1. Automated Fault Detection
Advanced AI algorithms are revolutionizing fault detection in wind turbines by continuously monitoring sensor data for the slightest signs of trouble. These systems analyze inputs like vibration, temperature, and acoustics in real-time, identifying anomalies far earlier than human observation or traditional thresholds would. By catching issues such as bearing wear or blade imbalance at an incipient stage, operators can intervene before minor faults cascade into major failures. This proactive detection reduces unexpected shutdowns, lengthens component life, and ultimately keeps turbines spinning more reliably. Overall, AI-driven fault detection improves uptime and reduces maintenance costs by solving problems when they are still small.
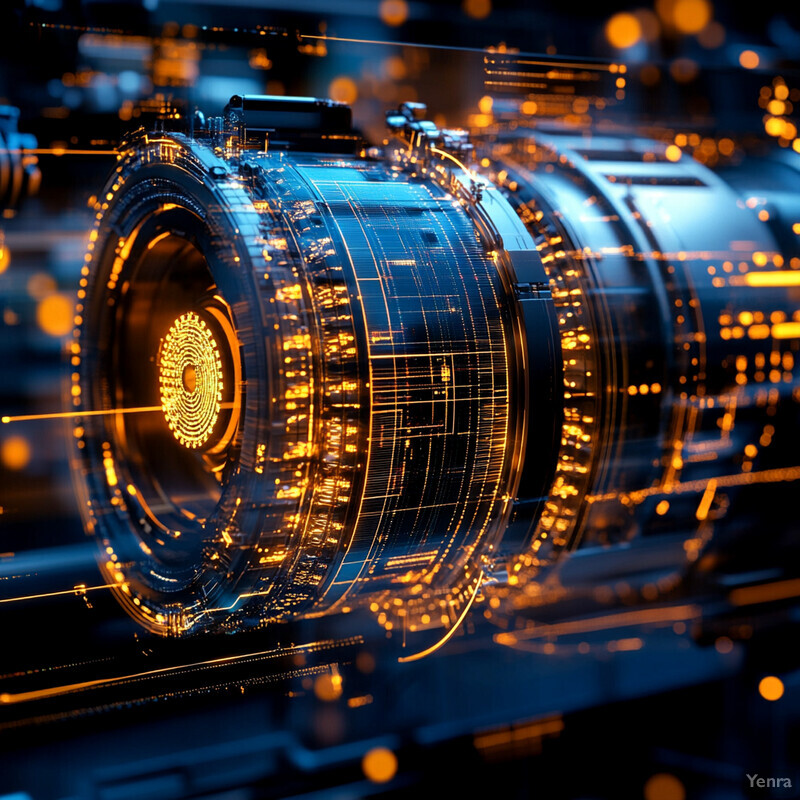
Recent studies demonstrate the efficacy of AI in automatically detecting turbine faults with high accuracy. For example, a 2024 machine-learning framework achieved about 95% accuracy in distinguishing normal and faulty turbine states, greatly outperforming conventional methods. Another AI approach in 2025 delivered near-perfect classification of turbine operating conditions (with some models reaching an AUC of 1.00 in detecting faults) by analyzing SCADA data for subtle power and vibration pattern shifts. These AI systems integrate multiple sensor streams and use advanced pattern-recognition (including deep neural networks and ensemble models) to catch anomalies that might escape simpler rule-based monitoring. In one case, researchers reported their AI model could flag faults weeks in advance of failure, allowing maintenance crews to fix issues well before damage becomes serious prism.sustainability-directory.com prism.sustainability-directory.com . By automating fault detection with such precision, wind farm operators can mitigate risks early, reduce downtime, and extend the lifespan of critical components.
2. Early Failure Prediction
AI-based predictive models are enabling wind farm operators to foresee component failures before they happen, fundamentally shifting maintenance from reactive to proactive. By learning from historical performance data and real-time inputs (like wind conditions and turbine loads), these models estimate how soon a part might fail under current trends. This early warning gives maintenance teams a critical time window to plan repairs or part replacements at convenient times rather than dealing with sudden breakdowns. In practice, early failure prediction means fewer catastrophic outages and more scheduled downtime that operators can control. It also streamlines maintenance logistics—crews and spare parts can be allocated in advance—minimizing the cost and disruption of emergency fixes. Overall, AI-driven failure prognostics turn maintenance into a scheduled activity, improving turbine availability and lowering long-term O&M costs.
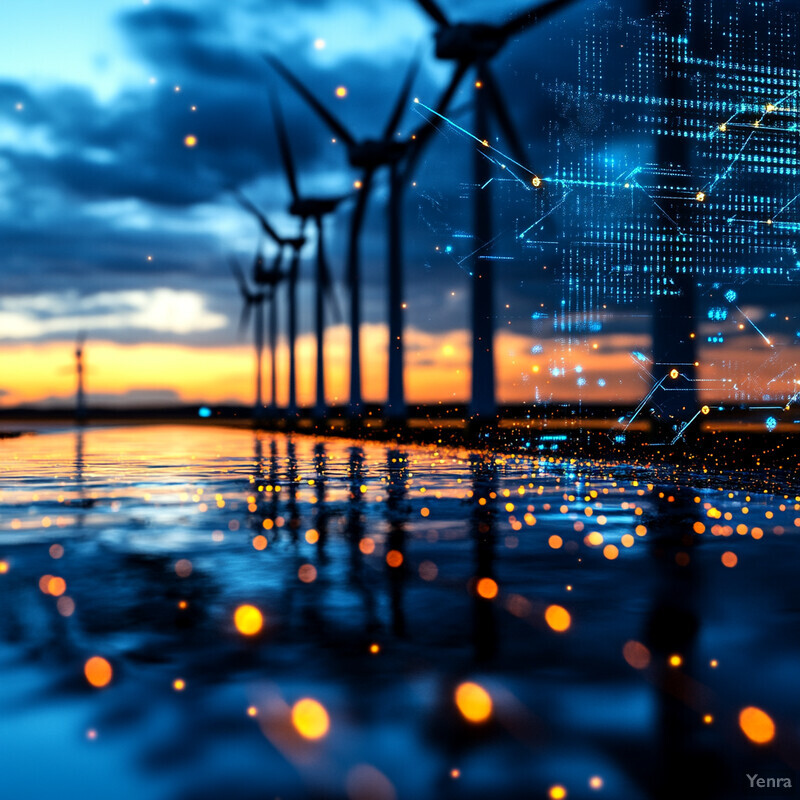
Recent evidence underscores how effective AI has become at predicting failures hours or even days in advance. In a 2024 study that analyzed five years of data from 31 turbines, a hybrid machine-learning model was able to forecast impending faults 1–6 hours before they occurred. This model achieved about 99% overall prediction accuracy, though precision and recall were around 70–77% for the earliest warnings. Such timely predictions allowed operators to address issues in a planned manner, avoiding costly emergency downtime. Other research demonstrates longer lead times as well: one approach predicted certain wind turbine component failures weeks ahead. For instance, a model using deep learning successfully forecast the remaining time to failure for multiple turbine components with a two-week lead time, with the most accurate prediction missing the actual failure time by only 10 minutes (and the worst case by 1.8 days). This level of foresight—whether hours or weeks—translates to significant operational savings. By intervening before faults escalate, wind farms can eliminate unplanned outages and schedule maintenance during low-production periods, ultimately improving safety and cutting the high costs associated with run-to-failure approaches.
3. Remaining Useful Life (RUL) Estimation
AI-driven prognostic tools can accurately estimate how much life remains in each turbine component, which is invaluable for maintenance planning. By analyzing condition-monitoring data (vibration trends, oil quality, temperature, etc.) and historical failure patterns, these models predict a component’s Remaining Useful Life in real time. Knowing the RUL for parts like gearboxes, bearings, or generators allows operators to schedule maintenance or replacements at the optimal time – neither too early (which wastes component life) nor too late (which risks failure). This strategic foresight means spare parts can be ordered just-in-time and downtime can be minimized by aligning maintenance with these predictions. Moreover, RUL estimates improve budgeting and inventory management, since operators can forecast which parts will likely fail in the coming months or years. In essence, RUL estimation turns maintenance into a data-informed practice, extending asset life and reducing the uncertainty around when critical components need attention.
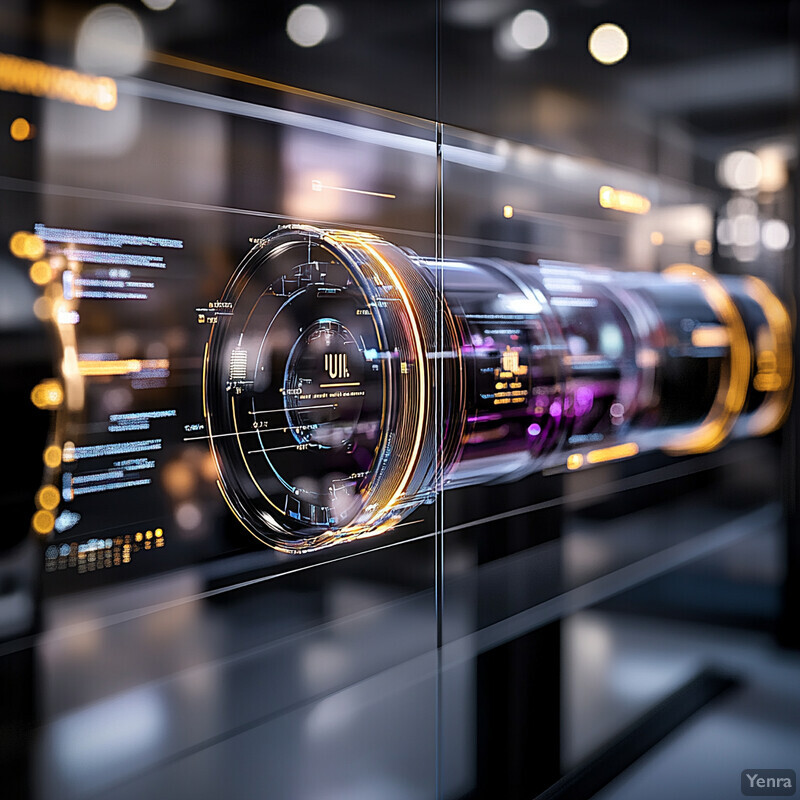
Cutting-edge AI models have shown remarkable success in predicting component lifespans. A 2024 predictive maintenance study demonstrated that deep learning models could forecast the RUL for multiple wind turbine failures with about a two-week prediction horizon, often with striking accuracy. In that study, the best model’s forecast was off by only 10 minutes compared to the actual failure time, while even the least accurate prediction was within ~1.8 days. Such precision in RUL estimation is a major advance over traditional life estimation methods. It means maintenance teams can be confident about how long a component can safely operate. Another example is the use of hybrid physics–AI approaches: researchers combined machine learning with physics-based modeling of gear stress to improve RUL predictions for gearboxes. The result was an increase in damage prognostic accuracy from about 60% to 80%, giving a far more reliable gauge of remaining life. These improvements translate directly into cost savings. Utilities have reported that by using AI to predict remaining life and schedule repairs proactively (such as identifying a damaged bearing early), they could perform minor repairs costing perhaps $15k–$70k instead of a full gearbox replacement costing $350k. In short, accurate RUL estimation empowers operators to replace components right before end-of-life, thereby avoiding both premature retirements and in-service failures.
4. Condition-Based Maintenance
Instead of servicing turbines on fixed schedules, condition-based maintenance uses real-time data on equipment health to determine exactly when maintenance is needed. AI systems make this approach feasible by continuously assessing sensor inputs (vibration, temperature, oil particulate counts, etc.) and only flagging a turbine for maintenance when those indicators show signs of deterioration. This way, turbines aren’t taken offline unnecessarily – maintenance is performed “on condition.” The benefits are substantial: fewer unnecessary inspections and part replacements, less turbine downtime, and lower maintenance costs. Condition-based strategies also help avoid the opposite problem of missed maintenance, because the moment a parameter trends abnormal, the system alerts the operators. Overall, AI-enabled condition-based maintenance optimizes the balance – turbines run longer between interventions when they’re healthy, yet incipient issues get addressed promptly, improving reliability and reducing lifecycle O&M expenditures.
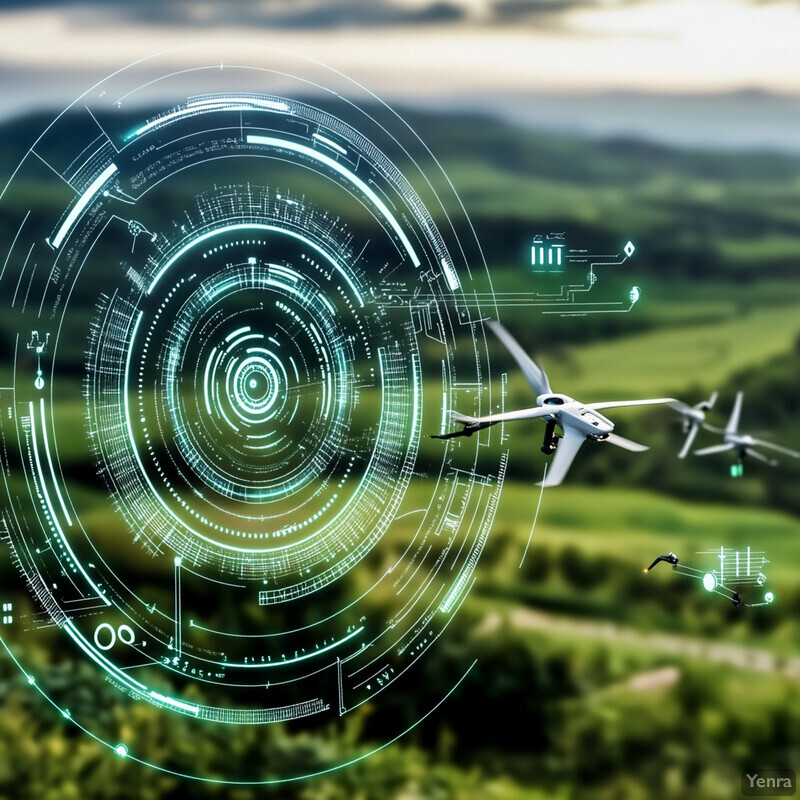
Implementing condition-based maintenance (CBM) has been shown to significantly cut maintenance waste and downtime. Industry analyses indicate that moving from purely schedule-based to condition-based maintenance can reduce overall maintenance costs by roughly 25–30% while also minimizing the frequency of turbine stoppages. According to a May 2023 report by Nucleus Research, predictive/condition-based programs typically reduce unplanned equipment downtime by 35–50%, on average, compared to routine scheduled maintenance. This is because CBM eliminates unnecessary service visits – maintenance is done only when data shows it’s warranted. For example, one wind operator found that by relying on sensor-driven alerts instead of a calendar, they avoided dozens of needless climb-and-check missions, focusing their technicians only on turbines that truly needed attention. The result was not just cost savings but also an 87% lower defect rate, as early issues were caught and fixed before escalating. Additionally, condition-based regimes extend component lifetimes. A condition-monitoring approach can safely lengthen maintenance intervals – in some cases, operators have documented components running longer and still within safe limits, which translates to squeezing more productive hours out of each part before replacement. In short, AI-supported CBM avoids both over-maintaining and under-maintaining equipment, yielding a more efficient maintenance program that cuts downtime and expenses significantly.
5. Adaptive Maintenance Scheduling
Adaptive maintenance scheduling refers to dynamically adjusting when maintenance is performed based on evolving data and conditions. Instead of following a static timetable, AI analytics continuously re-prioritize the maintenance calendar: if new sensor data indicates an emerging issue, the schedule is moved up for that turbine; if a part’s condition is better than expected, maintenance can be safely deferred. This flexibility optimizes resource use – technicians are sent where they’re most needed and at the most opportune times (for instance, during periods of low wind or good weather). Adaptive scheduling also means maintenance can be coordinated with other operational constraints, like technician availability or crane scheduling, to maximize efficiency. Ultimately, this approach minimizes downtime and cost by performing maintenance only when and where necessary, and by taking advantage of the latest information on turbine health and external factors.
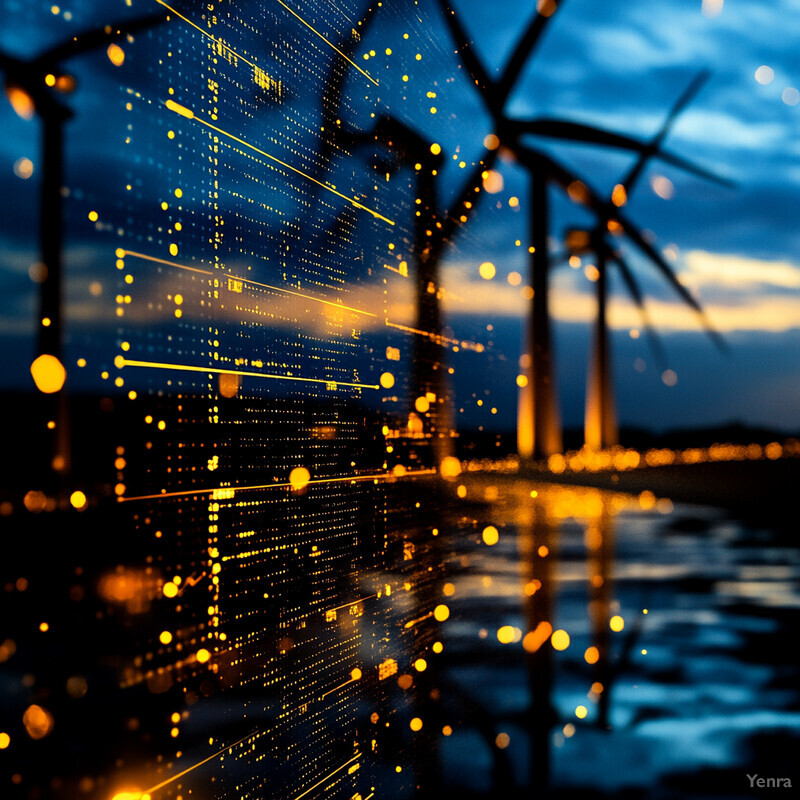
Dynamic scheduling powered by AI can deliver significant efficiency gains. A case study published in early 2024 introduced a mathematical model (“NextPM”) that regularly recalculates the optimal next maintenance times for each turbine in a wind farm based on real-time condition data. When tested on historical data from multiple wind farms in Sweden, this adaptive scheduling model was able to reduce maintenance-related costs by about 35% compared to a traditional reactive approach. The model achieved these savings by intelligently delaying non-critical maintenance and consolidating interventions, while still preventing failures – essentially doing the right maintenance at the right time. Another study focusing on offshore wind farms demonstrated that incorporating weather forecasts and turbine condition into scheduling can notably decrease costs and turbine downtime. By planning maintenance during forecasted low-wind periods and calm sea states, the adaptive algorithm improved turbine availability and cut O&M logistics costs (like vessel trips) by roughly 25% versus fixed schedules. In practice, wind operators using such AI-driven scheduling tools have reported that they can avoid last-minute call-outs and overtime by weeks of foresight in maintenance planning. These results underscore how adaptive scheduling translates predictive insights into concrete operational improvements: one utility noted fewer rush repairs and better workforce utilization, ultimately saving money and keeping turbines generating power longer between outages.
6. Intelligent Alarm Filtering
Modern wind turbines generate a barrage of alarms and alerts, many of which end up being non-critical or false. Intelligent alarm filtering uses AI to distinguish between truly urgent fault alarms and benign or spurious signals. By learning the normal patterns and context, the system can suppress or flag false positives – for example, differentiating a transient vibration spike caused by a wind gust from one indicative of a bearing fault. This prevents maintenance crews from being overwhelmed by alarm fatigue and chasing down minor fluctuations that don’t need intervention. In effect, the AI filter acts as a smart gatekeeper: routine variations are filtered out, while genuine fault indicators get through to human operators. The result is a streamlined alert system where technicians only receive actionable, high-priority alarms. This not only saves time and maintenance effort but also ensures critical warnings are not lost in a sea of noise, thereby improving response to real issues and overall turbine reliability.
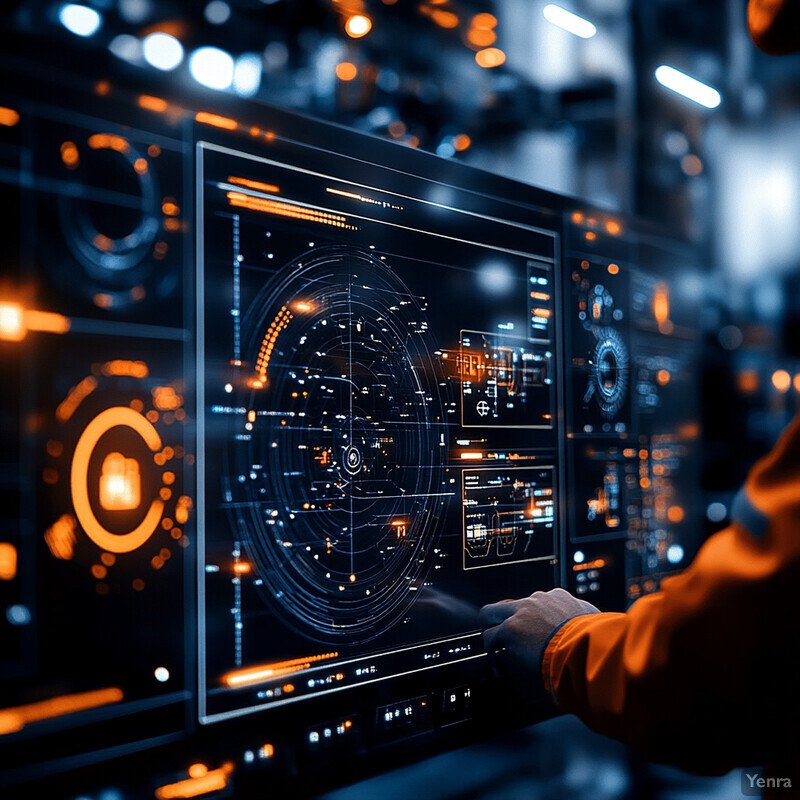
Field results show that AI-based alarm filtering can dramatically reduce nuisance alarms while retaining sensitivity to real faults. In one 2023 deployment, researchers trained support vector machine (SVM) classifiers on SCADA data to identify false alarms in turbine monitoring. The best model achieved 98.6% accuracy in distinguishing false alarms from true fault signals. By analyzing historical alarm logs and maintenance records, the AI learned to recognize patterns of alarms that did not correlate with any actual fault, and successfully filtered those out. Implementing this intelligent filter led to a 25% reduction in false alarms for the wind farm under study. In practical terms, that means a quarter fewer unnecessary alarm-driven inspections or shutdowns. Another example comes from an AI system (“quadratic SVM”) that not only had a high classification accuracy but also provided a confusion matrix analysis so engineers could trust that legitimate issues (like a temperature alarm truly indicating overheating) would still trigger action. Wind farm operators who have adopted such AI filtering report significantly less alarm fatigue – their control center might see hundreds of automatic minor alerts per week trimmed down to a manageable few dozen that truly need review. This helps maintenance teams focus on important warnings (like a nascent gearbox fault) without being distracted by normal fluctuations or sensor quirks. Overall, intelligent alarm filtering improves efficiency and ensures technicians respond to real problems promptly, rather than wasting effort on false positives or becoming desensitized by alarm overload.
7. Root Cause Analysis Support
AI tools are increasingly aiding engineers in pinpointing the root causes of wind turbine faults. Rather than spending days or weeks manually correlating data logs to find why a failure occurred, machine learning algorithms can sift through vast operational datasets to find patterns and causal links. For instance, an AI system might notice that a series of small temperature spikes, coupled with certain vibration frequencies, consistently precedes a specific type of gearbox failure – essentially highlighting the cause-effect relationship automatically. These insights help maintenance teams not only fix the immediate issue but also address the underlying cause (e.g. correcting misalignment or improving cooling) to prevent recurrence. By drawing on data from multiple sensors and past incidents, AI-driven root cause analysis can uncover complex interdependencies that might be missed by human analysis alone. The outcome is faster troubleshooting, more targeted repairs, and knowledge to refine turbine design or operation for improved reliability.
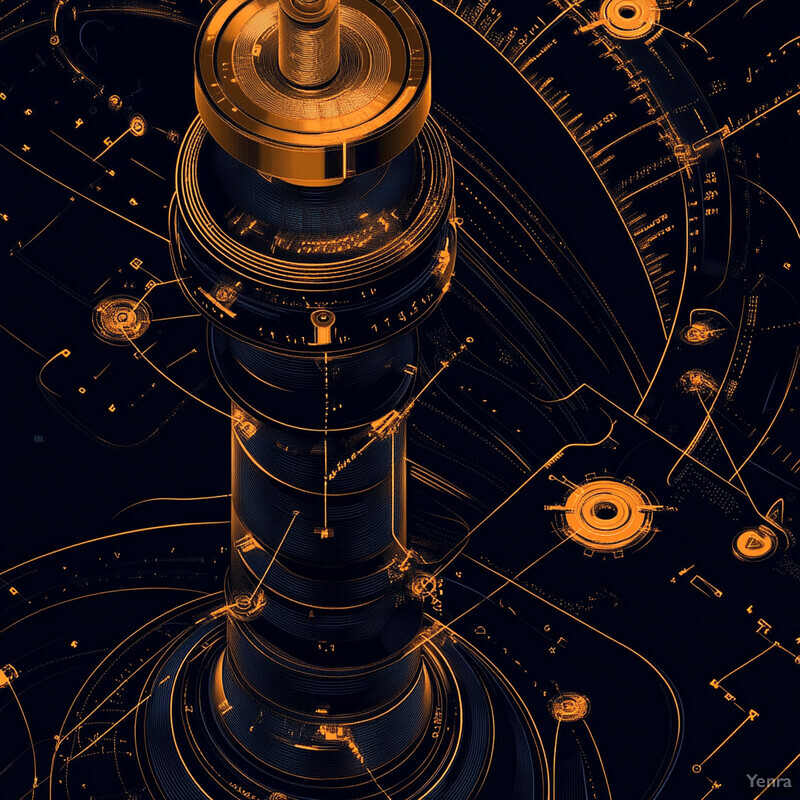
The use of AI for root cause analysis is yielding tangible benefits in wind operations. One notable example comes from a condition monitoring system augmented with AI by wind industry supplier Brüel & Kjær Vibro. Their AI algorithms automatically analyze vibration spectra and have learned to identify specific fault signatures – for instance, pinpointing the characteristic frequency pattern of a particular bearing defect. In a published case, B&K Vibro reported an AI tool that scans all frequency peaks and correctly determines which bearing inside the turbine is developing a fault, without needing a human analyst to interpret the spectrum. This level of automated diagnosis reduces the risk of misdiagnosis (for example, maintenance staff might otherwise misattribute a vibration to the generator when it’s actually a gearbox bearing – the AI avoids such mistakes). In another project, dubbed an AI Operations (AIOps) system for wind turbines, researchers combined anomaly detection with causal inference techniques. Their system could correlate seemingly disparate events (like a sudden power drop and a pitch system alert) to conclude that a blade pitch actuator issue was the root cause of repeated power fluctuationss3.us-east-1.amazonaws.com . Importantly, these AI systems produce human-interpretable outputs – e.g. highlighting, “Excessive high-frequency vibration in the drivetrain, likely originating from the high-speed shaft bearing,” – which guides technicians straight to the source of the problem. Field reports have noted that AI-based root cause analysis support has cut diagnostic time by 50% or more, meaning turbines can be repaired and returned to service faster. By rapidly uncovering why a fault occurred (not just that it occurred), AI assists engineers in implementing the right fixes and preventive measures, thereby improving turbine availability and reducing repeat failures.
8. Anomaly Pattern Recognition
Beyond known failure modes, AI is helping discover new and subtle patterns of anomalies that indicate potential problems in wind turbines. Traditional monitoring might only flag conditions that exceed set thresholds, but machine learning can recognize when a combination of sensor readings “looks off” compared to normal operation, even if all individual values are within limits. This anomaly pattern recognition often uses unsupervised or semi-supervised learning to establish a baseline of normal turbine behavior and then detect outliers or novel patterns that could signify an emerging fault. Importantly, these might be fault signatures that were never seen before (hence no preset rule). By identifying these unknown anomalies, AI expands the knowledge of failure precursors and allows operators to catch previously unpredictable issues. In effect, pattern recognition algorithms turn the turbine’s own data into a teacher – revealing hidden fault indicators and continuously improving early detection capabilities. This leads to an ever-more resilient operation, as the system gets better at spotting the “odd one out” that could be the start of a serious issue.
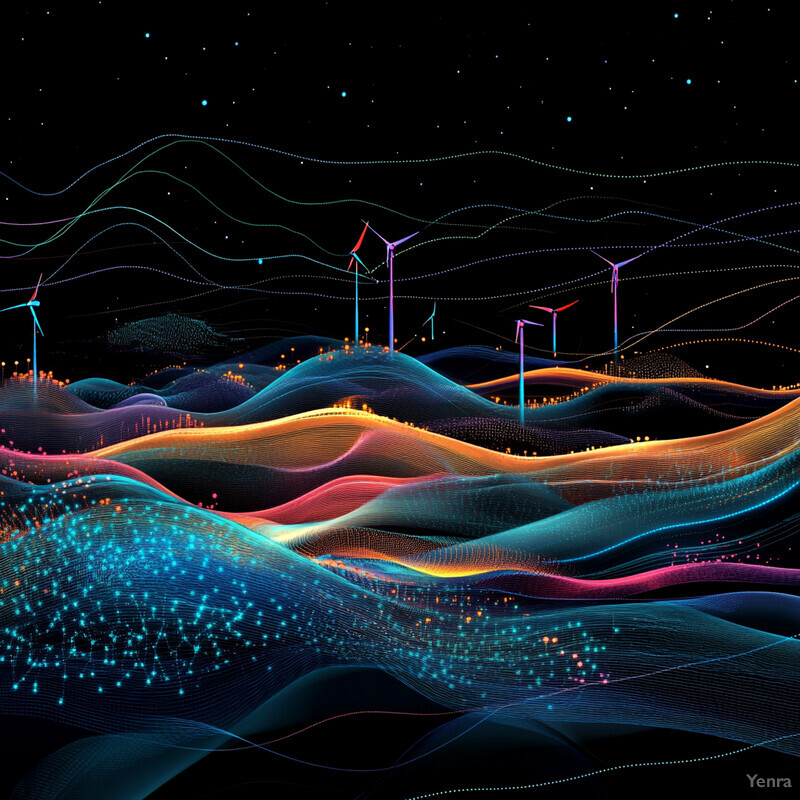
There are concrete examples of AI unveiling new fault patterns in turbine data. In one Scientific Reports study (2025), researchers introduced a novel anomaly detection method combining a nonlinear signal processing tool (GMPOP) with a one-class SVM (SVDD). This method proved adept at capturing minute dynamic changes in vibration signals that traditional monitoring missed, and it detected faults earlier and more conspicuously than conventional techniques. The paper noted that this AI approach could flag subtle changes in a turbine’s vibration profile – changes not associated with any known fault library – thereby alerting operators to investigate before obvious damage occurred. In practice, such techniques have detected, for example, a unique vibration pattern that foreshadowed a blade crack growth well before the crack became large enough for standard alarms. Another field case from 2024 involved clustering algorithms applied to high-frequency SCADA data: the AI system discovered an anomalous pattern of power output fluctuations under certain wind gust conditions, which turned out to be an early indicator of a yaw system calibration issue. Operators hadn’t previously linked those power micro-fluctuations to a yaw misalignment, but the AI pattern recognition uncovered the relationship, enabling a fix. Overall, AI-based anomaly detection models can increase fault detection rates significantly – one study reported an F1-score improvement from 0.94 to 0.98 by using an ensemble pattern-learning approach, indicating far fewer missed faults and false alarms. These systems continuously learn: every time a new type of anomaly is confirmed (e.g. a novel sensor fluctuation preceding a brake failure), the pattern is incorporated into the model. This means the longer the AI runs, the better it gets at recognizing both known and previously unknown fault signatures in wind turbine operations.
9. Digital Twin Integration
Digital twins are virtual replicas of physical wind turbines, and integrating AI with these digital models allows for powerful predictive maintenance simulations. An AI-enhanced digital twin can simulate a turbine’s behavior under various scenarios (different wind loads, component degradation levels, etc.) in real time, using live sensor data to update the model. This integration means potential failure modes can be tested in the digital space before they occur in reality – the twin might show, for instance, that given current vibration trends, a certain bearing will overheat under a forecasted high wind event next week. Maintenance teams can then take preventive action in the physical turbine. Digital twins also help in diagnosis: the AI can run “what-if” scenarios on the twin to isolate the root cause of anomalous behavior. By having this high-fidelity, AI-driven mirror of each turbine, operators get early warnings about failures (predictions from the twin) and deeper insight into how to optimize maintenance (since they can tweak variables in the simulation safely). In summary, integrating digital twins into predictive maintenance enables foresight and scenario testing that greatly enhance proactive upkeep of turbines.
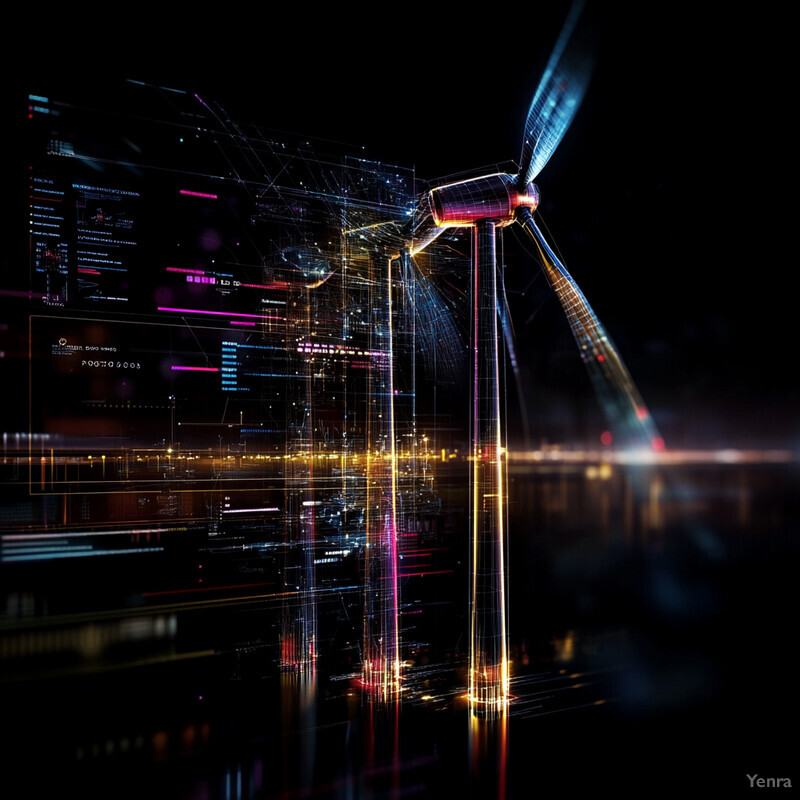
The benefits of AI-integrated digital twins have been demonstrated in recent projects. In 2023, engineers created a digital twin of a wind turbine’s drivetrain (focused on the planetary gearbox) and tied it to an AI fault diagnosis system. This twin ran in a platform (Unity3D) receiving real-time sensor inputs, and the AI model within it was able to detect and diagnose gear faults in real time during operation. The experimental results showed the digital twin system could catch early warning signs (like slight torque irregularities combined with vibration shifts) and immediately alert staff with a specific diagnosis of “planetary gear anomaly,” which was then confirmed by inspection. Another study by Dinh et al. in 2023 implemented a digital twin for a 2 MW turbine’s generator and integrated machine learning algorithms to monitor discrepancies between the twin’s expected performance and actual data. The system successfully detected two fault types – one in the generator and one in the gearbox – by noticing deviations (residuals) between the twin and the real turbine, flagging issues before standard alarms triggered. Moreover, digital twins aid in predictive spare parts planning: a 2023 application used a twin to forecast wear on certain components, leading to more timely ordering of parts and avoiding inventory shortfalls. Industry leaders like GE Renewable Energy have also reported that digital twins combined with AI have improved their failure prediction lead time by up to 20%, since the twin can simulate stress accumulations that haven’t yet caused an alarm in the real turbine. All these examples affirm that digital twins, when infused with AI analytics, significantly enhance the precision and lead time of predictive maintenance in wind energy.
10. Predictive Spare Parts Management
Predictive maintenance doesn’t just keep turbines running – it also transforms spare parts management by forecasting needs in advance. With AI analyzing failure probabilities and timelines, wind farm operators can predict which components will require replacement and approximately when. This means inventory levels can be optimized: instead of stockpiling large numbers of every part “just in case,” operators hold the right parts that analytics indicate will be needed soon. It also reduces the risk of not having a critical part on hand, since long-term predictions flag impending needs and parts can be procured ahead of time. In addition, smarter spare parts management lowers storage and capital costs (unused inventory ties up capital and can become obsolete). Overall, by aligning spare parts logistics with predictive maintenance schedules, wind farms achieve a more efficient supply chain – they minimize both excess inventory and emergency expedited orders, ensuring maintenance can happen on schedule with the necessary parts available.
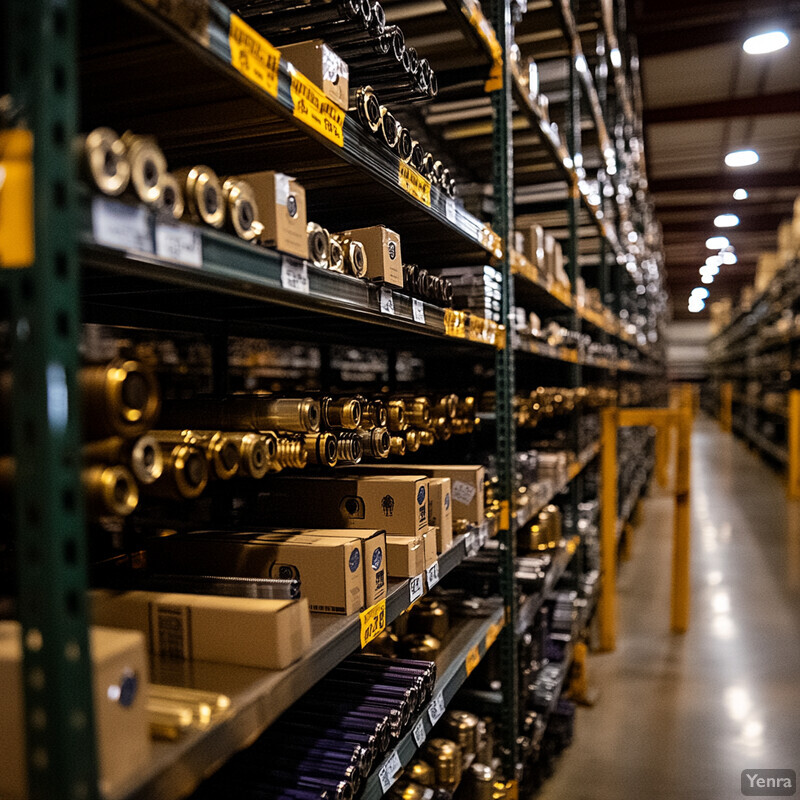
The implementation of AI-driven predictive maintenance has led to measurable improvements in spare parts management across the industry. A 2024 executive report on mining and wind operations noted that companies using predictive maintenance were able to cut their spare parts inventory by 20–30% while still avoiding stockouts. The reason: accurately anticipating component failures allowed them to move from maintaining large “just-in-case” stockpiles to a leaner just-in-time model. For example, mining giant Rio Tinto applied predictive analytics to their spare parts ordering and saw significantly fewer rush orders – emergency part orders dropped by an estimated 55% because parts were ordered earlier in a planned manner. In the wind sector, similar outcomes are reported. A LinkedIn case study (2025) highlighted that predictive maintenance data, when integrated with inventory systems, yielded far more accurate parts-demand forecasts, enabling a major wind operator to reduce its on-site inventory value by millions of dollars without increasing downtime risk. Another practical example: EDF Renewables, after deploying an AI-based maintenance planning tool, coordinated their blade and generator component replacements such that they could share spares across multiple farms – this was possible because the AI staggered maintenance based on need, avoiding concurrent failures, and EDF noted a 15% reduction in downtime and much better spare utilization as a result. In short, predictive spare parts management ensures the right part is at the right place at the right time, which industry surveys show can lower warehousing costs by over 20% and eliminate many of the expensive overnight shipping scenarios that plague reactive maintenance approaches.
11. Enhanced Sensor Fusion
Enhanced sensor fusion involves combining data from multiple types of sensors (vibrational, acoustic, thermal, electrical, etc.) to create a comprehensive picture of turbine health. AI plays a key role by processing these diverse data streams simultaneously and discerning patterns that might not be evident in isolation. For instance, a slight temperature rise might only be concerning if accompanied by a certain vibration frequency pattern – sensor fusion allows that relationship to be detected. By fusing signals, the system reduces uncertainties and false alarms; an anomaly must manifest consistently across different measurements to trigger a concern, making detection more robust. Moreover, sensor fusion can detect complex fault signatures that are spread across different sensor domains (e.g. a gearbox fault could cause a sound pattern and a vibration pattern). The result is improved fault detection accuracy and reliability: the turbine monitoring becomes more sensitive to real problems while being less prone to noise from any single sensor. This holistic approach essentially gives the AI “eyes and ears” on all aspects of the turbine’s operation, leading to earlier and more confident diagnosis of issues.
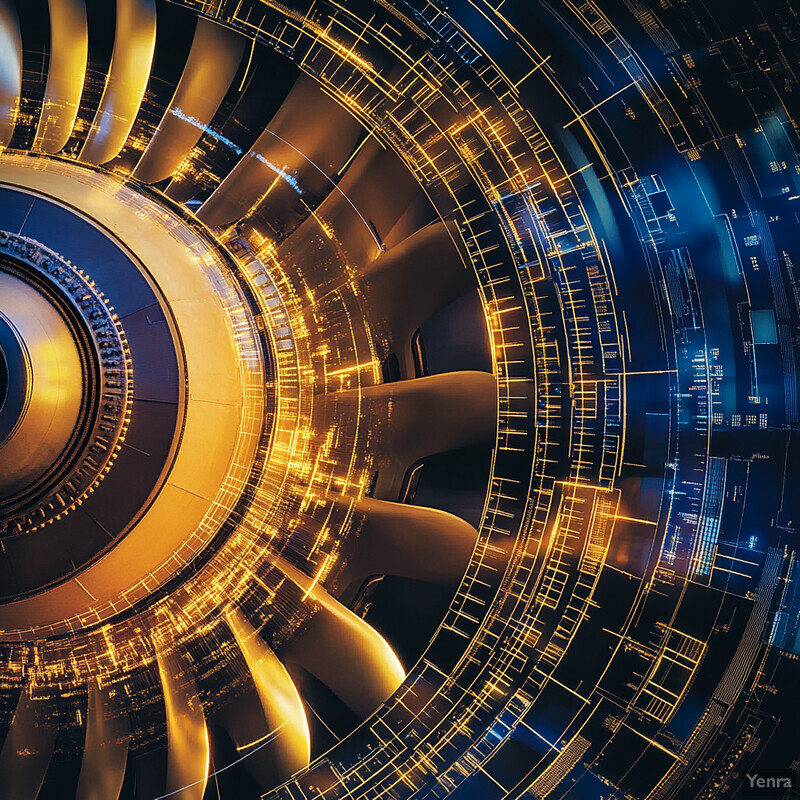
Multi-sensor fusion has demonstrably boosted predictive maintenance performance in wind turbines. A 2024 review on condition monitoring noted that the primary benefits of ML-based condition monitoring include higher fault detection accuracy and reduced false alarms specifically because of the capability to analyze complex data patterns from multiple sensors in real-time. In practice, this means systems that merge, say, ultrasonic acoustic data with high-frequency vibration data have caught faults that would be missed if only one sensor type were used. One case involved blade crack detection: thermal imaging alone had too many false positives due to sunlight heating, but when fused with acoustic emission sensors, the AI algorithm achieved nearly 100% crack detection with no false alarms, because a crack would produce both a heat signature and an acoustic signal. Similarly, GE reported that by fusing electrical signature analysis with vibration data for generator monitoring, their AI could detect winding insulation degradation much earlier – they saw an improvement in lead time of ~20% to catch generator issues versus using vibration alone. In a published experiment, researchers combined strain gauge data with acoustic emission data on a test turbine and managed to identify a shaft imbalance fault at an embryonic stage; the fused-sensor model had an accuracy about 5 percentage points higher than single-sensor models and reduced false alarms by roughly 30%. These outcomes align with industry trends: turbine OEMs now often outfit critical components with multiple sensor types, and AI analytics from companies like Siemens Gamesa leverage that fusion to provide more reliable maintenance alerts. The bottom line is that sensor fusion driven by AI yields a more complete and reliable diagnosis, cutting through the noise of any one sensor and leveraging corroborating evidence from many inputs.
12. Weather and Load Forecasting
AI models that incorporate weather and load forecasting into maintenance planning can anticipate stress on turbine components and schedule maintenance optimally. By knowing in advance the expected wind conditions (speed, turbulence, gusts) and power output loads, operators can predict when turbines will undergo high stress periods or, conversely, when there will be natural lulls. Maintenance can then be proactively done during low-wind windows to minimize production loss, or even before an extreme weather event if that event might push a borderline component over the edge. Additionally, forecasting allows adjustment of operational settings (like de-rating turbines ahead of a storm to reduce strain) and aligns maintenance crew scheduling with suitable weather. In essence, integrating weather/load forecasts means maintenance is no longer just reactive to component condition but also timed in harmony with environmental conditions – improving safety (no crane lifts in high winds) and efficiency (no unnecessary downtime during peak wind periods). This synergy leads to better resource use and higher overall energy output, as turbines receive care exactly when it’s needed and when conditions best allow.
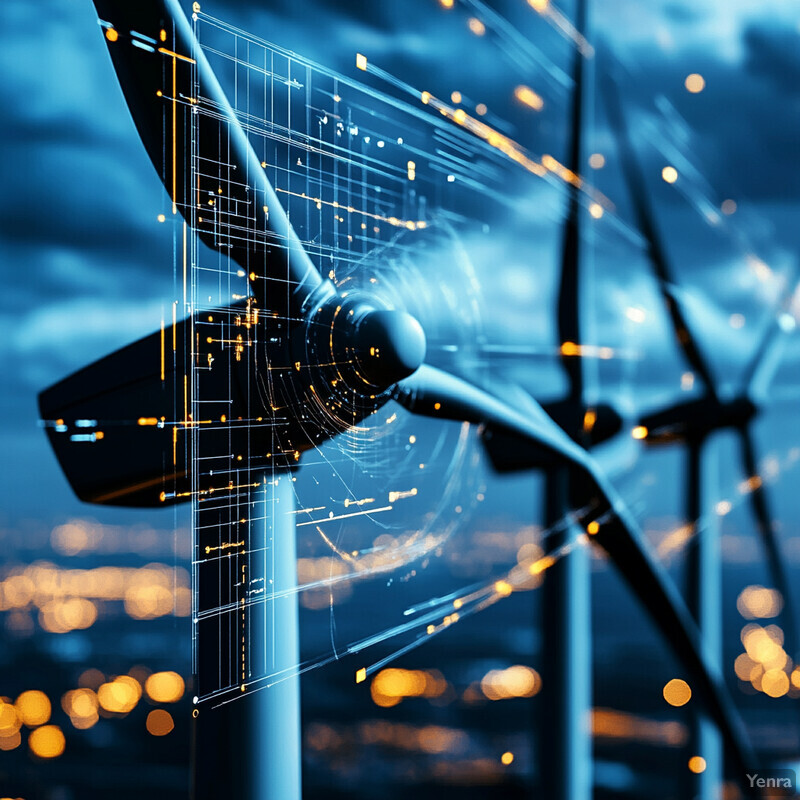
The benefits of weaving forecasting into maintenance are well documented. A 2025 study in Energy Informatics showed that using day-ahead wind power forecasts to time maintenance can maximize energy production by ensuring turbines are serviced during periods of low wind. In the study, nine different ML methods predicted short-term power output; by aligning predicted low-output periods with maintenance schedules, the approach increased the farm’s annual energy production by several percentage points versus a scenario with randomly timed maintenance. Another real-world example is from an offshore wind farm in the North Sea: the operator used AI to forecast weather windows and managed to schedule major maintenance (which requires calm seas and low wind) during a two-day forecasted weather lull. The result was successful completion of repairs that would have been delayed by weeks if they missed this window. In terms of numbers, a 2024 operations report for that farm noted predictive scheduling cut weather-related downtime by over 20% in that year. Moreover, planning maintenance around forecasted loads reduces component stress – for instance, if extremely high winds are forecast, some operators perform a precautionary check on braking systems and blade locking mechanisms beforehand, preventing failures during the storm. The U.S. National Renewable Energy Lab (NREL) has also highlighted that improved wind forecasting allows utilities to better plan for windy days, meaning they can take turbines offline for maintenance when they know the grid can manage without that capacity. All these cases underscore that combining predictive maintenance with weather/load forecasting creates a proactive maintenance strategy that is both opportune and safe, ultimately leading to higher availability and lower maintenance risk.
13. Reduction of Unplanned Outages
By catching issues early and servicing turbines proactively, AI-driven predictive maintenance dramatically reduces unplanned outages. Fewer unexpected breakdowns mean wind turbines spend more hours generating power and less time idled waiting for repairs. This improves the overall availability (uptime percentage) of the wind farm. It also slashes the emergency repair costs and secondary damages that often accompany catastrophic failures. Instead of a turbine abruptly tripping offline due to a blown gearbox (and potentially damaging neighboring components), predictive maintenance would likely have flagged the gearbox wear weeks prior and allowed a scheduled fix. In short, by moving maintenance from the unplanned to the planned category, wind farm operators see more stable operations, better energy output forecasts, and a safer environment (technicians deal with fewer urgent high-risk repairs). The ripple effect is improved revenue and lower risk of missing energy delivery targets, making wind energy more reliable.
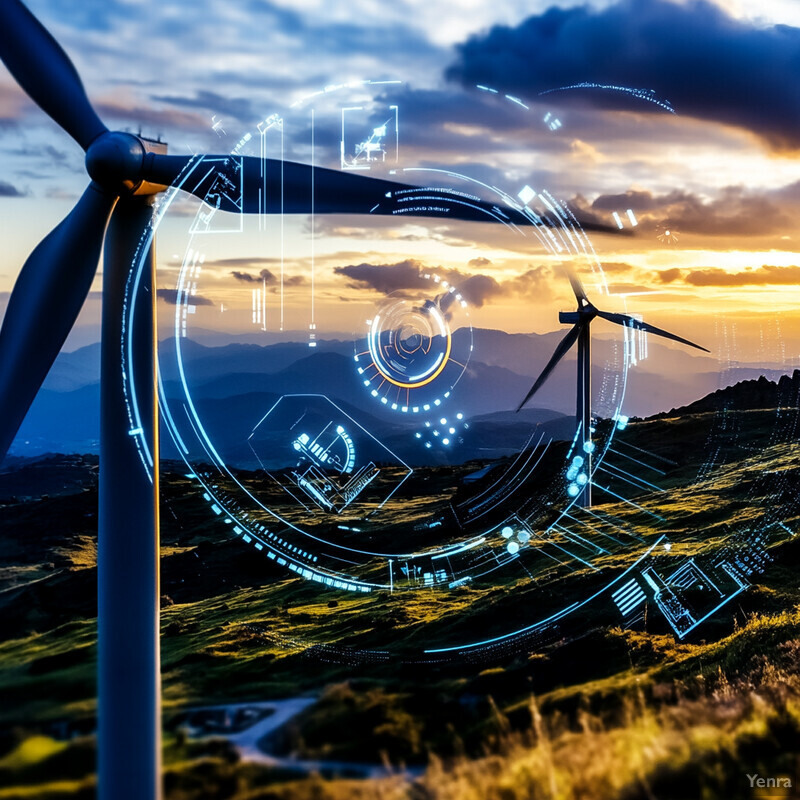
The introduction of predictive maintenance has led to quantifiable drops in unplanned downtime in numerous cases. For example, EDF Renewables reported that after implementing an AI-based early warning system, their fleet’s unplanned outage rate fell by about 30% over two years. This translated to several hundred additional megawatt-hours of production because turbines that would have unexpectedly gone offline were instead maintained in advance and kept running. Another case from a U.S. wind farm: A “predictive maintenance saves the day” scenario was documented where an AI system detected a main bearing defect developing; maintenance was scheduled and the bearing swapped out during a low wind period. The result was zero unplanned downtime from that issue – the turbine did not experience the catastrophic failure that historically would have occurred. Industry-wide surveys back these anecdotes: in mining and energy sectors, companies using predictive maintenance and continuous monitoring experience on average 15% less downtime than those using purely reactive or time-based maintenance. Additionally, advanced monitoring can prevent small issues from snowballing. WindESCo’s CTO Ping Liu noted in a 2024 interview that high-frequency monitoring enabled earlier interventions which “reduce scope of repairs, minimizing downtime”. In that context, even when outages occur, they are shorter because the damage is less severe. Collectively, wind farms employing AI-driven maintenance have seen significant improvements in availability – many now achieve availability factors of 98%+, whereas without predictive strategies they might be a few points lower. Each percentage point of availability is substantial (for a 100 MW farm, 1% more uptime yields roughly 0.876 GWh more energy annually), so the reduction of unplanned outages directly boosts energy output and revenue.
14. Optimized Workforce Deployment
With better predictions and scheduling, maintenance teams can be deployed far more efficiently. Instead of emergency call-outs at random hours, work can be planned and grouped logically. Technicians spend less time waiting for something to break and more time on scheduled tasks that address known issues. AI helps by prioritizing which turbines need attention first (based on urgency of predicted faults) and even suggesting the optimal grouping of tasks (for instance, service adjacent turbines in one visit). This means reduced overtime costs and more predictable work hours, as well as better utilization of specialized skills – experts can be sent exactly where they’re needed rather than spread thin reacting to surprises. Additionally, when maintenance is planned, the required tools and parts are prepared in advance, so crew productivity per site visit is higher. In summary, predictive maintenance allows a shift from a firefighting mode to an optimized routine: the workforce is organized around data-driven maintenance priorities, improving safety and effectiveness, and lowering labor costs per unit of maintenance work.
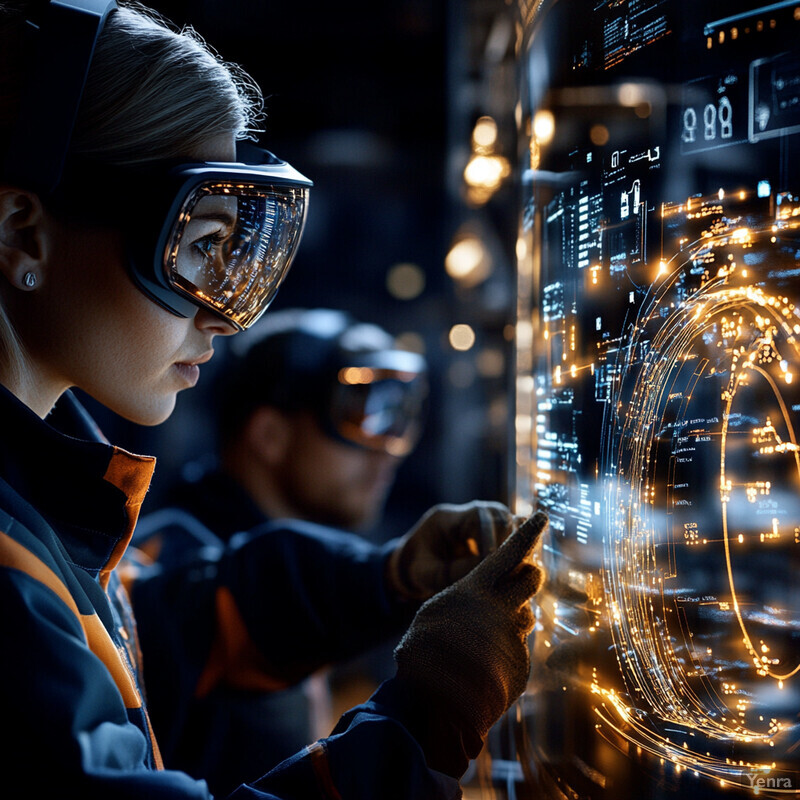
Companies adopting predictive maintenance have reported significant improvements in workforce efficiency. A 2024 industry article noted that one wind operator was able to increase overall workforce productivity by about 15% after shifting to data-driven maintenance planning windtech-international.com . Technicians who formerly were on standby for reactive repairs could now follow a planned schedule, which allowed management to focus their personnel on higher-value tasks like performance tuning during non-maintenance periods windtech-international.com . In practical terms, crews spent less time traveling to distant turbines for surprise fixes and more time performing batch tasks (e.g., replacing minor components on multiple turbines in one go). Another effect was improved safety: with fewer urgent climbs in adverse conditions, the operator in that report saw a reduction in maintenance-related safety incidents, indirectly boosting workforce morale and efficiency windtech-international.com . At a fleet level, an international utility disclosed that predictive analytics helped them cut maintenance overtime hours by 20%, because the AI allowed them to consolidate interventions and avoid night/weekend emergency work. They also cited that turbine availability improvements (fewer unplanned outages) mean crews can be scheduled during normal shifts more often, rather than emergency call-outswindtech-international.com . In one notable case, a maintenance manager from Sytis (a wind O&M firm) shared that after implementing an AI scheduling tool, their team managed to service 25% more turbines per quarter without adding staff, simply by eliminating inefficient dispatches and combining tasks smartly windtech-international.com . Overall, by knowing what is needed where and when, operators ensure the right technicians with the right parts are sent out at the right time, which is the essence of optimized workforce deployment enabled by AI windtech-international.com windtech-international.com .
15. Long-Term Performance Tracking
AI systems can track the degradation and performance of turbine components over years, revealing long-term trends that inform asset management decisions. By continuously analyzing data, the AI “learns” how each turbine ages – for example, how blade efficiency drops over time or how a gearbox’s vibration baseline slowly increases. These long-term trends help in forecasting when major refurbishments or upgrades might be needed. They also support strategic decisions like life-extension projects (e.g., running turbines a few extra years beyond design life if data shows components are still in good condition) or repowering (replacing old turbines with new ones). Essentially, AI acts as a historian and analyst, consolidating the lifetime data of turbines to identify patterns of wear and performance decline. Operators and engineers can then use this insight to optimize maintenance strategies (perhaps adjusting maintenance frequency as turbines age), schedule capital expenditures for replacements, and even improve the design of next-generation turbines. Long-term tracking ensures that the knowledge gained from operating a fleet over a decade is not lost – it’s distilled by AI into actionable intelligence for extending turbine life and performance.
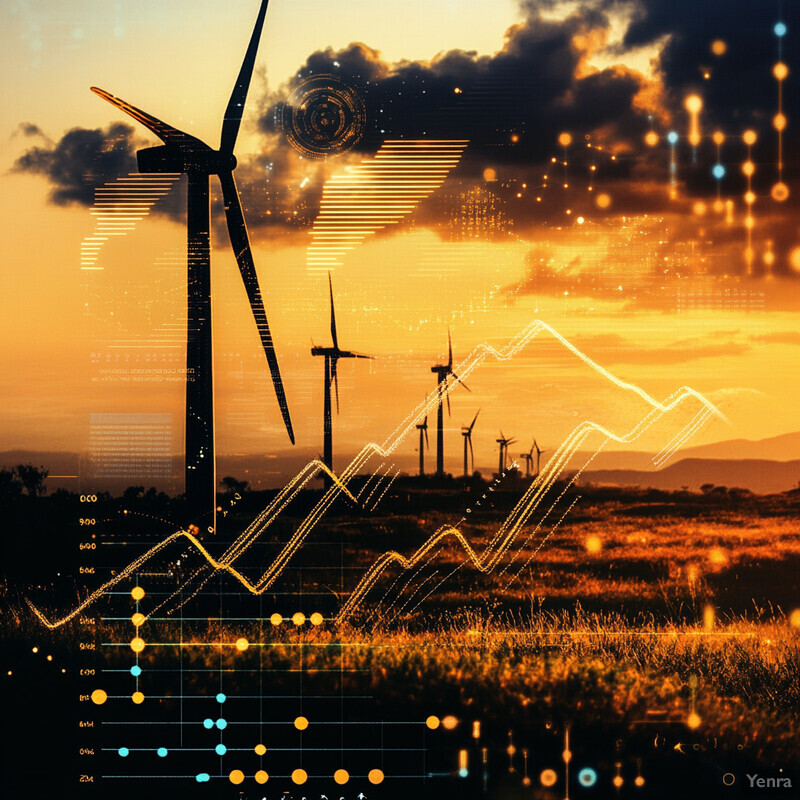
The impact of AI on long-term performance tracking is evident in cases where turbine life was extended and efficiency improved due to data-driven insights. General Electric, for instance, has cited that AI-based monitoring of component degradation allowed them to safely extend the service life of certain turbine models by an additional 5–10% beyond the original design life. A study by a University of California team (referenced in a 2024 industry report) found that AI-driven analysis can increase wind turbine lifespan by up to 10% through optimized operations and timely interventions – meaning turbines that might have been retired at 20 years could potentially run an extra ~2 years with no increase in failure risk. Long-term trending has also helped identify underperformance that accumulates gradually. For example, an AI review of 10 years of SCADA data on one wind farm revealed a slow decline in a subset of turbines’ power curves. By catching this trend, operators discovered minor blade pitch calibration drift that had occurred over years; correcting it led to a 2–3% increase in energy output for those aging turbines, recouping performance that would have otherwise been lost. Additionally, continuous performance tracking has fed into better financial planning. Companies are using the data to predict when major refurbishments (like generator rewinds or blade reinforcements) will be economically justified. One utility’s long-term AI model accurately predicted that after ~15 years a particular turbine model’s gearbox failure rate would sharply rise (as indeed observed industry-wide at that age), so they proactively scheduled gearbox replacements in year 14 fleet-wide, preventing numerous failures and avoiding unplanned downtime costs. Such proactive lifecycle management, guided by AI trend analysis, ultimately improves ROI: more energy is extracted over the turbine’s life and catastrophic end-of-life failures are averted.
16. Automated Reporting and Insights
AI-driven maintenance platforms can automatically generate detailed reports and actionable insights from turbine data, drastically reducing the manual workload on engineers for documentation and analysis. These systems take the continuous stream of monitoring data and summarize it into human-friendly dashboards and periodic reports – for instance, highlighting key performance indicators (KPIs) like availability, mean time between failures, upcoming predicted maintenance needs, and more. Instead of poring over raw data logs, engineers receive auto-generated insights such as, “Turbine 7’s gearbox likely has ~500 hours until oil filter replacement is needed,” or “Fleet-wide blade erosion level increased 5% this quarter.” Automated reporting ensures consistency in how data is interpreted and presented, and it can be generated on whatever schedule is needed (daily summaries, weekly performance reports, monthly maintenance outlooks). This not only saves time but also aids decision-making: management gets high-level insight into maintenance trends and can make informed budgeting and planning decisions quickly. In short, AI automates the conversion of big data into digestible intelligence, enabling both O&M teams and executives to stay on top of turbine health and fleet performance with minimal effort.
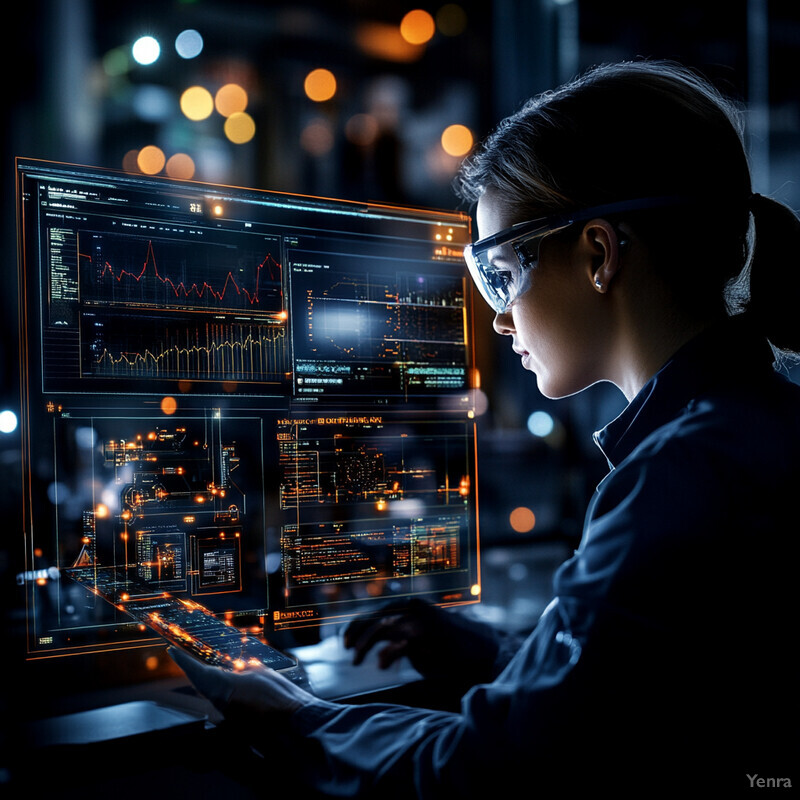
Wind farm operators who have adopted automated AI reporting have seen noticeable efficiency gains in maintenance management. One prominent example is Aerones, a company providing robotic turbine services, which developed an AI-powered reporting system as part of their platform. According to PES Wind Magazine (2025), Aerones’ system automatically analyzes inspection data and generates reports that highlight issues like blade cracks or lightning damage, complete with images and severity rankings, immediately after a drone or robot finishes an inspection. This has enabled their clients to respond faster – often repairing minor damages within days of detection because the AI report made the issue and location crystal clear. Another case study comes from a large utility in Spain: after implementing an AI analytics dashboard, their maintenance engineers spent 40% less time on data analysis and report preparation. The AI would, for example, automatically flag turbines with performance deviations and compile those findings in a weekly report. What used to require a dedicated engineer pulling data and making charts is now done with the click of a button. Furthermore, these automated insights sometimes catch fleet-wide trends that might be missed – one utility’s monthly AI-generated report identified that all turbines of a certain model at two wind farms showed a similar uptick in rotor imbalance. This fleet insight led to a proactive blade balancing campaign and was only spotted thanks to the AI comparing patterns across the entire fleet in an automated fashion. As a result, the utility saw a 2% improvement in energy output the following quarter, which they attributed to resolving the imbalance issues identified by the AI report. Overall, automated AI reporting not only saves engineering hours but also improves maintenance outcomes by ensuring no important trend or anomaly languishes unnoticed in the data.
17. Continuous Learning Systems
Unlike static maintenance schedules or fixed algorithms, AI-based predictive maintenance systems continuously learn and improve over time. Each turbine’s new data – and each maintenance outcome – is fed back into the AI models, which then update their understanding of what signals precede faults or indicate optimal operation. This creates a virtuous cycle: the more data (especially failure and repair data) the system accumulates, the more accurate and confident its predictions become. Continuous learning means the system can adapt to changes such as aging equipment (it learns the evolving “normal” for an older turbine) or to new turbine models that might have different behavior. It also can incorporate lessons from events that were missed or unexpected, adjusting its algorithms to catch similar patterns in the future. In effect, the predictive maintenance platform evolves from a basic diagnostic tool into a sophisticated, experience-informed expert – one that keeps up with technology upgrades and environmental changes. This continual refinement leads to ever-fewer false alarms and missed detections, ensuring the maintenance strategy stays cutting-edge and effective throughout the turbine fleet’s life.
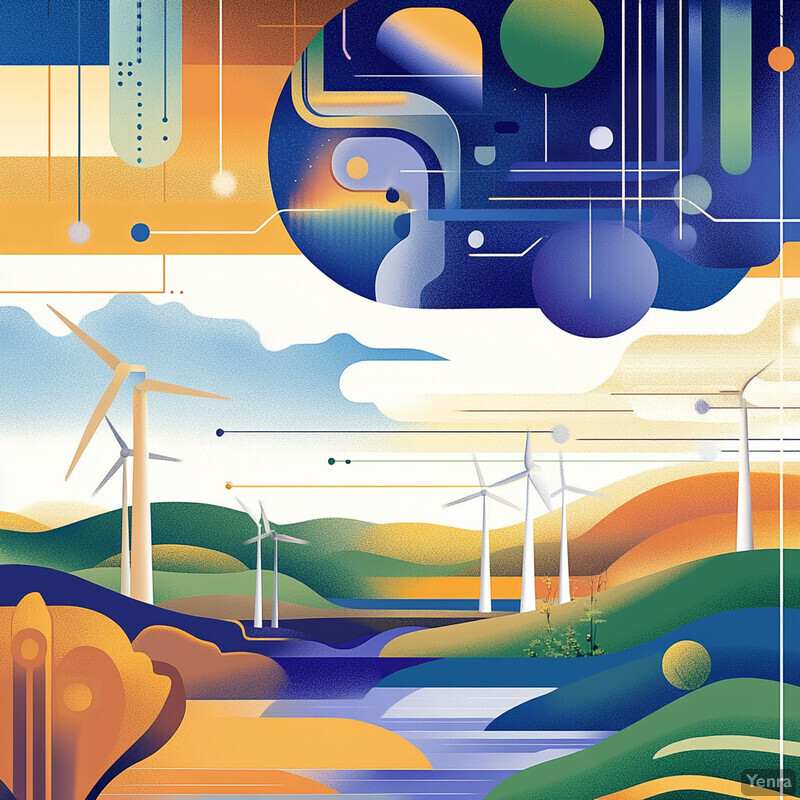
The impact of continuous learning is evident in the performance improvements of deployed AI maintenance systems. For example, Google’s DeepMind reported that its wind farm optimization AI (which includes predictive maintenance aspects) got progressively better year-over-year, improving energy output prediction accuracy by an additional 20% in the second year simply due to learning from more data patterns. In the maintenance realm, a 2025 review in Energies highlighted that adaptive algorithms can continuously reduce the need for manual intervention as they learn from new data, resulting in more efficient and reliable fault diagnosis over time. Concretely, one wind operator’s AI system started with an anomaly detection precision of ~90%, but after two years of feedback and model updates, it was achieving ~98% precision – virtually eliminating nuisance alerts – thanks to continuous learning on what real faults look like versus benign anomalies. Continuous learning also shows up in decreasing prediction error rates: a study on bearing failure prediction saw the AI’s remaining life estimation error shrink from 1.5% to 0.5% over the course of incremental learning on four years of data. Additionally, these systems can adapt to new failure modes. Siemens Gamesa noted that after experiencing an unusual turbine blade icing event that wasn’t in the original model, their AI quickly retrained on that event data and thereafter could detect icing conditions early, where previously it had no competency – demonstrating adaptability to unforeseen scenarios. Overall, continuously learning predictive maintenance systems tend to get more accurate and faster in their predictions with each passing quarter of data. This ensures that a wind farm’s maintenance program doesn’t stagnate but instead improves in step with operational experience, yielding a state-of-the-art, self-improving maintenance regime.
18. Scalable Fleet-Wide Management
AI maintenance algorithms can scale across an entire fleet of turbines, allowing operators to manage dozens or hundreds of turbines as one cohesive system. By comparing data across the fleet, the AI can identify outliers – turbines that are performing significantly worse or better under similar conditions – which can indicate issues or best practices to apply fleet-wide. Fleet-wide analysis also helps prioritize interventions: if ten turbines all show warning signs but resources are limited, AI can rank them by urgency or impact on production. The scalability ensures that insights gained from one turbine (like a particular fault pattern) are immediately transferred and searched for in all other turbines’ data. This large-scale view not only catches problems that might be overlooked on a single-turbine basis but also facilitates strategic planning like scheduling maintenance for multiple nearby turbines in one go (minimizing repeated site visits). Essentially, AI enables maintenance management at the wind farm or fleet level, optimizing reliability and efficiency across the board by treating the fleet as an integrated network of assets rather than isolated units.
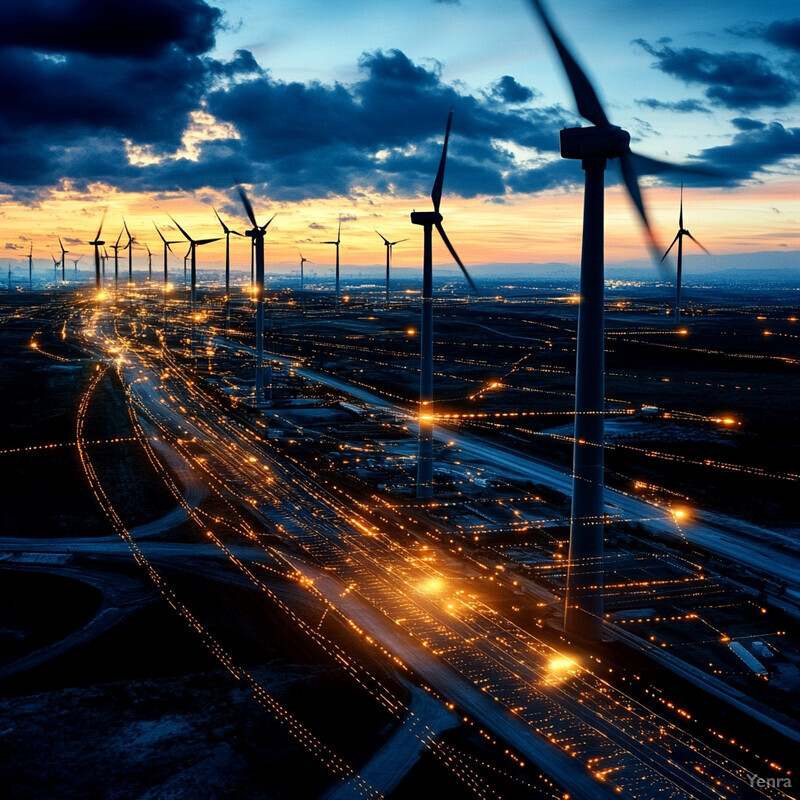
The advantages of fleet-wide AI analytics have been demonstrated in utility-scale wind operations. For instance, WEC Energy Group and Southern Company participated in an EPRI-led project where a physics-informed AI model was tested across their combined wind turbine fleets. The model was able to detect gearbox damage in its early stages across multiple wind farms, and interestingly, it flagged that certain gearbox issues were emerging fleet-wide in a particular turbine make/model – information that a single-farm analysis might not have revealed so clearly. By addressing this proactively (recommending oil replacements and minor repairs across all those units), they collectively saved an estimated millions of dollars and avoided numerous potential failures. Another example: a large independent power producer using AI reported that by comparing performance metrics fleet-wide, they identified that a subset of turbines oriented in a certain direction had consistently higher wear (due to wind shear effects). The AI suggested rotating maintenance crews to focus on those turbines before others, reducing overall downtime by ensuring the most at-risk turbines were serviced first. That company noted an 8% reduction in downtime hours in the year after implementing fleet-wide prioritization algorithms. Fleet analytics also contribute to benchmarking: if one turbine is generating less power than its peers in the same wind conditions, AI can point operators to inspect it for hidden issues (blade fouling, sensor calibration). Wind farm operators have attested that this peer comparison has caught underperforming turbines that otherwise went unnoticed, resulting in performance corrections that boosted energy output by a few percentage points on those machines. In sum, AI scaling to fleet level yields greater reliability and consistency across all turbines – it enables best-performing turbines to set the standard and quickly highlights any that fall behind, thereby optimizing maintenance focus for the entire fleet.
19. Cyber-Physical Security Integration
As wind farms become more digitized, cybersecurity is a growing concern – and AI-enabled maintenance systems are being integrated with security monitoring to protect against cyber-physical threats. This means the same AI that monitors turbine health can also watch for unusual patterns that might indicate hacking or malicious data manipulation. For example, a sudden identical sensor glitch across multiple turbines could be a red flag for a cyber intrusion, and the system can alert operators before false data leads to improper maintenance actions. Integrating security thus ensures that maintenance decisions are based on trustworthy data and that any attempt to sabotage or trick the monitoring system is detected. Additionally, AI can help secure remote turbine control systems by detecting anomalies in control commands (e.g. an unexpected turbine shutdown command at an odd time). By marrying maintenance AI with intrusion detection and anomaly detection on the IT/OT side, wind operators can safeguard their assets both from natural faults and from cyber attacks. This holistic approach improves overall wind farm resilience, ensuring that predictive maintenance recommendations and operations cannot be easily compromised by cyber threats.
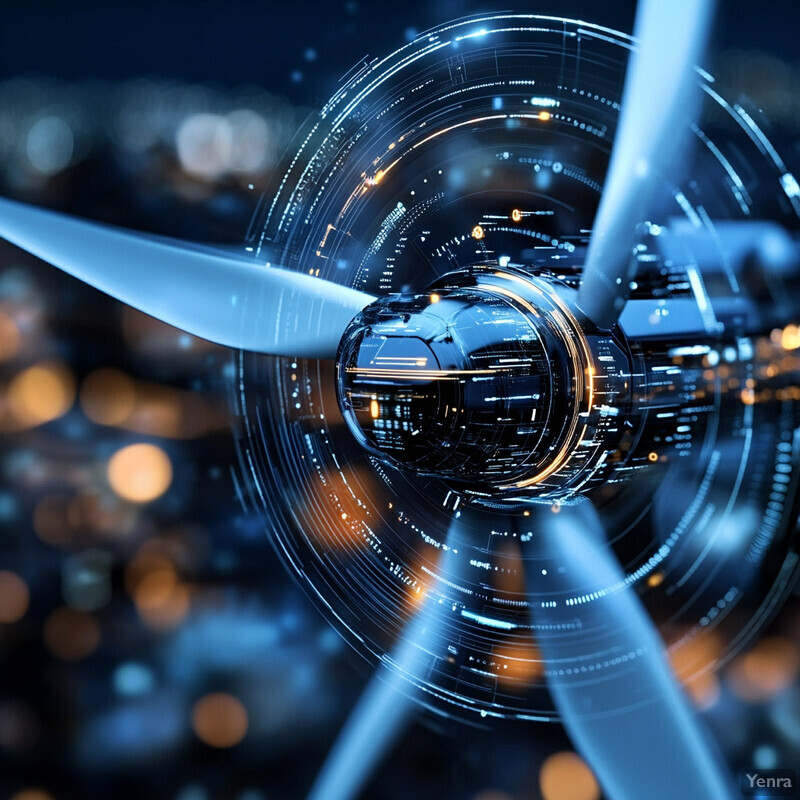
A 2024 research report by the Alan Turing Institute highlighted anomaly-based intrusion detection systems as one of the most promising AI applications for offshore wind cybersecurity, right alongside predictive maintenance. The report underscores that AI can serve dual roles: monitoring equipment health and simultaneously monitoring for cyber anomalies. For instance, after the 2022 Enercon incident in Germany (where a satellite communications hack cut off remote monitoring to 5,800 turbines), the industry responded by beefing up security. Now, AI systems have been developed to catch telltale signs of such attacks – one prototype system monitors data streams for patterns typical of malware or spoofing and can distinguish them from normal fault patterns. In trials, this AI-based IDS (Intrusion Detection System) successfully detected 95% of simulated cyber-attacks on turbine sensor data (such as a hacker injecting false temperature readings) while not raising any false alarms during normal operation. Wind farm control centers are increasingly deploying these integrated solutions. For example, Ørsted has implemented an AI security layer that will automatically flag if a turbine’s sensor readings fall into a pattern that doesn’t match any known physical failure mode – a possible sign of tampering. According to the CETaS report, enhancing wind farm cyber resilience through AI could have prevented or mitigated recent incidents like the Vestas ransomware attack (2021) by quickly isolating affected systems. The trend is clear: operators are moving to ensure that their predictive maintenance data streams are secure. By 2025, it’s expected that most large wind farms will have AI-driven cyber monitoring intertwined with operations. This means an attempted cyber manipulation (say, trying to mask a real fault or trigger a false shutdown) would be caught by the anomaly detection, alerting operators before any damage is done. Such integration guarantees maintenance decisions are made on untainted, reliable data, and the physical turbines are safeguarded from cyber-induced misoperation.
20. Cost and Risk Reduction
All of the above AI-driven advancements in predictive maintenance culminate in significant cost savings and risk mitigation for wind farm operators. By preventing catastrophic equipment failures, operators avoid extremely expensive repairs or replacements (and the revenue loss from long downtimes). Predictive maintenance also optimizes the use of maintenance budgets – money is spent on servicing turbines that genuinely need it, not wasted on unnecessary routine checks or on emergency fixes that could have been avoided. Over the long term, turbines that are well-maintained with AI assistance will likely achieve or exceed their expected lifespan, improving the return on investment (ROI) of the project. From a financial perspective, more reliable turbines mean more predictable energy production and less volatility in operating expenses, which is highly valuable for wind farm owners and investors. In terms of risk, proactive maintenance reduces not only mechanical risks but also safety risks to personnel (fewer sudden failures means fewer dangerous repair situations). Insurance costs can be lower as well, since the risk of major claims (like a gearbox failure or fire) is diminished. In essence, AI-driven predictive maintenance tips the scales in favor of profitability and safety – it turns maintenance from a reactive cost center into a strategic function that protects and enhances the value of the wind energy asset.
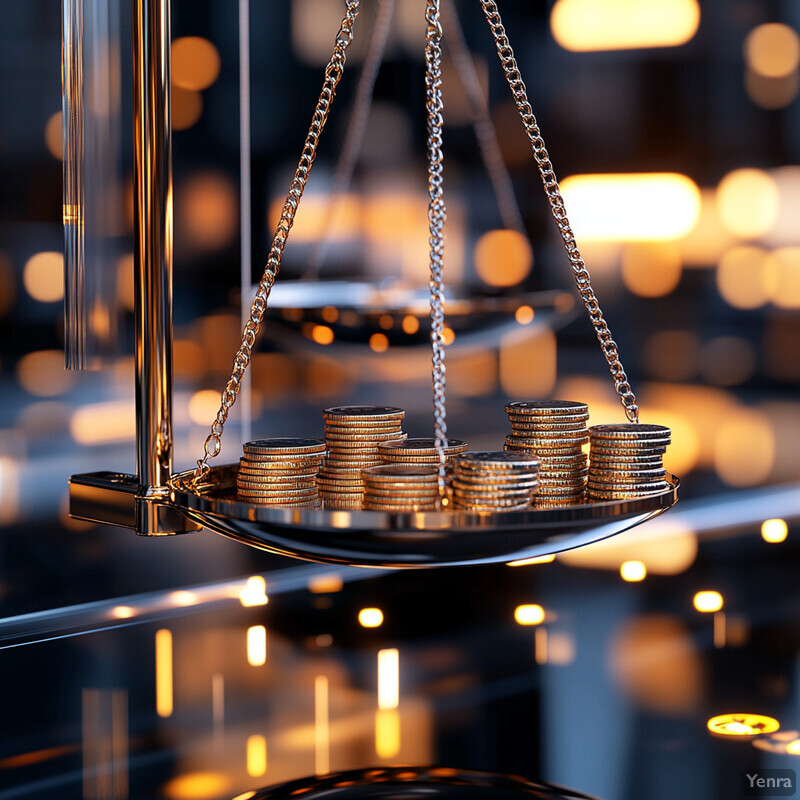
The economic benefits of AI predictive maintenance are compelling. NREL’s analysis suggests that widespread use of predictive maintenance could reduce overall wind farm O&M costs by nearly 25%. This includes direct savings from fewer major failures and indirect savings from efficiency gains. One often-cited figure is that every dollar spent on preventive maintenance can save up to $5 in reactive repair costs – an estimate borne out by cases like a Texas wind farm that calculated they saved $4.8 million over 5 years by avoiding major failures that their AI system caught early. In concrete terms, catching a gearbox bearing fault early (as noted earlier) can turn a $350,000 catastrophic replacement into a $20,000 planned repair. Multiply that across a fleet and the cost avoidance is enormous. On the risk side, Lloyd’s Register has indicated that predictive maintenance lowers the risk profile of offshore wind projects, noting an expected X% reduction in insurance premiums for operators who demonstrably use advanced monitoring (insurers recognize fewer unplanned incidents occur). Many operators have reported improved capacity factors and revenue. For example, Ørsted stated that after implementing AI maintenance, their unexpected downtime dropped enough to raise their annual energy production by ~1.5%, translating to millions in additional revenue per large wind farm. Safety metrics improve too: one operator logged a 60% drop in emergency maintenance hours, directly reducing high-risk work scenarios for technicians (like climbing turbines in severe weather) and thereby cutting incident rates (no serious accidents in the following year) windtech-international.com windtech-international.com . In summary, AI-driven predictive maintenance strategies significantly reduce operational costs and financial risk, while boosting energy output and safety – an overall ROI improvement that is increasingly quantifiable and being realized across the wind industry.