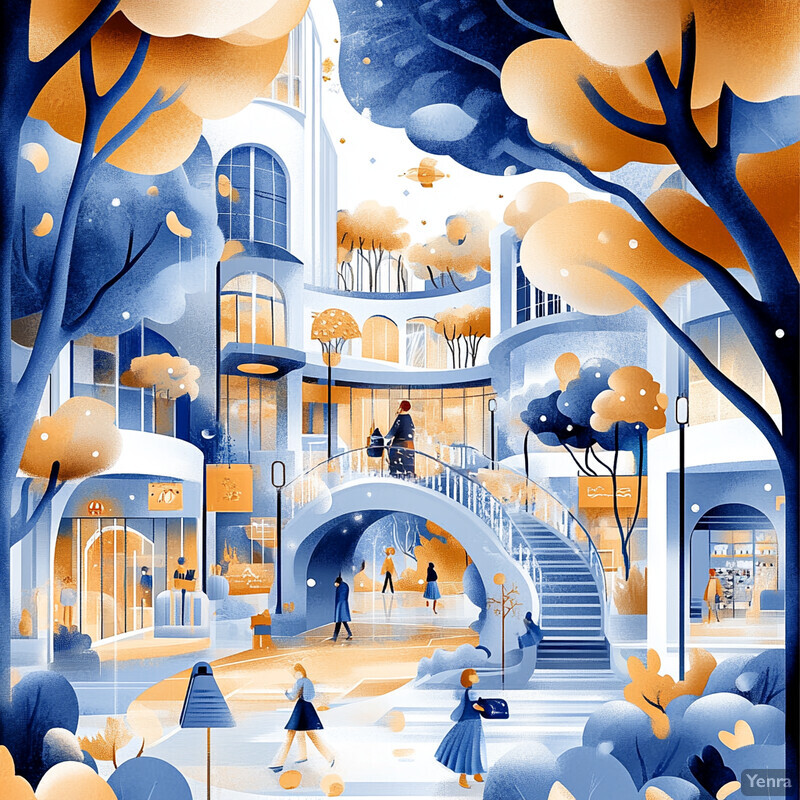
External events and conditions—like sporting championships, weather changes, holidays, and cultural festivals—strongly influence buying behaviors. AI pricing engines can factor in these variables, adjusting prices when a heatwave boosts demand for cooling appliances or lowering prices when a sudden storm deters store visits. By reacting to these contextual cues, retailers can take advantage of temporary opportunities, prevent lost sales due to mismatched pricing, and create a more dynamic and responsive pricing environment.
15. Integration with Inventory Management Systems
Real-time stock data merges with AI-driven pricing, balancing supply and demand on the fly.
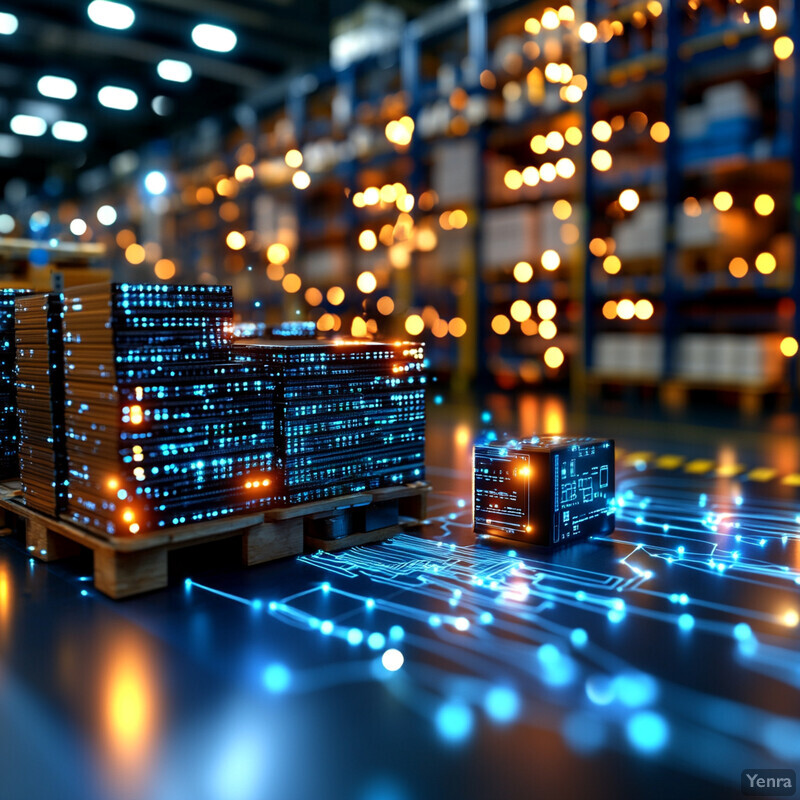
Inventory levels and pricing must work hand in hand to maximize profitability. AI integrates real-time stock data with pricing algorithms, suggesting when to discount slow-moving items or raise prices on scarce products. By balancing supply and demand more precisely, retailers reduce the risk of overstock, minimize costly markdowns, and ensure they don’t leave money on the table for in-demand products. This synergy creates a virtuous cycle in which inventory management informs smarter pricing decisions and vice versa.
16. Lifecycle Pricing Strategies
AI orchestrates prices across a product’s launch, peak, and clearance stages for optimal revenue capture.
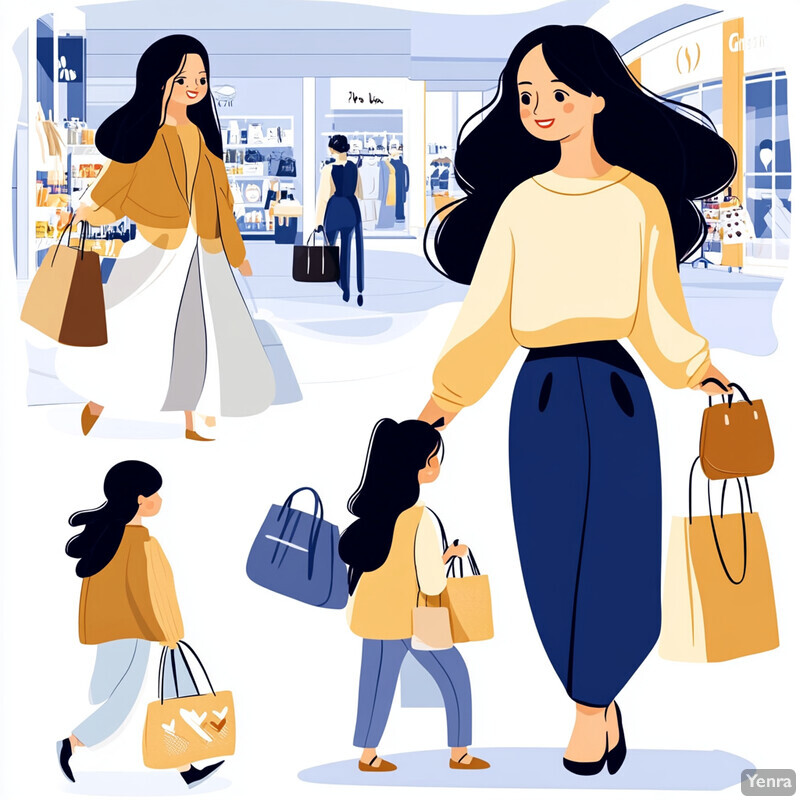
Products have different value trajectories over their lifetimes, from initial introduction to clearance sales. AI can guide retailers through these stages, starting with recommended introductory prices that reflect consumer interest and competition, and adjusting them as the product matures. Toward the end of the product’s life, AI can orchestrate strategic markdowns to clear inventory without severely damaging margins. In doing so, retailers capture optimal revenue at each stage of the product lifecycle and maintain healthier product portfolios.
17. Voice and Visual Commerce Adaptation
Voice shopping and image recognition data inform AI-driven price adjustments to fit each discovery method.
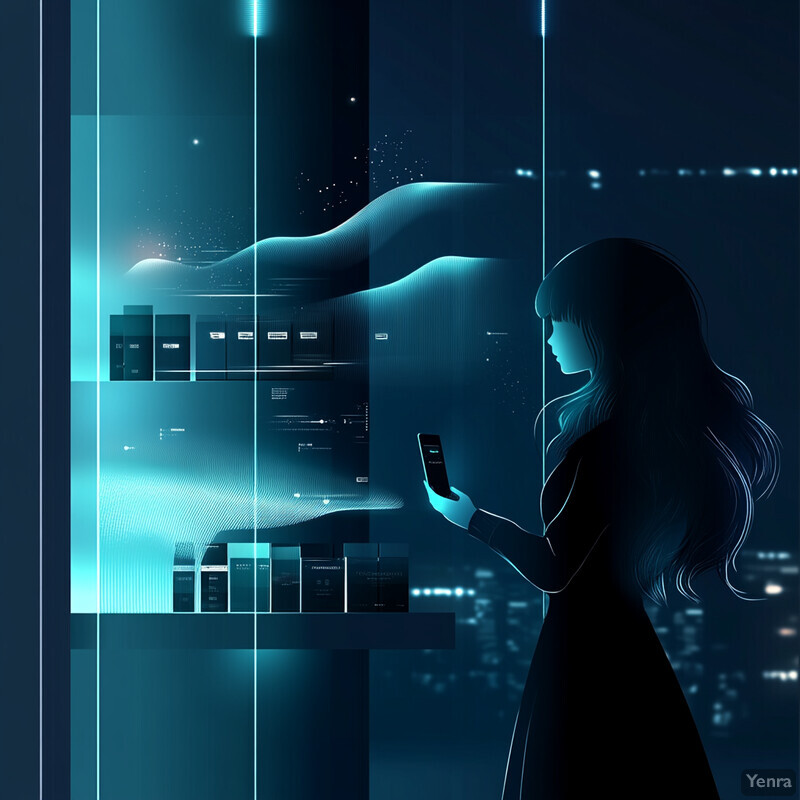
As consumers adopt new shopping methods—such as voice assistants or visual search—retailers must adapt their pricing accordingly. AI can determine whether voice shoppers, who often use quick, low-effort purchasing methods, respond differently to certain price points than customers who conduct visual searches. By correlating shopping channels with price sensitivity, conversion rates, and basket sizes, AI refines price recommendations that fit the unique characteristics of each channel. This ensures that no matter how customers discover products, they encounter a price that encourages conversion.
18. Automated Pricing Governance and Compliance
Embedded rules ensure AI's suggested prices comply with legal, policy, and brand guidelines before going live.
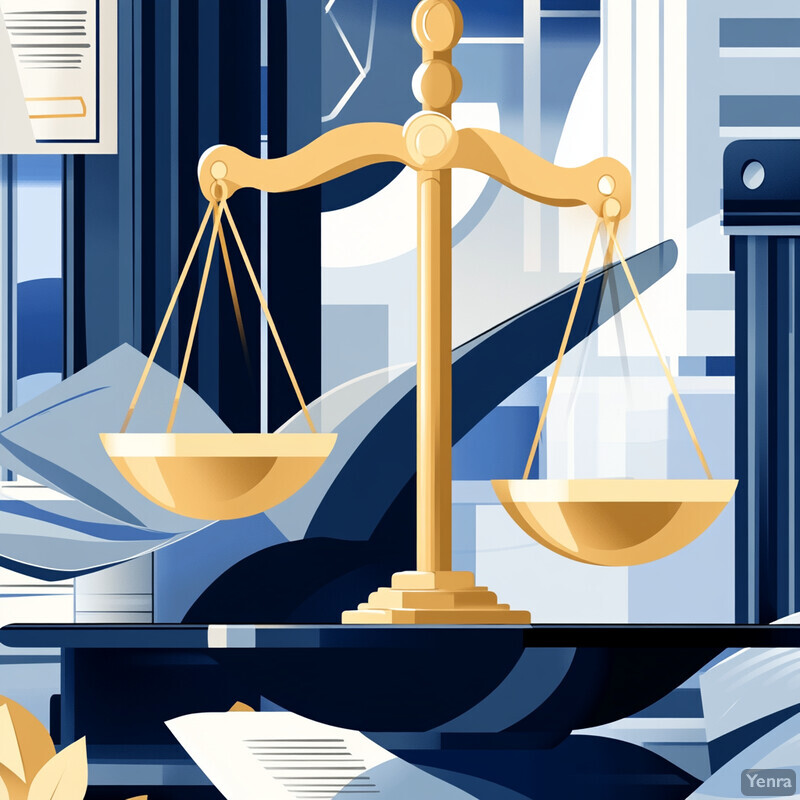
Retail pricing must often align with complex policies such as Minimum Advertised Pricing (MAP) agreements, brand guidelines, and legal constraints. AI can incorporate these rules into its pricing engine, ensuring that all suggested prices meet compliance criteria before they go live. This reduces the risk of legal conflicts, supplier disputes, or reputational harm. By automating compliance checks, retailers reduce manual oversight and streamline the pricing process, allowing them to focus on strategy rather than red tape.
19. Intelligent Bundling and Cross-Selling Strategies
AI identifies products that pair well and calculates bundle prices to increase average order value.
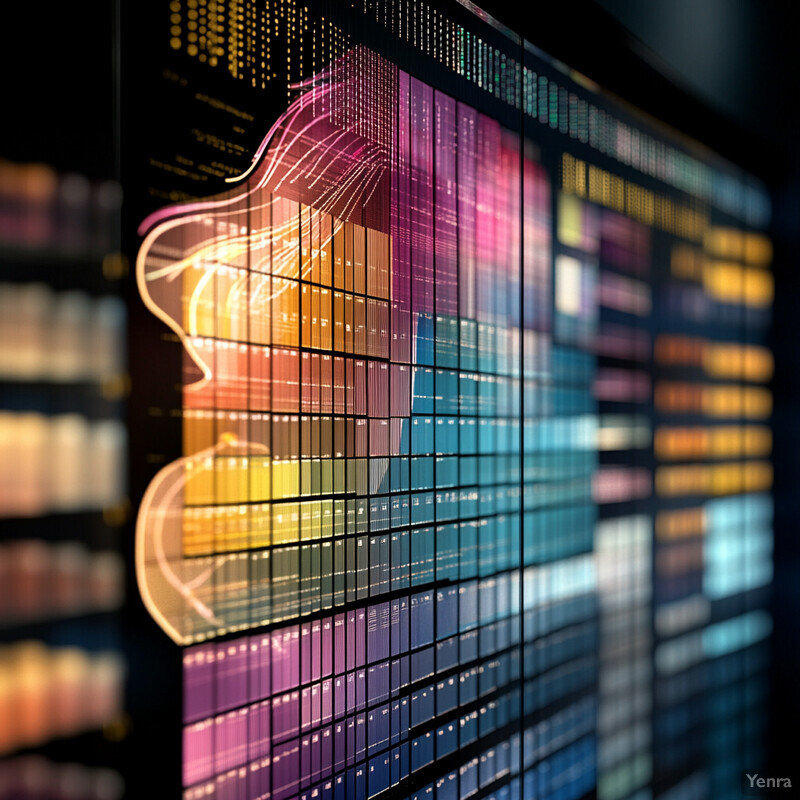
Well-crafted product bundles and cross-sell offers can drive higher order values, but determining the right combination of products and prices is challenging. AI analyzes transaction histories, product affinities, and customer preferences to identify which items complement each other and how to price them as a bundle. By continually testing and refining these offerings, AI ensures that bundles remain appealing and profitable. As a result, retailers increase both their top-line revenue and their customers’ perceived value, driving repeat purchases and customer satisfaction.
20. Continuous Self-Learning and Improvement
AI refines its algorithms with every new data point, constantly enhancing pricing precision.
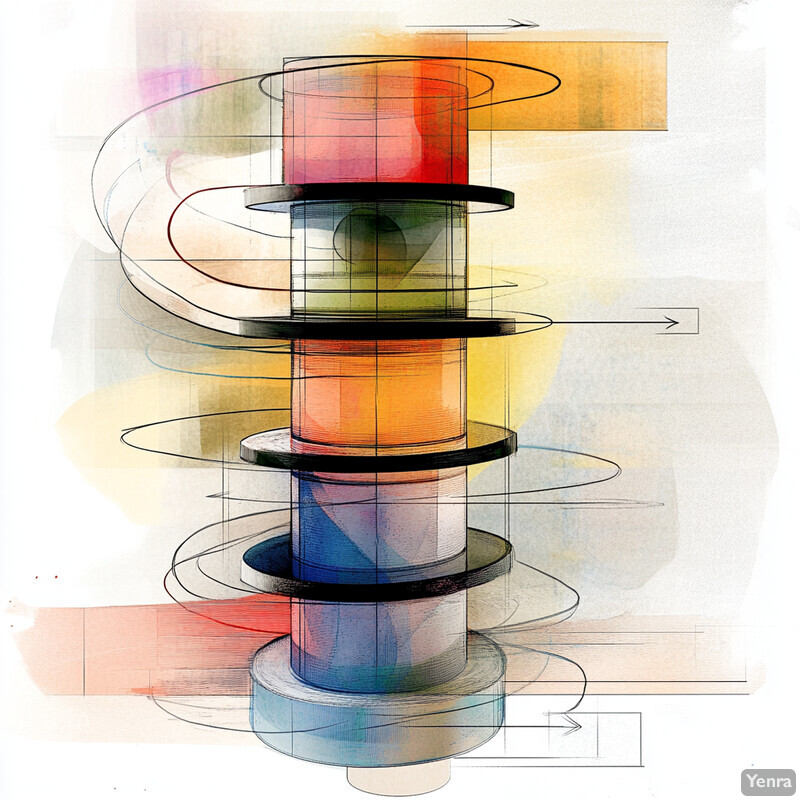
At the core of AI’s transformative power is its ability to learn continuously. Each new data point—be it a sale, a failed promotion, or a competitor’s move—helps AI models refine their parameters, identify emerging patterns, and improve their predictive accuracy. Over time, these iterative improvements lead to more stable margins, more accurate predictions, and pricing strategies that remain effective even as the market evolves. This self-improving cycle ensures that AI-driven price optimization tools become more valuable and reliable the longer they are in use.