1. Enhanced Demand Forecasting Accuracy
AI-driven models leverage machine learning algorithms to improve demand forecasting, reducing errors that often lead to stock-outs or excess inventory.
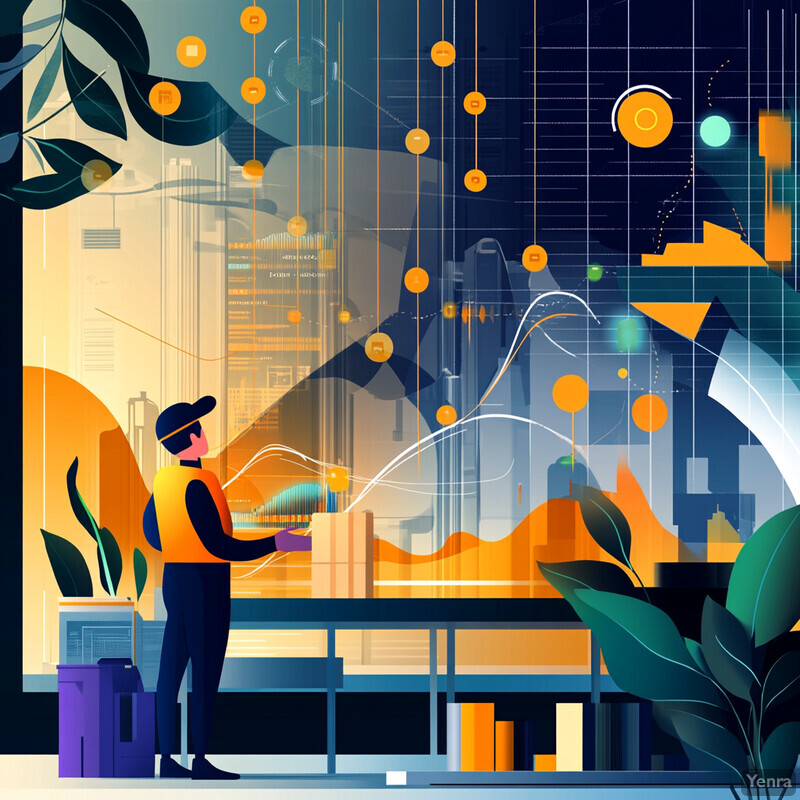
Traditional demand forecasting relies heavily on historical data and trend analysis, which often struggles to adapt when faced with rapidly changing market conditions, shifting consumer preferences, or unforeseen events. AI-driven predictive models, powered by machine learning algorithms, can analyze vast and varied datasets—from transactional sales records to social media discussions and online search trends—to uncover nuanced demand patterns. These models not only improve the accuracy of baseline forecasts but also quickly recalibrate when new data arrives. As a result, organizations can better match production and inventory levels to anticipated needs, minimize the risk of stock-outs or overstocks, and reduce overall carrying costs while maintaining robust service levels.
2. Real-Time Anomaly Detection
Advanced anomaly detection techniques identify irregularities in supply chain operations—such as sudden shifts in lead times or unusual purchase patterns—alerting teams to potential disruptions before they escalate.
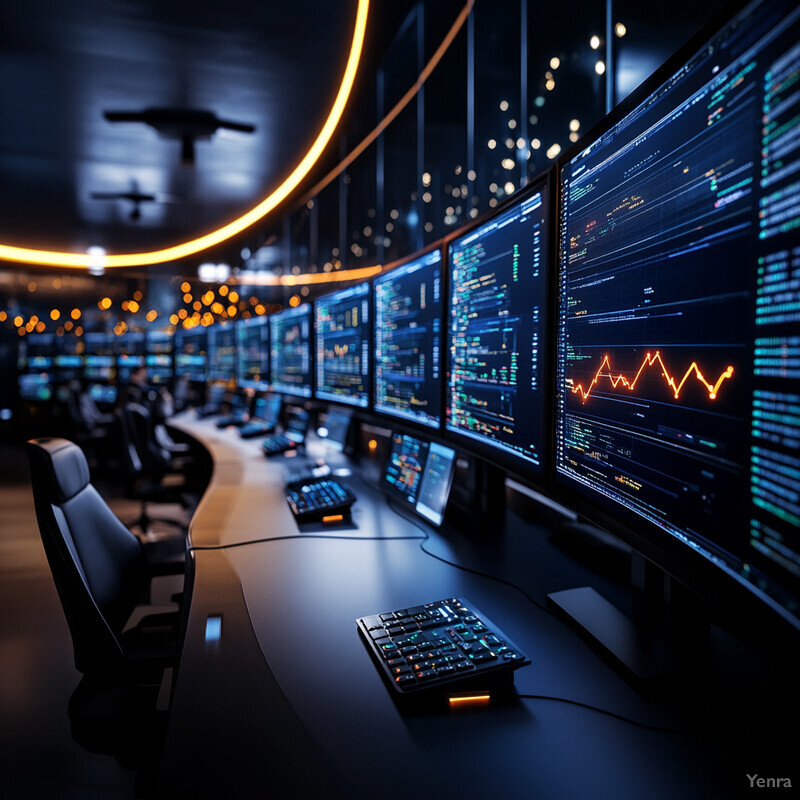
Supply chains are dynamic ecosystems, and even small deviations—unexpected spikes in lead times, unusual ordering patterns, or delayed supplier responses—can cascade into larger disruptions. AI-based anomaly detection tools scrutinize streams of operational data in real time, identifying irregularities as soon as they occur. These tools use machine learning to learn what constitutes ‘normal’ operations and then instantly flag deviations for further investigation. By catching and diagnosing these anomalies early, supply chain managers can intervene proactively, preventing minor issues from escalating into major problems that could halt production lines or disappoint customers.
3. Dynamic Supplier Risk Assessments
AI can continuously evaluate supplier performance and reliability, integrating data on financial health, delivery history, and compliance, enabling proactive risk mitigation.
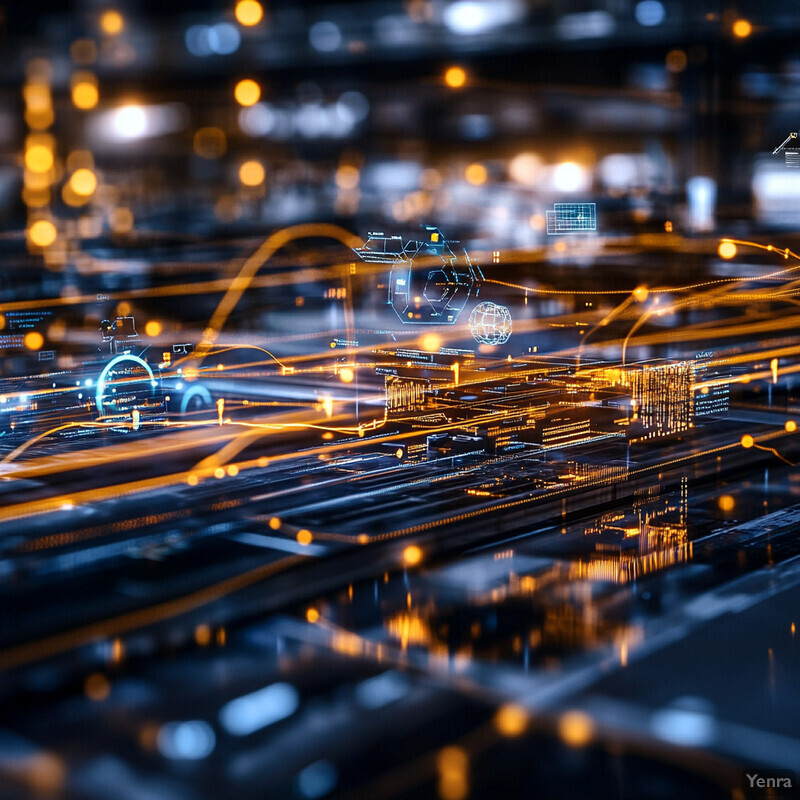
Supplier performance and reliability can shift over time due to factors like financial instability, labor unrest, regulatory changes, or capacity constraints. AI-powered risk assessment models continuously aggregate and analyze a wide range of indicators—supplier financial health reports, quality inspection results, past delivery timeliness, and compliance scores—to create a constantly updated risk profile of each vendor. Instead of relying on static, periodic evaluations, these models provide real-time insight into how supplier risk is evolving. This dynamic approach enables procurement and supply chain professionals to anticipate supplier failures or constraints well before they impact production, allowing for strategic diversification, renegotiation, or phased supplier replacement.
4. Early Warning Signals from External Data
Natural language processing and sentiment analysis on news, social media, and market reports provide early indicators of events (labor strikes, political instability, transportation disruptions) that could impact the supply chain.
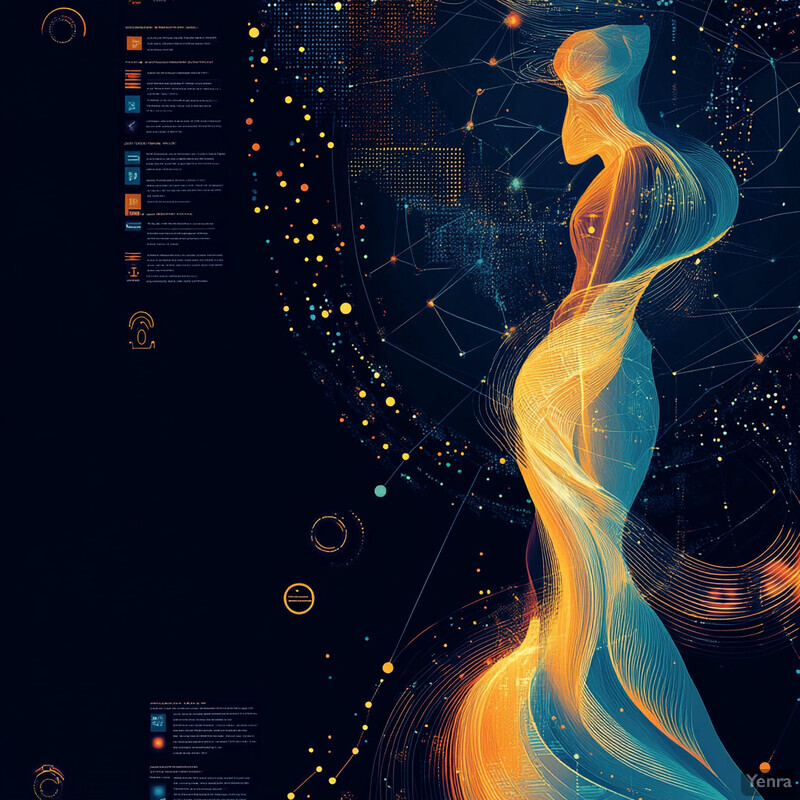
In a globally connected marketplace, disruptions can emerge from sources entirely outside an organization’s direct control, such as geopolitical tensions, natural disasters, or changes in consumer sentiment. AI-driven solutions harness natural language processing and sentiment analysis to sift through news reports, social media chatter, trade journals, and government bulletins. By detecting linguistic patterns and keywords that signal emerging threats—such as impending labor strikes, sudden regulatory actions, or critical port closures—these models offer early warnings. With this intelligence, companies can adjust their logistics strategies, re-route shipments, build up safety stock, or engage alternative suppliers before the risk materializes fully, enhancing resilience and reducing response times.
5. Predictive Maintenance of Logistics Assets
Machine learning models can predict equipment failures in transportation fleets, warehouses, and production lines, reducing downtime and maintaining throughput during peak demand periods.
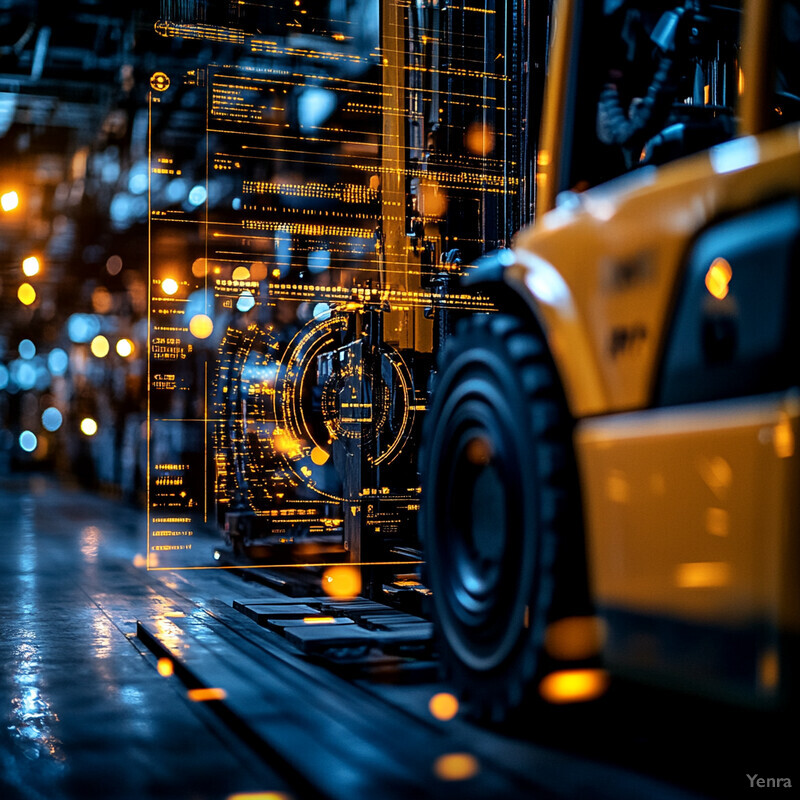
Logistics operations depend on the reliability of assets like trucks, forklifts, conveyor belts, and automated picking machines. Unexpected breakdowns can halt supply chain flow, delaying deliveries and increasing costs. AI-powered predictive maintenance models ingest data from sensors and machine logs—temperature readings, vibration measurements, fluid analyses—and apply machine learning to identify patterns that correlate with impending failures. By predicting when equipment is likely to need service, these models empower maintenance teams to schedule repairs at optimal times, reducing unplanned downtime and extending asset lifespans. Ultimately, this approach ensures steady throughput, improved reliability, and more predictable logistics performance.
6. Weather and Climate Impact Modeling
AI-driven predictive models incorporate meteorological data to foresee weather-related disruptions—storms, floods, hurricanes—that may delay shipments or damage goods.
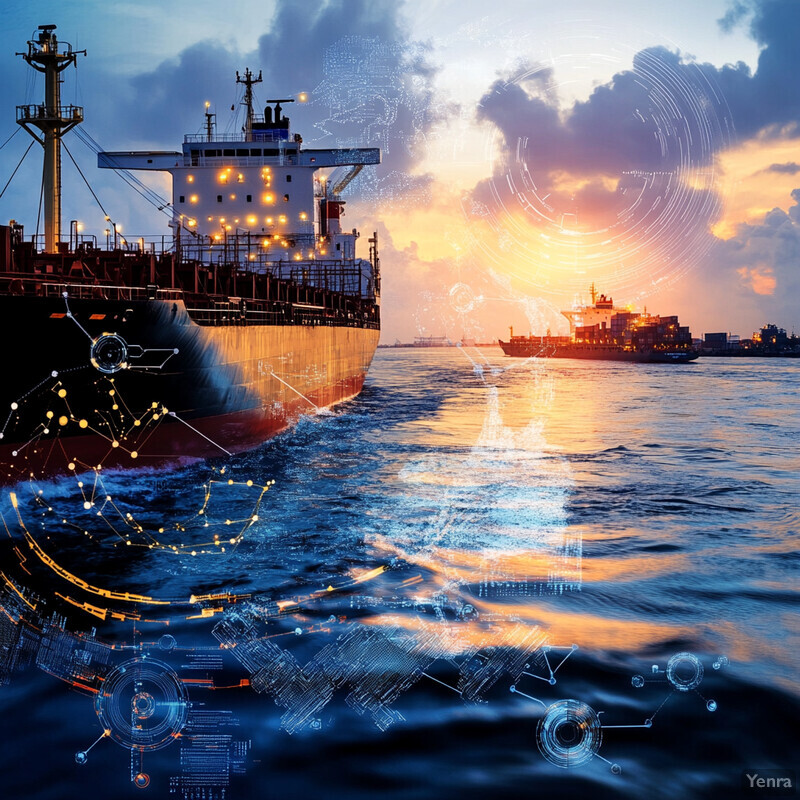
Extreme weather events and climate-related disruptions can cripple transportation networks, damage infrastructure, and alter supply and demand patterns. AI-based predictive models integrate detailed meteorological forecasts, historical weather data, and climate change projections into supply chain planning. Using advanced analytics, these systems correlate weather conditions with past shipping delays, damage claims, or performance downgrades. As a result, organizations gain foresight into how weather trends may affect future shipments, enabling them to preemptively redirect freight, reschedule pickups, reinforce packaging, or adjust production and distribution timelines. This level of preparedness helps safeguard inventory, maintain service levels, and reduce financial losses.
7. Geopolitical Risk Monitoring
Advanced analytics track geopolitical events and regulatory changes (tariffs, embargoes, local compliance shifts), helping supply chain managers anticipate and navigate emerging risks.
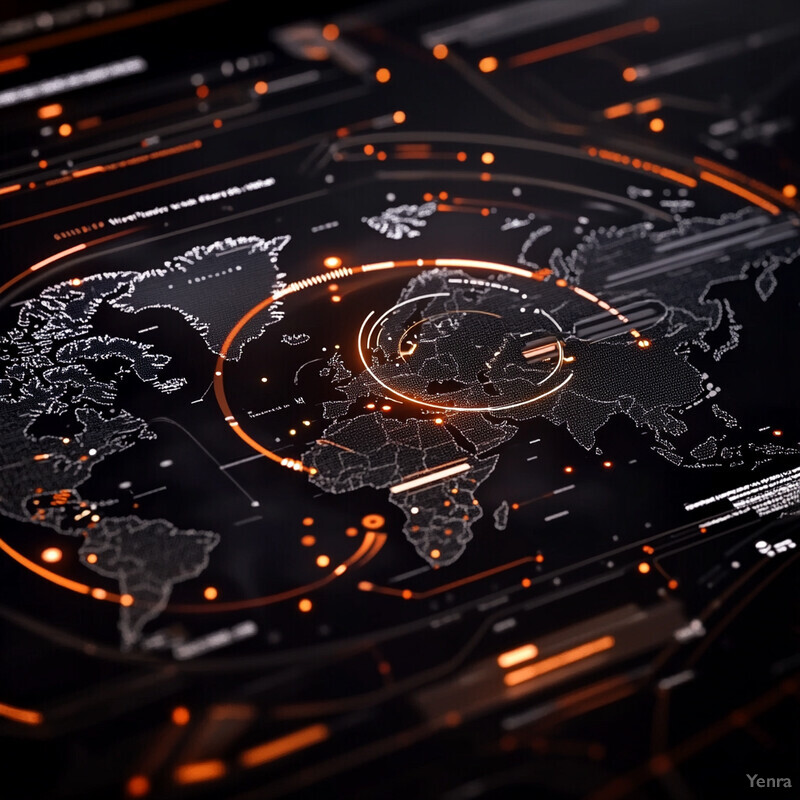
Political instability, trade disputes, new tariffs, border closures, and changes in international regulations can significantly disrupt global supply chains. AI-driven geopolitical risk monitoring tools scan political analyses, economic forecasts, customs data, and global news outlets to assess the stability of various regions and countries. By identifying emerging policy shifts, potential sanctions, or regulatory overhauls, these models help companies anticipate supply chain bottlenecks, shifting trade lanes, and the need to reconfigure sourcing strategies. With timely insights into the geopolitical landscape, organizations can stay compliant, mitigate volatility, negotiate better terms, and maintain a steady flow of goods across borders.
8. Currency and Commodity Price Fluctuation Prediction
Machine learning algorithms detect patterns in historical and live financial data, improving forecasts of currency exchange rates and commodity prices that affect procurement strategies.
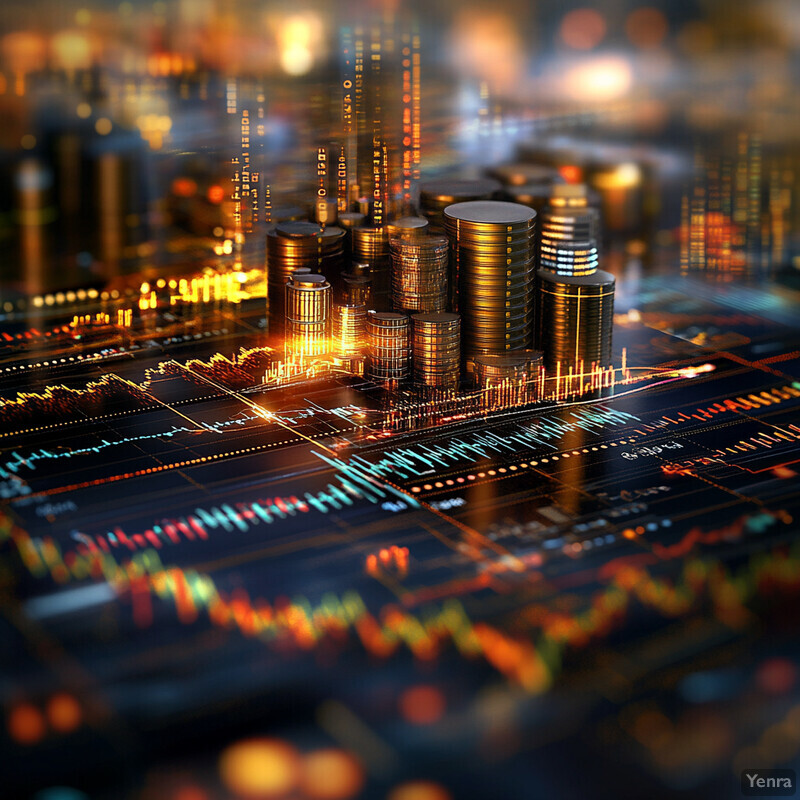
Volatile currency exchange rates and commodity prices introduce financial risk into procurement and logistics operations. AI-powered models blend historical pricing data, macroeconomic indicators, weather patterns (for commodities like crops), and even social media sentiment to forecast the probability of fluctuations in currency values or raw material costs. This foresight allows procurement teams to lock in favorable contracts, adjust safety stock levels, or diversify supply sources to hedge against price spikes. Over time, improved predictability in financial aspects of the supply chain enhances budgeting, cost control, and margin stability.
9. Automated Scenario Simulations
AI systems can rapidly run thousands of “what-if” scenarios to test the resilience of the supply chain under various disruptions, enabling informed contingency planning.
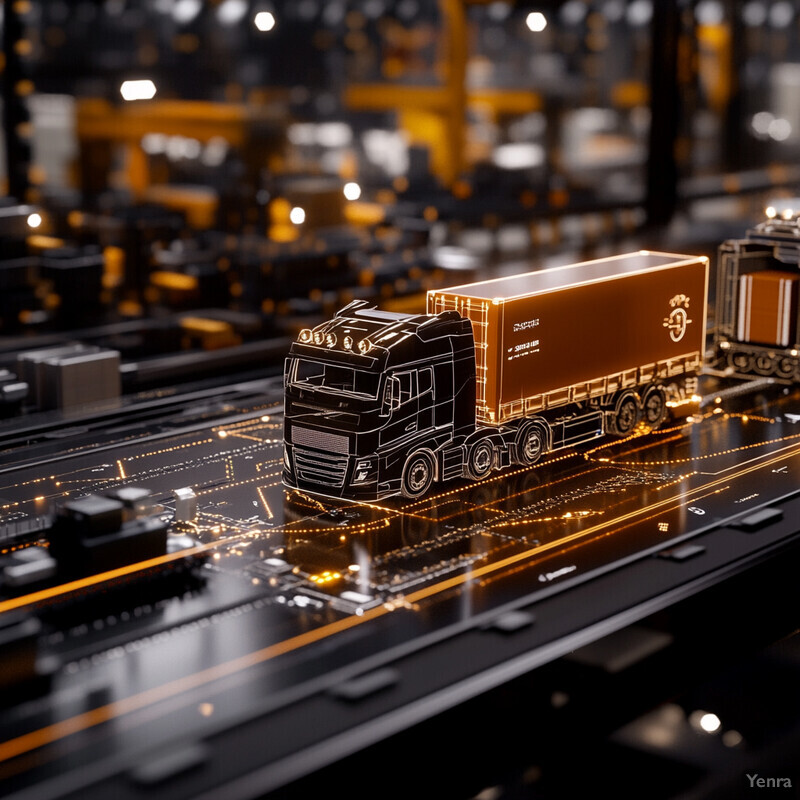
Planning for the unexpected is a core element of supply chain risk management. AI-driven simulation tools can rapidly generate thousands of “what-if” scenarios, modeling the supply chain’s response to hypothetical events like a critical supplier’s shutdown, a sudden demand surge, or a port closure. These tools consider multiple variables—such as lead times, transportation costs, storage capacities—and dynamically compute outcomes. This capability enables organizations to test resilience strategies, identify vulnerabilities, determine optimal buffer stocks, or evaluate contingency plans. By experimenting in a virtual environment, decision-makers can develop robust risk mitigation strategies that minimize disruption when real-world challenges arise.
10. Optimized Inventory Positioning
Predictive models guided by AI help determine optimal stock levels and distribution strategies, balancing cost and risk while increasing supply chain agility.
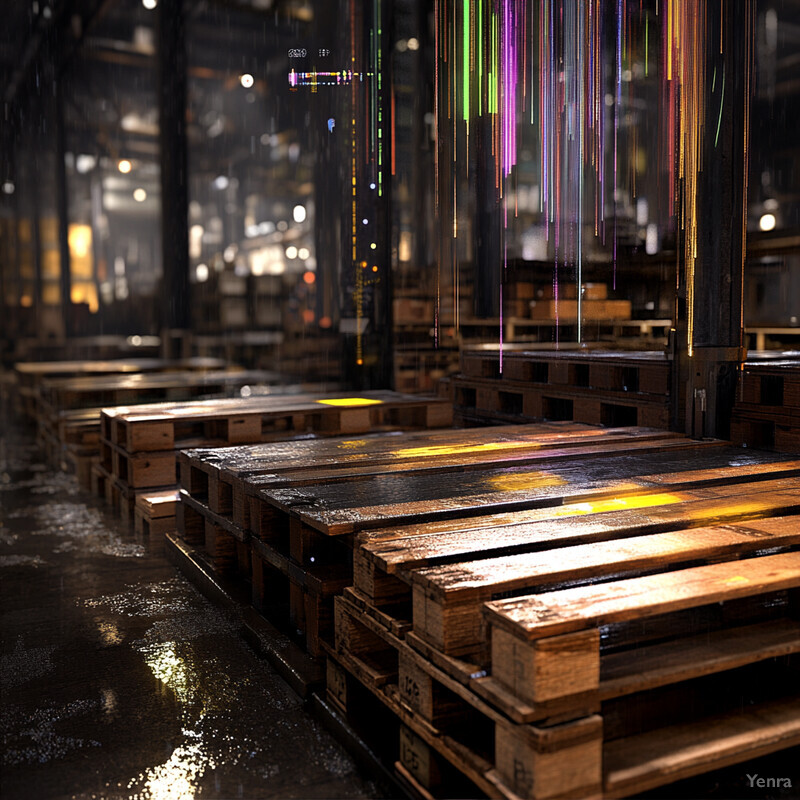
Inventory positioning—deciding what to stock and where to store it—is a delicate balance between cost containment and service reliability. AI-driven predictive models analyze a comprehensive range of demand signals, transportation constraints, seasonal trends, and supplier lead times to find the optimal inventory distribution strategy. By understanding where and when risks might arise, such as transportation strikes or supplier delays, these models guide inventory placement to preempt disruption. The result is a leaner, more agile network that reduces both excess inventory costs and the risk of running out of critical products, ensuring timely fulfillment and improved customer satisfaction.
11. Fraud and Compliance Detection
Advanced pattern recognition flags fraudulent invoices, unauthorized transactions, and non-compliance in sourcing, ensuring safer and more transparent supplier networks.
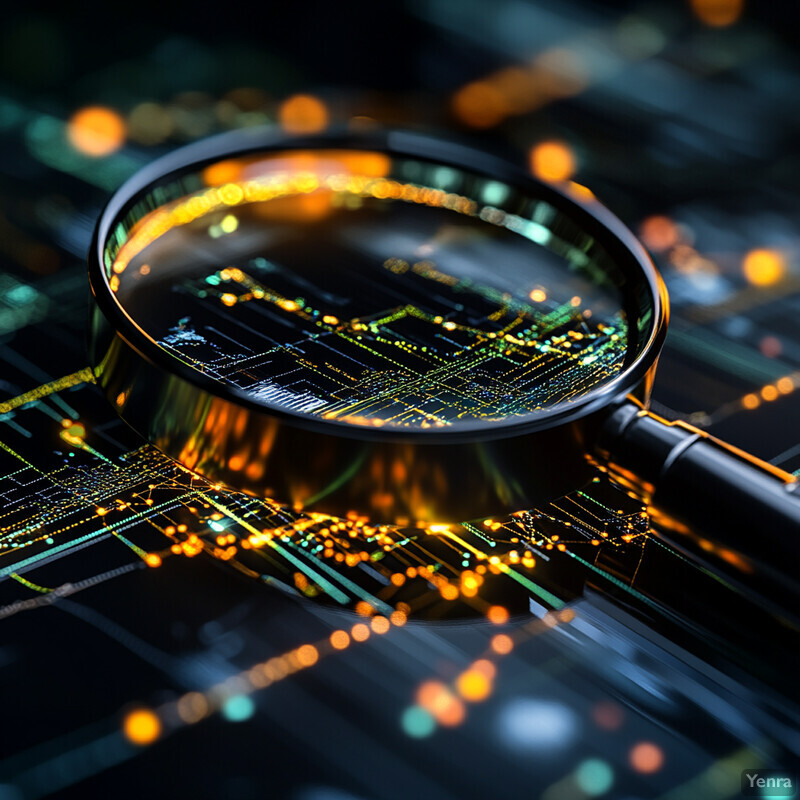
Supply chains often involve multiple handoffs, complex documentation, and a variety of transactions, all of which can create opportunities for fraud or non-compliance. AI solutions use pattern recognition and anomaly detection to scrutinize invoices, contracts, and financial transactions, identifying suspicious patterns—such as inflated charges, duplicate payments, or unapproved vendors. By cross-referencing these patterns with compliance requirements and supplier credentials, AI tools help organizations maintain a secure and transparent supply chain. Early detection of fraudulent activity prevents financial losses, reputational damage, and potential legal penalties, while fostering greater trust among stakeholders.
12. Supplier Network Resilience Scoring
AI consolidates data from multiple sources to provide a resilience score for each supplier, helping organizations choose partners less likely to fail under stress.
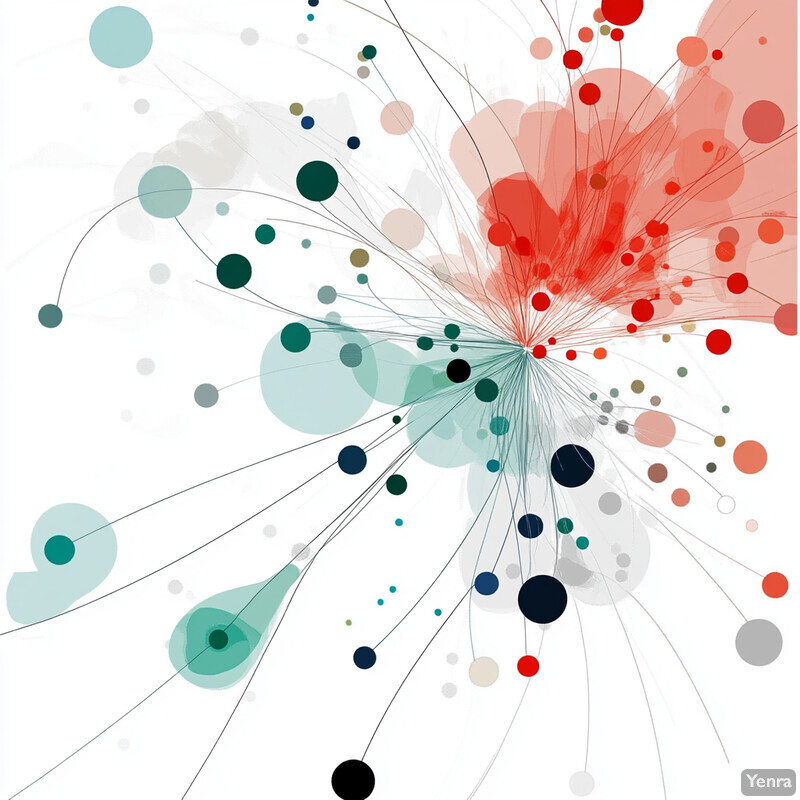
Not all suppliers are equally resilient in the face of disruptions. Some may be more likely to fail during economic downturns, while others might lack reliable backup plans for raw material shortages. AI-based models consolidate data on supplier financial health, past performance, industry benchmarks, certifications, and response times to generate a composite 'resilience score.' This score provides a standardized way to compare suppliers, guiding procurement professionals in choosing partners with robust contingency plans and strong track records. With access to these insights, organizations can build a more resilient supplier network, reducing their vulnerability to unexpected shocks.
13. Linking Operational and Financial Risk Indicators
By connecting operational metrics (like lead times and fill rates) with financial indicators, AI highlights systemic vulnerabilities and better aligns risk management with corporate goals.
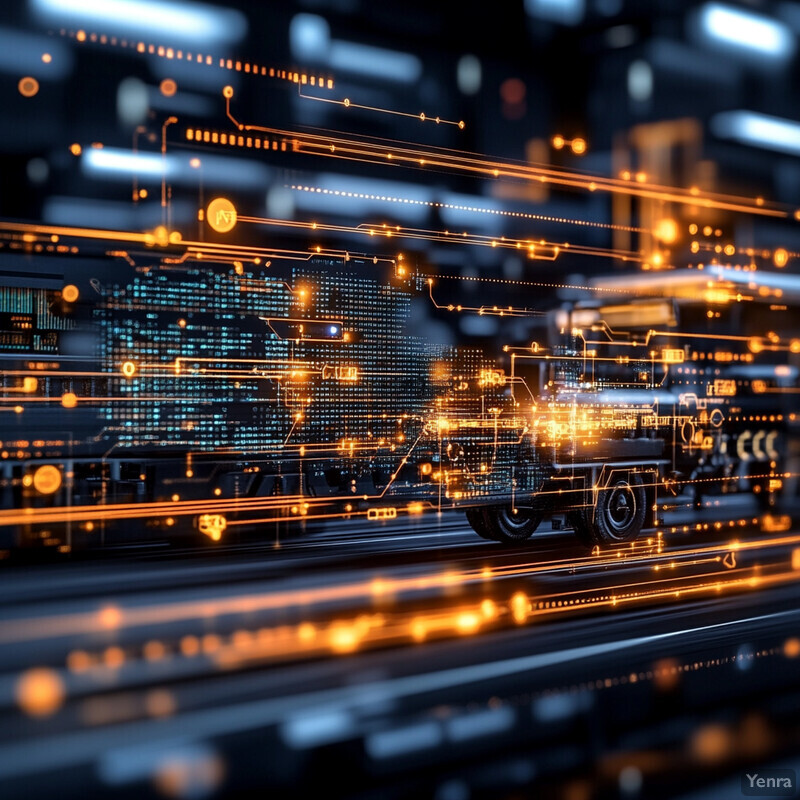
Operational performance indicators—like on-time delivery rates, cycle times, and service levels—have inherent financial implications, from working capital requirements to loss of sales. AI-driven analytics platforms link these metrics with financial data to highlight correlations and causal relationships. By understanding how certain operational inefficiencies, demand variations, or quality issues translate into increased costs or reduced revenues, organizations gain a more holistic view of risk. This integration allows for better alignment of supply chain strategies with corporate goals, enabling more informed resource allocation and proactive efforts to address operational vulnerabilities before they become financial burdens.
14. Machine Vision for Quality Control
Computer vision systems identify product defects and quality issues earlier in the supply chain, reducing recalls and improving supplier performance predictions.
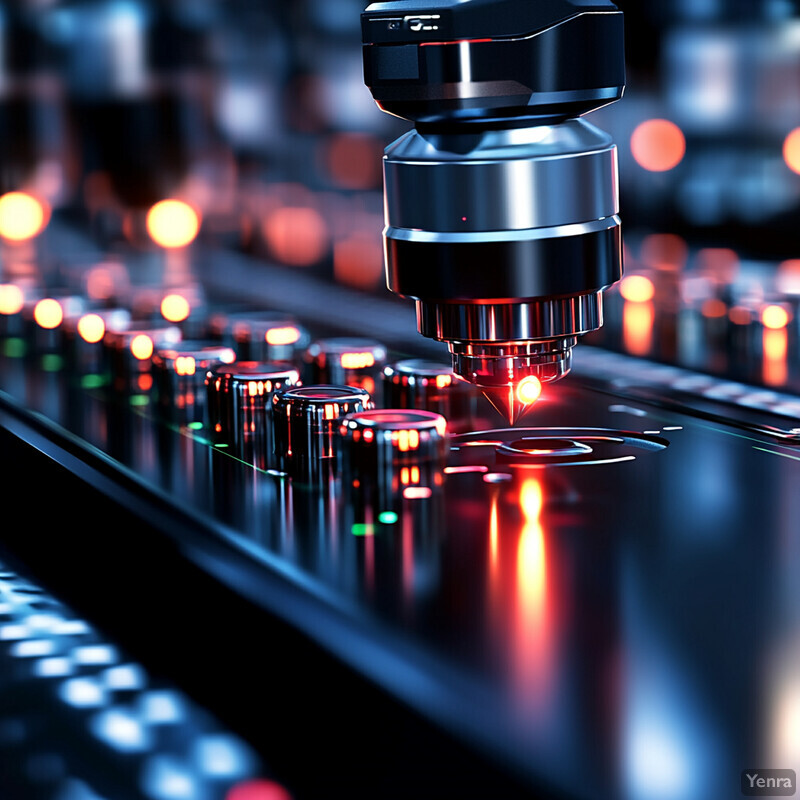
Quality defects not only impact customer satisfaction but can also have cascading effects on the supply chain if discovered too late. AI-driven machine vision systems inspect products on assembly lines or in warehouses, identifying defects—like surface imperfections, dimensional errors, or labeling inaccuracies—early in the process. By integrating quality assurance into predictive modeling, organizations can flag problematic batches, isolate affected materials, and anticipate how these quality issues might impact future shipments. This early detection reduces the risk of costly recalls, strengthens brand reputation, and ensures that downstream partners receive consistent, high-quality goods.
15. Adaptive Supply Chain Planning
Reinforcement learning techniques enable continuous learning from disruptions, improving the supply chain’s adaptive capacity and predictive accuracy over time.
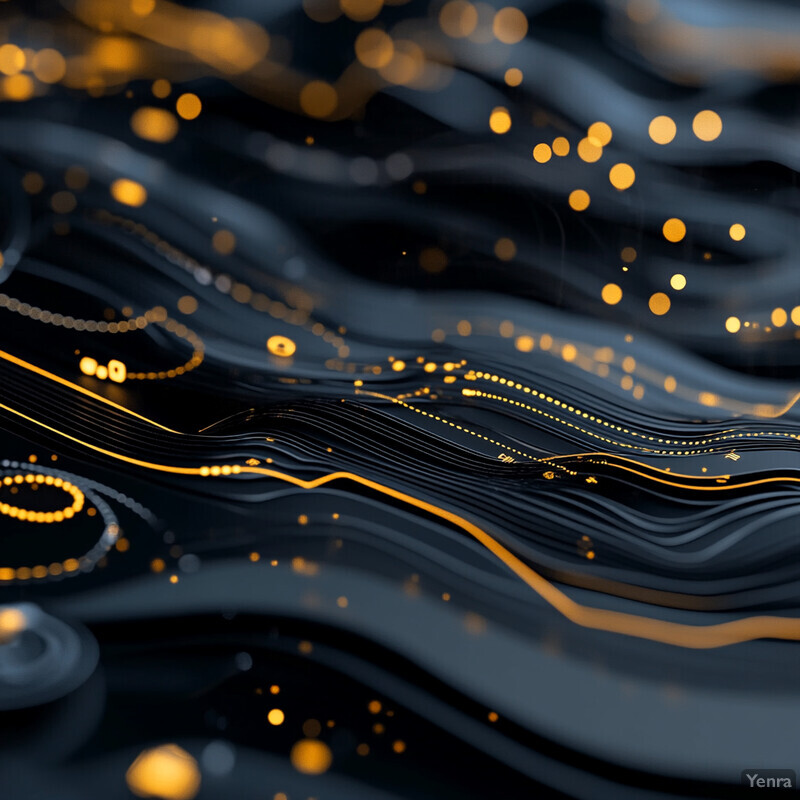
Forecasts and assumptions used in supply chain planning can quickly become outdated as conditions change. Adaptive AI models use reinforcement learning and other advanced techniques to continuously learn from new data, feedback loops, and outcomes. When disruptions occur—such as unexpected plant closures, new regulations, or sudden demand shifts—these models adjust their parameters and plans on the fly. The result is a more responsive supply chain that improves over time, reducing risk exposure by always staying one step ahead of emerging challenges and fine-tuning operational strategies as the environment evolves.
16. Enhanced Cybersecurity Posture
Predictive AI models can identify cybersecurity risks—phishing threats, ransomware attacks, system anomalies—before they compromise the digital supply chain infrastructure.
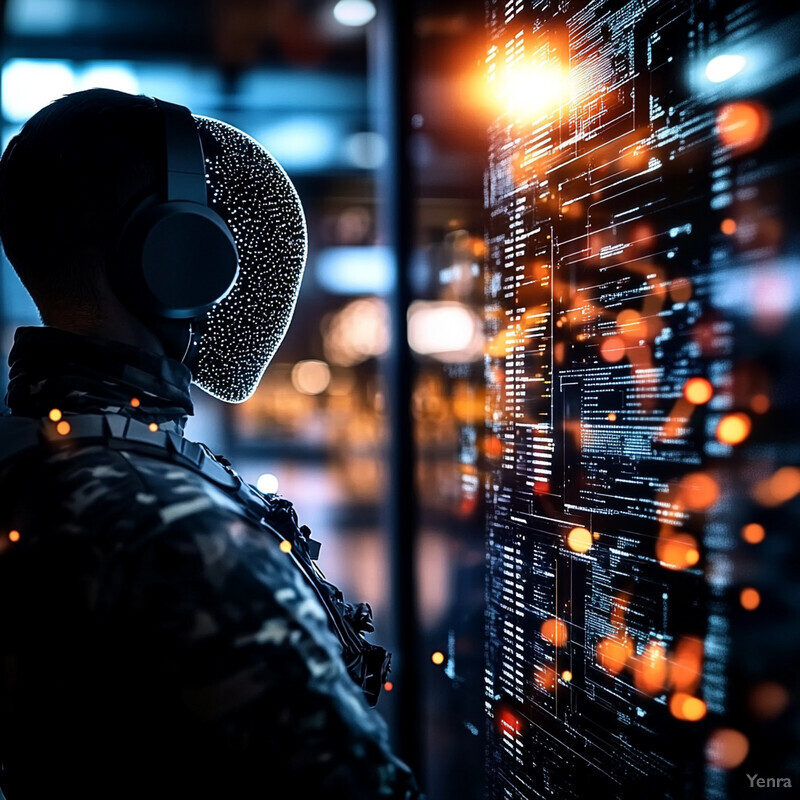
The digital transformation of supply chains has increased exposure to cybersecurity threats, from data breaches to ransomware attacks. AI-based cybersecurity tools scan network logs, user behavior, and system traffic patterns to detect suspicious activities before they compromise critical systems or data. By identifying anomalies—such as unusual login locations, attempts to access restricted databases, or unauthorized device connections—these models help security teams respond preemptively. Preventing cybersecurity incidents not only safeguards sensitive information and intellectual property but also preserves the integrity of digital platforms that underpin modern supply chain operations.
17. Transportation Route Optimization
AI considers traffic patterns, port congestion, labor availability, and fuel costs to pre-empt logistical bottlenecks and optimize delivery routes under various risk scenarios.
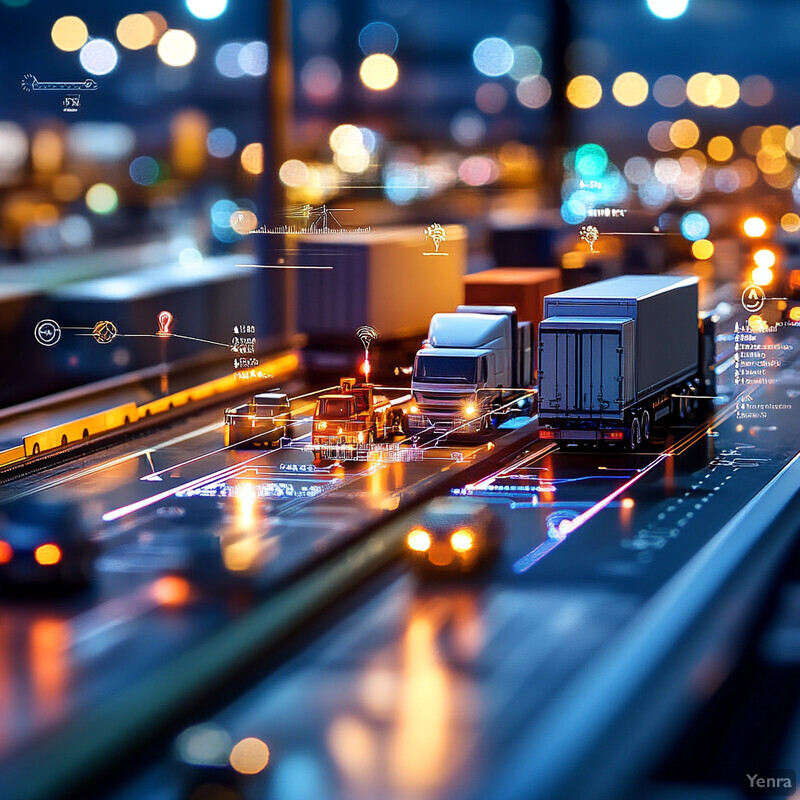
Route planning is not simply about finding the shortest path—disruptions like heavy traffic, weather conditions, labor strikes at distribution centers, or capacity constraints at ports must all be considered. AI-driven optimization models weigh these diverse factors to devise flexible and risk-aware transportation plans. By continuously recalculating the best routes as conditions evolve, these models help logistics planners minimize delays, fuel costs, and risk exposures. The outcome is a more efficient, reliable, and cost-effective transportation network that can swiftly adapt to unforeseen events, ensuring timely deliveries and preserving customer satisfaction.
18. Continuous Risk Monitoring Dashboards
AI-driven dashboards provide real-time, comprehensive views of all risk indicators, enhancing situational awareness and enabling swift, data-driven decisions.
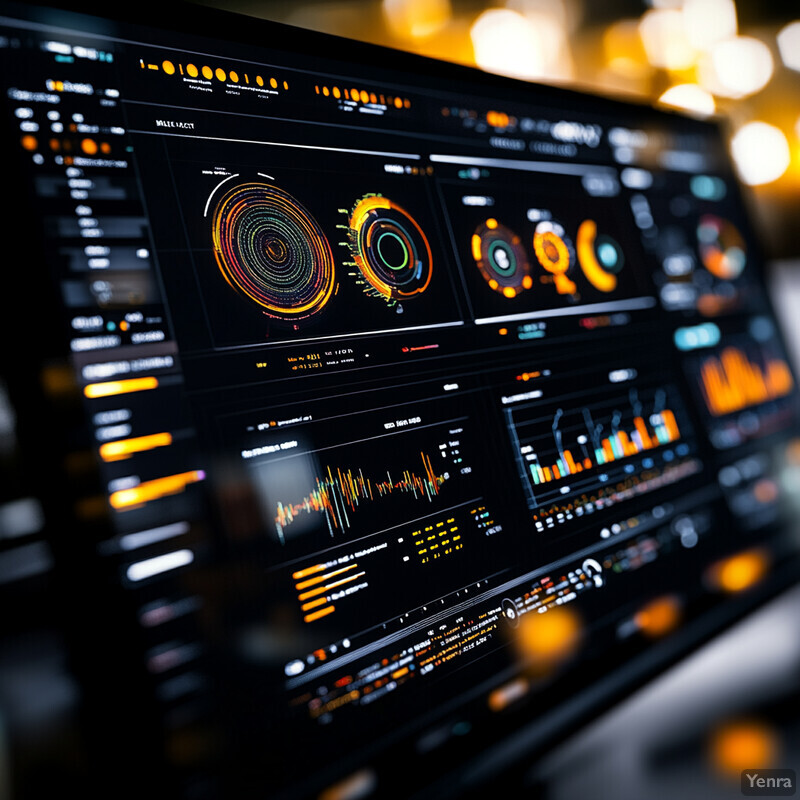
Managing supply chain risk requires a clear, current understanding of multiple risk factors simultaneously. AI-powered dashboards consolidate real-time data streams—supplier performance metrics, inventory levels, transportation conditions, regulatory updates, and more—into a single, intuitive interface. These platforms highlight trends, flag emerging threats, and provide drill-down capabilities for detailed analysis. By centralizing risk data, decision-makers can quickly identify priority issues, mobilize resources for intervention, and collaborate effectively across departments. This holistic visibility ensures that the supply chain remains resilient, agile, and prepared to address disruptions at any moment.
19. Integration with IoT Sensor Data
IoT-enabled AI models leverage sensor data from vehicles, containers, and warehouses, predicting issues like spoilage, temperature excursions, or mechanical failures.
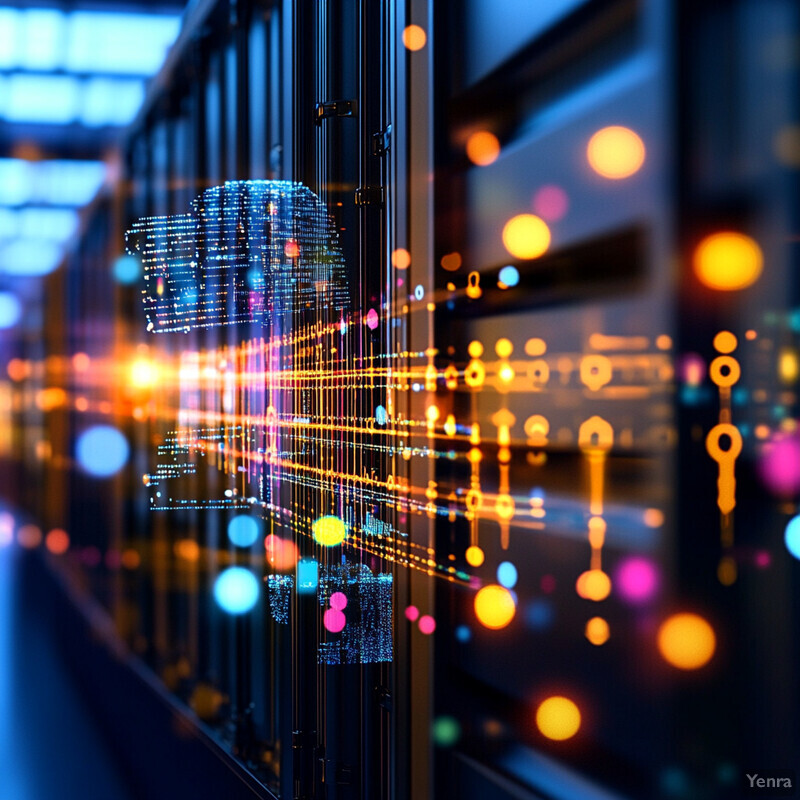
The Internet of Things (IoT) enables a more granular understanding of what is happening in the physical supply chain. Sensors attached to shipping containers, pallets, and warehouse equipment track environmental conditions such as temperature, humidity, vibration, or shock. AI models interpret this sensor data in real time, predicting spoilage in perishable goods, detecting tampering, or foreseeing equipment failures. By combining IoT insights with predictive risk modeling, companies can take preventive measures—rerouting sensitive shipments, adjusting storage conditions, or performing preemptive maintenance—ultimately reducing losses and boosting supply chain reliability.
20. Explainable Risk Modeling
Emerging Explainable AI techniques enhance transparency, helping stakeholders understand why the model predicts certain risks, increasing trust and facilitating informed decision-making.
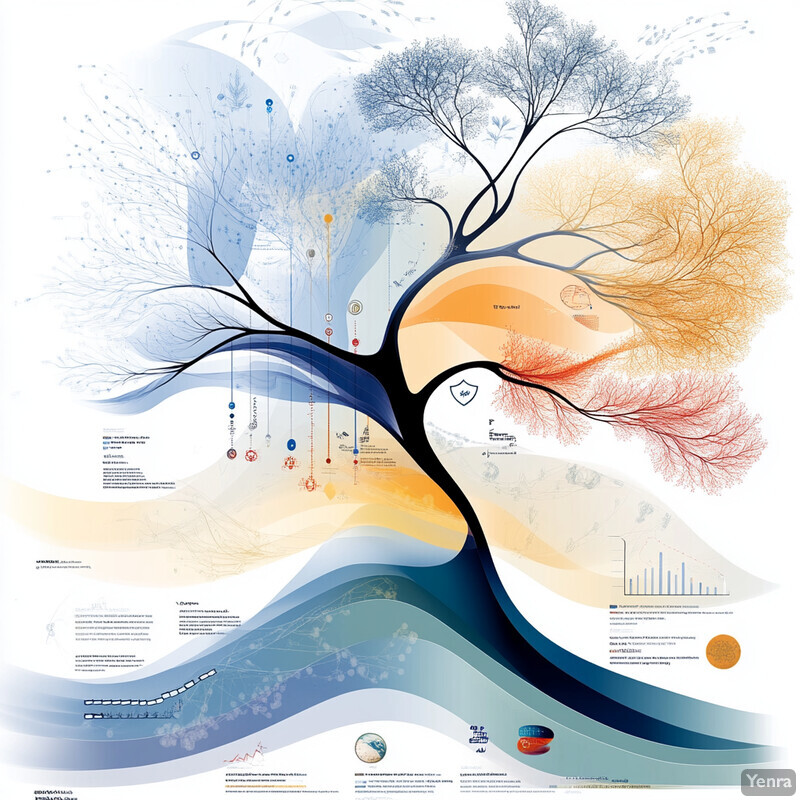
While AI models can be extraordinarily powerful, their complexity sometimes leads to a 'black box' effect where it’s unclear why a certain risk score or forecast was generated. Explainable AI (XAI) methods focus on transparency, providing human-friendly explanations for model outputs. This is crucial for building trust among stakeholders—such as managers, regulators, and customers—who need confidence in the analytics driving supply chain decisions. By highlighting which factors contributed most to a given prediction, XAI tools enable more insightful decision-making, easier compliance with regulatory requirements, and continuous improvement in model accuracy and fairness.